
An official website of the United States government
The .gov means it’s official. Federal government websites often end in .gov or .mil. Before sharing sensitive information, make sure you’re on a federal government site.
The site is secure. The https:// ensures that you are connecting to the official website and that any information you provide is encrypted and transmitted securely.
- Publications
- Account settings

Trending Articles
- Epinephrine promotes breast cancer metastasis through a ubiquitin-specific peptidase 22-mediated lipolysis circuit. Zhou Y, et al. Sci Adv. 2024. PMID: 39151008 Free PMC article.
- Deciphering the causes of sporadic late-onset cerebellar ataxias: a prospective study with implications for diagnostic work. Gebus O, et al. J Neurol. 2017. PMID: 28478596
- Association of Treatment Intensity and Adherence to Lipid-Lowering Therapy with Major Adverse Cardiovascular Events Among Post-MI Patients in Germany. Ahrens I, et al. Adv Ther. 2021. PMID: 33830461 Free PMC article.
- Schwann cell-secreted PGE 2 promotes sensory neuron excitability during development. Kantarci H, et al. Cell. 2024. PMID: 39142281
- Amelioration of nonalcoholic fatty liver disease by inhibiting the deubiquitylating enzyme RPN11. Zhou B, et al. Cell Metab. 2024. PMID: 39146936
Latest Literature
- Am J Clin Nutr (1)
- J Biol Chem (1)
- J Immunol (6)
- PLoS One (47)
- Pediatrics (2)
- Proc Natl Acad Sci U S A (20)
NCBI Literature Resources
MeSH PMC Bookshelf Disclaimer
The PubMed wordmark and PubMed logo are registered trademarks of the U.S. Department of Health and Human Services (HHS). Unauthorized use of these marks is strictly prohibited.
Loading metrics
Open Access
Peer-reviewed
Research Article
Assessing the impact of healthcare research: A systematic review of methodological frameworks
Roles Conceptualization, Data curation, Formal analysis, Funding acquisition, Investigation, Methodology, Validation, Writing – original draft, Writing – review & editing
Affiliation Centre for Patient Reported Outcomes Research, Institute of Applied Health Research, College of Medical and Dental Sciences, University of Birmingham, Birmingham, United Kingdom

Roles Conceptualization, Formal analysis, Funding acquisition, Methodology, Project administration, Supervision, Validation, Writing – review & editing
* E-mail: [email protected]
Roles Data curation, Formal analysis, Methodology, Validation, Writing – review & editing
Roles Formal analysis, Methodology, Supervision, Validation, Writing – review & editing
- Samantha Cruz Rivera,
- Derek G. Kyte,
- Olalekan Lee Aiyegbusi,
- Thomas J. Keeley,
- Melanie J. Calvert
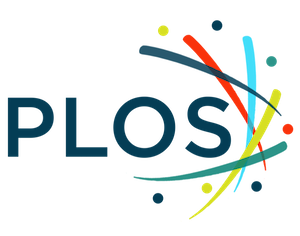
- Published: August 9, 2017
- https://doi.org/10.1371/journal.pmed.1002370
- Reader Comments
Increasingly, researchers need to demonstrate the impact of their research to their sponsors, funders, and fellow academics. However, the most appropriate way of measuring the impact of healthcare research is subject to debate. We aimed to identify the existing methodological frameworks used to measure healthcare research impact and to summarise the common themes and metrics in an impact matrix.
Methods and findings
Two independent investigators systematically searched the Medical Literature Analysis and Retrieval System Online (MEDLINE), the Excerpta Medica Database (EMBASE), the Cumulative Index to Nursing and Allied Health Literature (CINAHL+), the Health Management Information Consortium, and the Journal of Research Evaluation from inception until May 2017 for publications that presented a methodological framework for research impact. We then summarised the common concepts and themes across methodological frameworks and identified the metrics used to evaluate differing forms of impact. Twenty-four unique methodological frameworks were identified, addressing 5 broad categories of impact: (1) ‘primary research-related impact’, (2) ‘influence on policy making’, (3) ‘health and health systems impact’, (4) ‘health-related and societal impact’, and (5) ‘broader economic impact’. These categories were subdivided into 16 common impact subgroups. Authors of the included publications proposed 80 different metrics aimed at measuring impact in these areas. The main limitation of the study was the potential exclusion of relevant articles, as a consequence of the poor indexing of the databases searched.
Conclusions
The measurement of research impact is an essential exercise to help direct the allocation of limited research resources, to maximise research benefit, and to help minimise research waste. This review provides a collective summary of existing methodological frameworks for research impact, which funders may use to inform the measurement of research impact and researchers may use to inform study design decisions aimed at maximising the short-, medium-, and long-term impact of their research.
Author summary
Why was this study done.
- There is a growing interest in demonstrating the impact of research in order to minimise research waste, allocate resources efficiently, and maximise the benefit of research. However, there is no consensus on which is the most appropriate tool to measure the impact of research.
- To our knowledge, this review is the first to synthesise existing methodological frameworks for healthcare research impact, and the associated impact metrics by which various authors have proposed impact should be measured, into a unified matrix.
What did the researchers do and find?
- We conducted a systematic review identifying 24 existing methodological research impact frameworks.
- We scrutinised the sample, identifying and summarising 5 proposed impact categories, 16 impact subcategories, and over 80 metrics into an impact matrix and methodological framework.
What do these findings mean?
- This simplified consolidated methodological framework will help researchers to understand how a research study may give rise to differing forms of impact, as well as in what ways and at which time points these potential impacts might be measured.
- Incorporating these insights into the design of a study could enhance impact, optimizing the use of research resources.
Citation: Cruz Rivera S, Kyte DG, Aiyegbusi OL, Keeley TJ, Calvert MJ (2017) Assessing the impact of healthcare research: A systematic review of methodological frameworks. PLoS Med 14(8): e1002370. https://doi.org/10.1371/journal.pmed.1002370
Academic Editor: Mike Clarke, Queens University Belfast, UNITED KINGDOM
Received: February 28, 2017; Accepted: July 7, 2017; Published: August 9, 2017
Copyright: © 2017 Cruz Rivera et al. This is an open access article distributed under the terms of the Creative Commons Attribution License , which permits unrestricted use, distribution, and reproduction in any medium, provided the original author and source are credited.
Data Availability: All relevant data are within the paper and supporting files.
Funding: Funding was received from Consejo Nacional de Ciencia y Tecnología (CONACYT). The funders had no role in study design, data collection and analysis, decision to publish, or preparation of the manuscript ( http://www.conacyt.mx/ ).
Competing interests: I have read the journal's policy and the authors of this manuscript have the following competing interests: MJC has received consultancy fees from Astellas and Ferring pharma and travel fees from the European Society of Cardiology outside the submitted work. TJK is in full-time paid employment for PAREXEL International.
Abbreviations: AIHS, Alberta Innovates—Health Solutions; CAHS, Canadian Academy of Health Sciences; CIHR, Canadian Institutes of Health Research; CINAHL+, Cumulative Index to Nursing and Allied Health Literature; EMBASE, Excerpta Medica Database; ERA, Excellence in Research for Australia; HEFCE, Higher Education Funding Council for England; HMIC, Health Management Information Consortium; HTA, Health Technology Assessment; IOM, Impact Oriented Monitoring; MDG, Millennium Development Goal; NHS, National Health Service; MEDLINE, Medical Literature Analysis and Retrieval System Online; PHC RIS, Primary Health Care Research & Information Service; PRISMA, Preferred Reporting Items for Systematic Reviews and Meta-Analyses; PROM, patient-reported outcome measures; QALY, quality-adjusted life year; R&D, research and development; RAE, Research Assessment Exercise; REF, Research Excellence Framework; RIF, Research Impact Framework; RQF, Research Quality Framework; SDG, Sustainable Development Goal; SIAMPI, Social Impact Assessment Methods for research and funding instruments through the study of Productive Interactions between science and society
Introduction
In 2010, approximately US$240 billion was invested in healthcare research worldwide [ 1 ]. Such research is utilised by policy makers, healthcare providers, and clinicians to make important evidence-based decisions aimed at maximising patient benefit, whilst ensuring that limited healthcare resources are used as efficiently as possible to facilitate effective and sustainable service delivery. It is therefore essential that this research is of high quality and that it is impactful—i.e., it delivers demonstrable benefits to society and the wider economy whilst minimising research waste [ 1 , 2 ]. Research impact can be defined as ‘any identifiable ‘benefit to, or positive influence on the economy, society, public policy or services, health, the environment, quality of life or academia’ (p. 26) [ 3 ].
There are many purported benefits associated with the measurement of research impact, including the ability to (1) assess the quality of the research and its subsequent benefits to society; (2) inform and influence optimal policy and funding allocation; (3) demonstrate accountability, the value of research in terms of efficiency and effectiveness to the government, stakeholders, and society; and (4) maximise impact through better understanding the concept and pathways to impact [ 4 – 7 ].
Measuring and monitoring the impact of healthcare research has become increasingly common in the United Kingdom [ 5 ], Australia [ 5 ], and Canada [ 8 ], as governments, organisations, and higher education institutions seek a framework to allocate funds to projects that are more likely to bring the most benefit to society and the economy [ 5 ]. For example, in the UK, the 2014 Research Excellence Framework (REF) has recently been used to assess the quality and impact of research in higher education institutions, through the assessment of impact cases studies and selected qualitative impact metrics [ 9 ]. This is the first initiative to allocate research funding based on the economic, societal, and cultural impact of research, although it should be noted that research impact only drives a proportion of this allocation (approximately 20%) [ 9 ].
In the UK REF, the measurement of research impact is seen as increasingly important. However, the impact element of the REF has been criticised in some quarters [ 10 , 11 ]. Critics deride the fact that REF impact is determined in a relatively simplistic way, utilising researcher-generated case studies, which commonly attempt to link a particular research outcome to an associated policy or health improvement despite the fact that the wider literature highlights great diversity in the way research impact may be demonstrated [ 12 , 13 ]. This led to the current debate about the optimal method of measuring impact in the future REF [ 10 , 14 ]. The Stern review suggested that research impact should not only focus on socioeconomic impact but should also include impact on government policy, public engagement, academic impacts outside the field, and teaching to showcase interdisciplinary collaborative impact [ 10 , 11 ]. The Higher Education Funding Council for England (HEFCE) has recently set out the proposals for the REF 2021 exercise, confirming that the measurement of such impact will continue to form an important part of the process [ 15 ].
With increasing pressure for healthcare research to lead to demonstrable health, economic, and societal impact, there is a need for researchers to understand existing methodological impact frameworks and the means by which impact may be quantified (i.e., impact metrics; see Box 1 , 'Definitions’) to better inform research activities and funding decisions. From a researcher’s perspective, understanding the optimal pathways to impact can help inform study design aimed at maximising the impact of the project. At the same time, funders need to understand which aspects of impact they should focus on when allocating awards so they can make the most of their investment and bring the greatest benefit to patients and society [ 2 , 4 , 5 , 16 , 17 ].
Box 1. Definitions
- Research impact: ‘any identifiable benefit to, or positive influence on, the economy, society, public policy or services, health, the environment, quality of life, or academia’ (p. 26) [ 3 ].
- Methodological framework: ‘a body of methods, rules and postulates employed by a particular procedure or set of procedures (i.e., framework characteristics and development)’ [ 18 ].
- Pathway: ‘a way of achieving a specified result; a course of action’ [ 19 ].
- Quantitative metrics: ‘a system or standard of [quantitative] measurement’ [ 20 ].
- Narrative metrics: ‘a spoken or written account of connected events; a story’ [ 21 ].
Whilst previous researchers have summarised existing methodological frameworks and impact case studies [ 4 , 22 – 27 ], they have not summarised the metrics for use by researchers, funders, and policy makers. The aim of this review was therefore to (1) identify the methodological frameworks used to measure healthcare research impact using systematic methods, (2) summarise common impact themes and metrics in an impact matrix, and (3) provide a simplified consolidated resource for use by funders, researchers, and policy makers.
Search strategy and selection criteria
Initially, a search strategy was developed to identify the available literature regarding the different methods to measure research impact. The following keywords: ‘Impact’, ‘Framework’, and ‘Research’, and their synonyms, were used during the search of the Medical Literature Analysis and Retrieval System Online (MEDLINE; Ovid) database, the Excerpta Medica Database (EMBASE), the Health Management Information Consortium (HMIC) database, and the Cumulative Index to Nursing and Allied Health Literature (CINAHL+) database (inception to May 2017; see S1 Appendix for the full search strategy). Additionally, the nonindexed Journal of Research Evaluation was hand searched during the same timeframe using the keyword ‘Impact’. Other relevant articles were identified through 3 Internet search engines (Google, Google Scholar, and Google Images) using the keywords ‘Impact’, ‘Framework’, and ‘Research’, with the first 50 results screened. Google Images was searched because different methodological frameworks are summarised in a single image and can easily be identified through this search engine. Finally, additional publications were sought through communication with experts.
Following Preferred Reporting Items for Systematic Reviews and Meta-Analyses (PRISMA) guidelines (see S1 PRISMA Checklist ), 2 independent investigators systematically screened for publications describing, evaluating, or utilising a methodological research impact framework within the context of healthcare research [ 28 ]. Papers were eligible if they included full or partial methodological frameworks or pathways to research impact; both primary research and systematic reviews fitting these criteria were included. We included any methodological framework identified (original or modified versions) at the point of first occurrence. In addition, methodological frameworks were included if they were applicable to the healthcare discipline with no need of modification within their structure. We defined ‘methodological framework’ as ‘a body of methods, rules and postulates employed by a particular procedure or set of procedures (i.e., framework characteristics and development)’ [ 18 ], whereas we defined ‘pathway’ as ‘a way of achieving a specified result; a course of action’ [ 19 ]. Studies were excluded if they presented an existing (unmodified) methodological framework previously available elsewhere, did not explicitly describe a methodological framework but rather focused on a single metric (e.g., bibliometric analysis), focused on the impact or effectiveness of interventions rather than that of the research, or presented case study data only. There were no language restrictions.
Data screening
Records were downloaded into Endnote (version X7.3.1), and duplicates were removed. Two independent investigators (SCR and OLA) conducted all screening following a pilot aimed at refining the process. The records were screened by title and abstract before full-text articles of potentially eligible publications were retrieved for evaluation. A full-text screening identified the publications included for data extraction. Discrepancies were resolved through discussion, with the involvement of a third reviewer (MJC, DGK, and TJK) when necessary.
Data extraction and analysis
Data extraction occurred after the final selection of included articles. SCR and OLA independently extracted details of impact methodological frameworks, the country of origin, and the year of publication, as well as the source, the framework description, and the methodology used to develop the framework. Information regarding the methodology used to develop each methodological framework was also extracted from framework webpages where available. Investigators also extracted details regarding each framework’s impact categories and subgroups, along with their proposed time to impact (‘short-term’, ‘mid-term’, or ‘long-term’) and the details of any metrics that had been proposed to measure impact, which are depicted in an impact matrix. The structure of the matrix was informed by the work of M. Buxton and S. Hanney [ 2 ], P. Buykx et al. [ 5 ], S. Kuruvila et al. [ 29 ], and A. Weiss [ 30 ], with the intention of mapping metrics presented in previous methodological frameworks in a concise way. A consensus meeting with MJC, DGK, and TJK was held to solve disagreements and finalise the data extraction process.
Included studies
Our original search strategy identified 359 citations from MEDLINE (Ovid), EMBASE, CINAHL+, HMIC, and the Journal of Research Evaluation, and 101 citations were returned using other sources (Google, Google Images, Google Scholar, and expert communication) (see Fig 1 ) [ 28 ]. In total, we retrieved 54 full-text articles for review. At this stage, 39 articles were excluded, as they did not propose new or modified methodological frameworks. An additional 15 articles were included following the backward and forward citation method. A total of 31 relevant articles were included in the final analysis, of which 24 were articles presenting unique frameworks and the remaining 7 were systematic reviews [ 4 , 22 – 27 ]. The search strategy was rerun on 15 May 2017. A further 19 publications were screened, and 2 were taken forward to full-text screening but were ineligible for inclusion.
- PPT PowerPoint slide
- PNG larger image
- TIFF original image
https://doi.org/10.1371/journal.pmed.1002370.g001
Methodological framework characteristics
The characteristics of the 24 included methodological frameworks are summarised in Table 1 , 'Methodological framework characteristics’. Fourteen publications proposed academic-orientated frameworks, which focused on measuring academic, societal, economic, and cultural impact using narrative and quantitative metrics [ 2 , 3 , 5 , 8 , 29 , 31 – 39 ]. Five publications focused on assessing the impact of research by focusing on the interaction process between stakeholders and researchers (‘productive interactions’), which is a requirement to achieve research impact. This approach tries to address the issue of attributing research impact to metrics [ 7 , 40 – 43 ]. Two frameworks focused on the importance of partnerships between researchers and policy makers, as a core element to accomplish research impact [ 44 , 45 ]. An additional 2 frameworks focused on evaluating the pathways to impact, i.e., linking processes between research and impact [ 30 , 46 ]. One framework assessed the ability of health technology to influence efficiency of healthcare systems [ 47 ]. Eight frameworks were developed in the UK [ 2 , 3 , 29 , 37 , 39 , 42 , 43 , 45 ], 6 in Canada [ 8 , 33 , 34 , 44 , 46 , 47 ], 4 in Australia [ 5 , 31 , 35 , 38 ], 3 in the Netherlands [ 7 , 40 , 41 ], and 2 in the United States [ 30 , 36 ], with 1 model developed with input from various countries [ 32 ].
https://doi.org/10.1371/journal.pmed.1002370.t001
Methodological framework development
The included methodological frameworks varied in their development process, but there were some common approaches employed. Most included a literature review [ 2 , 5 , 7 , 8 , 31 , 33 , 36 , 37 , 40 – 46 ], although none of them used a recognised systematic method. Most also consulted with various stakeholders [ 3 , 8 , 29 , 31 , 33 , 35 – 38 , 43 , 44 , 46 , 47 ] but used differing methods to incorporate their views, including quantitative surveys [ 32 , 35 , 43 , 46 ], face-to-face interviews [ 7 , 29 , 33 , 35 , 37 , 42 , 43 ], telephone interviews [ 31 , 46 ], consultation [ 3 , 7 , 36 ], and focus groups [ 39 , 43 ]. A range of stakeholder groups were approached across the sample, including principal investigators [ 7 , 29 , 43 ], research end users [ 7 , 42 , 43 ], academics [ 3 , 8 , 39 , 40 , 43 , 46 ], award holders [ 43 ], experts [ 33 , 38 , 39 ], sponsors [ 33 , 39 ], project coordinators [ 32 , 42 ], and chief investigators [ 31 , 35 ]. However, some authors failed to identify the stakeholders involved in the development of their frameworks [ 2 , 5 , 34 , 41 , 45 ], making it difficult to assess their appropriateness. In addition, only 4 of the included papers reported using formal analytic methods to interpret stakeholder responses. These included the Canadian Academy of Health Sciences framework, which used conceptual cluster analysis [ 33 ]. The Research Contribution [ 42 ], Research Impact [ 29 ], and Primary Health Care & Information Service [ 31 ] used a thematic analysis approach. Finally, some authors went on to pilot their framework, which shaped refinements on the methodological frameworks until approval. Methods used to pilot the frameworks included a case study approach [ 2 , 3 , 30 , 32 , 33 , 36 , 40 , 42 , 44 , 45 ], contrasting results against available literature [ 29 ], the use of stakeholders’ feedback [ 7 ], and assessment tools [ 35 , 46 ].
Major impact categories
1. primary research-related impact..
A number of methodological frameworks advocated the evaluation of ‘research-related impact’. This encompassed content related to the generation of new knowledge, knowledge dissemination, capacity building, training, leadership, and the development of research networks. These outcomes were considered the direct or primary impacts of a research project, as these are often the first evidenced returns [ 30 , 62 ].
A number of subgroups were identified within this category, with frameworks supporting the collection of impact data across the following constructs: ‘research and innovation outcomes’; ‘dissemination and knowledge transfer’; ‘capacity building, training, and leadership’; and ‘academic collaborations, research networks, and data sharing’.
1 . 1 . Research and innovation outcomes . Twenty of the 24 frameworks advocated the evaluation of ‘research and innovation outcomes’ [ 2 , 3 , 5 , 7 , 8 , 29 – 39 , 41 , 43 , 44 , 46 ]. This subgroup included the following metrics: number of publications; number of peer-reviewed articles (including journal impact factor); citation rates; requests for reprints, number of reviews, and meta-analysis; and new or changes in existing products (interventions or technology), patents, and research. Additionally, some frameworks also sought to gather information regarding ‘methods/methodological contributions’. These advocated the collection of systematic reviews and appraisals in order to identify gaps in knowledge and determine whether the knowledge generated had been assessed before being put into practice [ 29 ].
1 . 2 . Dissemination and knowledge transfer . Nineteen of the 24 frameworks advocated the assessment of ‘dissemination and knowledge transfer’ [ 2 , 3 , 5 , 7 , 29 – 32 , 34 – 43 , 46 ]. This comprised collection of the following information: number of conferences, seminars, workshops, and presentations; teaching output (i.e., number of lectures given to disseminate the research findings); number of reads for published articles; article download rate and number of journal webpage visits; and citations rates in nonjournal media such as newspapers and mass and social media (i.e., Twitter and blogs). Furthermore, this impact subgroup considered the measurement of research uptake and translatability and the adoption of research findings in technological and clinical applications and by different fields. These can be measured through patents, clinical trials, and partnerships between industry and business, government and nongovernmental organisations, and university research units and researchers [ 29 ].
1 . 3 . Capacity building , training , and leadership . Fourteen of 24 frameworks suggested the evaluation of ‘capacity building, training, and leadership’ [ 2 , 3 , 5 , 8 , 29 , 31 – 35 , 39 – 41 , 43 ]. This involved collecting information regarding the number of doctoral and postdoctoral studentships (including those generated as a result of the research findings and those appointed to conduct the research), as well as the number of researchers and research-related staff involved in the research projects. In addition, authors advocated the collection of ‘leadership’ metrics, including the number of research projects managed and coordinated and the membership of boards and funding bodies, journal editorial boards, and advisory committees [ 29 ]. Additional metrics in this category included public recognition (number of fellowships and awards for significant research achievements), academic career advancement, and subsequent grants received. Lastly, the impact metric ‘research system management’ comprised the collection of information that can lead to preserving the health of the population, such as modifying research priorities, resource allocation strategies, and linking health research to other disciplines to maximise benefits [ 29 ].
1 . 4 . Academic collaborations , research networks , and data sharing . Lastly, 10 of the 24 frameworks advocated the collection of impact data regarding ‘academic collaborations (internal and external collaborations to complete a research project), research networks, and data sharing’ [ 2 , 3 , 5 , 7 , 29 , 34 , 37 , 39 , 41 , 43 ].
2. Influence on policy making.
Methodological frameworks addressing this major impact category focused on measurable improvements within a given knowledge base and on interactions between academics and policy makers, which may influence policy-making development and implementation. The returns generated in this impact category are generally considered as intermediate or midterm (1 to 3 years). These represent an important interim stage in the process towards the final expected impacts, such as quantifiable health improvements and economic benefits, without which policy change may not occur [ 30 , 62 ]. The following impact subgroups were identified within this category: ‘type and nature of policy impact’, ‘level of policy making’, and ‘policy networks’.
2 . 1 . Type and nature of policy impact . The most common impact subgroup, mentioned in 18 of the 24 frameworks, was ‘type and nature of policy impact’ [ 2 , 7 , 29 – 38 , 41 – 43 , 45 – 47 ]. Methodological frameworks addressing this subgroup stressed the importance of collecting information regarding the influence of research on policy (i.e., changes in practice or terminology). For instance, a project looking at trafficked adolescents and women (2003) influenced the WHO guidelines (2003) on ethics regarding this particular group [ 17 , 21 , 63 ].
2 . 2 . Level of policy impact . Thirteen of 24 frameworks addressed aspects surrounding the need to record the ‘level of policy impact’ (international, national, or local) and the organisations within a level that were influenced (local policy makers, clinical commissioning groups, and health and wellbeing trusts) [ 2 , 5 , 8 , 29 , 31 , 34 , 38 , 41 , 43 – 47 ]. Authors considered it important to measure the ‘level of policy impact’ to provide evidence of collaboration, coordination, and efficiency within health organisations and between researchers and health organisations [ 29 , 31 ].
2 . 3 . Policy networks . Five methodological frameworks highlighted the need to collect information regarding collaborative research with industry and staff movement between academia and industry [ 5 , 7 , 29 , 41 , 43 ]. A policy network emphasises the relationship between policy communities, researchers, and policy makers. This relationship can influence and lead to incremental changes in policy processes [ 62 ].
3. Health and health systems impact.
A number of methodological frameworks advocated the measurement of impacts on health and healthcare systems across the following impact subgroups: ‘quality of care and service delivering’, ‘evidence-based practice’, ‘improved information and health information management’, ‘cost containment and effectiveness’, ‘resource allocation’, and ‘health workforce’.
3 . 1 . Quality of care and service delivery . Twelve of the 24 frameworks highlighted the importance of evaluating ‘quality of care and service delivery’ [ 2 , 5 , 8 , 29 – 31 , 33 – 36 , 41 , 47 ]. There were a number of suggested metrics that could be potentially used for this purpose, including health outcomes such as quality-adjusted life years (QALYs), patient-reported outcome measures (PROMs), patient satisfaction and experience surveys, and qualitative data on waiting times and service accessibility.
3 . 2 . Evidence-based practice . ‘Evidence-based practice’, mentioned in 5 of the 24 frameworks, refers to making changes in clinical diagnosis, clinical practice, treatment decisions, or decision making based on research evidence [ 5 , 8 , 29 , 31 , 33 ]. The suggested metrics to demonstrate evidence-based practice were adoption of health technologies and research outcomes to improve the healthcare systems and inform policies and guidelines [ 29 ].
3 . 3 . Improved information and health information management . This impact subcategory, mentioned in 5 of the 24 frameworks, refers to the influence of research on the provision of health services and management of the health system to prevent additional costs [ 5 , 29 , 33 , 34 , 38 ]. Methodological frameworks advocated the collection of health system financial, nonfinancial (i.e., transport and sociopolitical implications), and insurance information in order to determine constraints within a health system.
3 . 4 . Cost containment and cost-effectiveness . Six of the 24 frameworks advocated the subcategory ‘cost containment and cost-effectiveness’ [ 2 , 5 , 8 , 17 , 33 , 36 ]. ‘Cost containment’ comprised the collection of information regarding how research has influenced the provision and management of health services and its implication in healthcare resource allocation and use [ 29 ]. ‘Cost-effectiveness’ refers to information concerning economic evaluations to assess improvements in effectiveness and health outcomes—for instance, the cost-effectiveness (cost and health outcome benefits) assessment of introducing a new health technology to replace an older one [ 29 , 31 , 64 ].
3 . 5 . Resource allocation . ‘Resource allocation’, mentioned in 6frameworks, can be measured through 2 impact metrics: new funding attributed to the intervention in question and equity while allocating resources, such as improved allocation of resources at an area level; better targeting, accessibility, and utilisation; and coverage of health services [ 2 , 5 , 29 , 31 , 45 , 47 ]. The allocation of resources and targeting can be measured through health services research reports, with the utilisation of health services measured by the probability of providing an intervention when needed, the probability of requiring it again in the future, and the probability of receiving an intervention based on previous experience [ 29 , 31 ].
3 . 6 . Health workforce . Lastly, ‘health workforce’, present in 3 methodological frameworks, refers to the reduction in the days of work lost because of a particular illness [ 2 , 5 , 31 ].
4. Health-related and societal impact.
Three subgroups were included in this category: ‘health literacy’; ‘health knowledge, attitudes, and behaviours’; and ‘improved social equity, inclusion, or cohesion’.
4 . 1 . Health knowledge , attitudes , and behaviours . Eight of the 24 frameworks suggested the assessment of ‘health knowledge, attitudes, behaviours, and outcomes’, which could be measured through the evaluation of levels of public engagement with science and research (e.g., National Health Service (NHS) Choices end-user visit rate) or by using focus groups to analyse changes in knowledge, attitudes, and behaviour among society [ 2 , 5 , 29 , 33 – 35 , 38 , 43 ].
4 . 2 . Improved equity , inclusion , or cohesion and human rights . Other methodological frameworks, 4 of the 24, suggested capturing improvements in equity, inclusion, or cohesion and human rights. Authors suggested these could be using a resource like the United Nations Millennium Development Goals (MDGs) (superseded by Sustainable Development Goals [SDGs] in 2015) and human rights [ 29 , 33 , 34 , 38 ]. For instance, a cluster-randomised controlled trial in Nepal, which had female participants, has demonstrated the reduction of neonatal mortality through the introduction of maternity health care, distribution of delivery kits, and home visits. This illustrates how research can target vulnerable and disadvantaged groups. Additionally, this research has been introduced by the World Health Organisation to achieve the MDG ‘improve maternal health’ [ 16 , 29 , 65 ].
4 . 3 . Health literacy . Some methodological frameworks, 3 of the 24, focused on tracking changes in the ability of patients to make informed healthcare decisions, reduce health risks, and improve quality of life, which were demonstrably linked to a particular programme of research [ 5 , 29 , 43 ]. For example, a systematic review showed that when HIV health literacy/knowledge is spread among people living with the condition, antiretroviral adherence and quality of life improve [ 66 ].
5. Broader economic impacts.
Some methodological frameworks, 9 of 24, included aspects related to the broader economic impacts of health research—for example, the economic benefits emerging from the commercialisation of research outputs [ 2 , 5 , 29 , 31 , 33 , 35 , 36 , 38 , 67 ]. Suggested metrics included the amount of funding for research and development (R&D) that was competitively awarded by the NHS, medical charities, and overseas companies. Additional metrics were income from intellectual property, spillover effects (any secondary benefit gained as a repercussion of investing directly in a primary activity, i.e., the social and economic returns of investing on R&D) [ 33 ], patents granted, licences awarded and brought to the market, the development and sales of spinout companies, research contracts, and income from industry.
The benefits contained within the categories ‘health and health systems impact’, ‘health-related and societal impact’, and ‘broader economic impacts’ are considered the expected and final returns of the resources allocated in healthcare research [ 30 , 62 ]. These benefits commonly arise in the long term, beyond 5 years according to some authors, but there was a recognition that this could differ depending on the project and its associated research area [ 4 ].
Data synthesis
Five major impact categories were identified across the 24 included methodological frameworks: (1) ‘primary research-related impact’, (2) ‘influence on policy making’, (3) ‘health and health systems impact’, (4) ‘health-related and societal impact’, and (5) ‘broader economic impact’. These major impact categories were further subdivided into 16 impact subgroups. The included publications proposed 80 different metrics to measure research impact. This impact typology synthesis is depicted in ‘the impact matrix’ ( Fig 2 and Fig 3 ).
CIHR, Canadian Institutes of Health Research; HTA, Health Technology Assessment; PHC RIS, Primary Health Care Research & Information Service; RAE, Research Assessment Exercise; RQF, Research Quality Framework.
https://doi.org/10.1371/journal.pmed.1002370.g002
AIHS, Alberta Innovates—Health Solutions; CAHS, Canadian Institutes of Health Research; IOM, Impact Oriented Monitoring; REF, Research Excellence Framework; SIAMPI, Social Impact Assessment Methods for research and funding instruments through the study of Productive Interactions between science and society.
https://doi.org/10.1371/journal.pmed.1002370.g003
Commonality and differences across frameworks
The ‘Research Impact Framework’ and the ‘Health Services Research Impact Framework’ were the models that encompassed the largest number of the metrics extracted. The most dominant methodological framework was the Payback Framework; 7 other methodological framework models used the Payback Framework as a starting point for development [ 8 , 29 , 31 – 35 ]. Additional methodological frameworks that were commonly incorporated into other tools included the CIHR framework, the CAHS model, the AIHS framework, and the Exchange model [ 8 , 33 , 34 , 44 ]. The capture of ‘research-related impact’ was the most widely advocated concept across methodological frameworks, illustrating the importance with which primary short-term impact outcomes were viewed by the included papers. Thus, measurement of impact via number of publications, citations, and peer-reviewed articles was the most common. ‘Influence on policy making’ was the predominant midterm impact category, specifically the subgroup ‘type and nature of policy impact’, in which frameworks advocated the measurement of (i) changes to legislation, regulations, and government policy; (ii) influence and involvement in decision-making processes; and (iii) changes to clinical or healthcare training, practice, or guidelines. Within more long-term impact measurement, the evaluations of changes in the ‘quality of care and service delivery’ were commonly advocated.
In light of the commonalities and differences among the methodological frameworks, the ‘pathways to research impact’ diagram ( Fig 4 ) was developed to provide researchers, funders, and policy makers a more comprehensive and exhaustive way to measure healthcare research impact. The diagram has the advantage of assorting all the impact metrics proposed by previous frameworks and grouping them into different impact subgroups and categories. Prospectively, this global picture will help researchers, funders, and policy makers plan strategies to achieve multiple pathways to impact before carrying the research out. The analysis of the data extraction and construction of the impact matrix led to the development of the ‘pathways to research impact’ diagram ( Fig 4 ). The diagram aims to provide an exhaustive and comprehensive way of tracing research impact by combining all the impact metrics presented by the different 24 frameworks, grouping those metrics into different impact subgroups, and grouping these into broader impact categories.
NHS, National Health Service; PROM, patient-reported outcome measure; QALY, quality-adjusted life year; R&D, research and development.
https://doi.org/10.1371/journal.pmed.1002370.g004
This review has summarised existing methodological impact frameworks together for the first time using systematic methods ( Fig 4 ). It allows researchers and funders to consider pathways to impact at the design stage of a study and to understand the elements and metrics that need to be considered to facilitate prospective assessment of impact. Users do not necessarily need to cover all the aspects of the methodological framework, as every research project can impact on different categories and subgroups. This review provides information that can assist researchers to better demonstrate impact, potentially increasing the likelihood of conducting impactful research and reducing research waste. Existing reviews have not presented a methodological framework that includes different pathways to impact, health impact categories, subgroups, and metrics in a single methodological framework.
Academic-orientated frameworks included in this review advocated the measurement of impact predominantly using so-called ‘quantitative’ metrics—for example, the number of peer-reviewed articles, journal impact factor, and citation rates. This may be because they are well-established measures, relatively easy to capture and objective, and are supported by research funding systems. However, these metrics primarily measure the dissemination of research finding rather than its impact [ 30 , 68 ]. Whilst it is true that wider dissemination, especially when delivered via world-leading international journals, may well lead eventually to changes in healthcare, this is by no means certain. For instance, case studies evaluated by Flinders University of Australia demonstrated that some research projects with non-peer-reviewed publications led to significant changes in health policy, whilst the studies with peer-reviewed publications did not result in any type of impact [ 68 ]. As a result, contemporary literature has tended to advocate the collection of information regarding a variety of different potential forms of impact alongside publication/citations metrics [ 2 , 3 , 5 , 7 , 8 , 29 – 47 ], as outlined in this review.
The 2014 REF exercise adjusted UK university research funding allocation based on evidence of the wider impact of research (through case narrative studies and quantitative metrics), rather than simply according to the quality of research [ 12 ]. The intention was to ensure funds were directed to high-quality research that could demonstrate actual realised benefit. The inclusion of a mixed-method approach to the measurement of impact in the REF (narrative and quantitative metrics) reflects a widespread belief—expressed by the majority of authors of the included methodological frameworks in the review—that individual quantitative impact metrics (e.g., number of citations and publications) do not necessary capture the complexity of the relationships involved in a research project and may exclude measurement of specific aspects of the research pathway [ 10 , 12 ].
Many of the frameworks included in this review advocated the collection of a range of academic, societal, economic, and cultural impact metrics; this is consistent with recent recommendations from the Stern review [ 10 ]. However, a number of these metrics encounter research ‘lag’: i.e., the time between the point at which the research is conducted and when the actual benefits arise [ 69 ]. For instance, some cardiovascular research has taken up to 25 years to generate impact [ 70 ]. Likewise, the impact may not arise exclusively from a single piece of research. Different processes (such as networking interactions and knowledge and research translation) and multiple individuals and organisations are often involved [ 4 , 71 ]. Therefore, attributing the contribution made by each of the different actors involved in the process can be a challenge [ 4 ]. An additional problem associated to attribution is the lack of evidence to link research and impact. The outcomes of research may emerge slowly and be absorbed gradually. Consequently, it is difficult to determine the influence of research in the development of a new policy, practice, or guidelines [ 4 , 23 ].
A further problem is that impact evaluation is conducted ‘ex post’, after the research has concluded. Collecting information retrospectively can be an issue, as the data required might not be available. ‘ex ante’ assessment is vital for funding allocation, as it is necessary to determine the potential forthcoming impact before research is carried out [ 69 ]. Additionally, ex ante evaluation of potential benefit can overcome the issues regarding identifying and capturing evidence, which can be used in the future [ 4 ]. In order to conduct ex ante evaluation of potential benefit, some authors suggest the early involvement of policy makers in a research project coupled with a well-designed strategy of dissemination [ 40 , 69 ].
Providing an alternate view, the authors of methodological frameworks such as the SIAMPI, Contribution Mapping, Research Contribution, and the Exchange model suggest that the problems of attribution are a consequence of assigning the impact of research to a particular impact metric [ 7 , 40 , 42 , 44 ]. To address these issues, these authors propose focusing on the contribution of research through assessing the processes and interactions between stakeholders and researchers, which arguably take into consideration all the processes and actors involved in a research project [ 7 , 40 , 42 , 43 ]. Additionally, contributions highlight the importance of the interactions between stakeholders and researchers from an early stage in the research process, leading to a successful ex ante and ex post evaluation by setting expected impacts and determining how the research outcomes have been utilised, respectively [ 7 , 40 , 42 , 43 ]. However, contribution metrics are generally harder to measure in comparison to academic-orientated indicators [ 72 ].
Currently, there is a debate surrounding the optimal methodological impact framework, and no tool has proven superior to another. The most appropriate methodological framework for a given study will likely depend on stakeholder needs, as each employs different methodologies to assess research impact [ 4 , 37 , 41 ]. This review allows researchers to select individual existing methodological framework components to create a bespoke tool with which to facilitate optimal study design and maximise the potential for impact depending on the characteristic of their study ( Fig 2 and Fig 3 ). For instance, if researchers are interested in assessing how influential their research is on policy making, perhaps considering a suite of the appropriate metrics drawn from multiple methodological frameworks may provide a more comprehensive method than adopting a single methodological framework. In addition, research teams may wish to use a multidimensional approach to methodological framework development, adopting existing narratives and quantitative metrics, as well as elements from contribution frameworks. This approach would arguably present a more comprehensive method of impact assessment; however, further research is warranted to determine its effectiveness [ 4 , 69 , 72 , 73 ].
Finally, it became clear during this review that the included methodological frameworks had been constructed using varied methodological processes. At present, there are no guidelines or consensus around the optimal pathway that should be followed to develop a robust methodological framework. The authors believe this is an area that should be addressed by the research community, to ensure future frameworks are developed using best-practice methodology.
For instance, the Payback Framework drew upon a literature review and was refined through a case study approach. Arguably, this approach could be considered inferior to other methods that involved extensive stakeholder involvement, such as the CIHR framework [ 8 ]. Nonetheless, 7 methodological frameworks were developed based upon the Payback Framework [ 8 , 29 , 31 – 35 ].
Limitations
The present review is the first to summarise systematically existing impact methodological frameworks and metrics. The main limitation is that 50% of the included publications were found through methods other than bibliographic databases searching, indicating poor indexing. Therefore, some relevant articles may not have been included in this review if they failed to indicate the inclusion of a methodological impact framework in their title/abstract. We did, however, make every effort to try to find these potentially hard-to-reach publications, e.g., through forwards/backwards citation searching, hand searching reference lists, and expert communication. Additionally, this review only extracted information regarding the methodology followed to develop each framework from the main publication source or framework webpage. Therefore, further evaluations may not have been included, as they are beyond the scope of the current paper. A further limitation was that although our search strategy did not include language restrictions, we did not specifically search non-English language databases. Thus, we may have failed to identify potentially relevant methodological frameworks that were developed in a non-English language setting.
In conclusion, the measurement of research impact is an essential exercise to help direct the allocation of limited research resources, to maximise benefit, and to help minimise research waste. This review provides a collective summary of existing methodological impact frameworks and metrics, which funders may use to inform the measurement of research impact and researchers may use to inform study design decisions aimed at maximising the short-, medium-, and long-term impact of their research.
Supporting information
S1 appendix. search strategy..
https://doi.org/10.1371/journal.pmed.1002370.s001
S1 PRISMA Checklist. Preferred Reporting Items for Systematic Reviews and Meta-Analyses (PRISMA) checklist.
https://doi.org/10.1371/journal.pmed.1002370.s002
Acknowledgments
We would also like to thank Mrs Susan Bayliss, Information Specialist, University of Birmingham, and Mrs Karen Biddle, Research Secretary, University of Birmingham.
- View Article
- PubMed/NCBI
- Google Scholar
- 3. HEFCE. REF 2014: Assessment framework and guidance on submissions 2011 [cited 2016 15 Feb]. Available from: http://www.ref.ac.uk/media/ref/content/pub/assessmentframeworkandguidanceonsubmissions/GOS%20including%20addendum.pdf .
- 8. Canadian Institutes of Health Research. Developing a CIHR framework to measure the impact of health research 2005 [cited 2016 26 Feb]. Available from: http://publications.gc.ca/collections/Collection/MR21-65-2005E.pdf .
- 9. HEFCE. HEFCE allocates £3.97 billion to universities and colleges in England for 2015–1 2015. Available from: http://www.hefce.ac.uk/news/newsarchive/2015/Name,103785,en.html .
- 10. Stern N. Building on Success and Learning from Experience—An Independent Review of the Research Excellence Framework 2016 [cited 2016 05 Aug]. Available from: https://www.gov.uk/government/uploads/system/uploads/attachment_data/file/541338/ind-16-9-ref-stern-review.pdf .
- 11. Matthews D. REF sceptic to lead review into research assessment: Times Higher Education; 2015 [cited 2016 21 Apr]. Available from: https://www.timeshighereducation.com/news/ref-sceptic-lead-review-research-assessment .
- 12. HEFCE. The Metric Tide—Report of the Independent Review of the Role of Metrics in Research Assessment and Management 2015 [cited 2016 11 Aug]. Available from: http://www.hefce.ac.uk/media/HEFCE,2014/Content/Pubs/Independentresearch/2015/The,Metric,Tide/2015_metric_tide.pdf .
- 14. LSE Public Policy Group. Maximizing the impacts of your research: A handbook for social scientists. http://www.lse.ac.uk/government/research/resgroups/LSEPublicPolicy/Docs/LSE_Impact_Handbook_April_2011.pdf . London: LSE; 2011.
- 15. HEFCE. Consultation on the second Research Excellence Framework. 2016.
- 18. Merriam-Webster Dictionary 2017. Available from: https://www.merriam-webster.com/dictionary/methodology .
- 19. Oxford Dictionaries—pathway 2016 [cited 2016 19 June]. Available from: http://www.oxforddictionaries.com/definition/english/pathway .
- 20. Oxford Dictionaries—metric 2016 [cited 2016 15 Sep]. Available from: https://en.oxforddictionaries.com/definition/metric .
- 21. WHO. WHO Ethical and Safety Guidelines for Interviewing Trafficked Women 2003 [cited 2016 29 July]. Available from: http://www.who.int/mip/2003/other_documents/en/Ethical_Safety-GWH.pdf .
- 31. Kalucy L, et al. Primary Health Care Research Impact Project: Final Report Stage 1 Adelaide: Primary Health Care Research & Information Service; 2007 [cited 2016 26 Feb]. Available from: http://www.phcris.org.au/phplib/filedownload.php?file=/elib/lib/downloaded_files/publications/pdfs/phcris_pub_3338.pdf .
- 33. Canadian Academy of Health Sciences. Making an impact—A preferred framework and indicators to measure returns on investment in health research 2009 [cited 2016 26 Feb]. Available from: http://www.cahs-acss.ca/wp-content/uploads/2011/09/ROI_FullReport.pdf .
- 39. HEFCE. RAE 2008—Guidance in submissions 2005 [cited 2016 15 Feb]. Available from: http://www.rae.ac.uk/pubs/2005/03/rae0305.pdf .
- 41. Royal Netherlands Academy of Arts and Sciences. The societal impact of applied health research—Towards a quality assessment system 2002 [cited 2016 29 Feb]. Available from: https://www.knaw.nl/en/news/publications/the-societal-impact-of-applied-health-research/@@download/pdf_file/20021098.pdf .
- 48. Weiss CH. Using social research in public policy making: Lexington Books; 1977.
- 50. Kogan M, Henkel M. Government and research: the Rothschild experiment in a government department: Heinemann Educational Books; 1983.
- 51. Thomas P. The Aims and Outcomes of Social Policy Research. Croom Helm; 1985.
- 52. Bulmer M. Social Science Research and Government: Comparative Essays on Britain and the United States: Cambridge University Press; 2010.
- 53. Booth T. Developing Policy Research. Aldershot, Gower1988.
- 55. Kalucy L, et al Exploring the impact of primary health care research Stage 2 Primary Health Care Research Impact Project Adelaide: Primary Health Care Research & Information Service (PHCRIS); 2009 [cited 2016 26 Feb]. Available from: http://www.phcris.org.au/phplib/filedownload.php?file=/elib/lib/downloaded_files/publications/pdfs/phcris_pub_8108.pdf .
- 56. CHSRF. Canadian Health Services Research Foundation 2000. Health Services Research and Evidence-based Decision Making [cited 2016 February]. Available from: http://www.cfhi-fcass.ca/migrated/pdf/mythbusters/EBDM_e.pdf .
- 58. W.K. Kellogg Foundation. Logic Model Development Guide 2004 [cited 2016 19 July]. Available from: http://www.smartgivers.org/uploads/logicmodelguidepdf.pdf .
- 59. United Way of America. Measuring Program Outcomes: A Practical Approach 1996 [cited 2016 19 July]. Available from: https://www.bttop.org/sites/default/files/public/W.K.%20Kellogg%20LogicModel.pdf .
- 60. Nutley S, Percy-Smith J and Solesbury W. Models of research impact: a cross sector review of literature and practice. London: Learning and Skills Research Centre 2003.
- 61. Spaapen J, van Drooge L. SIAMPI final report [cited 2017 Jan]. Available from: http://www.siampi.eu/Content/SIAMPI_Final%20report.pdf .
- 63. LSHTM. The Health Risks and Consequences of Trafficking in Women and Adolescents—Findings from a European Study 2003 [cited 2016 29 July]. Available from: http://www.oas.org/atip/global%20reports/zimmerman%20tip%20health.pdf .
- 70. Russell G. Response to second HEFCE consultation on the Research Excellence Framework 2009 [cited 2016 04 Apr]. Available from: http://russellgroup.ac.uk/media/5262/ref-consultation-response-final-dec09.pdf .
- Fact sheets
- Facts in pictures
Publications
- Questions and answers
- Tools and toolkits
- Endometriosis
- Excessive heat
- Mental disorders
- Polycystic ovary syndrome
- All countries
- Eastern Mediterranean
- South-East Asia
- Western Pacific
- Data by country
- Country presence
- Country strengthening
- Country cooperation strategies
- News releases
- Feature stories
- Press conferences
- Commentaries
- Photo library
- Afghanistan
- Cholera
- Coronavirus disease (COVID-19)
- Greater Horn of Africa
- Israel and occupied Palestinian territory
- Disease Outbreak News
- Situation reports
- Weekly Epidemiological Record
- Surveillance
- Health emergency appeal
- International Health Regulations
- Independent Oversight and Advisory Committee
- Classifications
- Data collections
- Global Health Estimates
- Mortality Database
- Sustainable Development Goals
- Health Inequality Monitor
- Global Progress
- World Health Statistics
- Partnerships
- Committees and advisory groups
- Collaborating centres
- Technical teams
- Organizational structure
- Initiatives
- General Programme of Work
- WHO Academy
- Investment in WHO
- WHO Foundation
- External audit
- Financial statements
- Internal audit and investigations
- Programme Budget
- Results reports
- Governing bodies
- World Health Assembly
- Executive Board
- Member States Portal
- Health topics /
Research is indispensable for resolving public health challenges – whether it be tackling diseases of poverty, responding to rise of chronic diseases, or ensuring that mothers have access to safe delivery practices.
Likewise, shared vulnerability to global threats, such as severe acute respiratory syndrome, Ebola virus disease, Zika virus and avian influenza has mobilized global research efforts in support of enhancing capacity for preparedness and response. Research is strengthening surveillance, rapid diagnostics and development of vaccines and medicines.
Public-private partnerships and other innovative mechanisms for research are concentrating on neglected diseases in order to stimulate the development of vaccines, drugs and diagnostics where market forces alone are insufficient.
Research for health spans 5 generic areas of activity:
- measuring the magnitude and distribution of the health problem;
- understanding the diverse causes or the determinants of the problem, whether they are due to biological, behavioural, social or environmental factors;
- developing solutions or interventions that will help to prevent or mitigate the problem;
- implementing or delivering solutions through policies and programmes; and
- evaluating the impact of these solutions on the level and distribution of the problem.
High-quality research is essential to fulfilling WHO’s mandate for the attainment by all peoples of the highest possible level of health. One of the Organization’s core functions is to set international norms, standards and guidelines, including setting international standards for research.
Under the “WHO strategy on research for health”, the Organization works to identify research priorities, and promote and conduct research with the following 4 goals:
- Capacity - build capacity to strengthen health research systems within Member States.
- Priorities - support the setting of research priorities that meet health needs particularly in low- and middle-income countries.
- Standards - develop an enabling environment for research through the creation of norms and standards for good research practice.
- Translation - ensure quality evidence is turned into affordable health technologies and evidence-informed policy.
- Prequalification of medicines by WHO
- Global Observatory on Health R&D
- Global Observatory on Health Research and Development
- Implementation research toolkit
- Ethics in implementation research: participant's guide
- Ethics in implementation research: facilitator's guide
- Ethics in epidemics, emergencies and disasters: Research, surveillance and patient care: WHO training manual
- WHA58.34 Ministerial Summit on Health Research
- WHA60.15 WHO's role and responsibilities in health research
- WHA63.21 WHO's role and responsibilities in health research
- EB115/30 Ministerial Summit on Health Research: report by the Secretariat
- Science division
WHO consults on action plan for sustainable clinical research infrastructure
WHO advisory group convenes its first meeting on responsible use of the life sciences in Geneva
Challenging harmful masculinities and engaging men and boys in sexual and reproductive health
Stakeholders convene in Uganda on responsible use of the life sciences

Target product profiles for animal plasma-derived antivenoms: antivenoms for treatment of snakebite envenoming...
We describe here WHO public-benefit Target Product Profiles (TPPs) for antivenoms intended to be used for treatment of snakebite envenoming in South Asia,...

WHO global research priorities for sexually transmitted infections
Sexually transmitted infections (STIs) are widespread globally and negatively affect sexual and reproductive health. Gaps in evidence and in available...

Report of the sixth meeting of the WHO Diagnostic Technical Advisory Group for Neglected Tropical Diseases:...
The World Health Organization’s Global Neglected Tropical Diseases Programme (WHO/NTD) manages a diverse portfolio of 21 diseases and disease groups,1 ...

Target product profile for a diagnostic test to confirm cure of visceral leishmaniasis
Leishmaniasis is caused by protozoan parasites which are transmitted by the bite of infected female phlebotomine sandflies. The disease is poverty-related...
Coordinating R&D on antimicrobial resistance
Ensuring responsible use of life sciences research
Prioritizing diseases for research and development in emergency contexts
Promoting research on Buruli ulcer
Research in maternal, perinatal, and adolescent health
Undertaking health law research
Feature story
One year on, Global Observatory on Health R&D identifies striking gaps and inequalities

Video: Open access to health: WHO joins cOAlition S

Video: Multisectional research on sleeping sickness in Tanzania in the context of climate change
Related health topics
Clinical trials
Global health ethics
Health Laws
Intellectual property and trade
Related links
Research and Development Blueprint
WHO Collaborating Centres
R&D Blueprint for Action to Prevent Epidemics
International Clinical Trials Registry Platform
- Share full article
Advertisement
Supported by
About 400 Million People Worldwide Have Had Long Covid, Researchers Say
The condition has put significant strain on patients and society — at a global economic cost of about $1 trillion a year, a new report estimates.

By Pam Belluck
Pam Belluck has been reporting about long Covid since the condition first emerged.
About 400 million people worldwide have been afflicted with long Covid, according to a new report by scientists and other researchers who have studied the condition. The team estimated that the economic cost — from factors like health care services and patients unable to return to work — is about $1 trillion worldwide each year, or about 1 percent of the global economy.
The report, published Friday in the journal Nature Medicine, is an effort to summarize the knowledge about and effects of long Covid across the globe four years after it first emerged.
It also aims to “provide a road map for policy and research priorities,” said one author, Dr. Ziyad Al-Aly, the chief of research and development at the V.A. St. Louis Health Care System and a clinical epidemiologist at Washington University in St. Louis. He wrote the paper with several other leading long Covid researchers and three leaders of the Patient-Led Research Collaborative, an organization formed by long Covid patients who are also professional researchers.
Among the conclusions:
About 6 percent of adults globally have had long Covid.
The authors evaluated scores of studies and metrics to estimate that as of the end of 2023, about 6 percent of adults and about 1 percent of children — or about 400 million people — had ever had long Covid since the pandemic began. They said the estimate accounted for the fact that new cases slowed in 2022 and 2023 because of vaccines and the milder Omicron variant.
They suggested that the actual number might be higher because their estimate included only people who developed long Covid after they had symptoms during the infectious stage of the virus, and it did not include people who had more than one Covid infection.
Many people have not fully recovered.
The authors cited studies suggesting that only 7 percent to 10 percent of long Covid patients fully recovered two years after developing long Covid. They added that “some manifestations of long Covid, including heart disease, diabetes, myalgic encephalomyelitis and dysautonomia are chronic conditions that last a lifetime.”
The consequences are far-reaching, the authors wrote: “Long Covid drastically affects patients’ well-being and sense of self, as well as their ability to work, socialize, care for others, manage chores and engage in community activities — which also affects patients’ families, caregivers and their communities.”
The report cited estimates that between two million and four million adults were out of work because of long Covid in 2022 and that people with long Covid were 10 percent less likely to be employed than those who were never infected with the virus. Long Covid patients often have to reduce their work hours, and one in four limit activities outside work in order to continue working, the report said.
We are having trouble retrieving the article content.
Please enable JavaScript in your browser settings.
Thank you for your patience while we verify access. If you are in Reader mode please exit and log into your Times account, or subscribe for all of The Times.
Thank you for your patience while we verify access.
Already a subscriber? Log in .
Want all of The Times? Subscribe .

Research Topics & Ideas: Healthcare
100+ Healthcare Research Topic Ideas To Fast-Track Your Project
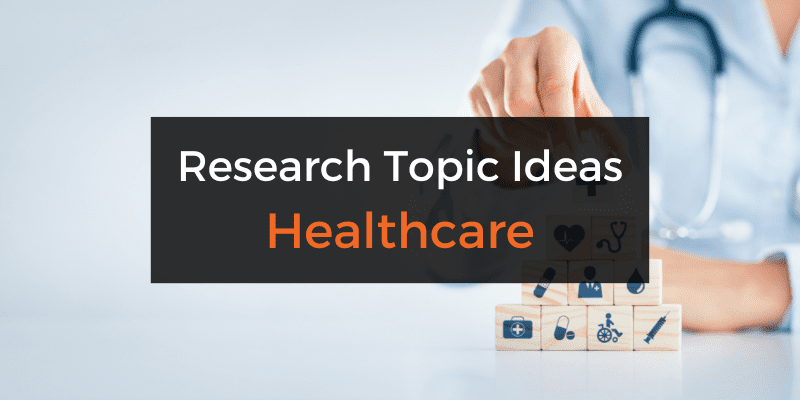
Finding and choosing a strong research topic is the critical first step when it comes to crafting a high-quality dissertation, thesis or research project. If you’ve landed on this post, chances are you’re looking for a healthcare-related research topic , but aren’t sure where to start. Here, we’ll explore a variety of healthcare-related research ideas and topic thought-starters across a range of healthcare fields, including allopathic and alternative medicine, dentistry, physical therapy, optometry, pharmacology and public health.
NB – This is just the start…
The topic ideation and evaluation process has multiple steps . In this post, we’ll kickstart the process by sharing some research topic ideas within the healthcare domain. This is the starting point, but to develop a well-defined research topic, you’ll need to identify a clear and convincing research gap , along with a well-justified plan of action to fill that gap.
If you’re new to the oftentimes perplexing world of research, or if this is your first time undertaking a formal academic research project, be sure to check out our free dissertation mini-course. In it, we cover the process of writing a dissertation or thesis from start to end. Be sure to also sign up for our free webinar that explores how to find a high-quality research topic.
Overview: Healthcare Research Topics
- Allopathic medicine
- Alternative /complementary medicine
- Veterinary medicine
- Physical therapy/ rehab
- Optometry and ophthalmology
- Pharmacy and pharmacology
- Public health
- Examples of healthcare-related dissertations
Allopathic (Conventional) Medicine
- The effectiveness of telemedicine in remote elderly patient care
- The impact of stress on the immune system of cancer patients
- The effects of a plant-based diet on chronic diseases such as diabetes
- The use of AI in early cancer diagnosis and treatment
- The role of the gut microbiome in mental health conditions such as depression and anxiety
- The efficacy of mindfulness meditation in reducing chronic pain: A systematic review
- The benefits and drawbacks of electronic health records in a developing country
- The effects of environmental pollution on breast milk quality
- The use of personalized medicine in treating genetic disorders
- The impact of social determinants of health on chronic diseases in Asia
- The role of high-intensity interval training in improving cardiovascular health
- The efficacy of using probiotics for gut health in pregnant women
- The impact of poor sleep on the treatment of chronic illnesses
- The role of inflammation in the development of chronic diseases such as lupus
- The effectiveness of physiotherapy in pain control post-surgery
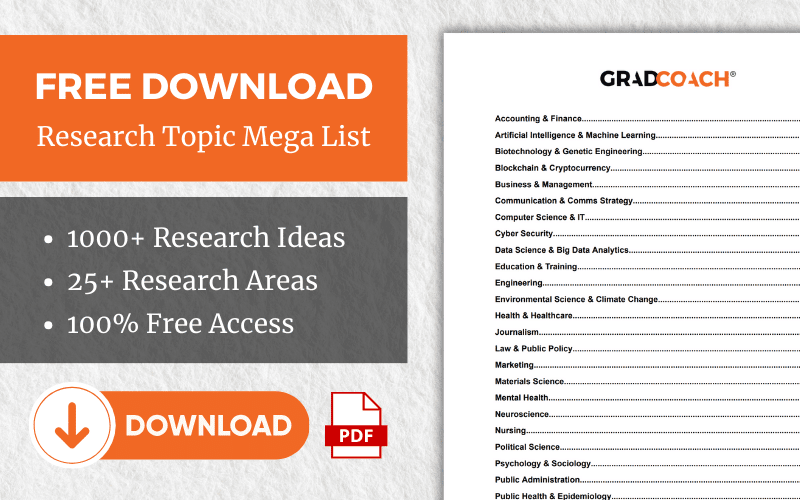
Topics & Ideas: Alternative Medicine
- The benefits of herbal medicine in treating young asthma patients
- The use of acupuncture in treating infertility in women over 40 years of age
- The effectiveness of homoeopathy in treating mental health disorders: A systematic review
- The role of aromatherapy in reducing stress and anxiety post-surgery
- The impact of mindfulness meditation on reducing high blood pressure
- The use of chiropractic therapy in treating back pain of pregnant women
- The efficacy of traditional Chinese medicine such as Shun-Qi-Tong-Xie (SQTX) in treating digestive disorders in China
- The impact of yoga on physical and mental health in adolescents
- The benefits of hydrotherapy in treating musculoskeletal disorders such as tendinitis
- The role of Reiki in promoting healing and relaxation post birth
- The effectiveness of naturopathy in treating skin conditions such as eczema
- The use of deep tissue massage therapy in reducing chronic pain in amputees
- The impact of tai chi on the treatment of anxiety and depression
- The benefits of reflexology in treating stress, anxiety and chronic fatigue
- The role of acupuncture in the prophylactic management of headaches and migraines
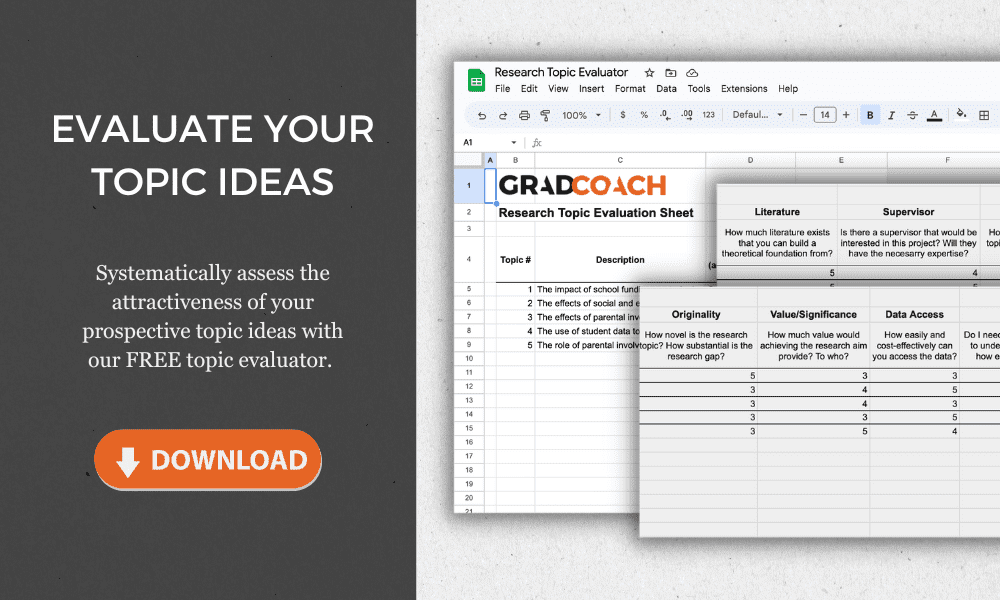
Topics & Ideas: Dentistry
- The impact of sugar consumption on the oral health of infants
- The use of digital dentistry in improving patient care: A systematic review
- The efficacy of orthodontic treatments in correcting bite problems in adults
- The role of dental hygiene in preventing gum disease in patients with dental bridges
- The impact of smoking on oral health and tobacco cessation support from UK dentists
- The benefits of dental implants in restoring missing teeth in adolescents
- The use of lasers in dental procedures such as root canals
- The efficacy of root canal treatment using high-frequency electric pulses in saving infected teeth
- The role of fluoride in promoting remineralization and slowing down demineralization
- The impact of stress-induced reflux on oral health
- The benefits of dental crowns in restoring damaged teeth in elderly patients
- The use of sedation dentistry in managing dental anxiety in children
- The efficacy of teeth whitening treatments in improving dental aesthetics in patients with braces
- The role of orthodontic appliances in improving well-being
- The impact of periodontal disease on overall health and chronic illnesses
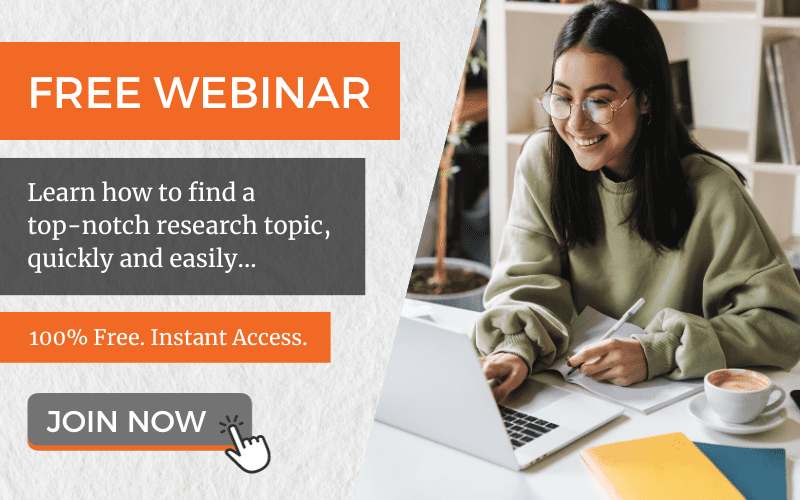
Tops & Ideas: Veterinary Medicine
- The impact of nutrition on broiler chicken production
- The role of vaccines in disease prevention in horses
- The importance of parasite control in animal health in piggeries
- The impact of animal behaviour on welfare in the dairy industry
- The effects of environmental pollution on the health of cattle
- The role of veterinary technology such as MRI in animal care
- The importance of pain management in post-surgery health outcomes
- The impact of genetics on animal health and disease in layer chickens
- The effectiveness of alternative therapies in veterinary medicine: A systematic review
- The role of veterinary medicine in public health: A case study of the COVID-19 pandemic
- The impact of climate change on animal health and infectious diseases in animals
- The importance of animal welfare in veterinary medicine and sustainable agriculture
- The effects of the human-animal bond on canine health
- The role of veterinary medicine in conservation efforts: A case study of Rhinoceros poaching in Africa
- The impact of veterinary research of new vaccines on animal health
Topics & Ideas: Physical Therapy/Rehab
- The efficacy of aquatic therapy in improving joint mobility and strength in polio patients
- The impact of telerehabilitation on patient outcomes in Germany
- The effect of kinesiotaping on reducing knee pain and improving function in individuals with chronic pain
- A comparison of manual therapy and yoga exercise therapy in the management of low back pain
- The use of wearable technology in physical rehabilitation and the impact on patient adherence to a rehabilitation plan
- The impact of mindfulness-based interventions in physical therapy in adolescents
- The effects of resistance training on individuals with Parkinson’s disease
- The role of hydrotherapy in the management of fibromyalgia
- The impact of cognitive-behavioural therapy in physical rehabilitation for individuals with chronic pain
- The use of virtual reality in physical rehabilitation of sports injuries
- The effects of electrical stimulation on muscle function and strength in athletes
- The role of physical therapy in the management of stroke recovery: A systematic review
- The impact of pilates on mental health in individuals with depression
- The use of thermal modalities in physical therapy and its effectiveness in reducing pain and inflammation
- The effect of strength training on balance and gait in elderly patients
Topics & Ideas: Optometry & Opthalmology
- The impact of screen time on the vision and ocular health of children under the age of 5
- The effects of blue light exposure from digital devices on ocular health
- The role of dietary interventions, such as the intake of whole grains, in the management of age-related macular degeneration
- The use of telemedicine in optometry and ophthalmology in the UK
- The impact of myopia control interventions on African American children’s vision
- The use of contact lenses in the management of dry eye syndrome: different treatment options
- The effects of visual rehabilitation in individuals with traumatic brain injury
- The role of low vision rehabilitation in individuals with age-related vision loss: challenges and solutions
- The impact of environmental air pollution on ocular health
- The effectiveness of orthokeratology in myopia control compared to contact lenses
- The role of dietary supplements, such as omega-3 fatty acids, in ocular health
- The effects of ultraviolet radiation exposure from tanning beds on ocular health
- The impact of computer vision syndrome on long-term visual function
- The use of novel diagnostic tools in optometry and ophthalmology in developing countries
- The effects of virtual reality on visual perception and ocular health: an examination of dry eye syndrome and neurologic symptoms
Topics & Ideas: Pharmacy & Pharmacology
- The impact of medication adherence on patient outcomes in cystic fibrosis
- The use of personalized medicine in the management of chronic diseases such as Alzheimer’s disease
- The effects of pharmacogenomics on drug response and toxicity in cancer patients
- The role of pharmacists in the management of chronic pain in primary care
- The impact of drug-drug interactions on patient mental health outcomes
- The use of telepharmacy in healthcare: Present status and future potential
- The effects of herbal and dietary supplements on drug efficacy and toxicity
- The role of pharmacists in the management of type 1 diabetes
- The impact of medication errors on patient outcomes and satisfaction
- The use of technology in medication management in the USA
- The effects of smoking on drug metabolism and pharmacokinetics: A case study of clozapine
- Leveraging the role of pharmacists in preventing and managing opioid use disorder
- The impact of the opioid epidemic on public health in a developing country
- The use of biosimilars in the management of the skin condition psoriasis
- The effects of the Affordable Care Act on medication utilization and patient outcomes in African Americans
Topics & Ideas: Public Health
- The impact of the built environment and urbanisation on physical activity and obesity
- The effects of food insecurity on health outcomes in Zimbabwe
- The role of community-based participatory research in addressing health disparities
- The impact of social determinants of health, such as racism, on population health
- The effects of heat waves on public health
- The role of telehealth in addressing healthcare access and equity in South America
- The impact of gun violence on public health in South Africa
- The effects of chlorofluorocarbons air pollution on respiratory health
- The role of public health interventions in reducing health disparities in the USA
- The impact of the United States Affordable Care Act on access to healthcare and health outcomes
- The effects of water insecurity on health outcomes in the Middle East
- The role of community health workers in addressing healthcare access and equity in low-income countries
- The impact of mass incarceration on public health and behavioural health of a community
- The effects of floods on public health and healthcare systems
- The role of social media in public health communication and behaviour change in adolescents
Examples: Healthcare Dissertation & Theses
While the ideas we’ve presented above are a decent starting point for finding a healthcare-related research topic, they are fairly generic and non-specific. So, it helps to look at actual dissertations and theses to see how this all comes together.
Below, we’ve included a selection of research projects from various healthcare-related degree programs to help refine your thinking. These are actual dissertations and theses, written as part of Master’s and PhD-level programs, so they can provide some useful insight as to what a research topic looks like in practice.
- Improving Follow-Up Care for Homeless Populations in North County San Diego (Sanchez, 2021)
- On the Incentives of Medicare’s Hospital Reimbursement and an Examination of Exchangeability (Elzinga, 2016)
- Managing the healthcare crisis: the career narratives of nurses (Krueger, 2021)
- Methods for preventing central line-associated bloodstream infection in pediatric haematology-oncology patients: A systematic literature review (Balkan, 2020)
- Farms in Healthcare: Enhancing Knowledge, Sharing, and Collaboration (Garramone, 2019)
- When machine learning meets healthcare: towards knowledge incorporation in multimodal healthcare analytics (Yuan, 2020)
- Integrated behavioural healthcare: The future of rural mental health (Fox, 2019)
- Healthcare service use patterns among autistic adults: A systematic review with narrative synthesis (Gilmore, 2021)
- Mindfulness-Based Interventions: Combatting Burnout and Compassionate Fatigue among Mental Health Caregivers (Lundquist, 2022)
- Transgender and gender-diverse people’s perceptions of gender-inclusive healthcare access and associated hope for the future (Wille, 2021)
- Efficient Neural Network Synthesis and Its Application in Smart Healthcare (Hassantabar, 2022)
- The Experience of Female Veterans and Health-Seeking Behaviors (Switzer, 2022)
- Machine learning applications towards risk prediction and cost forecasting in healthcare (Singh, 2022)
- Does Variation in the Nursing Home Inspection Process Explain Disparity in Regulatory Outcomes? (Fox, 2020)
Looking at these titles, you can probably pick up that the research topics here are quite specific and narrowly-focused , compared to the generic ones presented earlier. This is an important thing to keep in mind as you develop your own research topic. That is to say, to create a top-notch research topic, you must be precise and target a specific context with specific variables of interest . In other words, you need to identify a clear, well-justified research gap.
Need more help?
If you’re still feeling a bit unsure about how to find a research topic for your healthcare dissertation or thesis, check out Topic Kickstarter service below.
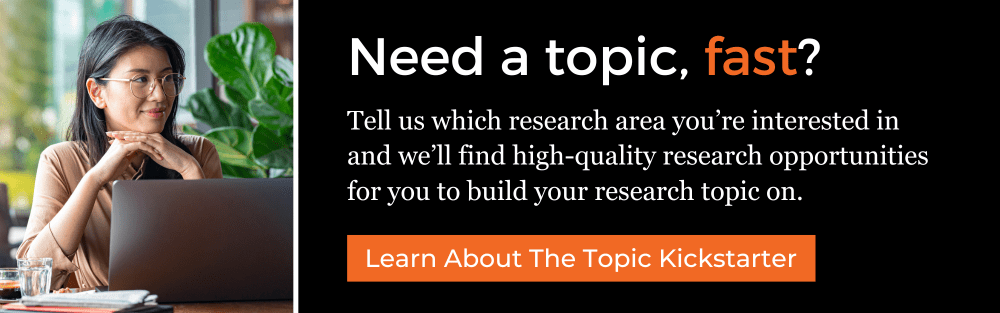

16 Comments
I need topics that will match the Msc program am running in healthcare research please
Hello Mabel,
I can help you with a good topic, kindly provide your email let’s have a good discussion on this.
Can you provide some research topics and ideas on Immunology?
Thank you to create new knowledge on research problem verse research topic
Help on problem statement on teen pregnancy
This post might be useful: https://gradcoach.com/research-problem-statement/
can you provide me with a research topic on healthcare related topics to a qqi level 5 student
Please can someone help me with research topics in public health ?
Hello I have requirement of Health related latest research issue/topics for my social media speeches. If possible pls share health issues , diagnosis, treatment.
I would like a topic thought around first-line support for Gender-Based Violence for survivors or one related to prevention of Gender-Based Violence
Please can I be helped with a master’s research topic in either chemical pathology or hematology or immunology? thanks
Can u please provide me with a research topic on occupational health and safety at the health sector
Good day kindly help provide me with Ph.D. Public health topics on Reproductive and Maternal Health, interventional studies on Health Education
may you assist me with a good easy healthcare administration study topic
May you assist me in finding a research topic on nutrition,physical activity and obesity. On the impact on children
I have been racking my brain for a while on what topic will be suitable for my PhD in health informatics. I want a qualitative topic as this is my strong area.
Submit a Comment Cancel reply
Your email address will not be published. Required fields are marked *
Save my name, email, and website in this browser for the next time I comment.
- Print Friendly
Skip to Content
Google Health research publications
Publishing our work allows us to share ideas and work collaboratively to advance healthcare. This is a comprehensive view of our publications and associated blog posts.
Blog Posts [more at Google Keyword Blog & Google Research Blog ]
by Karen DeSalvo
Google Keyword Blog | 19-Mar-2024
by Ronit Levavi Morad & Preeti Singh
Google Keyword Blog | 8-Mar-2024
Google Keyword Blog | 6-Feb-2024
by Aashima Gupta
Google Keyword Blog | 9-Jan-2024
by Jeff Dean, James Manyika, & Demis Hassabis
Google Research Blog | 22-Dec-2023
by Molly McHugh-Johnson
Google Keyword Blog | 20-Dec-2023
by Ivor Horn
Google Keyword Blog | 2-Nov-2023
by Yossi Mattia Shravya Shetty
Google Keyword Blog | 31-Oct-2023
by Yossi Mattias
Google Keyword Blog | 23-Oct-2023
by Nira Goren
Google Keyword Blog | 18-Oct-2023
by Michaell Howell
Google Keyword Blog | 9-Oct-2023
Google Keyword Blog | 3-Oct-2023
Google Keyword Blog | 18-Jul-2023
Blog Posts [more at Google Keyword Blog & Google AI Blog ]
by Susan Thomas
Google Keyword Blog | 5-Jul-2023
Google Keyword Blog | 13-Jun-2023
Google Keyword Blog | 23-May-2023
Google Keyword Blog | 22-May-2023
by Megan Jones Bell
Google Keyword Blog | 15-May-2023
Google Keyword Blog | 13-Apr-2023
Google Keyword Blog | 14-Mar-2023
by Greg Corrado & Yossi Matias
Google Research Blog | 23-Feb-2023
Google Keyword Blog | 26-Jan-2023
by Katie Malczyk
Google Keyword Blog | 17-Jan-2023
Google Keyword Blog | 5-Jan-2023
by Iz Conroy
Google Keyword Blog | 21-Dec-2022
by Hema Budaraju
Google Keyword Blog | 14-Dec-2022
Google Keyword Blog | 15-Nov-2022
Google Cloud Blog | 14-Nov-2022
by Jeff Dean
Google Keyword Blog | 2-Nov-2022
Google Keyword Blog | 27-Oct-2022
by Riva Sciuto
Google Keyword Blog | 19-Oct-2022
Google Keyword Blog | 12-Sep-2022
by Lauren Winer
Google Keyword Blog | 25-Aug-2022
by Anne Merritt
Google Keyword Blog | 20-Jul-2022
Google Keyword Blog | 17-May-2022
by Megan Jones Bell & Garth Graham
Google Keyword Blog | 10-May-2022
Google Keyword Blog | 24-Mar-2022
by Greg Corrado
Google Research Blog | 24-Mar-2022
by Paul Muret
Google Keyword Blog | 15-Mar-2022
Google Keyword Blog | 8-Mar-2022
Google Research Blog | 11-Jan-2022
Google Keyword Blog | 2-Dec-2021
Google Keyword Blog | 17-Oct-2021
by Alicia Cormie
Google Keyword Blog | 6-May-2021
Google Keyword Blog | 16-Apr-2021
Google Keyword Blog | 23- Feb-2021
Google Research Blog | 12-Jan-2021
by David Feinberg
LinkedIn Blog | 8-Dec-2020
by Dave Greenwood
Google Keyword Blog | 2-Dec-2020
by Anna Lurchenko
Google Design Blog | 29-Jul-2020
by Daniel Gillison, Jr
Google Keyword Blog | 28-May-2020
Google Research Blog | 9-Jan-2020
by Yun Liu & Po-Hsuan Cameron Chen
Google Research Blog | 10-Dec-2019
Google Keyword Blog | 20-Nov-2019
by Ruth Porat
Google Keyword Blog | 21-Oct-2019
by Dominic King
Google Keyword Blog | 18-Sep-2019
Google Research Blog | 15-Jan-2019
Google Keyword Blog | 17-Jun-2019
by Kent Walter
Google Keyword Blog | 13-Dec-2018
Google Research Blog | 12-Jan-2018
by Paula Schnurr & Teri Brister
Google Keyword Blog | 5-Dec-2017
by Mary Giliberti
Google Keyword Blog | 23-Aug-2017
by Katherine Chou
Google Research Blog | 17-May-2017
Google Research Blog | 12-Jan-2017
COVID-19 Blog Posts
Google Keyword Blog | 16-Jun-2022
COVID-19 Blog Posts [more at Google Keyword Blog ]
by Lauren Gallagher
Google Keyword Blog | 11-Feb-2022
Google Keyword Blog | 8-Dec-2021
by Tomer Shekel
Google Keyword Blog | 9-Jun-2021
by the COVID Response team, Google India
Google India Blog | 10-May-2021
Google Keyword Blog | 15-Apr-2021 [Spanish version]
by Stephen Ratcliffe
Google Keyword Blog | 24-Feb-2021
by Sundar Pichai
Google Keyword Blog | 25-Jan-2021
by Steph Hannon
Google Keyword Blog | 11-Dec-2020
by Karen DeSalvo & Kristie Canegallo
Google Keyword Blog | 10-Dec-2020
Google Keyword Blog | 24-Nov-2020 [Spanish version]
Google Keyword Blog
10-Nov-2020
Google Keyword Blog | 27-Oct-2020
Google Keyword Blog | 17-Sept-2020
by Mollie Javerbaum & Meghan Houghton
Google Keyword Blog | 10-Sep-2020
by Evgeniy Gabrilovich
Google Keyword Blog | 2-Sep-2020
by Dave Burke
Google Keyword Blog | 31-Jul-2020
by Apple & Google
Google Keyword Blog | 20-May-2020
by Megan Washam
Google Keyword Blog | 13-May-2020
Google Keyword Blog | 8-May-2020
Google Africa Blog
Google Africa Blog | 23-Apr-2020
Google Keyword Blog | 10-Apr-2020
by Julie Black
Google Keyword Blog | 6-Apr-2020
by Jen Fitzpatrick & Karen DeSalvo
Google Keyword Blog | 3-Apr-2020
by Emily Moxley
Google Keyword Blog | 21-Mar-2020
Google Keyword Blog | 15-Mar-2020
Google Keyword Blog | 6-Mar-2020
Lawrence, H. R., Schneider, R. A., Rubin, S. B., Mataric, M. J., McDuff, D. J. & Bell, M. J.
arXiv [cs.CL] (2024).
Graham, G., Goren, N., Sounderajah, V. & DeSalvo, K.
Nat. Med. (2024). [readcube]
Weng, W.-H., Sellergen, A., Kiraly, A. P., D’Amour, A., Park, J., Pilgrim, R., Pfohl, S., Lau, C., Natarajan, V., Azizi, S., Karthikesalingam, A., Cole-Lewis, H., Matias, Y., Corrado, G. S., Webster, D. R., Shetty, S., Prabhakara, S., Eswaran, K., Celi, L. A. G. & Liu, Y.
The Lancet Digital Health 6, e126–e130 (2024).
Howell M., Corrado G., DeSalvo K.
JAMA. 331(3):242–244 (2024).
Lehmann, L. S., Natarajan, V. & Peng, L. Chapter 39
(ed. Krittanawong, C.) Artificial Intelligence in Clinical Practice. 341–344 (Academic Press, 2024).
Lang, O., Traynis, I. & Liu, Y.
Nat Biomed Eng (2023). [readcube]
Deng, C.-Y., Mitani, A., Chen, C. W., Peng, L. H., Hammel, N. & Liu, Y
(eds. Yogesan, K., Goldschmidt, L., Cuadros, J. & Ricur, G.) 199–218. Springer International Publishing, 2023. [readcube]
Serghiou, S. & Rough, K.
Am. J. Epidemiol. (2023).
DeSalvo Karen B. & Howell Michael D.
NEJM Catalyst non-issue commentary (2023).
DeSalvo, K. B., Kadakia, K. T. & Chokshi, D. A.
JAMA Health Forum 2, e214051–e214051 (2021).
Kadakia, K. T., Howell, M. D. & DeSalvo, K. B.
JAMA 326, 385–386 (2021).
DeSalvo, K. B. & Kadakia, K. T.
Am. J. Public Health 111, S179–S181 (2021).
Sounderajah, V., Ashrafian, H., Rose, S., Shah, N. H., Ghassemi, M., Golub, R., Kahn, C. E., Jr, Esteva, A., Karthikesalingam, A., Mateen, B., Webster, D., Milea, D., Ting, D., Treanor, D., Cushnan, D., King, D., McPherson, D., Glocker, B., Greaves, F., Harling, L., Ordish, J., Cohen, J. F., Deeks, J., Leeflang, M., Diamond, M., McInnes, M. D. F., McCradden, M., Abràmoff, M. D., Normahani, P., Markar, S. R., Chang, S., Liu, X., Mallett, S., Shetty, S., Denniston, A., Collins, G. S., Moher, D., Whiting, P., Bossuyt, P. M. & Darzi, A.
Nat. Med. (2021).
Chen, P.-H. C., Mermel, C. H. & Liu, Y.
The Lancet Digital Health (2021). doi:10.1016/S2589-7500(21)00216-8
Kelly, C. J., Brown, A. P. Y. & Taylor, J. A.
(eds. Lidströmer, N. & Ashrafian, H.) 1–18 (Springer International Publishing, 2021).
Poplin, R., Zook, J. M. & DePristo, M.
JAMA 326, 268–269 (2021).
Mitani, A., Hammel, N. & Liu, Y.
Nature Biomedical Engineering 1–3 (2021). [readcube]
Esteva, A., Chou, K., Yeung, S., Naik, N., Madani, A., Mottaghi, A., Liu, Y., Topol, E., Dean, J. & Socher, R.
npj Digital Medicine 4, 5 (2021).
Steiner, D. F., Chen, P.-H. C. & Mermel, C. H.
Biochim. Biophys. Acta Rev. Cancer 1875, 188452 (2021).
Liu, Y., Yang, L., Phene, S. & Peng, L.
Artificial Intelligence in Medicine 247–264 (2021).
Warnert, E. A. H., Kasper, L., Meltzer, C. C., Lightfoote, J. B., Bucknor, M. D., Haroon, H., Duggan, G., Gowland, P., Wald, L., Miller, K. L., Morris, E. A. & Anazodo, U. C.
J. Magn. Reson. Imaging (2020). doi:10.1002/jmri.27476 [readcube]
Rakha, E. A., Toss, M., Shiino, S., Gamble, P., Jaroensri, R., Mermel, C. H. & Chen, P.-H. C.
J. Clin. Pathol. (2020). doi:10.1136/jclinpath-2020-206908
Sayres, R., Hammel, N. & Liu, Y.
Annals of Eye Science 5, 18–18 (2020).
Ibrahim, A., Gamble, P., Jaroensri, R., Abdelsamea, M. M., Mermel, C. H., Chen, P.-H. C. & Rakha, E. A.
Breast 49, 267–273 (2020).
Liu, Y., Chen, P.-H. C., Krause, J. & Peng, L.
JAMA 322, 1806–1816 (2019). [readcube]
Kelly, C. J., Karthikesalingam, A., Suleyman, M., Corrado, G., & King, D.
BMC Med. 17, 195 (2019).
Rajkomar, A., Hardt, M., Howell, M. D., Corrado, G., & Chin, M. H.
Ann. Intern. Med. 169(12):866-872 (2018).
Curiel-Lewandrowski, C., Novoa, R. A., Berry, E., Celebi, M. E., Codella, N., Giuste, F., Gutman, D., Halpern, A., Leachman, S., Liu, Y., Liu, Y., Reiter, O. & Tschandl, P.
599–628. Springer New York (2019).
Chen, C. P.-H., Liu, Y., & Peng, L.
Nat. Mater. 18, 410–414 (2019). [readcube]
Rajkomar, A., Dean, J., & Kohane I.
N. Engl. J. Med. 380:1347-1358 (2019).
Esteva, A., Robicquet, A., Ramsundar, B., Kuleshov, V., DePristo, M., Chou, K., Cui, C., Corrado, G., Thrun, S. & Dean, J.
Nat. Med. 25, 24–29 (2019). [readcube]
Rough K, Thompson J.
Ophthalmology. 125(8):1136-1138 (2018).
Wachter, R. M., Howell, M. D.
JAMA 320(1):25-26 (2018).
Cross-Specialty Applied AI
by Krishnamurthy (Dj) Dvijotham & Taylan Cemgil
Google Deepmind | 17-Jul-2023
by Shekoofeh Azizi & Laura Culp
Google Research Blog | 26-Apr-2023
by Alex D’Amour & Katherine Heller
Google Research Blog | 18-Oct-2021
by Shekoofeh Azizi
Google Research Blog | 13-Oct-2021
Publications
Reinke, A., Tizabi, M. D., Baumgartner, M., Eisenmann, M., Heckmann-Nötzel, D., Kavur, A. E., Rädsch, T., Sudre, C. H., Acion, L., Antonelli, M., Arbel, T., Bakas, S., Benis, A., Buettner, F., Cardoso, M. J., Cheplygina, V., Chen, J., Christodoulou, E., Cimini, B. A., Farahani, K., Ferrer, L., Galdran, A., van Ginneken, B., Glocker, B., Godau, P., Hashimoto, D. A., Hoffman, M. M., Huisman, M., Isensee, F., Jannin, P., Kahn, C. E., Kainmueller, D., Kainz, B., Karargyris, A., Kleesiek, J., Kofler, F., Kooi, T., Kopp-Schneider, A., Kozubek, M., Kreshuk, A., Kurc, T., Landman, B. A., Litjens, G., Madani, A., Maier-Hein, K., Martel, A. L., Meijering, E., Menze, B., Moons, K. G. M., Müller, H., Nichyporuk, B., Nickel, F., Petersen, J., Rafelski, S. M., Rajpoot, N., Reyes, M., Riegler, M. A., Rieke, N., Saez-Rodriguez, J., Sánchez, C. I., Shetty, S., Summers, R. M., Taha, A. A., Tiulpin, A., Tsaftaris, S. A., Van Calster, B., Varoquaux, G., Yaniv, Z. R., Jäger, P. F. & Maier-Hein, L.
Nat. Methods 21, 182–194 (2024). [readcube]
Brown, A., Tomasev, N., Freyberg, J., Liu, Y., Karthikesalingam, A. & Schrouff, J.
Nat. Commun. 14, 4314 (2023).
Dvijotham, K., Winkens, J., Barsbey, M., Ghaisas, S., Stanforth, R., Pawlowski, N., Strachan, P., Ahmed, Z., Azizi, S., Bachrach, Y., Culp, L., Daswani, M., Freyberg, J., Kelly, C., Kiraly, A., Kohlberger, T., McKinney, S., Mustafa, B., Natarajan, V., Geras, K., Witowski, J., Qin, Z. Z., Creswell, J., Shetty, S., Sieniek, M., Spitz, T., Corrado, G., Kohli, P., Cemgil, T. & Karthikesalingam, A.
Nat. Med. 1–7 (2023).
Azizi, S., Culp, L., Freyberg, J., Mustafa, B., Baur, S., Kornblith, S., Chen, T., Tomasev, N., Mitrović, J., Strachan, P., Mahdavi, S. S., Wulczyn, E., Babenko, B., Walker, M., Loh, A., Chen, P.-H. C., Liu, Y., Bavishi, P., McKinney, S. M., Winkens, J., Roy, A. G., Beaver, Z., Ryan, F., Krogue, J., Etemadi, M., Telang, U., Liu, Y., Peng, L., Corrado, G. S., Webster, D. R., Fleet, D., Hinton, G., Houlsby, N., Karthikesalingam, A., Norouzi, M. & Natarajan, V.
Nature Biomedical Engineering 1–24 (2023). [readcube]
Schrouff, J., Harris, N., Koyejo, O. O., Alabdulmohsin, I., Schnider, E., Opsahl-Ong, K., Brown, A., Roy, S., Mincu, D., Chen, C., Dieng, A., Liu, Y., Natarajan, V., Karthikesalingam, A., Heller, K. A., Chiappa, S. & D’Amour, A.
NeurIPS (2022).
McKinney, S. M.
medRxiv (2022).
Freeman, B., Hammel, N., Phene, S., Huang, A., Ackermann, R., Kanzheleva, O., Hutson, M., Taggart, C., Duong, Q. & Sayres, R.
HCOMP 9, 60–71 (2021).
Azizi, S., Mustafa, B., Ryan, F., Beaver, Z., Freyberg, J., Deaton, J., Loh, A., Karthikesalingam, A., Kornblith, S., Chen, T., Natarajan, V. & Norouzi, M.
Proceedings of the IEEE/CVF International Conference on Computer Vision (ICCV) 3478–3488 (2021).
Sadilek, A., Liu, L., Nguyen, D., Kamruzzaman, M., Serghiou, S., Rader, B., Ingerman, A., Mellem, S., Kairouz, P., Nsoesie, E. O., MacFarlane, J., Vullikanti, A., Marathe, M., Eastham, P., Brownstein, J. S., Arcas, B. A. Y., Howell, M. D. & Hernandez, J.
NPJ Digit Med 4, 132 (2021).
Mustafa, B., Loh, A., Freyberg, J., MacWilliams, P., Karthikesalingam, A., Houlsby, N. & Natarajan, V.
arXiv [cs.CV] (2021).
arXiv [eess.IV] (2021).
D’Amour, A., Heller, K., Moldovan, D., Adlam, B., Alipanahi, B., Beutel, A., Chen, C., Deaton, J., Eisenstein, J., Hoffman, M. D., Hormozdiari, F., Houlsby, N., Hou, S., Jerfel, G., Karthikesalingam, A., Lucic, M., Ma, Y., McLean, C., Mincu, D., Mitani, A., Montanari, A., Nado, Z., Natarajan, V., Nielson, C., Osborne, T. F., Raman, R., Ramasamy, K., Sayres, R., Schrouff, J., Seneviratne, M., Sequeira, S., Suresh, H., Veitch, V., Vladymyrov, M., Wang, X., Webster, K., Yadlowsky, S., Yun, T., Zhai, X. & Sculley, D.
arXiv [cs.LG] (2020).
Winkens, J., Bunel, R., Roy, A. G., Stanforth, R., Natarajan, V., Ledsam, J. R., MacWilliams, P., Kohli, P., Karthikesalingam, A., Kohl, S., Cemgil, T., Ali Eslami, S. M. & Ronneberger, O.
Hartman, T., Howell, M., Dean, J., Hoory, S., Slyper, R., Laish, I., Gilon, O, Vainstein, D., Corrado, G., Chou, K., Po, M., Williams, J., Ellis, S., Bee, G., Hassidim, A., Amira, R., Beryozkin, G., Szpektor, I., & Matias, Y.
BMC (2020).
Dermatology
by Pooja Rao
Google Research Blog | 19-Mar-2024
by Mike Schaekermann & Ivor Horn
Google Research Blog | 15-Mar-2024
by Dave Steiner & Rory Pilgrim
Google Research Blog | 8-Mar-2024
by Lou Wang
Google Keyword Blog | 14-Jun-2023
Google Keyword Blog | 08-Feb-2022
by Abhijit Guha Roy & Jie Ren
Google Research Blog | 27-Jan-2022
by Miles Hutson & Aaron Loh
TensorFlow Blog | 11-Oct-2021
by Peggy Bui & Yuan Liu
Google Keyword Blog | 18-May-2021
by Ayush Jain & Peggy Bui
Google Keyword Blog | 28-Apr-2021
by Timo Kohlberger & Yuan Liu
Google Research Blog | 19-Feb-2020
by Yuan Liu & Peggy Bui
Google Research Blog | 12-Sep-2019
Schaekermann, M., Spitz, T., Pyles, M., Cole-Lewis, H., Wulczyn, E., Pfohl, S. R., Martin, D., Jr, Jaroensri, R., Keeling, G., Liu, Y., Farquhar, S., Xue, Q., Lester, J., Hughes, C., Strachan, P., Tan, F., Bui, P., Mermel, C. H., Peng, L. H., Matias, Y., Corrado, G. S., Webster, D. R., Virmani, S., Semturs, C., Liu, Y., Horn, I. & Cameron Chen, P.-H.
eClinicalMedicine (2024).
Rikhye, R. V., Hong, G. E., Singh, P., Smith, M. A., Loh, A., Muralidharan, V., Wong, D., Sayres, R., Phung, M., Betancourt, N., Fong, B., Sahasrabudhe, R., Nasim, K., Eschholz, A., Matias, Y., Corrado, G. S., Chou, K., Webster, D. R., Bui, P., Liu, Y., Liu, Y., Ko, J. & Lin, S.
Mayo Clinic Proceedings: Digital Health (2024).
Alabdulmohsin, I.M., Schrouff, J., Koyejo, S.
35. NeurIPS (2022).
Vemulapalli, R., Morningstar, W. R., Mansfield, P. A., Eichner, H., Singhal, K., Afkanpour, A. & Green, B.
arXiv [cs.LG] (2022).
Huang, S. J., Liu, Y., Kanada, K., Corrado, G. S., Webster, D. R., Peng, L., Bui, P. & Liu, Y.
Skin Health and Disease (2021).
Guha Roy, A., Ren, J., Azizi, S., Loh, A., Natarajan, V., Mustafa, B., Pawlowski, N., Freyberg, J., Liu, Y., Beaver, Z., Vo, N., Bui, P., Winter, S., MacWilliams, P., Corrado, G. S., Telang, U., Liu, Y., Cemgil, T., Karthikesalingam, A., Lakshminarayanan, B. & Winkens, J.
Med. Image Analysis. 75, 102274 (2021). [ reading link ]
Weng, W.-H., Deaton, J., Natarajan, V., Elsayed, G. F. & Liu, Y.
JAMA Netw Open 4, e217249–e217249 (2021).
Machine Learning for Health NeurIPS Workshop (ML4H), PMLR 136:415-429 (2020).
Singh, N., Lee, K., Coz, D., Angermueller, C., Huang, S., Loh, A. & Liu, Y.
in 2020 IEEE/CVF Conference on Computer Vision and Pattern Recognition Workshops (CVPRW) 3172–3181 (2020).
Liu, Y., Jain, A., Eng, C., Way, D. H., Lee, K., Bui, P., Kanada, K., de Oliveira Marinho, G., Gallegos, J., Gabriele, S., Gupta, V., Singh, N., Natarajan, V., Hofmann-Wellenhof, R., Corrado, G. S., Peng, L. H., Webster, D. R., Ai, D., Huang, S., Liu, Y., Carter Dunn, R. & Coz, D.
Nat. Med. (2020). [readcube]
Ghorbani, A., Natarajan, V., Coz, D. & Liu, Y.
Machine Learning for Health NeurIPS Workshop (ML4H), PMLR 116:155-170 (2020).
Eng, C., Liu, Y. & Bhatnagar, R.
Br. J. Dermatol. (2019). readcube
by Yossi Matias & Ehud Rivlin
Google Research Blog | 5-Aug-2021
by Robin Suchan
Verily Press | 5-Aug-2021
by Daniel Freedman & Ehud Rivlin
Google Research Blog | 28-Aug-2020
Golany, T., Aides, A., Freedman, D., Rabani, N., Liu, Y., Rivlin, E., Corrado, G. S., Matias, Y., Khoury, W., Kashtan, H. & Reissman, P.
Surg. Endosc. (2022).
Livovsky, D. M., Veikherman, D., Golany, T., Aides, A., Dashinsky, V., Rabani, N., Ben Shimol, D., Blau, Y., Katzir, L., Shimshoni, I., Liu, Y., Segol, O., Goldin, E., Corrado, G., Lachter, J., Matias, Y., Rivlin, E. & Freedman, D.
Gastrointest. Endosc. (2021).
Freedman, D., Blau, Y., Katzir, L., Aides, A., Shimshoni, I., Veikherman, D., Golany, T., Gordon, A., Corrado, G., Matias, Y. & Rivlin, E.
IEEE Trans. Med. Imaging 1–1 (2020).
Epidemiological Forecasting
by Joel Shor & Sercan Arik
by Tomas Pfister
Google Cloud Blog | 15-Nov-2020
by Dario Sava
Google Cloud Blog | 3-Aug-2020
Tsai, T. C., Arik, S., Jacobson, B. H., Yoon, J., Yoder, N., Sava, D., Mitchell, M., Graham, G. & Pfister, T.
NPJ Digit Med 5, 59 (2022).
Cramer, E. Y., Ray, E. L., Lopez, V. K., Bracher, J., Brennen, A., Castro Rivadeneira, A. J., Gerding, A., Gneiting, T., House, K. H., Huang, Y., Jayawardena, D., Kanji, A. H., Khandelwal, A., Le, K., Mühlemann, A., Niemi, J., Shah, A., Stark, A., Wang, Y., Wattanachit, N., Zorn, M. W., Gu, Y., Jain, S., Bannur, N., Deva, A., Kulkarni, M., Merugu, S., Raval, A., Shingi, S., Tiwari, A., White, J., Abernethy, N. F., Woody, S., Dahan, M., Fox, S., Gaither, K., Lachmann, M., Meyers, L. A., Scott, J. G., Tec, M., Srivastava, A., George, G. E., Cegan, J. C., Dettwiller, I. D., England, W. P., Farthing, M. W., Hunter, R. H., Lafferty, B., Linkov, I., Mayo, M. L., Parno, M. D., Rowland, M. A., Trump, B. D., Zhang-James, Y., Chen, S., Faraone, S. V., Hess, J., Morley, C. P., Salekin, A., Wang, D., Corsetti, S. M., Baer, T. M., Eisenberg, M. C., Falb, K., Huang, Y., Martin, E. T., McCauley, E., Myers, R. L., Schwarz, T., Sheldon, D., Gibson, G. C., Yu, R., Gao, L., Ma, Y., Wu, D., Yan, X., Jin, X., Wang, Y.-X., Chen, Y., Guo, L., Zhao, Y., Gu, Q., Chen, J., Wang, L., Xu, P., Zhang, W., Zou, D., Biegel, H., Lega, J., McConnell, S., Nagraj, V. P., Guertin, S. L., Hulme-Lowe, C., Turner, S. D., Shi, Y., Ban, X., Walraven, R., Hong, Q.-J., Kong, S., van de Walle, A., Turtle, J. A., Ben-Nun, M., Riley, S., Riley, P., Koyluoglu, U., DesRoches, D., Forli, P., Hamory, B., Kyriakides, C., Leis, H., Milliken, J., Moloney, M., Morgan, J., Nirgudkar, N., Ozcan, G., Piwonka, N., Ravi, M., Schrader, C., Shakhnovich, E., Siegel, D., Spatz, R., Stiefeling, C., Wilkinson, B., Wong, A., Cavany, S., España, G., Moore, S., Oidtman, R., Perkins, A., Kraus, D., Kraus, A., Gao, Z., Bian, J., Cao, W., Lavista Ferres, J., Li, C., Liu, T.-Y., Xie, X., Zhang, S., Zheng, S., Vespignani, A., Chinazzi, M., Davis, J. T., Mu, K., Pastore Y Piontti, A., Xiong, X., Zheng, A., Baek, J., Farias, V., Georgescu, A., Levi, R., Sinha, D., Wilde, J., Perakis, G., Bennouna, M. A., Nze-Ndong, D., Singhvi, D., Spantidakis, I., Thayaparan, L., Tsiourvas, A., Sarker, A., Jadbabaie, A., Shah, D., Della Penna, N., Celi, L. A., Sundar, S., Wolfinger, R., Osthus, D., Castro, L., Fairchild, G., Michaud, I., Karlen, D., Kinsey, M., Mullany, L. C., Rainwater-Lovett, K., Shin, L., Tallaksen, K., Wilson, S., Lee, E. C., Dent, J., Grantz, K. H., Hill, A. L., Kaminsky, J., Kaminsky, K., Keegan, L. T., Lauer, S. A., Lemaitre, J. C., Lessler, J., Meredith, H. R., Perez-Saez, J., Shah, S., Smith, C. P., Truelove, S. A., Wills, J., Marshall, M., Gardner, L., Nixon, K., Burant, J. C., Wang, L., Gao, L., Gu, Z., Kim, M., Li, X., Wang, G., Wang, Y., Yu, S., Reiner, R. C., Barber, R., Gakidou, E., Hay, S. I., Lim, S., Murray, C., Pigott, D., Gurung, H. L., Baccam, P., Stage, S. A., Suchoski, B. T., Prakash, B. A., Adhikari, B., Cui, J., Rodríguez, A., Tabassum, A., Xie, J., Keskinocak, P., Asplund, J., Baxter, A., Oruc, B. E., Serban, N., Arik, S. O., Dusenberry, M., Epshteyn, A., Kanal, E., Le, L. T., Li, C.-L., Pfister, T., Sava, D., Sinha, R., Tsai, T., Yoder, N., Yoon, J., Zhang, L., Abbott, S., Bosse, N. I., Funk, S., Hellewell, J., Meakin, S. R., Sherratt, K., Zhou, M., Kalantari, R., Yamana, T. K., Pei, S., Shaman, J., Li, M. L., Bertsimas, D., Skali Lami, O., Soni, S., Tazi Bouardi, H., Ayer, T., Adee, M., Chhatwal, J., Dalgic, O. O., Ladd, M. A., Linas, B. P., Mueller, P., Xiao, J., Wang, Y., Wang, Q., Xie, S., Zeng, D., Green, A., Bien, J., Brooks, L., Hu, A. J., Jahja, M., McDonald, D., Narasimhan, B., Politsch, C., Rajanala, S., Rumack, A., Simon, N., Tibshirani, R. J., Tibshirani, R., Ventura, V., Wasserman, L., O’Dea, E. B., Drake, J. M., Pagano, R., Tran, Q. T., Ho, L. S. T., Huynh, H., Walker, J. W., Slayton, R. B., Johansson, M. A., Biggerstaff, M. & Reich, N. G.
Proc. Natl. Acad. Sci. U. S. A. 119, e2113561119 (2022).
Arık, S. Ö., Shor, J., Sinha, R., Yoon, J., Ledsam, J. R., Le, L. T., Dusenberry, M. W., Yoder, N. C., Popendorf, K., Epshteyn, A., Euphrosine, J., Kanal, E., Jones, I., Li, C.-L., Luan, B., Mckenna, J., Menon, V., Singh, S., Sun, M., Ravi, A. S., Zhang, L., Sava, D., Cunningham, K., Kayama, H., Tsai, T., Yoneoka, D., Nomura, S., Miyata, H. & Pfister, T.
NPJ Digit Med 4, 146 (2021).
Kapoor, A., Ben, X., Liu, L., Perozzi, B., Barnes, M., Blais, M. & O’Banion, S.
Arik, Li, Yoon, Sinha, Epshteyn, Le, Menon, Singh, Zhang, Nikoltchev, Sonthalia, Nakhost, Kanal & Pfister.
Adv. Neural Inf. Process. Syst. 2020.
Eye Diseases
by Michelle Budzyna & Molly McHugh-Johnson
Google Keyword Blog | 27-Sep-2023
by Kasumi Widner
Google Keyword Blog | 30-May-2023
by Angus Turner
Google Australia Blog | 7-Mar-2023
by Emma Beede
Google Keyword Blog | 25-Apr-2020
by Kasumi Widner & Sunny Virmani
Google Keyword Blog | 25-Feb-2019
Verily Blog | 25-Feb-2019
by Rory Sayres & Jonathan Krause
Google Research Blog | 13-Dec-2018
by Mustafa Suleyman
DeepMind Blog | 13-Aug-2018
by Lily Peng
Google Keyword Blog | 29-Nov-2016
by Lily Peng & Varun Gulshan
Google Research Blog | 29-Nov-2016
Bora, A., Tiwari, R., Bavishi, P., Virmani, S., Huang, R., Traynis, I., Corrado, G. S., Peng, L., Webster, D. R., Varadarajan, A. V., Pattanapongpaiboon, W., Chopra, R. & Ruamviboonsuk, P.
Transl. Vis. Sci. Technol. 12, 11 (2023).
Widner, K., Virmani, S., Krause, J., Nayar, J., Tiwari, R., Pedersen, E. R., Jeji, D., Hammel, N., Matias, Y., Corrado, G. S., Liu, Y., Peng, L. & Webster, D. R.
Nat. Med. 1–3 (2023). [readcube]
Srisubat, A., Kittrongsiri, K., Sangroongruangsri, S., Khemvaranan, C., Shreibati, J. B., Ching, J., Hernandez, J., Tiwari, R., Hersch, F., Liu, Y., Hanutsaha, P., Ruamviboonsuk, V., Turongkaravee, S., Raman, R. & Ruamviboonsuk, P.
Ophthalmol Ther (2023).
Chia, M. A., Hersch, F., Sayres, R., Bavishi, P., Tiwari, R., Keane, P. A. & Turner, A. W.
Br. J. Ophthalmol. (2023).
Ruamviboonsuk, P., Tiwari, R., Sayres, R., Nganthavee, V., Hemarat, K., Kongprayoon, A., Raman, R., Levinstein, B., Liu, Y., Schaekermann, M., Lee, R., Virmani, S., Widner, K., Chambers, J., Hersch, F., Peng, L. & Webster, D. R.
The Lancet Digital Health (2022).
Pedersen Elin Rønby, Cuadros Jorge, Khan Mahbuba, Fleischmann Sybille, Wolff Gregory, Hammel Naama, Liu Yun & Leung Geoffrey.
NEJM Catalyst (2021).
Wilson, M., Chopra, R., Wilson, M. Z., Cooper, C., MacWilliams, P., Liu, Y., Wulczyn, E., Florea, D., Hughes, C. O., Karthikesalingam, A., Khalid, H., Vermeirsch, S., Nicholson, L., Keane, P. A., Balaskas, K. & Kelly, C. J.
JAMA Ophthalmol. (2021).
Limwattanayingyong, J., Nganthavee, V., Seresirikachorn, K., Singalavanija, T., Soonthornworasiri, N., Ruamviboonsuk, V., Rao, C., Raman, R., Grzybowski, A., Schaekermann, M., Peng, L. H., Webster, D. R., Semturs, C., Krause, J., Sayres, R., Hersch, F., Tiwari, R., Liu, Y. & Ruamviboonsuk, P.
Journal of Diabetes Research, 1–8 (2020).
Hsu J, Phene S, Mitani A, Luo J, Hammel N, Krause J, Sayres R.
in ACM-CHIL [arXiv] (2020)
Bresnick, G., Cuadros, J. A., Khan, M., Fleischmann, S., Wolff, G., Limon, A., Chang, J., Jiang, L., Cuadros, P. & Pedersen, E. R.
BMJ Open Diabetes Research and Care 8, e001154 (2020).
Beede, E., Baylor, E., Hersch, F., Iurchenko, A., Wilcox, L., Ruamviboonsuk, P. & Vardoulakis, L. M.
Proceedings of the 2020 CHI Conference on Human Factors in Computing Systems 1–12. Association for Computing Machinery (2020).
Schaekermann, M., Cai, C. J., Huang, A. E. & Sayres, R.
Proceedings of the 2020 CHI Conference on Human Factors in Computing Systems 1–13. Association for Computing Machinery (2020).
Phene, S., Dunn, R. C., Hammel, N., Liu, Y., Krause, J., Kitade, N., Schaekermann, M., Sayres, R., Wu, D. J., Bora, A., Semturs, C., Misra, A., Huang, A. E., Spitze, A., Medeiros, F. A., Maa, A. Y., Gandhi, M., Corrado, G. S., Peng, L. & Webster, D. R.
Ophthalmology 126, 1627–1639 (2019).
Schaekermann, M., Hammel, N., Terry, M., Ali, T. K., Liu, Y., Basham, B., Campana, B., Chen, W., Ji, X., Krause, J., Corrado, G. S., Peng, L., Webster, D. R., Law, E. & Sayres, R.
Transl. Vis. Sci. Technol. 8, 40 (2019).
Gulshan, V., Rajan, R. P., Widner, K., Wu, D., Wubbels, P., Rhodes, T., Whitehouse, K., Coram, M., Corrado, G., Ramasamy, K., Raman, R., Peng, L. & Webster, D. R.
JAMA Ophthalmol. (2019).
Ruamviboonsuk, P., Krause, J., Chotcomwongse, P., Sayres, R., Raman, R., Widner, K., Campana, B. J. L., Phene, S., Hemarat, K., Tadarati, M., Silpa-Archa, S., Limwattanayingyong, J., Rao, C., Kuruvilla, O., Jung, J., Tan, J., Orprayoon, S., Kangwanwongpaisan, C., Sukumalpaiboon, R., Luengchaichawang, C., Fuangkaew, J., Kongsap, P., Chualinpha, L., Saree, S., Kawinpanitan, S., Mitvongsa, K., Lawanasakol, S., Thepchatri, C., Wongpichedchai, L., Corrado, G. S., Peng, L. & Webster, D. R.
npj Digit Med 2, 25 (2019).
Sayres, R., Taly, A., Rahimy, E., Blumer, K., Coz, D., Hammel, N., Krause, J., Narayanaswamy, A., Rastegar, Z., Wu, D., Xu, S., Barb, S., Joseph, A., Shumski, M., Smith, J., Sood, A. B., Corrado, G. S., Peng, L. & Webster, D. R.
Ophthalmology 126, 552–564 (2019).
Fauw, J., Ledsam, J.R., Romera-Paredes, B., Nikolov, S., Tomasev, N., Blackwell, S., Askham, H., Glorot, X., O’Donoghue, B., Visentin, D., van den Driessche, G., Lakshminarayanan, B., Meyer, C., Mackinder, F., Bouton, S., Ayoub, K., Chopra, R., King, D., Karthikesalingam, A., Hughes, C.O., Raine, R., Hughes, J., Sim, D. A., Egan, C., Tufail, A., Montgomery, H., Hassabis, D., Rees, G., Back, T., Khaw, P.T., Suleyman, M., Cornebise, J., Keane, P.A., & Ronneberger, O.
Nat. Med. 24, 1342–1350 (2018). [readcube]
Krause, J., Gulshan, V., Rahimy, E., Karth, P., Widner, K., Corrado, G. S., Peng, L., & Webster, D.R.
Ophthalmology 125, 1264–1272 (2018). [arXiv]
Bouskill, K., Smith-Morris, C., Bresnick, G., Cuadros, J. & Pedersen, E. R.
BMC Health Services Research 18, (2018).
Smith-Morris, C., Bresnick, G. H., Cuadros, J., Bouskill, K. E. & Pedersen, E. R.
Med. Anthropol. 39, 109–122 (2018).
Guan, M., Gulshan, V., Dai, A, Hinton, G.
AAAI Conference on Artificial Intelligence (2018).
Gulshan, V., Peng, L., Coram, M., Stumpe, M. C., Wu, D., Narayanaswamy, A., Venugopalan, S., Widner, K., Madams, T., Cuadros, J., Ramasamy, K., Nelson, P., Mega, J., & Webster, D.
JAMA 316, 2402–2410 (2016).
Blog Posts [more at Fitbit Blog ]
Google Keyword Blog | 6-Mar-2024
by Mike Darling
Google Keyword Blog | 24-Jan-2024
by Javier L. Prieto
Google Keyword Blog | 17-Jan-2024
by Amy McDonough
Google Keyword Blog | 26-Oct-2023
Google Keyword Blog | 19-Oct-2023
by Sandeep Waraich
Google Keyword Blog | 4-Oct-2023
by TJ Varghese
Google Keyword Blog | 28-Sep-2023
by Maggie Stanphill & Bhanu Narasimhan
Google Keyword Blog | 1-Aug-2023
by Elena Perez & Samy Abdel-Ghaffer
Google Keyword Blog | 2-Jun-2023
by Elena Perez
Google Keyword Blog | 4-May-2023
Google Keyword Blog | 16-Mar-2023
by Zahra Barnes
Google Keyword Blog | 20-Dec-2022
Google Keyword Blog | 6-Oct-2022
Google Keyword Blog | 29-Sep-2022
Google Keyword Blog | 24-Aug-2022
by The Fitbit Team
Google Keyword Blog | 22-Jun-2022
Google Keyword Blog | 11-Apr-2022
Speed, C., Arneil, T., Harle, R., Wilson, A., Karthikesalingam, A., McConnell, M. & Phillips, J.
PLOS Digit Health 2, e0000236 (2023).
Lubitz, S. A., Faranesh, A. Z., Selvaggi, C., Atlas, S. J., McManus, D. D., Singer, D. E., Pagoto, S., McConnell, M. V., Pantelopoulos, A. & Foulkes, A. S.
Circulation (2022).
Natarajan, A., Su, H.-W. & Heneghan, C.
Front. Physiol. 13, 898251 (2022).
Natarajan, A., Su, H.-W., Heneghan, C., Blunt, L., O’Connor, C. & Niehaus, L.
NPJ Digit Med 4, 136 (2021).
Blog Posts [more at DeepVariant Blog ]
by Andrew Carroll
Google Keyword Blog | 10-May-2023
by Andrew Carroll & Kishwar Shafin
Google Research Blog | 10-May-2023
Babak Behsaz & Andrew Carroll
Google Research Blog | 27-Apr-2023
by Sara Ahadi & Andrew Carroll
Google Research Blog | 11-Apr-2023
Google Keyword Blog | 26-Oct-2022
by Andrew Carroll & Pi-Chuan Chang
Google Keyword Blog | 13-Jan-2022
by Farhad Hormozdiari & Andrew Carroll
Google Open Source Blog | 11-Jan-2022
by Andrew Carroll & Cory McLean
Google Research Blog | 23-Jun-2021
Google Research Blog | 18-Sep-2020
by Pi-Chuan Chang & Lizzie Dorfman
Google Research Blog | 19-Apr-2018
by Mark DePristo & Ryan Poplin
Google Research Blog | 4-Dec-2017
by Phing Lee
Google Keyword Blog | 17-Nov-2017
Kolmogorov, M., Billingsley, K. J., Mastoras, M., Meredith, M., Monlong, J., Lorig-Roach, R., Asri, M., Alvarez Jerez, P., Malik, L., Dewan, R., Reed, X., Genner, R. M., Daida, K., Behera, S., Shafin, K., Pesout, T., Prabakaran, J., Carnevali, P., Yang, J., Rhie, A., Scholz, S. W., Traynor, B. J., Miga, K. H., Jain, M., Timp, W., Phillippy, A. M., Chaisson, M., Sedlazeck, F. J., Blauwendraat, C. & Paten, B.
Nat. Methods 20, 1483–1492 (2023).
Rhie, A., Nurk, S., Cechova, M., Hoyt, S. J., Taylor, D. J., Altemose, N., Hook, P. W., Koren, S., Rautiainen, M., Alexandrov, I. A., Allen, J., Asri, M., Bzikadze, A. V., Chen, N.-C., Chin, C.-S., Diekhans, M., Flicek, P., Formenti, G., Fungtammasan, A., Garcia Giron, C., Garrison, E., Gershman, A., Gerton, J. L., Grady, P. G. S., Guarracino, A., Haggerty, L., Halabian, R., Hansen, N. F., Harris, R., Hartley, G. A., Harvey, W. T., Haukness, M., Heinz, J., Hourlier, T., Hubley, R. M., Hunt, S. E., Hwang, S., Jain, M., Kesharwani, R. K., Lewis, A. P., Li, H., Logsdon, G. A., Lucas, J. K., Makalowski, W., Markovic, C., Martin, F. J., Mc Cartney, A. M., McCoy, R. C., McDaniel, J., McNulty, B. M., Medvedev, P., Mikheenko, A., Munson, K. M., Murphy, T. D., Olsen, H. E., Olson, N. D., Paulin, L. F., Porubsky, D., Potapova, T., Ryabov, F., Salzberg, S. L., Sauria, M. E. G., Sedlazeck, F. J., Shafin, K., Shepelev, V. A., Shumate, A., Storer, J. M., Surapaneni, L., Taravella Oill, A. M., Thibaud-Nissen, F., Timp, W., Tomaszkiewicz, M., Vollger, M. R., Walenz, B. P., Watwood, A. C., Weissensteiner, M. H., Wenger, A. M., Wilson, M. A., Zarate, S., Zhu, Y., Zook, J. M., Eichler, E. E., O’Neill, R. J., Schatz, M. C., Miga, K. H., Makova, K. D. & Phillippy, A. M.
Nature (2023).
Liao, W.-W., Asri, M., Ebler, J., Doerr, D., Haukness, M., Hickey, G., Lu, S., Lucas, J. K., Monlong, J., Abel, H. J., Buonaiuto, S., Chang, X. H., Cheng, H., Chu, J., Colonna, V., Eizenga, J. M., Feng, X., Fischer, C., Fulton, R. S., Garg, S., Groza, C., Guarracino, A., Harvey, W. T., Heumos, S., Howe, K., Jain, M., Lu, T.-Y., Markello, C., Martin, F. J., Mitchell, M. W., Munson, K. M., Mwaniki, M. N., Novak, A. M., Olsen, H. E., Pesout, T., Porubsky, D., Prins, P., Sibbesen, J. A., Sirén, J., Tomlinson, C., Villani, F., Vollger, M. R., Antonacci-Fulton, L. L., Baid, G., Baker, C. A., Belyaeva, A., Billis, K., Carroll, A., Chang, P.-C., Cody, S., Cook, D. E., Cook-Deegan, R. M., Cornejo, O. E., Diekhans, M., Ebert, P., Fairley, S., Fedrigo, O., Felsenfeld, A. L., Formenti, G., Frankish, A., Gao, Y., Garrison, N. A., Giron, C. G., Green, R. E., Haggerty, L., Hoekzema, K., Hourlier, T., Ji, H. P., Kenny, E. E., Koenig, B. A., Kolesnikov, A., Korbel, J. O., Kordosky, J., Koren, S., Lee, H., Lewis, A. P., Magalhães, H., Marco-Sola, S., Marijon, P., McCartney, A., McDaniel, J., Mountcastle, J., Nattestad, M., Nurk, S., Olson, N. D., Popejoy, A. B., Puiu, D., Rautiainen, M., Regier, A. A., Rhie, A., Sacco, S., Sanders, A. D., Schneider, V. A., Schultz, B. I., Shafin, K., Smith, M. W., Sofia, H. J., Abou Tayoun, A. N., Thibaud-Nissen, F., Tricomi, F. F., Wagner, J., Walenz, B., Wood, J. M. D., Zimin, A. V., Bourque, G., Chaisson, M. J. P., Flicek, P., Phillippy, A. M., Zook, J. M., Eichler, E. E., Haussler, D., Wang, T., Jarvis, E. D., Miga, K. H., Garrison, E., Marschall, T., Hall, I. M., Li, H. & Paten, B.
Nature 617, 312–324 (2023).
Cosentino, J., Behsaz, B., Alipanahi, B., McCaw, Z. R., Hill, D., Schwantes-An, T.-H., Lai, D., Carroll, A., Hobbs, B. D., Cho, M. H., McLean, C. Y. & Hormozdiari, F.
Nat. Genet. (2023).
Liu, D., Belyaeva, A., Shafin, K., Chang, P.-C., Carroll, A. & Cook, D. E.
bioRxiv (2022).
Belyaeva, A., Shor, J., Cook, D. E., Shafin, K., Liu, D., Töpfer, A., Wenger, A. M., Rowell, W. J., Yang, H., Kolesnikov, A., McLean, C. Y., Nattestad, M., Carroll, A. & Chang, P.-C.
Yuan, B., McLean, C. Y., Hormozdiari, F. I. & Cosentino, J.
Baid, G., Cook, D. E., Shafin, K., Yun, T., Llinares-López, F., Berthet, Q., Belyaeva, A., Töpfer, A., Wenger, A. M., Rowell, W. J., Yang, H., Kolesnikov, A., Ammar, W., Vert, J.-P., Vaswani, A., McLean, C. Y., Nattestad, M., Chang, P.-C. & Carroll, A.
Nat. Biotechnol. (2022).
Wagner, J., Olson, N. D., Harris, L., Khan, Z., Farek, J., Mahmoud, M., Stankovic, A., Kovacevic, V., Yoo, B., Miller, N., Rosenfeld, J. A., Ni, B., Zarate, S., Kirsche, M., Aganezov, S., Schatz, M. C., Narzisi, G., Byrska-Bishop, M., Clarke, W., Evani, U. S., Markello, C., Shafin, K., Zhou, X., Sidow, A., Bansal, V., Ebert, P., Marschall, T., Lansdorp, P., Hanlon, V., Mattsson, C.-A., Barrio, A. M., Fiddes, I. T., Xiao, C., Fungtammasan, A., Chin, C.-S., Wenger, A. M., Rowell, W. J., Sedlazeck, F. J., Carroll, A., Salit, M. & Zook, J. M.
Cell Genom 2, (2022).
Markello, C., Huang, C., Rodriguez, A., Carroll, A., Chang, P.-C., Eizenga, J., Markello, T., Haussler, D. & Paten, B.
Genome Res. 32, 893–903 (2022).
Goenka, S. D., Gorzynski, J. E., Shafin, K., Fisk, D. G., Pesout, T., Jensen, T. D., Monlong, J., Chang, P.-C., Baid, G., Bernstein, J. A., Christle, J. W., Dalton, K. P., Garalde, D. R., Grove, M. E., Guillory, J., Kolesnikov, A., Nattestad, M., Ruzhnikov, M. R. Z., Samadi, M., Sethia, A., Spiteri, E., Wright, C. J., Xiong, K., Zhu, T., Jain, M., Sedlazeck, F. J., Carroll, A., Paten, B. & Ashley, E. A.
Gorzynski, J. E., Goenka, S. D., Shafin, K., Jensen, T. D., Fisk, D. G., Grove, M. E., Spiteri, E., Pesout, T., Monlong, J., Baid, G., Bernstein, J. A., Ceresnak, S., Chang, P.-C., Christle, J. W., Chubb, H., Dalton, K. P., Dunn, K., Garalde, D. R., Guillory, J., Knowles, J. W., Kolesnikov, A., Ma, M., Moscarello, T., Nattestad, M., Perez, M., Ruzhnikov, M. R. Z., Samadi, M., Setia, A., Wright, C., Wusthoff, C. J., Xiong, K., Zhu, T., Jain, M., Sedlazeck, F. J., Carroll, A., Paten, B. & Ashley, E. A.
N. Engl. J. Med. (2022).
Gorzynski, J. E., Goenka, S. D., Shafin, K., Jensen, T. D., Fisk, D. G., Grove, M. E., Spiteri, E., Pesout, T., Monlong, J., Bernstein, J. A., Ceresnak, S., Chang, P.-C., Christle, J. W., Chubb, H., Dunn, K., Garalde, D. R., Guillory, J., Ruzhnikov, M. R. Z., Wright, C., Wusthoff, C. J., Xiong, K., Hollander, S. A., Berry, G. J., Jain, M., Sedlazeck, F. J., Carroll, A., Paten, B. & Ashley, E. A.
Circ Genom Precis Med 15, e003591 (2022).
Sirén, J., Monlong, J., Chang, X., Novak, A. M., Eizenga, J. M., Markello, C., Sibbesen, J. A., Hickey, G., Chang, P.-C., Carroll, A., Gupta, N., Gabriel, S., Blackwell, T. W., Ratan, A., Taylor, K. D., Rich, S. S., Rotter, J. I., Haussler, D., Garrison, E. & Paten, B.
Science 374 (2021).
McCaw, Z. R., Colthurst, T., Yun, T., Furlotte, N. A., Carroll, A., Alipanahi, B., McLean, C. Y. & Hormozdiari, F.
Nat. Commun. 13, 241 (2022).
O’Connell, J., Yun, T., Moreno, M., Li, H., Litterman, N., Kolesnikov, A., Noblin, E., Chang, P.-C.,Shastri, A., Dorfman, E. H., Shringarpure, S., Auton, A., Carroll, A. & McLean, C. Y.
Communications Biology 4, 1–9 (2021).
Shafin, K., Pesout, T., Chang, P.-C., Nattestad, M., Kolesnikov, A., Goel, S., Baid, G., Kolmogorov, M., Eizenga, J. M., Miga, K. H., Carnevali, P., Jain, M., Carroll, A. & Paten, B.
Nat. Methods 18, 1322–1332 (2021). [readcube]
Baid, G., Cook, D. E., Shafin, K., Yun, T., Llinares-López, F., Berthet, Q., Wenger, A. M., Rowell, W. J., Nattestad, M., Yang, H., Kolesnikov, A., Töpfer, A., Ammar, W., Vert, J.-P., Vaswani, A., McLean, C. Y., Chang, P.-C. & Carroll, A.
bioRxiv 2021.08.31.458403 (2021).
Alipanahi, B., Hormozdiari, F., Behsaz, B., Cosentino, J., McCaw, Z. R., Schorsch, E., Sculley, D., Dorfman, E. H., Foster, P. J., Peng, L. H., Phene, S., Hammel, N., Carroll, A., Khawaja, A. P. & McLean, C. Y.
Am. J. Hum. Genet. (2021).
Yun, T., Li, H., Chang, P-C., Lin, M., Carroll, A., & McLean, C. Y.
Bioinformatics 36, 5582-5589 (2021).
Yadlowsky, S., Yun, T., McLean, C. & D’Amour, A.
arXiv [stat.ML] (2021).
Alipanahi, B., Hormozdiari, F., Behsaz, B., Cosentino, J., McCaw, Z. R., Schorsch, E., Sculley, D., Dorfman, E. H., Phene, S., Hammel, N., Carroll, A., Khawaja, A. P. & McLean, C. Y.
arXiv [q-bio.GN] (2020).
McLean, C. Y., Hwang, Y., Poplin, R. & DePristo, M. A.
Bioinformatics 35, 4389–4391 (2019).
Wenger, A. M., Peluso, P., Rowell, W. J., Chang, P.-C., Hall, R. J., Concepcion, G. T., Ebler, J., Fungtammasan, A., Kolesnikov, A., Olson, N. D., Töpfer, A., Alonge, M., Mahmoud, M., Qian, Y., Chin, C.-S., Phillippy, A. M., Schatz, M. C., Myers, G., DePristo, M. A., Ruan, J., Marschall, T., Sedlazeck, F. J., Zook, J. M., Li, H., Koren, S., Carroll, A., Rank, D. R. & Hunkapiller, M. W.
Nat. Biotechnol. 37, 1155–1162 (2019). [readcube]
Poplin, R., Chang, P.-C., Alexander, D., Schwartz, S., Colthurst, T., Ku, A., Newburger, D., Dijamco, J., Nguyen, N., Afshar, P. T., Gross, S. S., Dorfman, L., McLean, C. Y. & DePristo, M. A.
Nat. Biotechnol. 36, 983–987 (2018). [readcube]
Telenti, A., Lippert, C., Chang, P.-C. & DePristo, M.
Hum. Mol. Genet. 27, R63–R71 (2018).
Kelley, D. R., Reshef, Y. A., Bileschi, M., Belanger, D., McLean, C. Y. & Snoek, J.
Genome Res. 28, 739–750 (2018).
Google Health Studies
by Nicholas Allen
Google Keyword Blog | 23-May-2022
by Jon Morgan & Paul Eastham
Google Keyword Blog | 9-Dec-2020
Health Sensors and Signals
Google Keyword Blog | 25-Jan-2024
by Stephanie Scott
by Xiaoran "Van" Fan & Trausti Thormundsson
Google Research Blog | 27-Oct-2023
by Michael Dixon & Reena Singhal Lee
Google Research Blog | 9-Nov-2021
by Ashton Udall
Google Keyword Blog | 16-Mar-2021
Google Research Blog | 16-Mar-2021
by Shwetak Patel
Google Keyword Blog | 4-Feb-2021
Fan, X., Pearl, D., Howard, R., Shangguan, L. & Thormundsson, T. APG.
Proceedings of the 29th Annual International Conference on Mobile Computing and Networking 1–15. Association for Computing Machinery (2023).
Yang, Y., Liu, X., Wu, J., Borac, S., Katabi, D., Poh, M.-Z. & McDuff, D.
arXiv [cs.LG] (ICLR 2023).
Bae, S., Borac, S., Emre, Y., Wang, J., Wu, J., Kashyap, M., Kang, S.-H., Chen, L., Moran, M., Cannon, J., Teasley, E. S., Chai, A., Liu, Y., Wadhwa, N., Krainin, M., Rubinstein, M., Maciel, A., McConnell, M. V., Patel, S., Corrado, G. S., Taylor, J. A., Zhan, J. & Po, M. J.
bioRxiv (2021).
Dixon, M., Schneider, L. D., Yu, J., Hsu, J., Pathak, A., Shin, D., Lee, R. S., Malhotra, M., Mixter, K., McConnell, M. V., Taylor, J. A., Patel, S. N.,
Google Whitepaper (2021).
Large Language Models
Blog Posts [more at Med-PaLM site ]
by Yossi Matias
by Dr. Ivor Horn
by Alan Karthikesalingam & Vivek Natarajan
Google Research Blog | 12-Jan-2024
by Yossi Matias & Aashima Gupta
Google Cloud Blog | 13-Dec-2023
by Michael Howell
by Aashima Gupta & Greg Corrado
Google Cloud Blog | 29-Aug-2023
by Greg Corrado and Yossi Matias
Google Research Blog | 3-Aug-2023
by James Manyika & Jeff Dean
Google Keyword Blog | 25-May-2023
by Aashima Gupta & Amy Waldron
Google Cloud Blog | 13-April-2023
Pfohl, S. R., Cole-Lewis, H., Sayres, R., Neal, D., Asiedu, M., Dieng, A., Tomasev, N., Rashid, Q. M., Azizi, S., Rostamzadeh, N., McCoy, L. G., Celi, L. A., Liu, Y., Schaekermann, M., Walton, A., Parrish, A., Nagpal, C., Singh, P., Dewitt, A., Mansfield, P., Prakash, S., Heller, K., Karthikesalingam, A., Semturs, C., Barral, J., Corrado, G., Matias, Y., Smith-Loud, J., Horn, I. & Singhal, K.
arXiv [cs.CY] (2024).
Tu, T., Azizi, S., Driess, D., Schaekermann, M., Amin, M., Chang, P.-C., Carroll, A., Lau, C., Tanno, R., Ktena, I., Mustafa, B., Chowdhery, A., Liu, Y., Kornblith, S., Fleet, D., Mansfield, P., Prakash, S., Wong, R., Virmani, S., Semturs, C., Sara Mahdavi, S., Green, B., Dominowska, E., Aguera y Arcas, B., Barral, J., Webster, D., Corrado, G. S., Matias, Y., Singhal, K., Florence, P., Karthikesalingam, A. & Natarajan, V.
NEJM AI (2024).
Tu, T., Palepu, A., Schaekermann, M., Saab, K., Freyberg, J., Tanno, R., Wang, A., Li, B., Amin, M., Tomasev, N., Azizi, S., Singhal, K., Cheng, Y., Hou, L., Webson, A., Kulkarni, K., Sara Mahdavi, S., Semturs, C., Gottweis, J., Barral, J., Chou, K., Corrado, G. S., Matias, Y., Karthikesalingam, A. & Natarajan, V.
arXiv [cs.AI] (2024).
Goel, A., Gueta, A., Gilon, O., Liu, C., Erell, S., Nguyen, L. H., Hao, X., Jaber, B., Reddy, S., Kartha, R., Steiner, J., Laish, I. & Feder, A.
arXiv [cs.CL] (2023).
McDuff, D., Schaekermann, M., Tu, T., Palepu, A., Wang, A., Garrison, J., Singhal, K., Sharma, Y., Azizi, S., Kulkarni, K., Hou, L., Cheng, Y., Liu, Y., Sara Mahdavi, S., Prakash, S., Pathak, A., Semturs, C., Patel, S., Webster, D. R., Dominowska, E., Gottweis, J., Barral, J., Chou, K., Corrado, G. S., Matias, Y., Sunshine, J., Karthikesalingam, A. & Natarajan, V.
arXiv [cs.CY] (2023)
Tanno, R., Barrett, D. G. T., Sellergren, A., Ghaisas, S., Dathathri, S., See, A., Welbl, J., Singhal, K., Azizi, S., Tu, T., Schaekermann, M., May, R., Lee, R., Man, S., Ahmed, Z., Mahdavi, S., Belgrave, D., Natarajan, V., Shetty, S., Kohli, P., Huang, P.-S., Karthikesalingam, A. & Ktena, I.
arXiv [eess.IV] (2023).
Galatzer-Levy, I. R., McDuff, D., Natarajan, V., Karthikesalingam, A. & Malgaroli, M.
Xu, S., Yang, L., Kelly, C., Sieniek, M., Kohlberger, T., Ma, M., Weng, W.-H., Kiraly, A., Kazemzadeh, S., Melamed, Z., Park, J., Strachan, P., Liu, Y., Lau, C., Singh, P., Chen, C., Etemadi, M., Kalidindi, S. R., Matias, Y., Chou, K., Corrado, G. S., Shetty, S., Tse, D., Prabhakara, S., Golden, D., Pilgrim, R., Eswaran, K. & Sellergren, A.
arXiv [cs.CV] (2023).
Belyaeva, A., Cosentino, J., Hormozdiari, F., Eswaran, K., Shetty, S., Corrado, G., Carroll, A., McLean, C. Y. & Furlotte, N. A.
arXiv [q-bio.QM] (2023).
Liu, X., McDuff, D., Kovacs, G., Galatzer-Levy, I., Sunshine, J., Zhan, J., Poh, M.-Z., Liao, S., Di Achille, P. & Patel, S.
Singhal, K., Tu, T., Gottweis, J., Sayres, R., Wulczyn, E., Hou, L., Clark, K., Pfohl, S., Cole-Lewis, H., Neal, D., Schaekermann, M., Wang, A., Amin, M., Lachgar, S., Mansfield, P., Prakash, S., Green, B., Dominowska, E., Aguera y Arcas, B., Tomasev, N., Liu, Y., Wong, R., Semturs, C., Sara Mahdavi, S., Barral, J., Webster, D., Corrado, G. S., Matias, Y., Azizi, S., Karthikesalingam, A. & Natarajan, V.
Singhal, K., Azizi, S., Tu, T., Sara Mahdavi, S., Wei, J., Chung, H. W., Scales, N., Tanwani, A., Cole-Lewis, H., Pfohl, S., Payne, P., Seneviratne, M., Gamble, P., Kelly, C., Scharli, N., Chowdhery, A., Mansfield, P., Aguera y Arcas, B., Webster, D., Corrado, G. S., Matias, Y., Chou, K., Gottweis, J., Tomasev, N., Liu, Y., Rajkomar, A., Barral, J., Semturs, C., Karthikesalingam, A. & Natarajan, V.
Medical Audio
by Laurent El Shafey and Izhak Shafran
16-Aug-2019
by Julie Cattiau
Google Keyword Blog | 7-May-2019
by Katherine Chou and Chung-Cheng Chiu
Google Research Blog | 21-Nov-2017
Blankemeier, L., Baur, S., Weng, W.-H., Garrison, J., Matias, Y., Prabhakara, S., Ardila, D. & Nabulsi, Z.
arXiv [cs.LG] (2023).
Shafran, I., Du, N., Tran, L., Perry, A., Keyes, L., Knichel, M., Domin, A., Huang, L., Chen, Y., Li, G., Wang, M., El Shafey, L., Soltau, H. & Paul, J. S.
Proceedings of the Language Resources and Evaluation Conference. arXiv [cs.CL] (2020).
Du, N., Chen, K., Kannan, A., Tran, L., Chen, Y. & Shafran, I.
Proceedings of the Annual Meeting of the Association of Computational Linguistics. arXiv [cs.LG] (2019).
Rajkomar, A., Kannan, A., Chen, K., Vardoulakis, L., Chou, K., Cui, C., & Dean, J.
JAMA Intern. Med. 179, 836–838 (2019).
El Shafey, L., Soltau, H. & Shafran, I.
Proceedings of Interspeech. arXiv [cs.CL] (2019).
Du, N., Wang, M., Tran, L., Li, G. & Shafran, I.
Proc. Empirical Methods in Natural Language Processing. arXiv [cs.CL] (2019).
Chiu, C.-C., Tripathi, A., Chou, K., Co, C., Jaitly, N., Jaunzeikare, D., Kannan, A., Nguyen, P., Sak, H., Sankar, A., Tansuwan, J., Wan, N., Wu, Y., & Zhang X.
arXiv [cs.CL] (2017).
Medical Records
by Alvin Rajkoma and Eric Loreaux
Google Research Blog | 24-Jan-2023
by Jinsung Yoon and Sercan O. Arik
Google Research Blog | 21-Dec-2022
by Subhrajit Roy & Diana Mincu
Google Research Blog | 22-Jul-2021
by Kathryn Rough & Alvin Rajkomar
Google Research Blog | 2-Apr-2020
by Alvin Rajkomar & Eyal Oren
Google Research Blog | 22-Jan-2019
by Demis Hassabis & Mustafa Suleyman & Dominic King
DeepMind Blog | 13-Nov-2018
Google Research Blog | 8-May-2018
by Patrik Sundberg & Eyal Oren
Google Research Blog | 2-Mar-2018
Seneviratne, M. G., Li, R. C., Schreier, M., Lopez-Martinez, D., Patel, B. S., Yakubovich, A., Kemp, J. B., Loreaux, E., Gamble, P., El-Khoury, K., Vardoulakis, L., Wong, D., Desai, J., Chen, J. H., Morse, K. E., Downing, N. L., Finger, L. T., Chen, M.-J. & Shah, N.
BMJ Health Care Inform 29, (2022).
Loreaux, E., Yu, K., Kemp, J., Seneviratne, M., Chen, C., Roy, S., Protsyuk, I., Harris, N., D’Amour, A., Yadlowsky, S. & Chen, M.-J.
Rajkomar, A., Loreaux, E., Liu, Y., Kemp, J., Li, B., Chen, M.-J., Zhang, Y., Mohiuddin, A. & Gottweis, J.
Nat. Commun. 13, 7456 (2022).
Stupp, D., Barequet, R., Lee, I.-C., Oren, E., Feder, A., Benjamini, A., Hassidim, A., Matias, Y., Ofek, E. & Rajkomar, A.
Roy, S., Mincu, D., Loreaux, E., Mottram, A., Protsyuk, I., Harris, N., Xue, Y., Schrouff, J., Montgomery, H., Connell, A., Tomasev, N., Karthikesalingam, A. & Seneviratne, M.
J. Am. Med. Inform. Assoc. (2021).
Tomašev, N., Harris, N., Baur, S., Mottram, A., Glorot, X., Rae, J. W., Zielinski, M., Askham, H., Saraiva, A., Magliulo, V., Meyer, C., Ravuri, S., Protsyuk, I., Connell, A., Hughes, C. O., Karthikesalingam, A., Cornebise, J., Montgomery, H., Rees, G., Laing, C., Baker, C. R., Osborne, T. F., Reeves, R., Hassabis, D., King, D., Suleyman, M., Back, T., Nielson, C., Seneviratne, M. G., Ledsam, J. R. & Mohamed, S.
Nat. Protoc. 1–23 (2021). [readcube]
Xue Y, Du N, Mottram A, Seneviratne A, Dai AM.
NeurIPS (2020).
Xue Y, Zhou D, Du N, Dai A, Xu Z, Zhang K, Cui C.
KDD (2020).
Choi E, Xu Z, Li Y, Dusenberry MW, Flores G, Xue Y, Dai AM.
AAAI (2020).
Dusenberry MW, Tran D, Choi E, Kemp J, Nixon J, Jerfel G, Heller K, & Dai AM.
ACM CHIL (2020).
Hardt M, Rajkomar A, Flores G, Dai A, Howell M, Corrado G, Cui C, & Hardt M.
Rough, K., Dai, A. M., Zhang, K., Xue, Y., Vardoulakis, L. M., Cui, C., Butte, A. J., Howell, M. D. & Rajkomar, A.
Clin. Pharmacol. Ther. 108, 145–154 (2020).
Tomašev, N., Glorot, X., Rae, J. W., Zielinski, M., Askham, H., Saraiva, A., Mottram, A., Meyer, C., Ravuri, S., Protsyuk, I., Connell, A., Hughes, C. O., Karthikesalingam, A., Cornebise, J., Montgomery, H., Rees, G., Laing, C., Baker, C. R., Peterson, K., Reeves, R., Hassabis, D., King, D., Suleyman, M., Back, T., Nielson, C., Ledsam, J. R. & Mohamed, S.
Nature 572, 116–119 (2019). [readcube]
Connell, A., Montgomery, H., Martin, P., Nightingale, C., Sadeghi-Alavijeh, O., King, D., Karthikesalingam, A., Hughes, C., Back, T., Ayoub, K., Suleyman, M., Jones, G., Cross, J., Stanley, S., Emerson, M., Merrick, C., Rees, G., Laing, C. & Raine, R.
npj Digit Med 2, 67 (2019).
Connell A., Raine R., Martin P., Barbosa E.C., Morris S., Nightingale C., Sadeghi-Alavijeh O., King D., Karthikesalingam A., Hughes C., Back T., Ayoub K., Suleyman M., Jones G., Cross J., Stanley S., Emerson M., Merrick C., Rees G., Montgomery H., & Laing C.
J Med Internet Res 21(7):e13147 (2019).
Connell A, Black G, Montgomery H, Martin P, Nightingale C, King D, Karthikesalingam A, Hughes C, Back T, Ayoub K, Suleyman M, Jones G, Cross J, Stanley S, Emerson M, Merrick C, Rees G, Laing C, & Raine R.
J Med Internet Res 21(7):e13143 (2019).
Kemp J, Rajkomar A, & Dai AM.
arXiv [cs.LG] (2019).
Pfohl SR, Dai AM, & Heller K.
Xue Y, Zhou D, Du N, Dai AM, Xu Z, Zhang K,& Cui C.
Zhang K, Xue Y, Flores G, Rajkomar A, Cui C, & Dai AM.
Rajkomar A, Oren E, Chen K, Dai AM, Hajaj N, Hardt M, Liu PJ, Liu X, Marcus J, Sun M, Sundberg P, Yee H, Zhang K, Zhang Y, Flores G, Duggan GE, Irvine J, Le Q, Litsch K, Mossin A, Tansuwan J, Wang, Wexler J, Wilson J, Ludwig D, Volchenboum SL, Chou K, Pearson M, Madabushi S, Shah NH, Butte AJ, Howell MD, Cui C, Corrado GS, Dean J.
npj Digital Med 1, 18 (2018).
Novel Biomarkers
by Boris Babenko & Akib Uddin
Google Research Blog | 24-Mar-2023
by Boris Babenko & Naama Hammel
by Terry Spitz & Jim Winkens
Google Keyword Blog | 18-May-2020
by Jason Yim, Reena Chopra, Jeffrey De Fauw & Joseph Ledsam
DeepMind Blog | 18-May-2020
by Akinori Mitani
Google Keyword Blog | 28-Jan-2020
Google Research Blog | 2-Feb-2018
Lang, O., Yaya-Stupp, D., Traynis, I., Cole-Lewis, H., Bennett, C. R., Lyles, C. R., Lau, C., Irani, M., Semturs, C., Webster, D. R., Corrado, G. S., Hassidim, A., Matias, Y., Liu, Y., Hammel, N. & Babenko, B.
EBioMedicine 102, 105075 (2024).
Ahadi, S., Wilson, K. A., Jr, Babenko, B., McLean, C. Y., Bryant, D., Pritchard, O., Kumar, A., Carrera, E. M., Lamy, R., Stewart, J. M., Varadarajan, A., Berndl, M., Kapahi, P. & Bashir, A.
Elife 12, (2023).
Babenko, B., Traynis, I., Chen, C., Singh, P., Uddin, A., Cuadros, J., Daskivich, L. P., Maa, A. Y., Kim, R., Kang, E. Y.-C., Matias, Y., Corrado, G. S., Peng, L., Webster, D. R., Semturs, C., Krause, J., Varadarajan, A. V., Hammel, N. & Liu, Y.
The Lancet Digital Health (2023).
Babenko, B., Mitani, A., Traynis, I., Kitade, N., Singh, P., Maa, A. Y., Cuadros, J., Corrado, G. S., Peng, L., Webster, D. R., Varadarajan, A., Hammel, N. & Liu, Y.
Nat Biomed Eng (2022).
Liu, X., Ali, T. K., Singh, P., Shah, A., McKinney, S. M., Ruamviboonsuk, P., Turner, A. W., Keane, P. A., Chotcomwongse, P., Nganthavee, V., Chia, M., Huemer, J., Cuadros, J., Raman, R., Corrado, G. S., Peng, L., Webster, D. R., Hammel, N., Varadarajan, A. V., Liu, Y., Chopra, R. & Bavishi, P.
Ophthalmol Retina (2022).
Mitani, A., Traynis, I., Singh, P., Corrado, G. S., Webster, D. R., Peng, L. H., Varadarajan, A. V., Liu, Y. & Hammel, N.
doi:10.1101/2021.12.30.21268488
Bora, A., Balasubramanian, S., Babenko, B., Virmani, S., Venugopalan, S., Mitani, A., de Oliveira Marinho, G., Cuadros, J., Ruamviboonsuk, P., Corrado, G. S., Peng, L., Webster, D. R., Varadarajan, A. V., Hammel, N., Liu, Y. & Bavishi, P.
The Lancet Digital Health (2020). doi:10.1016/S2589-7500(20)30250-8
Moraes, G., Fu, D. J., Wilson, M., Khalid, H., Wagner, S. K., Korot, E., Ferraz, D., Faes, L., Kelly, C. J., Spitz, T., Patel, P. J., Balaskas, K., Keenan, T. D. L., Keane, P. A. & Chopra, R.
Ophthalmology (2020). doi:10.1016/j.ophtha.2020.09.025
Narayanaswamy, A., Venugopalan, S., Webster, D. R., Peng, L., Corrado, G. S., Ruamviboonsuk, P., Bavishi, P., Brenner, M., Nelson, P. C. & Varadarajan, A. V.
Medical Image Computing and Computer Assisted Intervention – MICCAI 2020 273–283 (2020). doi:10.1007/978-3-030-59710-8_27 arXiv
Yim, J., Chopra, R., Spitz, T., Winkens, J., Obika, A., Kelly, C., Askham, H., Lukic, M., Huemer, J., Fasler, K., Moraes, G., Meyer, C., Wilson, M., Dixon, J., Hughes, C., Rees, G., Khaw, P. T., Karthikesalingam, A., King, D., Hassabis, D., Suleyman, M., Back, T., Ledsam, J. R., Keane, P. A. & De Fauw, J.
Varadarajan, A. V., Bavishi, P., Ruamviboonsuk, P., Chotcomwongse, P., Venugopalan, S., Narayanaswamy, A., Cuadros, J., Kanai, K., Bresnick, G., Tadarati, M., Silpa-Archa, S., Limwattanayingyong, J., Nganthavee, V., Ledsam, J. R., Keane, P. A., Corrado, G. S., Peng, L. & Webster, D. R.
Nat. Commun. 11, 130 (2020).
Mitani, A., Huang, A., Venugopalan, S., Corrado, G. S., Peng, L., Webster, D. R., Hammel, N., Liu, Y. & Varadarajan, A. V.
Nat Biomed Eng (2019). [readcube]
Babenko, B., Balasubramanian, S., Blumer, K. E., Corrado, G. S., Peng, L., Webster, D. R., Hammel, N. & Varadarajan, A. V.
arXiv [cs.CV] (2019).
aradarajan, A.V., Poplin, R., Blumer, K., Angermueller, C., Lesdam, J., Chopra, R., Keane, P.A., Corrado, G. S., Peng, L., Webster, D. R.
Invest. Ophthalmol. Vis. Sci. 59, 2861–2868 (2018).
Poplin, R., Varadarajan, A. V., Blumer, K., Liu, Y., McConnell, M. V., Corrado, G. S., Peng, L., & Webster, D. R.
Nat. Biomed. Eng. 2, 158–164 (2018). [readcube]
Open Health Stack
Blog Posts [more at OHS Blog ]
by Yossi Mattia & Shravya Shetty
by Richa Tiwari
Open Health Stack Blog | 10-Oct-2023.
Open Health Stack Blog | 2-Sep-2023.
by Abirami Sukumaran & Omar Ismail
Google Cloud Blog | 17-Aug-2023.
by Fred Hersch
Google Keyword Blog | 14-Mar-2023.
by Katherine Chou & Sudhi Herle
Android Developers Blog | 24-Mar-2022
by Fred Hersch & Jing Tang
Mehl, G. L., Seneviratne, M. G., Berg, M. L., Bidani, S., Distler, R. L., Gorgens, M., Kallander, K. E., Labrique, A. B., Landry, M. S., Leitner, C., Lubell-Doughtie, P. B., Marcelo, A. D., Matias, Y., Nelson, J., Nguyen, V., Nsengimana, J. P., Orton, M., Otzoy Garcia, D. R., Oyaole, D. R., Ratanaprayul, N., Roth, S., Schaefer, M. P., Settle, D., Tang, J., Tien-Wahser, B., Wanyee, S. & Hersch, F.
Oxf Open Digit Health, (2023).
Digital Public Goods Alliance - Promoting digital public goods to create a more equitable world (2023). 14-Dec-2023.
Google Open Source Blog | 17-May-2023
by Justin Krogue, Yun Liu, Po-Hsuan Cameron Chen & Ellery A
Nature Portfolio Health Community Blog | 10-May-2023
by Ellery Wulczyn and Yun Liu
Google Research Blog | 14-Mar-2023
Google Cloud Blog | 12-Dec-2022
Verily Blog | 16-Mar-2022
by Po-Hsuan Cameron Chen & Maggie Demkin
Google Research Blog | 11-Feb-2022
by Po-Hsuan Cameron Chen & Yun Liu
Google Keyword Blog | 23-Sept-2021
by People + AI Research
People + AI Research Blog | 14-May-2021
by Dave Steiner, Yun Liu, Craig Mermel, Kurt Zatloukal, Heimo Muller, Markus Plass
npj Digital Medicine Blog | 19-Apr-2021
Google Cloud Blog | 2-Sep-2020
by Kunal Nagpal & Craig Mermel
Google Keyword Blog | 23-Jul-2020
by Narayan Hedge & Carrie Cai
Google Research Blog | 19-July-2019
by Martin Stumpe & Craig Mermel
Google Research Blog | 16-Nov-2018
Google Research Blog | 12-Oct-2018
Google Research Blog | 16-Apr-2018
by Martin Stumpe & Lily Peng
Google Research Blog | 3-Mar-2017
McNeil, C., Wong, P. F., Sridhar, N., Wang, Y., Santori, C., Wu, C.-H., Homyk, A., Gutierrez, M., Behrooz, A., Tiniakos, D., Burt, A. D., Pai, R. K., Tekiela, K., Cameron Chen, P.-H., Fischer, L., Martins, E. B., Seyedkazemi, S., Freedman, D., Kim, C. C. & Cimermancic, P.
Mod. Pathol. 37, 100377 (2023).
Lai, J., Ahmed, F., Vijay, S., Jaroensri, T., Loo, J., Vyawahare, S., Agarwal, S., Jamil, F., Matias, Y., Corrado, G. S., Webster, D. R., Krause, J., Liu, Y., Chen, P.-H. C., Wulczyn, E. & Steiner, D. F.
Krogue, J. D., Azizi, S., Tan, F., Flament-Auvigne, I., Brown, T., Plass, M., Reihs, R., Müller, H., Zatloukal, K., Richeson, P., Corrado, G. S., Peng, L. H., Mermel, C. H., Liu, Y., Chen, P.-H. C., Gombar, S., Montine, T., Shen, J., Steiner, D. F. & Wulczyn, E.
Commun. Med. 3, 59 (2023).
L’Imperio, V., Wulczyn, E., Plass, M., Müller, H., Tamini, N., Gianotti, L., Zucchini, N., Reihs, R., Corrado, G. S., Webster, D. R., Peng, L. H., Chen, P.-H. C., Lavitrano, M., Liu, Y., Steiner, D. F., Zatloukal, K. & Pagni, F.
JAMA Netw Open 6, e2254891 (2023).
Jaroensri, R., Wulczyn, E., Hegde, N., Brown, T., Flament-Auvigne, I., Tan, F., Cai, Y., Nagpal, K., Rakha, E. A., Dabbs, D. J., Olson, N., Wren, J. H., Thompson, E. E., Seetao, E., Robinson, C., Miao, M., Beckers, F., Corrado, G. S., Peng, L. H., Mermel, C. H., Liu, Y., Steiner, D. F. & Chen, P.-H. C.
npj Breast Cancer 8, 1–12 (2022).
Bulten, W., Kartasalo, K., Chen, P.-H. C., Ström, P., Pinckaers, H., Nagpal, K., Cai, Y., Steiner, D. F., van Boven, H., Vink, R., Hulsbergen-van de Kaa, C., van der Laak, J., Amin, M. B., Evans, A. J., van der Kwast, T., Allan, R., Humphrey, P. A., Grönberg, H., Samaratunga, H., Delahunt, B., Tsuzuki, T., Häkkinen, T., Egevad, L., Demkin, M., Dane, S., Tan, F., Valkonen, M., Corrado, G. S., Peng, L., Mermel, C. H., Ruusuvuori, P., Litjens, G. & Eklund, M.
Nat. Med. 1–10 (2022).
Sadhwani, A., Chang, H.-W., Behrooz, A., Brown, T., Auvigne-Flament, I., Patel, H., Findlater, R., Velez, V., Tan, F., Tekiela, K., Wulczyn, E., Yi, E. S., Mermel, C. H., Hanks, D., Chen, P.-H. C., Kulig, K., Batenchuk, C., Steiner, D. F. & Cimermancic, P.
Sci. Rep. 11, 1–11 (2021).
Gamble, P., Jaroensri, R., Wang, H., Tan, F., Moran, M., Brown, T., Flament-Auvigne, I., Rakha, E. A., Toss, M., Dabbs, D. J., Regitnig, P., Olson, N., Wren, J. H., Robinson, C., Corrado, G. S., Peng, L. H., Liu, Y., Mermel, C. H., Steiner, D. F. & Chen, P.-H. C.
Communications Medicine 1, 1–12 (2021).
Wulczyn, E., Nagpal, K., Symonds, M., Moran, M., Plass, M., Reihs, R., Nader, F., Tan, F., Cai, Y., Brown, T., Flament-Auvigne, I., Amin, M. B., Stumpe, M. C., Müller, H., Regitnig, P., Holzinger, A., Corrado, G. S., Peng, L. H., Chen, P.-H. C., Steiner, D. F., Zatloukal, K., Liu, Y. & Mermel, C. H.
Communications Medicine 1, 1–8 (2021).
Cai, C.J., Steiner, D., Wilcox, L., Terry, M. and Winter, S.
Proceedings of the ACM SIGCHI Conference on Human Factors in Computing Systems, ACM (2021).
Wulczyn, E., Steiner, D. F., Moran, M., Plass, M., Reihs, R., Tan, F., Flament-Auvigne, I., Brown, T., Regitnig, P., Chen, P.-H. C., Hegde, N., Sadhwani, A., MacDonald, R., Ayalew, B., Corrado, G. S., Peng, L. H., Tse, D., Müller, H., Xu, Z., Liu, Y., Stumpe, M. C., Zatloukal, K. & Mermel, C. H.
npj Digital Medicine 4, 1–13 (2021).
Steiner, D. F., Nagpal, K., Sayres, R., Foote, D. J., Wedin, B. D., Pearce, A., Cai, C. J., Winter, S. R., Symonds, M., Yatziv, L., Kapishnikov, A., Brown, T., Flament-Auvigne, I., Tan, F., Stumpe, M. C., Jiang, P.-P., Liu, Y., Chen, P.-H. C., Corrado, G. S., Terry, M. & Mermel, C. H.
JAMA Netw Open 3, e2023267–e2023267 (2020).
Nagpal, K., Foote, D., Tan, F., Liu, Y., Chen, P.-H. C., Steiner, D. F., Manoj, N., Olson, N., Smith, J. L., Mohtashamian, A., Peterson, B., Amin, M. B., Evans, A. J., Sweet, J. W., Cheung, C., van der Kwast, T., Sangoi, A. R., Zhou, M., Allan, R., Humphrey, P. A., Hipp, J. D., Gadepalli, K., Corrado, G. S., Peng, L. H., Stumpe, M. C. & Mermel, C. H.
JAMA Oncol (2020).
Wulczyn, E., Steiner, D. F., Xu, Z., Sadhwani, A., Wang, H., Flament-Auvigne, I., Mermel, C. H., Chen, P.-H. C., Liu, Y. & Stumpe, M. C.
PLOS ONE 15, e0233678 (2020).
Kohlberger, T., Liu, Y., Moran, M., Chen, P.-H. C., Brown, T., Hipp, J. D., Mermel, C. H. & Stumpe, M. C.
J. Pathol. Inform. 10, 39 (2019).
Chen, P.C., Gadepalli, K., MacDonald, R., Liu, Y., Kadowaki, S., Nagpal, K., Kohlberger, T., Dean, J., Corrado, G.S., Hipp, J.D., Mermel, C.H., Stumpe, M. C.
Nat Med 25, 1453–1457 (2019). [readcube]
Liu, Y., Kohlberger, T., Norouzi, M., Dahl, G. E., Smith, J. L., Mohtashamian, A., Olson, N., Peng, L.H., Hipp, J.D., Stumpe, M.C. (2019).
Arch. Pathol. Lab. Med. 143, 859–868 (2019).
Hegde, N., Hipp, J. D., Liu, Y., Emmert-Buck, M., Reif, E., Smilkov, D., Terry, M., Cai, C. J., Amin, M. B., Mermel, C. H., Nelson, P. Q., Peng, L. H., Corrado, G. S. & Stumpe, M. C.
npj Digit Med 2, 56 (2019).
Cai, C.J., Winter, S., Steiner, D., Wilcox, L. and Terry, M.
Proceedings of the ACM on Human-computer Interaction, 3(CSCW), pp.1-24 (2019)
Cai, C.J., Reif, E., Hegde, N., Hipp, J., Kim, B., Smilkov, D., Wattenberg, M., Viegas, F., Corrado, G.S., Stumpe, M.C. and Terry, M.
In Proceedings of the 2019 CHI Conference on Human Factors in Computing Systems (pp. 1-14) (2019).
Nagpal, K., Foote, D., Liu, Y., Chen, P.H.C., Wulczyn, E., Tan, F., Olson, N., Smith, J.L., Mohtashamian, A., Wren, J.H., Corrado, G.S., MacDonald, R., Peng, L. H., Amin, M.B., Evans, A.J., Sanjoi, A.R., Mermel, C. H., Hipp, J. D., Stumpe, M. C.
npj Digit. Med. 2, 48 (2019).
Steiner, D. F., MacDonald, R., Liu, Y., Truszkowski, P., Hipp, J. D., Gammage, C., Thng, F., Peng, L., Stumpe, M.C.
Am. J. Surg. Pathol. 42, 1636–1646 (2018).
Liu, Y., Gadepalli, K., Norouzi, M., Dahl, G.E., Kohlberger, T., Boyko, A., Venugopalan, S., Timofeev, A., Nelson, P.Q., Corrado, G.S. and Hipp, J.D., Peng, L., Stumpe, M. C.
arXiv preprint arXiv:1703.02442 (2017).
Public & Environmental Health
by Kristina Gligoric
Nature Portfolio Health Community Blog | 22-Nov-2023
Google Keyword | 10-Oct-2023
by Charlotte Stanton
Google Africa Blog | 9-May-2023
by Kate Brandt
Google Keyword Blog | 29-Mar-2023
by Adam Sadilek & Xerxes Dotiwalla
Google Research Blog | 12-Nov-2019
Wong, K. L. M., Banke-Thomas, A., Olubodun, T., Macharia, P. M., Stanton, C., Sundararajan, N., Shah, Y., Prasad, G., Kansal, M., Vispute, S., Shekel, T., Ogunyemi, O., Gwacham-Anisiobi, U., Wang, J., Abejirinde, I.-O. O., Makanga, P. T., Afolabi, B. B. & Beňová, L.
Commun. Med. 4, 34 (2024).
Gligorić, K., Kamath, C., Weiss, D. J., Bavadekar, S., Liu, Y., Shekel, T., Schulman, K. & Gabrilovich, E.
Communications Medicine 3, 1–11 (2023).
Macharia, P. M., Wong, K. L. M., Olubodun, T., Beňová, L., Stanton, C., Sundararajan, N., Shah, Y., Prasad, G., Kansal, M., Vispute, S., Shekel, T., Gwacham-Anisiobi, U., Ogunyemi, O., Wang, J., Abejirinde, I.-O. O., Makanga, P. T., Afolabi, B. B. & Banke-Thomas, A.
Sci Data 10, 736 (2023).
Veneri, P., Kaufmann, T., Vispute, S., Shekel, T., Gabrilovich, E., Wellenius, G. A., Dijkstra, L. & Kansal, M.
(Organisation for Economic Co-Operation and Development (OECD), 2023).
Weintraub, R. L., Miller, K., Rader, B., Rosenberg, J., Srinath, S., Woodbury, S. R., Schultheiss, M. D., Kansal, M., Vispute, S., Serghiou, S., Flores, G., Kumok, A., Shekel, T., Gabrilovich, E., Ahmad, I., Chiang, M. E. & Brownstein, J. S.
Am. J. Public Health e1–e5 (2023).
Gupta, J., Tay, Y., Kamath, C., Tran, V., Metzler, D., Bavadekar, S., Sun, M. & Gabrilovich, E.
Proceedings of the 2022 Conference on Empirical Methods in Natural Language Processing. 521–530 (2022).
Vaidyanathan, U., Sun, Y., Shekel, T., Chou, K., Galea, S., Gabrilovich, E. & Wellenius, G. A.
Sci. Rep. 12, 8946 (2022). [readcube]
Wahltinez, O., Cheung, A., Alcantara, R., Cheung, D., Daswani, M., Erlinger, A., Lee, M., Yawalkar, P., Lê, P., Navarro, O. P., Brenner, M. P. & Murphy, K.
Sci Data 9, 162 (2022).
Malahy, S., Sun, M., Spangler, K., Leibler, J., Lane, K., Bavadekar, S., Kamath, C., Kumok, A., Sun, Y., Gupta, J., Griffith, T., Boulanger, A., Young, M., Stanton, C., Mayer, Y., Smith, K., Shekel, T., Chou, K., Corrado, G., Levy, J., Szpiro, A., Gabrilovich, E. & Wellenius, G. A.
arXiv [cs.SI] (2021).
Bavadekar, S., Boulanger, A., Davis, J., Desfontaines, D., Gabrilovich, E., Gadepalli, K., Ghazi, B., Griffith, T., Gupta, J., Kamath, C., Kraft, D., Kumar, R., Kumok, A., Mayer, Y., Manurangsi, P., Patankar, A., Perera, I. M., Scott, C., Shekel, T., Miller, B., Smith, K., Stanton, C., Sun, M., Young, M. & Wellenius, G.
arXiv [cs.CR] (2021).
Woskie, L. R., Hennessy, J., Espinosa, V., Tsai, T. C., Vispute, S., Jacobson, B. H., Cattuto, C., Gauvin, L., Tizzoni, M., Fabrikant, A., Gadepalli, K., Boulanger, A., Pearce, A., Kamath, C., Schlosberg, A., Stanton, C., Bavadekar, S., Abueg, M., Hogue, M., Oplinger, A., Chou, K., Corrado, G., Shekel, T., Jha, A. K., Wellenius, G. A. & Gabrilovich, E.
PLoS One 16, e0253071 (2021).
Wellenius, G. A., Vispute, S., Espinosa, V., Fabrikant, A., Tsai, T. C., Hennessy, J., Dai, A., Williams, B., Gadepalli, K., Boulanger, A., Pearce, A., Kamath, C., Schlosberg, A., Bendebury, C., Mandayam, C., Stanton, C., Bavadekar, S., Pluntke, C., Desfontaines, D., Jacobson, B. H., Armstrong, Z., Gipson, B., Wilson, R., Widdowson, A., Chou, K., Oplinger, A., Shekel, T., Jha, A. K. & Gabrilovich, E.
Nat. Commun. 12, 3118 (2021).
Venkatramanan, S., Sadilek, A., Fadikar, A., Barrett, C. L., Biggerstaff, M., Chen, J., Dotiwalla, X., Eastham, P., Gipson, B., Higdon, D., Kucuktunc, O., Lieber, A., Lewis, B. L., Reynolds, Z., Vullikanti, A. K., Wang, L. & Marathe, M.
Nat. Commun. 12, 726 (2021).
Weiss, D. J., Nelson, A., Vargas-Ruiz, C. A., Gligorić, K., Bavadekar, S., Gabrilovich, E., Bertozzi-Villa, A., Rozier, J., Gibson, H. S., Shekel, T., Kamath, C., Lieber, A., Schulman, K., Shao, Y., Qarkaxhija, V., Nandi, A. K., Keddie, S. H., Rumisha, S., Amratia, P., Arambepola, R., Chestnutt, E. G., Millar, J. J., Symons, T. L., Cameron, E., Battle, K. E., Bhatt, S. & Gething, P. W.
Nat. Med. (2020).
Abueg, M., Hinch, R., Wu, N., Liu, L., Probert, W. J. M., Wu, A., Eastham, P., Shafi, Y., Rosencrantz, M., Dikovsky, M., Cheng, Z., Nurtay, A., Abeler-Dörner, L., Bonsall, D. G., McConnell, M. V., O’Banion, S. & Fraser, C.
medRxiv (2020). doi:10.1101/2020.08.29.20184135
Bavadekar, S., Dai, A., Davis, J., Desfontaines, D., Eckstein, I., Everett, K., Fabrikant, A., Flores, G., Gabrilovich, E., Gadepalli, K., Glass, S., Huang, R., Kamath, C., Kraft, D., Kumok, A., Marfatia, H., Mayer, Y., Miller, B., Pearce, A., Perera, I. M., Ramachandran, V., Raman, K., Roessler, T., Shafran, I., Shekel, T., Stanton, C., Stimes, J., Sun, M., Wellenius, G. & Zoghi, M.
arXiv [cs.CR] (2020).
Wellenius, G. A., Vispute, S., Espinosa, V., Fabrikant, A., Tsai, T. C., Hennessy, J., Williams, B., Gadepalli, K., Boulanger, A., Pearce, A., Kamath, C., Schlosberg, A., Bendebury, C., Stanton, C., Bavadekar, S., Pluntke, C., Desfontaines, D., Jacobson, B., Armstrong, Z., Gipson, B., Wilson, R., Widdowson, A., Chou, K., Oplinger, A., Shekel, T., Jha, A. K. & Gabrilovich, E.
arXiv [q-bio.PE] (2020).
Aktay, A., Bavadekar, S., Cossoul, G., Davis, J., Desfontaines, D., Fabrikant, A., Gabrilovich, E., Gadepalli, K., Gipson, B., Guevara, M., Kamath, C., Kansal, M., Lange, A., Mandayam, C., Oplinger, A., Pluntke, C., Roessler, T., Schlosberg, A., Shekel, T., Vispute, S., Vu, M., Wellenius, G., Williams, B. & Wilson, R. J.
Ruktanonchai, N. W., Floyd, J. R., Lai, S., Ruktanonchai, C. W., Sadilek, A., Rente-Lourenco, P., Ben, X., Carioli, A., Gwinn, J., Steele, J. E., Prosper, O., Schneider, A., Oplinger, A., Eastham, P. & Tatem, A. J.
Science 369, 1465–1470 (2020).
Sadilek, A., Hswen, Y., Bavadekar, S., Shekel, T., Brownstein, J. S. & Gabrilovich, E.
npj Digital Medicine 3, 1–12 (2020).
Bassolas, A., Barbosa-Filho, H., Dickinson, B., Dotiwalla, X., Eastham, P., Gallotti, R., Ghoshal, G., Gipson, B., Hazarie, S. A., Kautz, H., Kucuktunc, O., Lieber, A., Sadilek, A., & Ramasco, J. J.
Nat. Commun. 10, 4817 (2019).
Sadilek, A., Caty, S., DiPrete, L., Mansour, R., Schenk Jr., T., Bergtholdt, M., Jha, A., Ramaswami P., & Gabrilovich E.
npj Digital Med 1, 36 (2018).
by Atilla Kiraly & Rory Pilgrim
Google Research Blog | 20-Mar-2024
by Shravya Shetty
by Yossi Matias & Shravya Shetty
Google Africa Blog | 31-Oct-2023
by Angelica Willis & Akib Uddin
TensorFlow Blog | 20-Jun-2023
by Perry Nelson & Aisha Walcott-Bryant
Google Africa Blog | 1-Jun-2023
Google Keyword Blog | 28-Nov-2022
by Nicole Linton
Google Keyword Blog | 21-Oct-2022
by Akib Uddin & Andrew Sellergren
Google Research Blog | 19-Jul-2022
Google Japan Blog | 25-Nov-2021
by Zaid Nabulsi & Po-Hsuan Cameron Chen
Google Research Blog | 1-Sep-2021
by Rory Pilgrim & Shruthi Prabhakara
by Sunny Jansen & Krish Eswaran
Google Keyword Blog | 25-Feb-2021
by Cian Hughes
Google Keyword Blog | 29-Oct-2020
by Shravya Shetty & Daniel Tse
Google Keyword Blog | 1-Jan-2020
by Dave Steiner & Shravya Shetty
Google Research Blog | 3-Dec-2019
Google Keyword Blog | 20-May-2019
Kiraly, A. P., Cunningham, C. A., Najafi, R., Nabulsi, Z., Yang, J., Lau, C., Ledsam, J. R., Ye, W., Ardila, D., McKinney, S. M., Pilgrim, R., Liu, Y., Saito, H., Shimamura, Y., Etemadi, M., Melnick, D., Jansen, S., Corrado, G. S., Peng, L., Tse, D., Shetty, S., Prabhakara, S., Naidich, D. P., Beladia, N. & Eswaran, K.
Radiol Artif Intell e230079 (2024).
Lucido, J. J., DeWees, T. A., Leavitt, T. R., Anand, A., Beltran, C. J., Brooke, M. D., Buroker, J. R., Foote, R. L., Foss, O. R., Gleason, A. M., Hodge, T. L., Hughes, C. O., Hunzeker, A. E., Laack, N. N., Lenz, T. K., Livne, M., Morigami, M., Moseley, D. J., Undahl, L. M., Patel, Y., Tryggestad, E. J., Walker, M. Z., Zverovitch, A. & Patel, S. H.
Front. Oncol. 13, (2023).
Lee, C., Willis, A., Chen, C., Sieniek, M., Watters, A., Stetson, B., Uddin, A., Wong, J., Pilgrim, R., Chou, K., Tse, D., Shetty, S. & Gomes, R. G.
JAMA Netw Open 6, e2248685 (2023).
Gomes, R. G., Vwalika, B., Lee, C., Willis, A., Sieniek, M., Price, J. T., Chen, C., Kasaro, M. P., Taylor, J. A., Stringer, E. M., McKinney, S. M., Sindano, N., Dahl, G. E., Goodnight, W., Gilmer, J., Chi, B. H., Lau, C., Spitz, T., Saensuksopa, T., Liu, K., Tiyasirichokchai, T., Wong, J., Pilgrim, R., Uddin, A., Corrado, G., Peng, L., Chou, K., Tse, D., Stringer, J. S. A. & Shetty, S.
Communications Medicine 2, 1–9 (2022).
Kazemzadeh, S., Yu, J., Jamshy, S., Pilgrim, R., Nabulsi, Z., Chen, C., Beladia, N., Lau, C., McKinney, S. M., Hughes, T., Kiraly, A. P., Kalidindi, S. R., Muyoyeta, M., Malemela, J., Shih, T., Corrado, G. S., Peng, L., Chou, K., Chen, P.-H. C., Liu, Y., Eswaran, K., Tse, D., Shetty, S. & Prabhakara, S.
Radiology 212213 (2022).
Sellergren, A. B., Chen, C., Nabulsi, Z., Li, Y., Maschinot, A., Sarna, A., Huang, J., Lau, C., Kalidindi, S. R., Etemadi, M., Garcia-Vicente, F., Melnick, D., Liu, Y., Eswaran, K., Tse, D., Beladia, N., Krishnan, D. & Shetty, S.
Radiology 212482 (2022).
Anand, A., Beltran, C. J., Brooke, M. D., Buroker, J. R., DeWees, T. A., Foote, R. L., Foss, O. R., Hughes, C. O., Hunzeker, A. E., John Lucido, J., Morigami, M., Moseley, D. J., Pafundi, D. H., Patel, S. H., Patel, Y., Ridgway, A. K., Tryggestad, E. J., Wilson, M. Z., Xi, L. & Zverovitch, A.
medRxiv 2021.12.07.21266421 (2021).
Nabulsi, Z., Sellergren, A., Jamshy, S., Lau, C., Santos, E., Kiraly, A. P., Ye, W., Yang, J., Pilgrim, R., Kazemzadeh, S., Yu, J., Kalidindi, S. R., Etemadi, M., Garcia-Vicente, F., Melnick, D., Corrado, G. S., Peng, L., Eswaran, K., Tse, D., Beladia, N., Liu, Y., Chen, P.-H. C. & Shetty, S.
Sci. Rep. 11, 1–15 (2021).
Nikolov, S., Blackwell, S., Zverovitch, A., Mendes, R., Livne, M., De Fauw, J., Patel, Y., Meyer, C., Askham, H., Romera-Paredes, B., Kelly, C., Karthikesalingam, A., Chu, C., Carnell, D., Boon, C., D’Souza, D., Moinuddin, S. A., Garie, B., McQuinlan, Y., Ireland, S., Hampton, K., Fuller, K., Montgomery, H., Rees, G., Suleyman, M., Back, T., Hughes, C. O., Ledsam, J. R. & Ronneberger, O.
J. Med. Internet Res. 23, e26151 (2021).
Duggan, G. E., Reicher, J. J., Liu, Y., Tse, D. & Shetty, S.
Br J Radiol. 94, 20210435 (2021).
McKinney, S. M., Sieniek, M., Godbole, V., Godwin, J., Antropova, N., Ashrafian, H., Back, T., Chesus, M., Corrado, G. S., Darzi, A., Etemadi, M., Garcia-Vicente, F., Gilbert, F. J., Halling-Brown, M., Hassabis, D., Jansen, S., Karthikesalingam, A., Kelly, C. J., King, D., Ledsam, J. R., Melnick, D., Mostofi, H., Peng, L., Reicher, J. J., Romera-Paredes, B., Sidebottom, R., Suleyman, M., Tse, D., Young, K. C., De Fauw, J. & Shetty, S.
Nature 577, 89–94 (2020). [readcube]
Majkowska, A., Mittal, S., Steiner, D. F., Reicher, J. J., McKinney, S. M., Duggan, G. E., Eswaran, K., Cameron Chen, P.-H., Liu, Y., Kalidindi, S. R., Ding, A., Corrado, G. S., Tse, D. & Shetty, S.
Radiology 191293 (2019).
Ardila, D., Kiraly, A. P., Bharadwaj, S., Choi, B., Reciher, J. J., Peng, L., Tse, D., Etemadi, M., Ye, W., Corrado, G., Naidich, D. P., Shetty, S.
Nat. Med. 25, 954–961 (2019). [readcube]
Blog Posts [more at Youtube Official Blog ]
by The YouTube Team
Youtube Official Blog | 6-Feb-2024
by Garth Graham
Youtube Official Blog | 10-Jan-2024
Youtube Official Blog | 14-Dec-2023
by James Beser
Youtube Official Blog | 2-Nov-2023
Youtube Official Blog | 7-Sep-2023
Youtube Official Blog
15-Aug-2023
12-Jun-2023
15-May-2023
Youtube Official Blog | 18-Apr-2023
by Jessica DiVento Dzuban
Youtube Official Blog | 7-Oct-2022
Youtube Official Blog | 1-Mar-2023
Youtube Official Blog | 27-Oct-2022
Youtube Official Blog | 28–Sep-2022
Youtube Official Blog | 12–Sep-2022
Youtube Official Blog | 15-Jun-2022
Youtube Official Blog | 24-Mar-2022
Youtube Official Blog | 26-Jan-2022
Youtube Official Blog | 13-Oct-2021
Youtube Official Blog | 19-Jul-2021
Thank you for visiting nature.com. You are using a browser version with limited support for CSS. To obtain the best experience, we recommend you use a more up to date browser (or turn off compatibility mode in Internet Explorer). In the meantime, to ensure continued support, we are displaying the site without styles and JavaScript.
- View all journals
Medical research articles from across Nature Portfolio
Medical research involves research in a wide range of fields, such as biology, chemistry, pharmacology and toxicology with the goal of developing new medicines or medical procedures or improving the application of those already available. It can be viewed as encompassing preclinical research (for example, in cellular systems and animal models) and clinical research (for example, clinical trials).
The MARIPOSA trials — implications for the treatment of EGFR -mutant NSCLC
In the past 2 years, substantial improvements have been made in the management of advanced-stage EGFR -mutant non-small-cell lung cancer. Recent studies have suggested added benefit from the combination of third-generation tyrosine-kinase inhibitors with either chemotherapy or a bispecific antibody targeting EGFR and MET. Herein, we summarize these advances and their implications for clinical practice.
- Fatemeh Ardeshir-Larijani
- Suresh S. Ramalingam
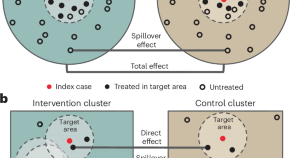
Spillover effects of targeted malaria interventions benefit neighboring areas
In a setting of low malaria transmission, preventive interventions that target human and mosquito parasite reservoirs located near malaria cases reduced malaria among non-recipients up to 3 km away. Accounting for these ‘spillover effects’ reveals a higher population-level health benefit, and increased cost-effectiveness compared with previous analyses.

The formation and maintenance of peri-implant fibrosis requires skeletal cells expressing the leptin receptor
The cellular composition of fibrous tissue around orthopaedic implants and the genetic pathways involved in its formation are unclear. We find that leptin-receptor-expressing cells activate the adhesion G-protein-coupled receptor F5 (ADGRF5) pathway to form peri-implant fibrous tissue. Inhibition of ADGRF5 in leptin-receptor-expressing cells can prevent and reverse peri-implant fibrosis.
Related Subjects
- Drug development
- Epidemiology
- Experimental models of disease
- Genetics research
- Outcomes research
- Paediatric research
- Preclinical research
- Stem-cell research
- Clinical trial design
- Translational research
Latest Research and Reviews
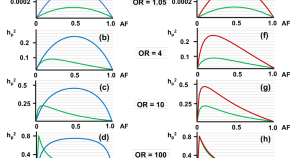
Contribution of rare variants to heritability of a disease is much greater than conventionally estimated: modification of allele distribution model
- Yoshiro Nagao
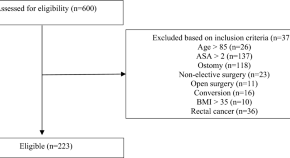
Morbidity after accelerated enhanced recovery protocol for colon cancer surgery
- Misha A. T. Sier
- Sarah L. Dekkers
- Jan H. M. B. Stoot
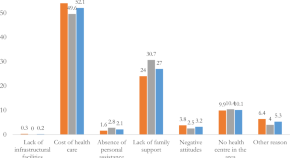
Healthcare services access challenges and determinants among persons with disabilities in Bangladesh
- Mizanur Rahman
- Md Shohel Rana
- Md Nuruzzaman Khan
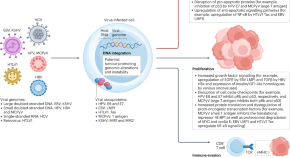
Applications of cell therapy in the treatment of virus-associated cancers
Several different viruses have a role in cancer pathogenesis, contributing to the development of various haematological malignancies and solid tumours via diverse, multifaceted mechanisms. However, this viral aetiology presents a unique opportunity for adoptive virus-specific T cell (VST) therapy. This Review summarizes the mechanisms of viral carcinogenesis and describes the current clinical experience with adoptive cellular immunotherapies for virus-related cancers, predominantly using non-genetically modified VSTs. The authors also discuss challenges and future directions for the ongoing clinical development of VST therapies.
- Chase D. McCann
- Catherine M. Bollard
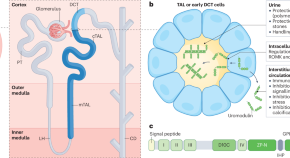
Advances in uromodulin biology and potential clinical applications
In this Review, the authors examine advances in uromodulin biology, including the existence of non-polymeric forms of the protein, its versatile functions, crosstalk with the immune system, its potential as a biomarker and its role in kidney disease, as well as considering how uromodulin might be targeted therapeutically.
- Azuma Nanamatsu
- Larissa de Araújo
- Tarek M. El-Achkar
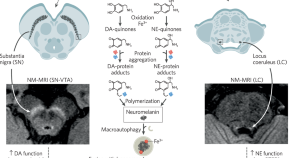
Neuromelanin-sensitive MRI for mechanistic research and biomarker development in psychiatry
- Kenneth Wengler
- Paula Trujillo
- Guillermo Horga
News and Comment
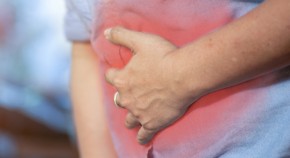
IL-23 inhibitors go head to head in Crohn’s disease
Risankizumab outperformed ustekinumab for the treatment of moderate to severe Crohn’s disease in patients refractory to anti-TNF therapy.
- Karen O’Leary
Inulin supplementation in pediatric obesity management: a critical appraisal of efficacy and limitations
- Yanggang Hong
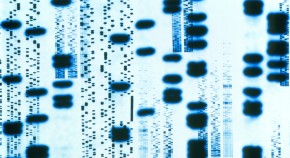
Child with ultra-rare disease gets a treatment just for her
Therapy designed for one seems to have improved a young girl’s quality of life.
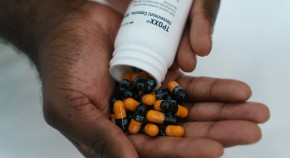
Hopes dashed for drug aimed at monkeypox virus spreading in Africa
Early results from clinical trial show that the antiviral drug tecovirimat is no better than placebo against the clade I virus type.
- Mariana Lenharo
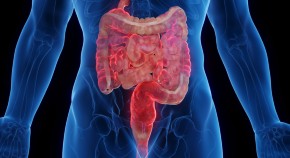
The mysteries of inflammatory bowel disease are being cracked — offering hope for new therapies
Advances in understanding the causes of the autoimmune disorder could aid in matching people with the right treatment.
- Heidi Ledford
Quick links
- Explore articles by subject
- Guide to authors
- Editorial policies

Reference management. Clean and simple.
The top list of research databases for medicine and healthcare
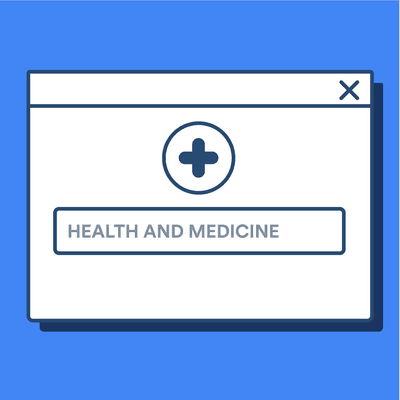
3. Cochrane Library
4. pubmed central (pmc), 5. uptodate, frequently asked questions about research databases for medicine and healthcare, related articles.
Web of Science and Scopus are interdisciplinary research databases and have a broad scope. For biomedical research, medicine, and healthcare there are a couple of outstanding academic databases that provide true value in your daily research.
Scholarly databases can help you find scientific articles, research papers , conference proceedings, reviews and much more. We have compiled a list of the top 5 research databases with a special focus on healthcare and medicine.
PubMed is the number one source for medical and healthcare research. It is hosted by the National Institutes of Health (NIH) and provides bibliographic information including abstracts and links to the full text publisher websites for more than 28 million articles.
- Coverage: around 35 million items
- Abstracts: ✔
- Related articles: ✔
- References: ✘
- Cited by: ✘
- Links to full text: ✔
- Export formats: XML, NBIB
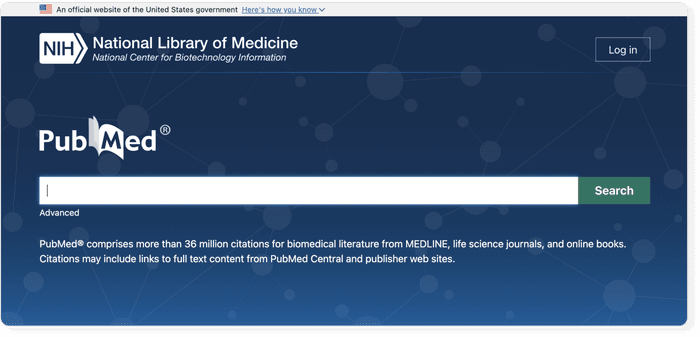
Pro tip: Use a reference manager like Paperpile to keep track of all your sources. Paperpile integrates with PubMed and many popular databases. You can save references and PDFs directly to your library using the Paperpile buttons and later cite them in thousands of citation styles:
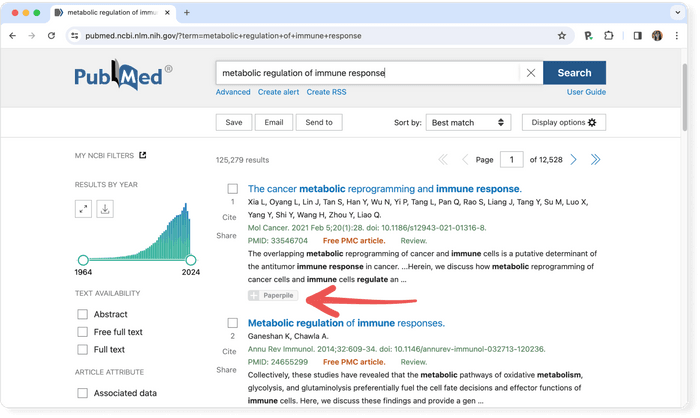
EMBASE (Excerpta Medica Database) is a proprietary research database that also includes PubMed. It can also be accessed by other database providers such as Ovid .
- Coverage: 38 million articles
- References: ✔
- Cited by: ✔
- Full text: ✔ (requires institutional subscription to EMBASE and individual publishers)
- Export formats: RIS
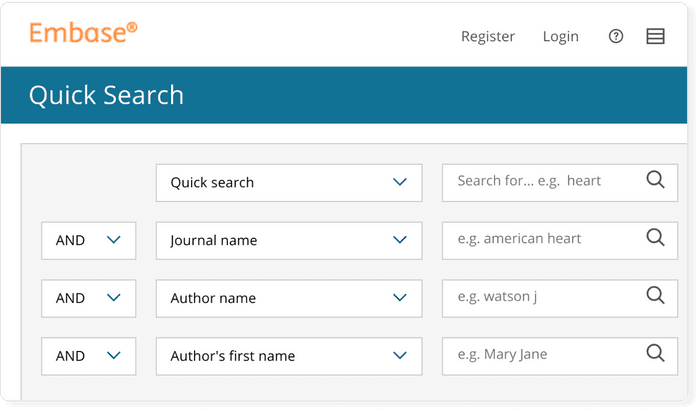
The Cochrane Library is best know for its systematic reviews. There are 53 review groups around the world that ensure that the published reviews are of high-quality and evidence based. Articles are updated over time to reflect new research.
- Coverage: several thousand high quality reviews
- Full text: ✔
- Export formats: RIS, BibTeX
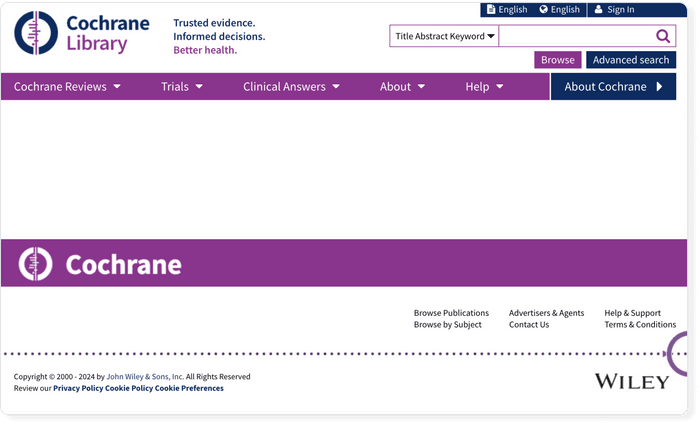
PubMed Central is the free, open access branch of PubMed. It includes full-text versions for all indexed papers. You might also want to check out its sister site Europe PMC .
- Coverage: more than 8 million articles
- Export formats: APA, MLA, AMA, RIS, NBIB
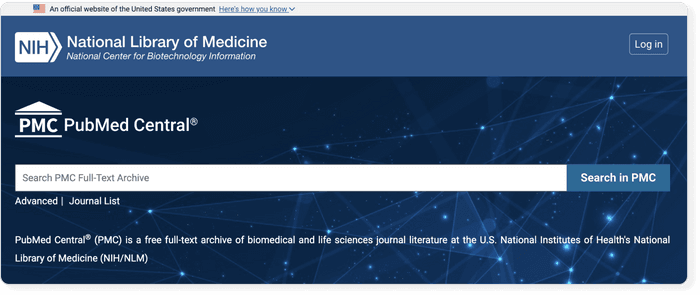
Like the Cochrane Library, UpToDate provides detailed reviews for clinical topics. Reviews are constantly updated to provide an up-to-date view.
- Coverage: several thousand articles from over 420 peer-reviewed journals
- Related articles: ✘
- Full text: ✔ (requires institutional subscription)
- Export formats: ✘
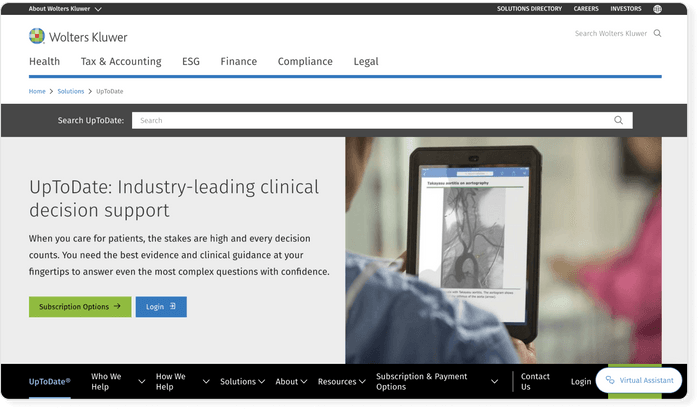
PubMed is the number one source for medical and healthcare research. It is hosted at the National Institutes of Health (NIH) and provides bibliographic information including abstracts and links to the full text publisher websites for more than 35 million items.
EMBASE (Excerpta Medica Database) is a proprietary research database that also includes in its corpus PubMed. It can also be accessed by other database providers such as Ovid.
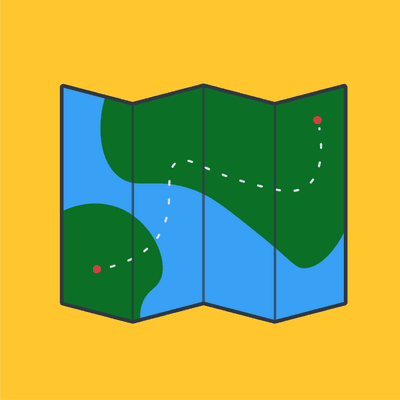

An official website of the United States government
The .gov means it’s official. Federal government websites often end in .gov or .mil. Before sharing sensitive information, make sure you’re on a federal government site.
The site is secure. The https:// ensures that you are connecting to the official website and that any information you provide is encrypted and transmitted securely.
- Publications
- Account settings
Preview improvements coming to the PMC website in October 2024. Learn More or Try it out now .
- Advanced Search
- Journal List
- Wiley Open Access Collection

A practice‐based model to guide nursing science and improve the health and well‐being of patients and caregivers
Sherry s. chesak.
1 Nursing Research Division, Mayo Clinic, Rochester MN, USA
Lori M. Rhudy
Cindy tofthagen.
2 Nursing Research Division, Mayo Clinic, Jacksonville FL, USA
Linda L. Chlan
Associated data.
Data sharing not applicable to this article as no datasets were generated or analyzed during the current study.
Aims and Objectives
The purpose of this paper is to describe a model to guide nursing science in a clinical practice‐based setting. Exemplars are provided to highlight the application of this nursing research model, which can be applied to other clinical settings that aim to fill evidence gaps in the literature.
Nurse scientists are well positioned to develop new knowledge aimed at identifying global health solutions to multiple disparities. The generation and application of this knowledge are essential to inform and guide professional nursing practice. While a number of evidence‐based practice models exist to guide the integration of literature findings and other sources of evidence into practice, there is a need for additional models that serve as a guide and focus for the conduct of research in distinct scientific areas in practice‐based settings.
Model development and description.
Mayo Clinic is a large, comprehensive healthcare system with a mission to address unmet patient needs through practice, research and education. PhD‐prepared nurse scientists engage in practice‐based research as an integral component of Mayo Clinic's mission. A practice‐based nursing research model was developed with the intent to advance nursing research in a clinical setting.
The components of the Mayo Clinic Nursing Research model include symptom science, self‐management science and caregiving science. The generation of nursing science is focused on addressing needs of patients with complex health conditions, inclusive of caregivers.
Conclusions
While clinical settings provide rich opportunities for the conduct of research, priorities need to be established in which to focus scientific endeavours. The Mayo Clinic Nursing Research model may be applicable to nurses around the globe who are engaged in the generation of knowledge to guide practice.
Relevance to Clinical Practice
The Mayo Clinic Nursing Research model can be used by nurse scientists embedded in healthcare settings to address clinically relevant questions, advance the generation of new nursing knowledge and ultimately improve the health and well‐being of patients and caregivers.
What does this paper contribute to the wider global clinical community?
- There is a need for additional models to guide the conduct of nursing research in clinical settings.
- The Mayo Clinic Nursing Research Model was developed as a model to guide the generation of new nursing knowledge in a clinical, practice‐based setting.
- The model can be used in a variety of clinical settings for researchers who aim to fill evidence gaps in the literature.
1. INTRODUCTION
Nursing is the largest profession in health care, with continued growth expected over the next several years (Grady & Hinshaw, 2017 ). Nursing science plays a critical role in addressing health challenges, generating new knowledge and translating evidence to practice to improve patient outcomes (Grady, 2017 ; Powell, 2015 ). Furthermore, nursing science integrates biobehavioural approaches to better understand patients' needs and preferences, develop individualised symptom management interventions (Trego, 2017 ), advance interventions to promote self‐management of chronic conditions and thus promote well‐being and quality of life (Grady, 2017 ; Powell, 2015 ). Patients' healthcare needs are becoming increasingly more complex, giving rise to the need for practice‐based research. The clinical practice setting provides an opportunity to conduct research, by which patients' and caregivers needs and outcomes may be addressed and improved.
The purpose of this paper is to present the Mayo Clinic Nursing Research (MCNR) model (Figure (Figure1)—a 1 )—a model developed to guide and focus nursing science generation in a practice‐based setting with an emphasis on promoting the health and well‐being of patients and caregivers with complex needs. The components of the model are described, and exemplars of the generation of practice‐based nursing knowledge are presented.

Mayo clinic nursing research model [Color figure can be viewed at wileyonlinelibrary.com ]
2. BACKGROUND
Over a century ago, Florence Nightingale recognised not only the need for formal training for nurses but also the power of the nurse to improve patient outcomes (Nightingale, 1992 ). This is still true in today's healthcare environment. Nurses can help fill a critical need not only for the education and training of healthcare workers, but also for the design and testing of solutions to common health problems (National Institutes of Health, 2015 ). As noted by Dr. Patricia Grady, director emeritus of the National Institute of Nursing Research (NINR), ‘…nurse scientists can use their expertise in clinical research and their understanding of the relationship between behaviour and biology to further expand the reach and impact of nursing science in the larger community’ (National Institute of Nursing Research, 2016 , p. 6). However, recommended models for the structure and organisation of nursing research in clinical settings are scarce.
PhD‐prepared nurse scientists (sometimes referred to as nurse researchers) design and implement research studies to improve health‐related outcomes. Although most nurse scientists are employed in academic settings such as schools/colleges of nursing, there is an emerging trend for nurse scientists to have full‐time appointments in practice settings (Robichaud‐Ekstrand, 2016 ). The nurse scientist role has wide variability in how it is operationalised but can be described in three ways. First, in academic settings, Boyer's model of scholarship includes discovery, integration, application and teaching to frame the discussion of discovery and practice in nursing (Boyer, 1990 ; Hickey et al., 2019 ). Academic service partnerships have emerged as strategies to close the academic‐practice gap by connecting clinical practice with academia in order to meet mutually beneficial goals (Sadeghnezhad et al., 2018 ). Examples of programmes in academic‐service partnerships include preparation of new graduate nurses, patient safety initiatives, transitions‐in‐care programmes, advancement of evidence‐based nursing and opportunities for clinical research (Sadeghnezhad et al., 2018 ). While such programmes inform the advancement of nursing research as a component of evidence‐based practice in clinical settings, they are less informative in guiding the generation of knowledge among nurse scientists embedded in clinical settings.
In a second approach, a nurse scientist supports evidence‐based practice, quality improvement, the conduct of research by clinical nurses and, if applicable, ANCC Magnet Recognition Program® activities (Kowalski, 2020 ). A third approach similarly involves embedding nurse scientists in clinical practice settings but the role is focused on the conduct and facilitation of nursing‑oriented research, rather than simply providing support for research conducted by others (Chan et al., 2010 ). This third approach is used in the setting in which this model was developed.
Evidence‐based practice models such as the Iowa Model and the Johns Hopkins Nursing Evidence‐Based Practice Model have been adopted to guide translation of evidence to practice but they have limited utility in describing the infrastructure, focus and outcomes of nursing research in a clinical setting. The Iowa Model Revised: Evidence‐Based Practice to Promote Excellence in Health Care uses an algorithm to guide evidence‐based practice processes from identification of a trigger to integrating and sustaining a practice change (Buckwalter et al., 2017 ). The conduct of research is included in the Iowa Model as a strategy to be used when insufficient evidence exists to recommend a practice change. The Johns Hopkins Nursing Evidence‐Based Practice Model (Dang & Dearholt, 2018 ) includes a patient‐centred approach and incorporates a continuum of Inquiry–Practice/Learning–Practice Improvement as a method to ensure that best practices are applied to patient care. However, the model is centred on an evidence‐based practice approach, which differs from research in that research involves systematic investigation of phenomena to discover new information or reach new understandings and conclusions to generate new knowledge (Cohen et al., 2015 ; Hickey et al., 2019 ). The Joanna Briggs Institute (JBI) (Joanna Briggs Institute, 2016 ), based in the Faculty of Health and Medical Sciences at the University of Adelaide, South Australia, aims to promote evidence‐based decision‐making by promoting the use of the best available evidence. JBI, through its JBI Collaboration, works with universities and hospitals around the world to synthesise, transfer and implement evidence that is culturally relevant and applicable across diverse healthcare settings internationally.
The NINR sets strategic funding and training priorities that advance nursing science to enhance the health and well‐being of individuals across diverse populations (National Institute of Nursing Research, 2016 ). Current research priorities established by the NINR include four scientific foci: symptom science, wellness, self‐management of chronic conditions, and end‐of‐life and palliative care (National Institute of Nursing Research, 2016 ). In addition, all areas of NINR's research programmes place an emphasis on promoting innovation and developing the nurse scientists of the 21st century (National Institute of Nursing Research, 2016 ). Recognising that symptoms are the primary reason patients seek care, the NINR developed the symptom science model to advance research. The symptom science model describes an analytical sequence beginning with a sequelae or cluster of symptoms, which are then characterised into a phenotype with biological correlates, followed by the application of research methods that can be used to identify targets for therapeutic and clinical interventions (Cashion & Grady, 2015 ).
Nurse scientists are well positioned to develop new knowledge aimed at identifying global health solutions to social, economic, psychological and biological disparities. The generation and application of this knowledge are essential to provide the best available evidence to inform and guide professional nursing practice. While a number of evidence‐based practice models exist to guide the integration of literature findings and other sources of evidence into practice, there is a need for additional models that serve as a guide and focus for the conduct of research in distinct scientific areas in practice‐based settings. Therefore, the project team identified a need for the development of a model articulating the goals and strategies to advance nursing research within their institution, and which would have broad applicability to other institutions and nurse scientists embedded in the clinical practice.
Mayo Clinic is a large academic medical centre that incorporates practice, education and research into its mission, which has been emulated in the Department of Nursing and the Division of Nursing Research for over three decades. Today, the Mayo Clinic Nursing Research Division is an enterprise‐wide unit providing infrastructure and support for nursing research at its sites in Mayo Clinic. A cadre of PhD‐prepared nurse scientists lead independent programmes of research and provide consultation to all staff in research‐related matters, including scientific review of research protocols. In addition, small cadres of registered nurses providing direct patient care conduct research studies under the mentorship of a nurse scientist. These clinical nurse scholars identify clinically relevant questions that are investigated by an independent research study (Chlan et al., 2019 ). Details of this programme are described elsewhere (Chlan et al., 2019 ; National Institute of Nursing Research, 2016 ).
The project team developed a model of nursing research to guide the foci for nurse scientists' research at the institution and to generate new nursing knowledge based on needs that arise from the practice setting. The model was also intended to encompass strategic priorities established both by the institution and the field of nursing science. No ethics approval was required for this project.
The team started the process of model development by conducting a literature review regarding (1) existing models of nursing research and evidence‐based practice, (2) nursing science, (3) the nurse scientist role, (4) national and international nursing research strategic priorities and (5) research strategies to transform health care. In addition, the team sought input from multidisciplinary stakeholders at the institution regarding their perception of the current and potential future contributions of nursing science to the practice. Finally, organisational resources describing the research environment were used to inform the model. Thus, it is a model rooted in practice, rather than a theory‐based model.
4.1. The Mayo Clinic nursing research model
The MCNR model is focused on three primary areas across multiple diseases, illnesses, and healthcare settings: symptom science, self‐management science and caregiving science. With a focus in these areas, nurse scientists leverage team science, big data, innovation and technology to move knowledge generation quicker along the discovery, translation and application continuum to meet the needs of patients and caregivers.
The following assumptions informed the development of the model. First, nursing research is vital for the generation of new knowledge to improve the health and well‐being of patients and their caregivers. Second, the health and well‐being of individuals with complex conditions are enhanced by developing and testing patient‐centred interventions through research that focuses on the science of symptom assessment and management, self‐management and caregiving. The MCNR model was developed to guide how this vision will be implemented in a clinical setting with programmes of nursing research aligned to inform and transform health care.
4.2. Patients and caregivers as the focal point of the model
At the centre of the model (Figure (Figure1) 1 ) are the patient and caregiver with complex needs—medical, physical or psychosocial—around which all other elements in the model centre. The nurse scientist focuses on a better understanding of those needs and the testing of interventions used to address them, with the definitive goal of improving patients' and caregivers' health and well‐being. For the purposes of this model, health is defined from a holistic, phenomenological perspective of optimal overall physical, mental, spiritual, social and role functioning (Saylor, 2004 ; Watson, 2008 ); and well‐being is designated as individuals' perceptions, judgements and expectations regarding their health (Saylor, 2004 ; Sullivan, 2003 ). These foci are consistent with the patient‐centred model of care in which patients are viewed as a whole and their individual viewpoints and characteristics are taken into consideration when making decisions regarding care (Zhao et al., 2016 ). It is also congruent with the mission and values of Mayo Clinic (Mayo Clinic, 2021 ), as well as the profession of nursing (Spurlock, 2019 ).
4.3. MCNR model scientific foci
The generation of symptom science, self‐management science and caregiving science are the scientific foci that promote the health and well‐being of patients and caregivers in a practice‐based, patient‐centred clinical setting. It is through the conduct of scientific investigation in these three main areas, described below, that nursing research seeks solutions to unmet, complex health needs of patients and caregivers.
Symptom science seeks to transform the practice using biological, clinical and/or behavioural approaches to investigate symptoms aiming to individualise care and assess patient‐reported outcomes such as quality of life and well‐being (Grady, 2017 ). Self‐management science is based on a complex set of cognitive and behavioural self‐regulation responses that individuals engage in to manage chronic illnesses or factors that increase the risk for illness (Araújo‐Soares et al., 2019 ). Research to support self‐management includes developing and evaluating a broad range of interventions often focused on providing education and guidance for managing specific illnesses, partnering with healthcare providers and coping with challenges of living with chronic illness (Allegrante et al., 2019 ).
Caregiving science is research that explores effective approaches to reduce burden on and promote the health and well‐being of professional and lay caregivers (Grady, 2017 ). Research that examines methods to include caregivers in the care process and to design and test interventions that include them has the potential to significantly contribute to improved patient outcomes and patient‐centred care (Littleton‐Kearney & Grady, 2018 ).
4.4. Leveraging team science, big data, innovation, and technology
In addition to cutting‐edge research methods, nurse scientists leverage team science, big data, innovation and technology as tools, resources and methods to seek solutions to unmet health needs of patients and caregivers (Brennan & Bakken, 2015 ; Conn, 2019 ; Grady & Gough, 2018 ). Within the MCNR model, these four resources and methodologies contribute to the advancement of nursing science in the areas of symptom, self‐management, and caregiving. Team science leverages the strengths and expertise of professionals trained in different disciplines or nursing specialties through a collaborative effort to address a scientific challenge (Bennett & Gadlin, 2012 ). Team‐based research initiatives can be uni‐ or multidisciplinary groups, and teams can be large or small (Conn, 2019 ). In team science, multiple stakeholders contribute unique perspectives on the topic at hand and are deeply engaged in the project (Bennett et al., 2018 ). The World Health Organisation has acknowledged the importance of team‐based research through implementation of nursing collaborating centres, which focus on collaborative research of global or regional importance (National Institutes of Health, 2015 ).
Big data science allows researchers to analyse large and complex volumes of information that are newly available at unprecedented rates from sources such as electronic health records, large databases, sensor‐enabled equipment, imaging techniques, smart devices and high‐throughput genetic sequencing methods (Fernandes et al., 2012 ). Through the application of big data research methods, including artificial intelligence, researchers can discover new ways of understanding and addressing the needs of the patient (Fernandes et al., 2012 ). For example, big data methodologies can be implemented to maximise the utility of patient‐reported outcome data in order to capture the patients' perspectives on how their disease, and the treatment of their disease, is impacting their lives. These data can be used to inform clinical decision‐making, predict long‐term outcomes and identify future innovations in health technologies and other interventions (Calvert et al., 2015 ). This patient‐centric approach ultimately allows healthcare providers to have a better understanding of how individuals are living with and managing their illness, and to make more informed decisions regarding personalised interventions that will have a measurable impact on the patient experience (Brennan & Bakken, 2015 ).
Innovation is defined as a creative, fast‐moving endeavour that involves scientific methods and improvisation to design unique solutions that change the world (Mayo Clinic Center for Innovation, 2020 ). Innovative research uses novel theoretical concepts, methodologies and interventions to challenge current clinical practice paradigms. Innovations in health care can be seen in product innovation for the introduction of new types of goods and services, and in process innovation, which is centred on enhancing internal processes for the production of high‐quality care (Arshad et al., 2018 ; Govindasamy & Wattal, 2018 ; Thune & Mina, 2016 ).
Technology in medical research involves innovations that impact health or healthcare delivery (Healthcare News & Insights, 2020 ; Martins & Del Sasso, 2008 ). Biotechnology, machine learning, pharmaceuticals, information technology, remote monitoring and medical devices are examples of technology. Other technologies include software and applications for self‐management and symptom tracking. Technologies can maximise efficiency and access to health care, such as digital solutions to connect patients to the appropriate provider (National Institute of Mental Health, 2020 ).
4.5. Discovery‐translation‐application continuum
Research conducted at Mayo Clinic occurs along a continuum to address unmet patient needs. The process by which new information makes its way into practice along this continuum is through discovery, translation and application, depicted in the outermost ring of the model in Figure Figure1. 1 . Discovery uses scientific methods to seek solutions to improve the health and well‐being of patients with complex conditions; translation is the development and testing of possible solutions; and application is the dissemination, integration, and evaluation of solutions into practice (Ammerman et al., 2014 ).
Nursing research contributes to innovation at all points along the discovery‐translation‐application continuum, continually advancing science, transforming patient care and improving outcomes (Grady, 2017 ). Guided by the MCNR model, nurse scientists discover answers to puzzling clinical questions that can be translated and applied directly to clinical practice to improve patient care as rapidly and as safely as possible. There are at least seven implementation science models or frameworks available to guide translation of findings to practice. Systematic reviews show variability in their scope and application so selection of an implementation framework according to the context of change is key (Dintrans et al., 2019 ; Moullin et al., 2015 ). In our setting, translation is achieved through clinical partnerships where the department's evidence‐based practice model is used to guide implementation. As depicted in the model in circular form (Figure (Figure1), 1 ), this process is iterative rather than linear. Discoveries are made through observation, discussion or other forms of data. These discoveries, seen through the nursing lens, may have broader applications to be considered. Further, empirical evidence is needed prior to implementing new discoveries into practice. During implementation, new discoveries and applications may come to light.
5. EXEMPLARS OF THE MAYO CLINIC NURSING RESEARCH MODEL
The overall purpose of the MCNR model is to provide a coordinated focus and consistent approach that guides and prioritises practice‐based nursing research. Nurse scientists use the model in their own focused areas of research as well as to guide nurses in the conduct of research that arises from their practice. Outlined below are exemplars of how the MCNR model guides the conduct of practice‐based research among nurse scientists at Mayo Clinic. Examples of how the model has informed research are presented. Not all aspects of the model are evident in each exemplar.
The first nursing research exemplar, within the domain of symptom science (second ring of the MCNR model), aims to address unmet needs of critically ill patients (centre of model) related to comfort‐promoting interventions. Under the mentorship of a PhD‐prepared nurse scientist, this descriptive, cross‐sectional study is being conducted by two practising ICU nurses who first identified in their own clinical setting the problems of: (1) numerous sources of discomfort among ICU patients; (2) the absence of objective assessment of these discomforts as distinct from objective assessment of pain; and (3) the inability to intervene appropriately with effective comfort‐promoting interventions. Next, they identified the distinction between discomfort and pain. They are currently assessing, describing and quantifying the contributing sources of discomfort experienced by nonmechanically ventilated ICU patients using the Discomforts Perceived by ICU Patients instrument, a modified version of the French instrument Inconforts des Patients de REAnimation (IPREA) questionnaire (Baumstarck et al., 2019 ). The end‐product of this study will be the discovery of new knowledge (outer ring of model) to inform ICU nursing practice regarding discomfort‐producing stimuli. Future areas of investigation would include developing and testing interventions (translation of possible solutions through clinical trials), of which those that are found to be effective would then be directly applied in the setting of ICU clinical nursing practice contributing to symptom science for critically ill patients.
An exemplar within the domain of caregiving science (second ring of MCNR model) is a multidisciplinary trial co‐led by a nurse scientist and physician (team science—third ring of model). The investigators noted that patients with advanced cancer or those nearing the end‐of‐life experience significant, unique distress related to their disease, treatment and impending mortality. In addition, they noted a lack of evidence on best methods to manage psychosocial distress in patients and caregivers with complex needs (centre of model). Thus, they designed a study to determine the feasibility of a modified version of the Resilient Living Program (The Resilient Option, 2020 ) that is tailored to the needs of patients with advanced cancer and their adult caregivers. Outcomes of the study include feasibility of participant recruitment, acceptability of the intervention and self‐reports of resilience, quality of life, stress, anxiety, sleep, fatigue and caregiver role overload. Findings from this study will lead to the discovery (outer ring of model) of best practices for integrating a resilience training programme within the care of patients with complex needs (centre of model), and their caregivers. Future studies will examine the outcomes of revised training programmes that are more effectively tailored to the unique needs of these populations.
Recognising the emotional distress their patients endure, a group of nurses working on the bone marrow transplant (BMT) unit expressed interest in specific nursing interventions to support their patients' emotional well‐being. Although they knew from their clinical experience that hospitalisation for BMT is quite stressful, they wanted to have a better understanding of when the most distressing times were for the patients, and what aspects of undergoing BMT were the most stressful. A review of the literature did not identify the specific information they were seeking. In collaboration with a nurse scientist and social workers on the unit, they implemented a descriptive study aimed at answering their questions. The study is in progress, and when finished, the results will inform both nursing and social work practice. This is an example of how clinical nurses identified a need centred around the health and well‐being of complex patients (centre of the MCNR model), focused on symptom science (second ring of the model), and used team science (third ring of the model) to discover new information (outer ring of the model) from which nursing interventions can be developed and tested.
The final nursing research exemplar is within the domains of symptom science and self‐management science (second ring of the MCNR model) to address the unmet needs of complex critically ill patients (centre of model). As of this writing, a randomised controlled clinical trial is testing the efficacy of self‐administered versus intensive care unit (ICU) nurse‐administered sedative therapy for anxiety in critically ill patients receiving mechanical ventilatory support (1R01 {"type":"entrez-nucleotide","attrs":{"text":"HL130881","term_id":"1051909465","term_text":"HL130881"}} HL130881 ). Primary outcomes of the study include anxiety, duration of mechanical ventilation, delirium, level of arousal, alertness and sedative exposure. Post‐ICU outcomes are also being examined and include functional status, depression and health‐related quality of life. Findings from this clinical trial will be applied to the practice setting (outer ring of the model) to implement patient‐centred interventions that improve not only ICU outcomes but also quality of life during the trajectory of recovery from critical illness and injury.
6. DISCUSSION
The MCNR model guides nursing research across settings and prioritises inquiry on symptom science, self‐management science and caregiving science. The model is unique in that it specifically focuses on generation of nursing knowledge through the focus and conduct of research in a practice‐based clinical setting. Few such models have been found in the literature; those that are available focus on advancing bedside nurses' involvement in research (Brewer et al., 2009 ; Stutzman et al., 2016 ). Robust programmes of nursing research remain relatively uncommon in clinical settings (Robichaud‐Ekstrand, 2016 ). Availability of time and resources needed to facilitate clinical research are often constrained. Even in large academic medical centres with institutional commitment, the contributions of nursing research often go unrecognised, even from within the nursing profession. The MCNR model can be used to communicate the scope and focus of nursing research, from which studies can be developed to address significant problems impacted by nursing practice.
In creating the MCNR model, we sought to demonstrate the unique contributions of nursing research at our institution and develop a framework to guide the overall direction of nursing research. This model may have limited application in nonclinical settings; however, other institutions may glean information to develop similar models tailored to their settings. Adaptation of the model to fit a specific organisational context and available resources may be necessary. Although the model is implemented in a setting rich in human and other resources to guide nursing science, it could easily be used in settings with more limited resources to help frame the scope and function of nursing science. However, this model was primarily developed for use in clinical settings in which some resources for the conduct of research exist. Unfortunately, there are still many settings where the resources needed to facilitate nursing research are sparse or non‐existent.
The MCNR model can also be integrated with existing models of nursing research. The National Institutes of Health Symptom Science Model is one example of a complementary model that can be used in tandem with the MCNR. The Symptom Science Model provides a guide for researchers to study complex symptoms experienced by individuals and incorporates the components of phenotypic characterisation, biomarker discovery and clinical application, with an overall goal of symptom reduction and improvement (Cashion et al., 2016 ). These methodologic components can be used to advance the care of patients with complex needs in the context of the institutional priorities and infrastructure described in the model. The MCNR model can be applied in several ways to advance scientific knowledge in the areas of symptoms, self‐management and caregiving. The model incorporates advancements in biological sciences, technology and big data methods to meet the needs of patients in a holistic way using nursing's unique body of knowledge (Henly et al., 2015 ). While nurse scientists may not have extensive expertise in all areas, collaborating with other scientists and clinicians who have complementary expertise ensures that investigations incorporate the best science and technology from other fields to inform nursing knowledge and practice.
As nurse scientists are increasingly employed in clinical settings, it will become more important to evaluate and publish outcomes of models, including this one. Nursing research within our institution is evolving to best meet the needs of patients. The MCNR model is a step in the process to define our direction and differentiate our areas of expertise from those of other disciplines.
The model is not without limitations. The MCNR Model was developed by nurse scientists within the Division of Nursing Research to serve as a guide and focus for our conduct of research, and to communicate our work with others. It is a reflection of the current foci of nursing research at a single institution and, as noted earlier, may need to be adapted to meet the needs of other institutions. It is intended to serve as a starting point for the infrastructure needed to generate research ideas and to serve as a guide to focus the conduct of research in distinct scientific areas in practice‐based settings. It is not intended to constrain research foci that are outside of this model. The model may be of lower utility in settings where nurse scientists are not available. It will be revisited periodically by the research team and stakeholders to ensure that it reflects the current focus of nursing research throughout the institution.
7. CONCLUSION
Nurse scientists embedded in healthcare settings are uniquely positioned to inform translation of research findings to practice. As health care evolves and the needs of patients and caregivers become more complex, the importance of studying symptoms, self‐management and caregiving is becoming increasingly critical. Nurse scientists leverage team science, big data, innovation and technology to move knowledge generation along the continuum of discovery, translation and application. The MCNR model can be used to advance generation of new nursing knowledge to improve the health and well‐being of patients and caregivers.
8. RELEVANCE TO CLINICAL PRACTICE
The MCNR model can be used by nurse scientists embedded in healthcare settings to address clinically relevant questions and ultimately improve the overall physical, mental, spiritual, social and role functioning of patients and caregivers, as well as to enhance individuals' perceptions, judgements and expectations regarding their health. The model provides a structure for addressing nursing science priorities through the discovery, translation and application continuum, and advancing the generation of new nursing knowledge.
CONFLICT OF INTEREST
The authors report no conflicts of interest with this manuscript.
AUTHOR CONTRIBUTIONS
Conception and design of the work, drafting of the article, critical revisions of the article and final approval of the version to be published: All authors.
DATA AVAILABILITY STATEMENT
- Allegrante, J. P. , Wells, M. T. , & Peterson, J. C. (2019). Interventions to support behavioral self‐management of chronic diseases . Annual Review of Public Health , 40 , 127–146. [ PMC free article ] [ PubMed ] [ Google Scholar ]
- Ammerman, A. , Smith, T. W. , & Calancie, L. J. A. (2014). Practice‐based evidence in public health: Improving reach, relevance, and results . Annual Review of Public Health , 35 , 47–63. [ PubMed ] [ Google Scholar ]
- Araújo‐Soares, V. , Hankonen, N. , Presseau, J. , Rodrigues, A. , & Sniehotta, F. F. (2019). Developing behavior change interventions for self‐management in chronic illness: An integrative overview . European Psychologist , 24 ( 1 ), 7. [ PMC free article ] [ PubMed ] [ Google Scholar ]
- Arshad, H. , Radić, M. , & Radić, D. (2018). Patterns of frugal innovation in healthcare . Technology Innovation Management Review , 8 ( 4 ), 28–37. [ Google Scholar ]
- Baumstarck, K. , Boucekine, M. , Estagnasie, P. , Geantot, M.‐A. , Berric, A. , Simon, G. , Floccard, B. , Signouret, T. , Fromentin, M. , Nyunga, M. , Sossou, A. , Venot, M. , Robert, R. , Follin, A. , Audibert, J. , Renault, A. , Garrouste‐Orgeas, M. , Collange, O. , Levrat, Q. , … Kalfon, P. (2019). Assessment of patients' self‐perceived intensive care unit discomforts: Validation of the 18‐item version of the IPREA . Health and Quality of Life Outcomes , 17 ( 1 ), 29. [ PMC free article ] [ PubMed ] [ Google Scholar ]
- Bennett, L. M. , & Gadlin, H. (2012). Collaboration and team science: from theory to practice . Journal of Investigative Medicine , 60 ( 5 ), 768–775. [ PMC free article ] [ PubMed ] [ Google Scholar ]
- Bennett, L. M. , Gadlin, H. , & Marchand, C. (2018). Collaboration team science: Field guide . US Department of Health & Human Services, National Institutes of Health. [ Google Scholar ]
- Boyer, E. (1990). Scholarship reconsidered: Priorities for the professoriate . Retrieved from Princeton, NJ: https://www.hadinur.com/paper/BoyerScholarshipReconsidered.pdf [ Google Scholar ]
- Brennan, P. F. , & Bakken, S. (2015). Nursing needs big data and big data needs nursing . Journal of Nursing Scholarship , 47 ( 5 ), 477–484. [ PubMed ] [ Google Scholar ]
- Brewer, B. B. , Brewer, M. A. , & Schultz, A. A. (2009). A collaborative approach to building the capacity for research and evidence‐based practice in community hospitals . Nursing Clinics of North America , 44 ( 1 ), 11–25, ix. 10.1016/j.cnur.2008.10.003 [ PubMed ] [ CrossRef ] [ Google Scholar ]
- Buckwalter, K. C. , Cullen, L. , Hanrahan, K. , Kleiber, C. , McCarthy, A. M. , Rakel, B. , Steelman, V. , Tripp‐Reimer, T. , & Tucker, S. (2017). Iowa model of evidence‐based practice: Revisions and validation . Worldviews on Evidence‐Based Nursing , 14 ( 3 ), 175–182. 10.1111/wvn.12223. [ PubMed ] [ CrossRef ] [ Google Scholar ]
- Calvert, M. , Thwaites, R. , Kyte, D. , & Devlin, N. (2015). Putting patient‐reported outcomes on the ‘Big Data Road Map’ . Journal of the Royal Society of Medicine , 108 ( 8 ), 299–303. [ PMC free article ] [ PubMed ] [ Google Scholar ]
- Cashion, A. K. , Gill, J. , Hawes, R. , Henderson, W. A. , & Saligan, L. (2016). National Institutes of Health Symptom Science Model sheds light on patient symptoms . Nursing Outlook , 64 ( 5 ), 499–506. 10.1016/j.outlook.2016.05.008. [ PMC free article ] [ PubMed ] [ CrossRef ] [ Google Scholar ]
- Cashion, A. K. , & Grady, P. A. (2015). The National Institutes of Health/National Institutes of Nursing Research intramural research program and the development of the National Institutes of Health Symptom Science Model . Nursing Outlook , 63 ( 4 ), 484–487. 10.1016/j.outlook.2015.03.001. [ PMC free article ] [ PubMed ] [ CrossRef ] [ Google Scholar ]
- Chan, R. , Gardner, G. E. , Webster, J. , & Geary, A. (2010). Building research capacity in the nursing workforce: The design and evaluation of the nurse researcher role . Australian Journal of Advanced Nursing , 27 ( 4 ), 62–69. [ Google Scholar ]
- Chlan, L. L. , Johnson, P. O. , Peterson, P. E. , Striegel, L. , Au, G. , Fritsche, A. , Bursiek, A. , Engelmann, A. , & Tofthagen, C. (2019). Strategies to promote nurses' engagement in clinical research: Description of two nurse scholar programs . Journal of Professional Nursing , 36 ( 3 ), 111–115. 10.1016/j.profnurs.2019.11.001. [ PubMed ] [ CrossRef ] [ Google Scholar ]
- Cohen, M. Z. , Thompson, C. B. , Yates, B. , Zimmerman, L. , & Pullen, C. H. (2015). Implementing common data elements across studies to advance research . Nursing Outlook , 63 ( 2 ), 181–188. 10.1016/j.outlook.2014.11.006. [ PMC free article ] [ PubMed ] [ CrossRef ] [ Google Scholar ]
- Conn, V. S. (2019). Team science: Challenging opportunity . SAGE Publications. [ PubMed ] [ Google Scholar ]
- Dang, D. , & Dearholt, S. L. (Eds.) (2018). Johns Hopkins nursing evidence‐based practice: Model and guidelines (3rd ed.). Sigma Theta Tau International. [ Google Scholar ]
- Dintrans, P. V. , Bossert, T. J. , Sherry, J. , & Kruk, M. E. (2019). A synthesis of implementation science frameworks and application to global health gaps . Global Health Research and Policy , 4 ( 1 ), 25. [ PMC free article ] [ PubMed ] [ Google Scholar ]
- Fernandes, L. M. , O'Connor, M. , Weaver, V. J. J. O. A. (2012). Big data, bigger outcomes: Healthcare is embracing the big data movement, hoping to revolutionize HIM by distilling vast collection of data for specific analysis . Journal of AHIMA , 83 ( 10 ), 38–43. [ PubMed ] [ Google Scholar ]
- Govindasamy, S. , & Wattal, S. (2018). Linking dynamic capabilities and healthcare innovations: A case study approach. Paper presented at the 2018 engaged management scholarship conference: Philadelphia, PA . [ Google Scholar ]
- Grady, P. A. (2017). Advancing science, improving lives: NINR's new strategic plan and the future of nursing science . Journal of Nursing Scholarship , 49 ( 3 ), 247. [ PubMed ] [ Google Scholar ]
- Grady, P. A. , & Gough, L. L. (2018). Using nursing science to inform health policy: The role of the National Institute of Nursing Research . Annual Review of Nursing Research , 36 ( 1 ), 131–149. [ PubMed ] [ Google Scholar ]
- Grady, P. A. , & Hinshaw, A. S. (2017). Using nursing research to shape health policy . Springer Publishing Company. [ Google Scholar ]
- Healthcare News & Insights (2020). Medical technology . Healthcare Business & Technology. http://www.healthcarebusinesstech.com/medical‐technology/ [ Google Scholar ]
- Henly, S. J. , McCarthy, D. O. , Wyman, J. F. , Stone, P. W. , Redeker, N. S. , McCarthy, A. M. , & Conley, Y. P. (2015). Integrating emerging areas of nursing science into PhD programs . Nursing Outlook , 63 ( 4 ), 408–416. 10.1016/j.outlook.2015.04.010. [ PubMed ] [ CrossRef ] [ Google Scholar ]
- Hickey, J. , Duffy, L. V. , Hinkle, J. L. , Prendergast, V. , Rhudy, L. M. , Sullivan, C. , & Villanueva, N. E. (2019). Scholarship in neuroscience nursing . Journal of Neuroscience Nursing , 51 ( 5 ), 243–248. [ PubMed ] [ Google Scholar ]
- Joanna Briggs Institute (2016). The JBI model of evidence‐based healthcare . https://joannabriggs.org/jbi‐approach.html [ Google Scholar ]
- Kowalski, M. O. (2020). Nursing research at an ANCC Magnet® designated medical center . Applied Nursing Research , 55 , 151290. [ PubMed ] [ Google Scholar ]
- Littleton‐Kearney, M. T. , & Grady, P. A. (2018). The science of caregiving bringing voices together: summary of National Institute of Nursing Research's 2017 summit . Nursing Outlook , 66 ( 2 ), 157–159. [ PubMed ] [ Google Scholar ]
- Martins, C. R. , & Dal Sasso, G. T. M. (2008). Technology: definitions and reflections for nursing and health care practice . Texto & Contexto ‐ Enfermagem , 17 ( 1 ), 13–14. [ Google Scholar ]
- Mayo Clinic (2021). Mayo Clinic mission and values . https://www.mayoclinic.org/about‐mayo‐clinic/mission‐values [ Google Scholar ]
- Mayo Clinic Center for Innovation (2020). What we do . http://centerforinnovation.mayo.edu/what‐we‐do/ [ Google Scholar ]
- Moullin, J. C. , Sabater‐Hernández, D. , Fernandez‐Llimos, F. , & Benrimoj, S. I. (2015). A systematic review of implementation frameworks of innovations in healthcare and resulting generic implementation framework . Health Research Policy and Systems , 13 ( 1 ), 16. 10.1186/s12961-015-0005-z. [ PMC free article ] [ PubMed ] [ CrossRef ] [ Google Scholar ]
- National Institute of Mental Health (2020, September 2019). Technology and the future of mental health treatment . https://www.nimh.nih.gov/health/topics/technology‐and‐the‐future‐of‐mental‐health‐treatment/index.shtml [ Google Scholar ]
- National Institute of Nursing Research (2016). The NIINR strategic plan: Advancing science, improving lives . https://www.ninr.nih.gov/sites/files/docs/NINR_StratPlan2016_reduced.pdf [ Google Scholar ]
- National Institutes of Health (2015). Global health matters . https://www.fic.nih.gov/News/GlobalHealthMatters/january‐february‐2015/Documents/fogarty‐nih‐global‐health‐matters‐newsletter‐january‐february‐2015.pdf [ Google Scholar ]
- Nightingale, F. (1992). Notes on nursing: What it is, and what it is not . Lippincott Williams & Wilkins. [ Google Scholar ]
- Powell, K. (2015). Nursing research: Nurses know best . Nature , 522 ( 7557 ), 507–509. [ PubMed ] [ Google Scholar ]
- Robichaud‐Ekstrand, S. (2016). New Brunswick nurses' views on nursing research, and factors influencing their research activities in clinical practice . Nursing & Health Sciences , 18 ( 2 ), 246–255. 10.1111/nhs.12261. [ PubMed ] [ CrossRef ] [ Google Scholar ]
- Sadeghnezhad, M. , Heshmati Nabavi, F. , Najafi, F. , Kareshki, H. , & Esmaily, H. (2018). Mutual benefits in academic‐service partnership: An integrative review . Nurse Education Today , 68 , 78–85. 10.1016/j.nedt.2018.05.019. [ PubMed ] [ CrossRef ] [ Google Scholar ]
- Saylor, C. (2004). The circle of health: A health definition model . Journal of Holistic Nursing , 22 ( 2 ), 97–115. [ PubMed ] [ Google Scholar ]
- Spurlock, D. Jr (2019). Toward an inclusive vision of both nursing practice and nursing science . Journal of Nursing Education , 58 ( 6 ), 319–320. 10.3928/01484834-20190521-01. [ PubMed ] [ CrossRef ] [ Google Scholar ]
- Stutzman, S. , Olson, D. , Supnet, C. , Harper, C. , Brown‐Cleere, S. , McCulley, B. , & Goldberg, M. (2016). Promoting bedside nurse‐led research through a dedicated neuroscience nursing research fellowship . Journal of Nursing Administration , 46 ( 12 ), 648–653. 10.1097/nna.0000000000000421. [ PubMed ] [ CrossRef ] [ Google Scholar ]
- Sullivan, M. (2003). The new subjective medicine: taking the patient's point of view on health care and health . Social Science & Medicine , 56 ( 7 ), 1595–1604. [ PubMed ] [ Google Scholar ]
- The Resilient Option (2020). Our research . https://www.resilientoption.com/p/our‐research [ Google Scholar ]
- Thune, T. , & Mina, A. (2016). Hospitals as innovators in the health‐care system: A literature review and research agenda . Research Policy , 45 ( 8 ), 1545–1557. [ Google Scholar ]
- Trego, L. L. (2017). Developing a military nurse scientist program of research: A military women's health exemplar . Nursing Outlook , 65 ( 5 ), S130–S139. [ PubMed ] [ Google Scholar ]
- Watson, J. (2008). Nursing: The philosophy and science of caring (Rev ed.). University Press of Colorado. [ Google Scholar ]
- Zhao, J. , Gao, S. , Wang, J. , Liu, X. , & Hao, Y. (2016). Differentiation between two healthcare concepts: Person‐centered and patient‐centered care . International Journal of Nursing Sciences , 3 ( 4 ), 398–402. [ Google Scholar ]
Academia.edu no longer supports Internet Explorer.
To browse Academia.edu and the wider internet faster and more securely, please take a few seconds to upgrade your browser .
- We're Hiring!
- Help Center
- Health Sciences
- Most Cited Papers
- Most Downloaded Papers
- Newest Papers
- Last »
- Public Health Follow Following
- Medicine Follow Following
- Epidemiology Follow Following
- Pharmacology Follow Following
- Health Promotion Follow Following
- Pharmacy Follow Following
- Research Methodology Follow Following
- Health Policy Follow Following
- Medical Sciences Follow Following
- Social epidemiology Follow Following
Enter the email address you signed up with and we'll email you a reset link.
- Academia.edu Journals
- We're Hiring!
- Help Center
- Find new research papers in:
- Earth Sciences
- Cognitive Science
- Mathematics
- Computer Science
- Academia ©2024
- See us on facebook
- See us on twitter
- See us on youtube
- See us on linkedin
- See us on instagram
Massive biomolecular shifts occur in our 40s and 60s, Stanford Medicine researchers find
Time marches on predictably, but biological aging is anything but constant, according to a new Stanford Medicine study.
August 14, 2024 - By Rachel Tompa

We undergo two periods of rapid change, averaging around age 44 and age 60, according to a Stanford Medicine study. Ratana21 /Shutterstock.com
If it’s ever felt like everything in your body is breaking down at once, that might not be your imagination. A new Stanford Medicine study shows that many of our molecules and microorganisms dramatically rise or fall in number during our 40s and 60s.
Researchers assessed many thousands of different molecules in people from age 25 to 75, as well as their microbiomes — the bacteria, viruses and fungi that live inside us and on our skin — and found that the abundance of most molecules and microbes do not shift in a gradual, chronological fashion. Rather, we undergo two periods of rapid change during our life span, averaging around age 44 and age 60. A paper describing these findings was published in the journal Nature Aging Aug. 14.
“We’re not just changing gradually over time; there are some really dramatic changes,” said Michael Snyder , PhD, professor of genetics and the study’s senior author. “It turns out the mid-40s is a time of dramatic change, as is the early 60s. And that’s true no matter what class of molecules you look at.”
Xiaotao Shen, PhD, a former Stanford Medicine postdoctoral scholar, was the first author of the study. Shen is now an assistant professor at Nanyang Technological University Singapore.
These big changes likely impact our health — the number of molecules related to cardiovascular disease showed significant changes at both time points, and those related to immune function changed in people in their early 60s.
Abrupt changes in number
Snyder, the Stanford W. Ascherman, MD, FACS Professor in Genetics, and his colleagues were inspired to look at the rate of molecular and microbial shifts by the observation that the risk of developing many age-linked diseases does not rise incrementally along with years. For example, risks for Alzheimer’s disease and cardiovascular disease rise sharply in older age, compared with a gradual increase in risk for those under 60.
The researchers used data from 108 people they’ve been following to better understand the biology of aging. Past insights from this same group of study volunteers include the discovery of four distinct “ ageotypes ,” showing that people’s kidneys, livers, metabolism and immune system age at different rates in different people.

Michael Snyder
The new study analyzed participants who donated blood and other biological samples every few months over the span of several years; the scientists tracked many different kinds of molecules in these samples, including RNA, proteins and metabolites, as well as shifts in the participants’ microbiomes. The researchers tracked age-related changes in more than 135,000 different molecules and microbes, for a total of nearly 250 billion distinct data points.
They found that thousands of molecules and microbes undergo shifts in their abundance, either increasing or decreasing — around 81% of all the molecules they studied showed non-linear fluctuations in number, meaning that they changed more at certain ages than other times. When they looked for clusters of molecules with the largest changes in amount, they found these transformations occurred the most in two time periods: when people were in their mid-40s, and when they were in their early 60s.
Although much research has focused on how different molecules increase or decrease as we age and how biological age may differ from chronological age, very few have looked at the rate of biological aging. That so many dramatic changes happen in the early 60s is perhaps not surprising, Snyder said, as many age-related disease risks and other age-related phenomena are known to increase at that point in life.
The large cluster of changes in the mid-40s was somewhat surprising to the scientists. At first, they assumed that menopause or perimenopause was driving large changes in the women in their study, skewing the whole group. But when they broke out the study group by sex, they found the shift was happening in men in their mid-40s, too.
“This suggests that while menopause or perimenopause may contribute to the changes observed in women in their mid-40s, there are likely other, more significant factors influencing these changes in both men and women. Identifying and studying these factors should be a priority for future research,” Shen said.
Changes may influence health and disease risk
In people in their 40s, significant changes were seen in the number of molecules related to alcohol, caffeine and lipid metabolism; cardiovascular disease; and skin and muscle. In those in their 60s, changes were related to carbohydrate and caffeine metabolism, immune regulation, kidney function, cardiovascular disease, and skin and muscle.
It’s possible some of these changes could be tied to lifestyle or behavioral factors that cluster at these age groups, rather than being driven by biological factors, Snyder said. For example, dysfunction in alcohol metabolism could result from an uptick in alcohol consumption in people’s mid-40s, often a stressful period of life.
The team plans to explore the drivers of these clusters of change. But whatever their causes, the existence of these clusters points to the need for people to pay attention to their health, especially in their 40s and 60s, the researchers said. That could look like increasing exercise to protect your heart and maintain muscle mass at both ages or decreasing alcohol consumption in your 40s as your ability to metabolize alcohol slows.
“I’m a big believer that we should try to adjust our lifestyles while we’re still healthy,” Snyder said.
The study was funded by the National Institutes of Health (grants U54DK102556, R01 DK110186-03, R01HG008164, NIH S10OD020141, UL1 TR001085 and P30DK116074) and the Stanford Data Science Initiative.
- Rachel Tompa Rachel Tompa is a freelance science writer.
About Stanford Medicine
Stanford Medicine is an integrated academic health system comprising the Stanford School of Medicine and adult and pediatric health care delivery systems. Together, they harness the full potential of biomedicine through collaborative research, education and clinical care for patients. For more information, please visit med.stanford.edu .
Hope amid crisis
Psychiatry’s new frontiers
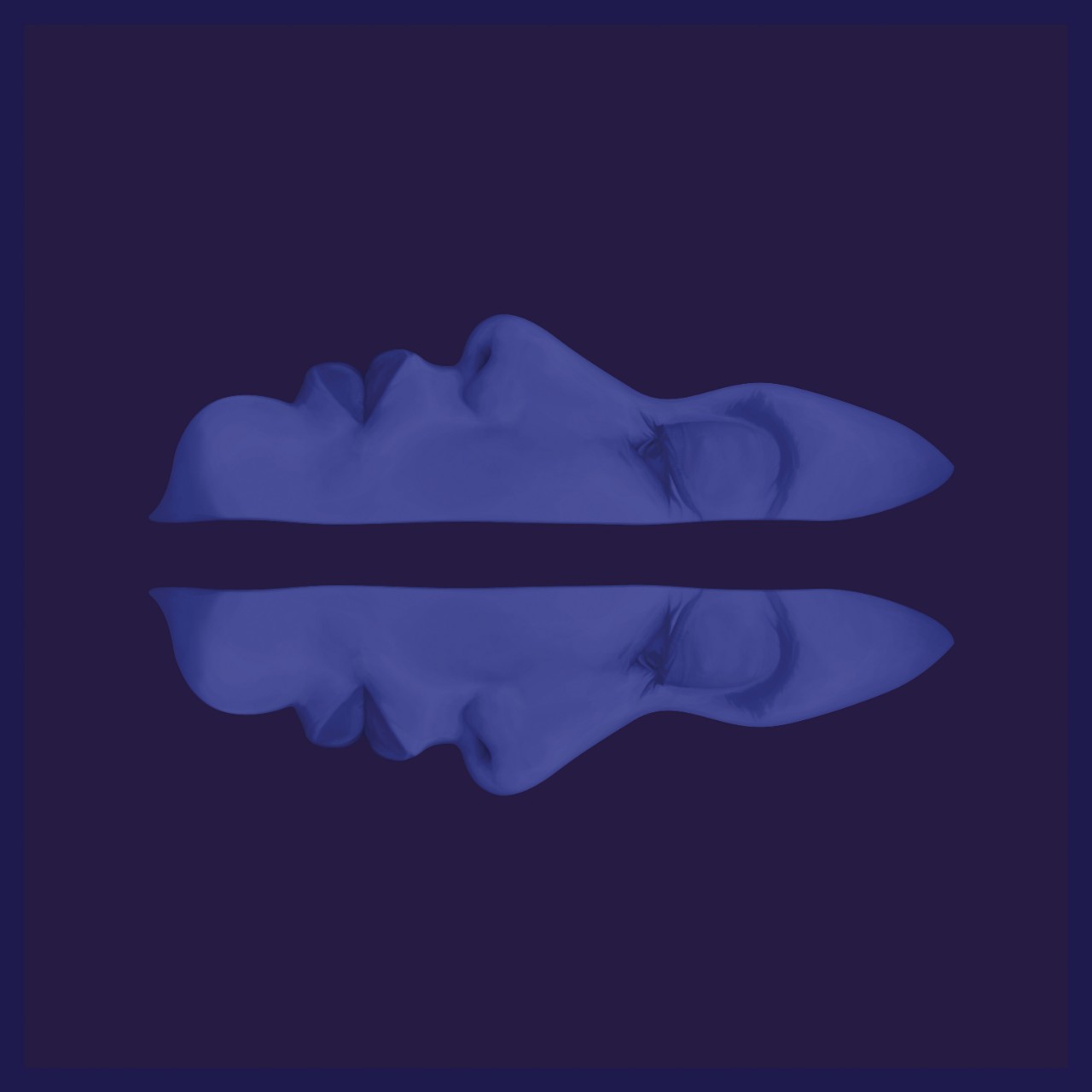

IMAGES
COMMENTS
The New England Journal of Medicine (NEJM) is a weekly general medical journal that publishes new medical research and review articles, and editorial opinion on a wide variety of topics of ...
Here, the authors investigate the fluctuations of physiological indices along aging trajectories and observed a characteristic decrease in the organism state recovery rate. Timothy V. Pyrkov ...
Here, using a large-scale cohort study, the authors show that moderate-to-vigorous PA at the optimal time of day robustly predicts lower mortality risk and may maximize the beneficial effect of PA ...
PubMed® comprises more than 37 million citations for biomedical literature from MEDLINE, life science journals, and online books. Citations may include links to full text content from PubMed Central and publisher web sites.
The health sciences study all aspects of health, disease and healthcare. This field of study aims to develop knowledge, interventions and technology for use in healthcare to improve the treatment ...
3.3 million articles on ScienceDirect are open access. Articles published open access are peer-reviewed and made freely available for everyone to read, download and reuse in line with the user license displayed on the article. ScienceDirect is the world's leading source for scientific, technical, and medical research.
Health Science Reports is a broad scope open access journal publishing research and commentaries across all medical and health sciences disciplines, including clinical care, public health, and epidemiology. We welcome clinical studies, scientific research with significant clinical implications, as well as reports on methods and research design, health services, public health, and medical ...
JOURNAL HOMEPAGE. Research Methods in Medicine & Health Sciences is a peer reviewed journal, publishing rigorous research on established "gold standard" methods and new cutting edge research methods in the health sciences and clinical medicine. View full journal description. This journal is a member of the Committee on Publication Ethics ...
SUBMIT PAPER. The Journal of Public Health Research is an online Open Access, peer-reviewed scholarly journal in the field of public health science. The aim of the journal is to stimulate debate and dissemination of knowledge in the public health field in order to improve … | View full journal description.
Advanced. Journal List. PubMed Central ® (PMC) is a free full-text archive of biomedical and life sciences journal literature at the U.S. National Institutes of Health's National Library of Medicine (NIH/NLM)
Recent years have seen a revolution in the domain of medical science, with ground-breaking discoveries changing health care as we once knew it [].These advances have considerably improved disease diagnosis, treatment, and management, improving patient outcomes and quality of life [2-5].These innovations range from the creation of novel medications and treatments to the utilization of cutting ...
Introduction. The evolution in the digital era has led to the confluence of healthcare and technology resulting in the emergence of newer data-related applications [].Due to the voluminous amounts of clinical data generated from the health care sector like the Electronic Health Records (EHR) of patients, prescriptions, clinical reports, information about the purchase of medicines, medical ...
Methods and findings. Two independent investigators systematically searched the Medical Literature Analysis and Retrieval System Online (MEDLINE), the Excerpta Medica Database (EMBASE), the Cumulative Index to Nursing and Allied Health Literature (CINAHL+), the Health Management Information Consortium, and the Journal of Research Evaluation from inception until May 2017 for publications that ...
Artificial intelligence (AI) is a powerful and disruptive area of computer science, with the potential to fundamentally transform the practice of medicine and the delivery of healthcare. In this review article, we outline recent breakthroughs in the application of AI in healthcare, describe a roadmap to building effective, reliable and safe AI ...
Health: An Interdisciplinary Journal for the Social Study of Health, Illness and Medicine. Impact Factor: 1.9 5-Year Impact Factor: 2.3. JOURNAL HOMEPAGE. Health: is published six times per year and attempts in each number to offer a mix of articles that inform or that provoke debate. The readership of the journal is wide and drawn from ...
Harvard Medical School has created a large department dedicated to developing and teaching young scientists about the emerging field of computational medicine. This field uses new mathematical techniques to make sense of the thousands of numbers generated in experiments measuring various molecules. Analyzing such "big data" was once unimaginable.
Health research entails systematic collection or analysis of data with the intent to develop generalizable knowledge to understand health challenges and mount an improved response to them. The full spectrum of health research spans five generic areas of activity: measuring the health problem; understanding its cause(s); elaborating solutions; translating the solutions or evidence into policy ...
Pam Belluck is a health and science reporter, covering a range of subjects, including reproductive health, long Covid, brain science, neurological disorders, mental health and genetics. More about ...
Racial and social-economic inequalities in systemic chemotherapy use among adult glioblastoma patients following surgery and radiotherapy. Fei Xu. , Xin Hua. & Weiqiong Ni. Article. 17 August 2024 ...
Finding and choosing a strong research topic is the critical first step when it comes to crafting a high-quality dissertation, thesis or research project. If you've landed on this post, chances are you're looking for a healthcare-related research topic, but aren't sure where to start. Here, we'll explore a variety of healthcare-related research ideas and topic thought-starters across a ...
Google Health research publications Publishing our work allows us to share ideas and work collaboratively to advance healthcare. This is a comprehensive view of our publications and associated blog posts. ... Annals of Eye Science 5, 18-18 (2020). Reviews Artificial intelligence in digital breast pathology: Techniques and applications
Medical research articles from across Nature Portfolio. Medical research involves research in a wide range of fields, such as biology, chemistry, pharmacology and toxicology with the goal of ...
However, ASD is also highly heritable (27-30) and therefore is expected to have a substantial contribution from common (27, 31) and rare variation transmitted from parents to their autistic offspring.Indeed, at least 50% of genetic risk is predicted to be due to common variation, 15 to 20% is due to de novo variation and other Mendelian forms, and the remaining genetic risk is yet to be ...
We have compiled a list of the top 5 research databases with a special focus on healthcare and medicine. Organize your papers in one place. Try Paperpile. No credit card needed. Get 30 days free. 1. PubMed. PubMed is the number one source for medical and healthcare research. It is hosted by the National Institutes of Health (NIH) and provides ...
While, on paper, mainland China has had a 75-year commitment to universal health coverage from 1949 to 2024, in practice, access to health insurance and care has been a privilege closely related to...
The Journal of Sport and Health Science (JSHS) is a peer-reviewed, international, multidisciplinary journal dedicated to the advancement of sport, exercise, physical activity, and health sciences. JSHS publishes original and impactful research, topical reviews, editorials, opinion, and commentary papers relating physical and mental health ...
The purpose of this paper is to present the Mayo Clinic Nursing Research (MCNR) model (Figure (Figure1)—a 1)—a model developed to guide and focus nursing science generation in a practice‐based setting with an emphasis on promoting the health and well‐being of patients and caregivers with complex needs. The components of the model are ...
This paper proposes a distributed solution based on blockchain technology for trusted Health Information Exchange (HIE). In addition to exchange of EHR between patient and doctor, the proposed system is also used in other aspects of healthcare such as improving the insurance claim and making data available for research organizations.
These big changes likely impact our health — the number of molecules related to cardiovascular disease showed significant changes at both time points, and those related to immune function changed in people in their early 60s. ... Although much research has focused on how different molecules increase or decrease as we age and how biological ...
A novel educational program for clinicians provided a foundation about climate change and the impact of fossil fuel-related pollution on individual health, and how healthcare systems contribute to ...