IEEE Account
- Change Username/Password
- Update Address

Purchase Details
- Payment Options
- Order History
- View Purchased Documents
Profile Information
- Communications Preferences
- Profession and Education
- Technical Interests
- US & Canada: +1 800 678 4333
- Worldwide: +1 732 981 0060
- Contact & Support
- About IEEE Xplore
- Accessibility
- Terms of Use
- Nondiscrimination Policy
- Privacy & Opting Out of Cookies
A not-for-profit organization, IEEE is the world's largest technical professional organization dedicated to advancing technology for the benefit of humanity. © Copyright 2024 IEEE - All rights reserved. Use of this web site signifies your agreement to the terms and conditions.
Advertisement
An automated essay scoring systems: a systematic literature review
- Published: 23 September 2021
- Volume 55 , pages 2495–2527, ( 2022 )
Cite this article
- Dadi Ramesh ORCID: orcid.org/0000-0002-3967-8914 1 , 2 &
- Suresh Kumar Sanampudi 3
42k Accesses
124 Citations
5 Altmetric
Explore all metrics
Assessment in the Education system plays a significant role in judging student performance. The present evaluation system is through human assessment. As the number of teachers' student ratio is gradually increasing, the manual evaluation process becomes complicated. The drawback of manual evaluation is that it is time-consuming, lacks reliability, and many more. This connection online examination system evolved as an alternative tool for pen and paper-based methods. Present Computer-based evaluation system works only for multiple-choice questions, but there is no proper evaluation system for grading essays and short answers. Many researchers are working on automated essay grading and short answer scoring for the last few decades, but assessing an essay by considering all parameters like the relevance of the content to the prompt, development of ideas, Cohesion, and Coherence is a big challenge till now. Few researchers focused on Content-based evaluation, while many of them addressed style-based assessment. This paper provides a systematic literature review on automated essay scoring systems. We studied the Artificial Intelligence and Machine Learning techniques used to evaluate automatic essay scoring and analyzed the limitations of the current studies and research trends. We observed that the essay evaluation is not done based on the relevance of the content and coherence.
Similar content being viewed by others
Automated Essay Scoring Systems
Automated Essay Scoring System Based on Rubric
Explore related subjects.
- Artificial Intelligence
Avoid common mistakes on your manuscript.
1 Introduction
Due to COVID 19 outbreak, an online educational system has become inevitable. In the present scenario, almost all the educational institutions ranging from schools to colleges adapt the online education system. The assessment plays a significant role in measuring the learning ability of the student. Most automated evaluation is available for multiple-choice questions, but assessing short and essay answers remain a challenge. The education system is changing its shift to online-mode, like conducting computer-based exams and automatic evaluation. It is a crucial application related to the education domain, which uses natural language processing (NLP) and Machine Learning techniques. The evaluation of essays is impossible with simple programming languages and simple techniques like pattern matching and language processing. Here the problem is for a single question, we will get more responses from students with a different explanation. So, we need to evaluate all the answers concerning the question.
Automated essay scoring (AES) is a computer-based assessment system that automatically scores or grades the student responses by considering appropriate features. The AES research started in 1966 with the Project Essay Grader (PEG) by Ajay et al. ( 1973 ). PEG evaluates the writing characteristics such as grammar, diction, construction, etc., to grade the essay. A modified version of the PEG by Shermis et al. ( 2001 ) was released, which focuses on grammar checking with a correlation between human evaluators and the system. Foltz et al. ( 1999 ) introduced an Intelligent Essay Assessor (IEA) by evaluating content using latent semantic analysis to produce an overall score. Powers et al. ( 2002 ) proposed E-rater and Intellimetric by Rudner et al. ( 2006 ) and Bayesian Essay Test Scoring System (BESTY) by Rudner and Liang ( 2002 ), these systems use natural language processing (NLP) techniques that focus on style and content to obtain the score of an essay. The vast majority of the essay scoring systems in the 1990s followed traditional approaches like pattern matching and a statistical-based approach. Since the last decade, the essay grading systems started using regression-based and natural language processing techniques. AES systems like Dong et al. ( 2017 ) and others developed from 2014 used deep learning techniques, inducing syntactic and semantic features resulting in better results than earlier systems.
Ohio, Utah, and most US states are using AES systems in school education, like Utah compose tool, Ohio standardized test (an updated version of PEG), evaluating millions of student's responses every year. These systems work for both formative, summative assessments and give feedback to students on the essay. Utah provided basic essay evaluation rubrics (six characteristics of essay writing): Development of ideas, organization, style, word choice, sentence fluency, conventions. Educational Testing Service (ETS) has been conducting significant research on AES for more than a decade and designed an algorithm to evaluate essays on different domains and providing an opportunity for test-takers to improve their writing skills. In addition, they are current research content-based evaluation.
The evaluation of essay and short answer scoring should consider the relevance of the content to the prompt, development of ideas, Cohesion, Coherence, and domain knowledge. Proper assessment of the parameters mentioned above defines the accuracy of the evaluation system. But all these parameters cannot play an equal role in essay scoring and short answer scoring. In a short answer evaluation, domain knowledge is required, like the meaning of "cell" in physics and biology is different. And while evaluating essays, the implementation of ideas with respect to prompt is required. The system should also assess the completeness of the responses and provide feedback.
Several studies examined AES systems, from the initial to the latest AES systems. In which the following studies on AES systems are Blood ( 2011 ) provided a literature review from PEG 1984–2010. Which has covered only generalized parts of AES systems like ethical aspects, the performance of the systems. Still, they have not covered the implementation part, and it’s not a comparative study and has not discussed the actual challenges of AES systems.
Burrows et al. ( 2015 ) Reviewed AES systems on six dimensions like dataset, NLP techniques, model building, grading models, evaluation, and effectiveness of the model. They have not covered feature extraction techniques and challenges in features extractions. Covered only Machine Learning models but not in detail. This system not covered the comparative analysis of AES systems like feature extraction, model building, and level of relevance, cohesion, and coherence not covered in this review.
Ke et al. ( 2019 ) provided a state of the art of AES system but covered very few papers and not listed all challenges, and no comparative study of the AES model. On the other hand, Hussein et al. in ( 2019 ) studied two categories of AES systems, four papers from handcrafted features for AES systems, and four papers from the neural networks approach, discussed few challenges, and did not cover feature extraction techniques, the performance of AES models in detail.
Klebanov et al. ( 2020 ). Reviewed 50 years of AES systems, listed and categorized all essential features that need to be extracted from essays. But not provided a comparative analysis of all work and not discussed the challenges.
This paper aims to provide a systematic literature review (SLR) on automated essay grading systems. An SLR is an Evidence-based systematic review to summarize the existing research. It critically evaluates and integrates all relevant studies' findings and addresses the research domain's specific research questions. Our research methodology uses guidelines given by Kitchenham et al. ( 2009 ) for conducting the review process; provide a well-defined approach to identify gaps in current research and to suggest further investigation.
We addressed our research method, research questions, and the selection process in Sect. 2 , and the results of the research questions have discussed in Sect. 3 . And the synthesis of all the research questions addressed in Sect. 4 . Conclusion and possible future work discussed in Sect. 5 .
2 Research method
We framed the research questions with PICOC criteria.
Population (P) Student essays and answers evaluation systems.
Intervention (I) evaluation techniques, data sets, features extraction methods.
Comparison (C) Comparison of various approaches and results.
Outcomes (O) Estimate the accuracy of AES systems,
Context (C) NA.
2.1 Research questions
To collect and provide research evidence from the available studies in the domain of automated essay grading, we framed the following research questions (RQ):
RQ1 what are the datasets available for research on automated essay grading?
The answer to the question can provide a list of the available datasets, their domain, and access to the datasets. It also provides a number of essays and corresponding prompts.
RQ2 what are the features extracted for the assessment of essays?
The answer to the question can provide an insight into various features so far extracted, and the libraries used to extract those features.
RQ3, which are the evaluation metrics available for measuring the accuracy of algorithms?
The answer will provide different evaluation metrics for accurate measurement of each Machine Learning approach and commonly used measurement technique.
RQ4 What are the Machine Learning techniques used for automatic essay grading, and how are they implemented?
It can provide insights into various Machine Learning techniques like regression models, classification models, and neural networks for implementing essay grading systems. The response to the question can give us different assessment approaches for automated essay grading systems.
RQ5 What are the challenges/limitations in the current research?
The answer to the question provides limitations of existing research approaches like cohesion, coherence, completeness, and feedback.
2.2 Search process
We conducted an automated search on well-known computer science repositories like ACL, ACM, IEEE Explore, Springer, and Science Direct for an SLR. We referred to papers published from 2010 to 2020 as much of the work during these years focused on advanced technologies like deep learning and natural language processing for automated essay grading systems. Also, the availability of free data sets like Kaggle (2012), Cambridge Learner Corpus-First Certificate in English exam (CLC-FCE) by Yannakoudakis et al. ( 2011 ) led to research this domain.
Search Strings : We used search strings like “Automated essay grading” OR “Automated essay scoring” OR “short answer scoring systems” OR “essay scoring systems” OR “automatic essay evaluation” and searched on metadata.
2.3 Selection criteria
After collecting all relevant documents from the repositories, we prepared selection criteria for inclusion and exclusion of documents. With the inclusion and exclusion criteria, it becomes more feasible for the research to be accurate and specific.
Inclusion criteria 1 Our approach is to work with datasets comprise of essays written in English. We excluded the essays written in other languages.
Inclusion criteria 2 We included the papers implemented on the AI approach and excluded the traditional methods for the review.
Inclusion criteria 3 The study is on essay scoring systems, so we exclusively included the research carried out on only text data sets rather than other datasets like image or speech.
Exclusion criteria We removed the papers in the form of review papers, survey papers, and state of the art papers.
2.4 Quality assessment
In addition to the inclusion and exclusion criteria, we assessed each paper by quality assessment questions to ensure the article's quality. We included the documents that have clearly explained the approach they used, the result analysis and validation.
The quality checklist questions are framed based on the guidelines from Kitchenham et al. ( 2009 ). Each quality assessment question was graded as either 1 or 0. The final score of the study range from 0 to 3. A cut off score for excluding a study from the review is 2 points. Since the papers scored 2 or 3 points are included in the final evaluation. We framed the following quality assessment questions for the final study.
Quality Assessment 1: Internal validity.
Quality Assessment 2: External validity.
Quality Assessment 3: Bias.
The two reviewers review each paper to select the final list of documents. We used the Quadratic Weighted Kappa score to measure the final agreement between the two reviewers. The average resulted from the kappa score is 0.6942, a substantial agreement between the reviewers. The result of evolution criteria shown in Table 1 . After Quality Assessment, the final list of papers for review is shown in Table 2 . The complete selection process is shown in Fig. 1 . The total number of selected papers in year wise as shown in Fig. 2 .
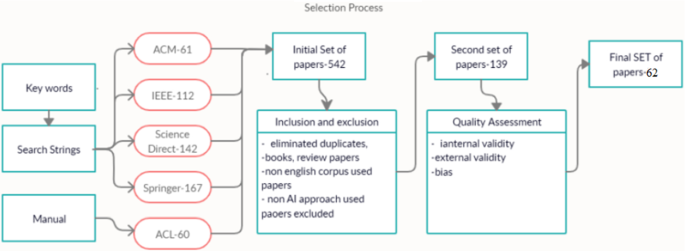
Selection process
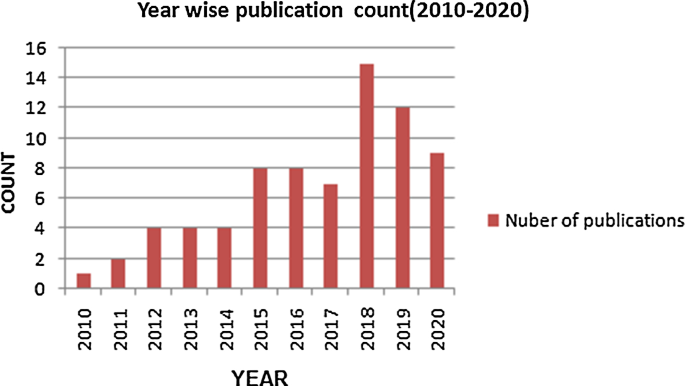
Year wise publications
3.1 What are the datasets available for research on automated essay grading?
To work with problem statement especially in Machine Learning and deep learning domain, we require considerable amount of data to train the models. To answer this question, we listed all the data sets used for training and testing for automated essay grading systems. The Cambridge Learner Corpus-First Certificate in English exam (CLC-FCE) Yannakoudakis et al. ( 2011 ) developed corpora that contain 1244 essays and ten prompts. This corpus evaluates whether a student can write the relevant English sentences without any grammatical and spelling mistakes. This type of corpus helps to test the models built for GRE and TOFEL type of exams. It gives scores between 1 and 40.
Bailey and Meurers ( 2008 ), Created a dataset (CREE reading comprehension) for language learners and automated short answer scoring systems. The corpus consists of 566 responses from intermediate students. Mohler and Mihalcea ( 2009 ). Created a dataset for the computer science domain consists of 630 responses for data structure assignment questions. The scores are range from 0 to 5 given by two human raters.
Dzikovska et al. ( 2012 ) created a Student Response Analysis (SRA) corpus. It consists of two sub-groups: the BEETLE corpus consists of 56 questions and approximately 3000 responses from students in the electrical and electronics domain. The second one is the SCIENTSBANK(SemEval-2013) (Dzikovska et al. 2013a ; b ) corpus consists of 10,000 responses on 197 prompts on various science domains. The student responses ladled with "correct, partially correct incomplete, Contradictory, Irrelevant, Non-domain."
In the Kaggle (2012) competition, released total 3 types of corpuses on an Automated Student Assessment Prize (ASAP1) (“ https://www.kaggle.com/c/asap-sas/ ” ) essays and short answers. It has nearly 17,450 essays, out of which it provides up to 3000 essays for each prompt. It has eight prompts that test 7th to 10th grade US students. It gives scores between the [0–3] and [0–60] range. The limitations of these corpora are: (1) it has a different score range for other prompts. (2) It uses statistical features such as named entities extraction and lexical features of words to evaluate essays. ASAP + + is one more dataset from Kaggle. It is with six prompts, and each prompt has more than 1000 responses total of 10,696 from 8th-grade students. Another corpus contains ten prompts from science, English domains and a total of 17,207 responses. Two human graders evaluated all these responses.
Correnti et al. ( 2013 ) created a Response-to-Text Assessment (RTA) dataset used to check student writing skills in all directions like style, mechanism, and organization. 4–8 grade students give the responses to RTA. Basu et al. ( 2013 ) created a power grading dataset with 700 responses for ten different prompts from US immigration exams. It contains all short answers for assessment.
The TOEFL11 corpus Blanchard et al. ( 2013 ) contains 1100 essays evenly distributed over eight prompts. It is used to test the English language skills of a candidate attending the TOFEL exam. It scores the language proficiency of a candidate as low, medium, and high.
International Corpus of Learner English (ICLE) Granger et al. ( 2009 ) built a corpus of 3663 essays covering different dimensions. It has 12 prompts with 1003 essays that test the organizational skill of essay writing, and13 prompts, each with 830 essays that examine the thesis clarity and prompt adherence.
Argument Annotated Essays (AAE) Stab and Gurevych ( 2014 ) developed a corpus that contains 102 essays with 101 prompts taken from the essayforum2 site. It tests the persuasive nature of the student essay. The SCIENTSBANK corpus used by Sakaguchi et al. ( 2015 ) available in git-hub, containing 9804 answers to 197 questions in 15 science domains. Table 3 illustrates all datasets related to AES systems.
3.2 RQ2 what are the features extracted for the assessment of essays?
Features play a major role in the neural network and other supervised Machine Learning approaches. The automatic essay grading systems scores student essays based on different types of features, which play a prominent role in training the models. Based on their syntax and semantics and they are categorized into three groups. 1. statistical-based features Contreras et al. ( 2018 ); Kumar et al. ( 2019 ); Mathias and Bhattacharyya ( 2018a ; b ) 2. Style-based (Syntax) features Cummins et al. ( 2016 ); Darwish and Mohamed ( 2020 ); Ke et al. ( 2019 ). 3. Content-based features Dong et al. ( 2017 ). A good set of features appropriate models evolved better AES systems. The vast majority of the researchers are using regression models if features are statistical-based. For Neural Networks models, researches are using both style-based and content-based features. The following table shows the list of various features used in existing AES Systems. Table 4 represents all set of features used for essay grading.
We studied all the feature extracting NLP libraries as shown in Fig. 3 . that are used in the papers. The NLTK is an NLP tool used to retrieve statistical features like POS, word count, sentence count, etc. With NLTK, we can miss the essay's semantic features. To find semantic features Word2Vec Mikolov et al. ( 2013 ), GloVe Jeffrey Pennington et al. ( 2014 ) is the most used libraries to retrieve the semantic text from the essays. And in some systems, they directly trained the model with word embeddings to find the score. From Fig. 4 as observed that non-content-based feature extraction is higher than content-based.
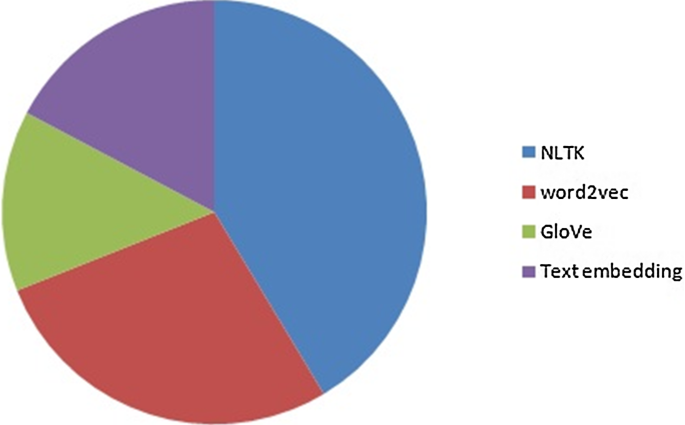
Usages of tools
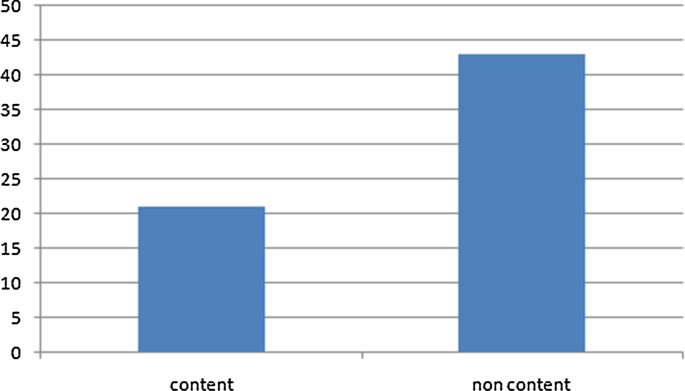
Number of papers on content based features
3.3 RQ3 which are the evaluation metrics available for measuring the accuracy of algorithms?
The majority of the AES systems are using three evaluation metrics. They are (1) quadrated weighted kappa (QWK) (2) Mean Absolute Error (MAE) (3) Pearson Correlation Coefficient (PCC) Shehab et al. ( 2016 ). The quadratic weighted kappa will find agreement between human evaluation score and system evaluation score and produces value ranging from 0 to 1. And the Mean Absolute Error is the actual difference between human-rated score to system-generated score. The mean square error (MSE) measures the average squares of the errors, i.e., the average squared difference between the human-rated and the system-generated scores. MSE will always give positive numbers only. Pearson's Correlation Coefficient (PCC) finds the correlation coefficient between two variables. It will provide three values (0, 1, − 1). "0" represents human-rated and system scores that are not related. "1" represents an increase in the two scores. "− 1" illustrates a negative relationship between the two scores.
3.4 RQ4 what are the Machine Learning techniques being used for automatic essay grading, and how are they implemented?
After scrutinizing all documents, we categorize the techniques used in automated essay grading systems into four baskets. 1. Regression techniques. 2. Classification model. 3. Neural networks. 4. Ontology-based approach.
All the existing AES systems developed in the last ten years employ supervised learning techniques. Researchers using supervised methods viewed the AES system as either regression or classification task. The goal of the regression task is to predict the score of an essay. The classification task is to classify the essays belonging to (low, medium, or highly) relevant to the question's topic. Since the last three years, most AES systems developed made use of the concept of the neural network.
3.4.1 Regression based models
Mohler and Mihalcea ( 2009 ). proposed text-to-text semantic similarity to assign a score to the student essays. There are two text similarity measures like Knowledge-based measures, corpus-based measures. There eight knowledge-based tests with all eight models. They found the similarity. The shortest path similarity determines based on the length, which shortest path between two contexts. Leacock & Chodorow find the similarity based on the shortest path's length between two concepts using node-counting. The Lesk similarity finds the overlap between the corresponding definitions, and Wu & Palmer algorithm finds similarities based on the depth of two given concepts in the wordnet taxonomy. Resnik, Lin, Jiang&Conrath, Hirst& St-Onge find the similarity based on different parameters like the concept, probability, normalization factor, lexical chains. In corpus-based likeness, there LSA BNC, LSA Wikipedia, and ESA Wikipedia, latent semantic analysis is trained on Wikipedia and has excellent domain knowledge. Among all similarity scores, correlation scores LSA Wikipedia scoring accuracy is more. But these similarity measure algorithms are not using NLP concepts. These models are before 2010 and basic concept models to continue the research automated essay grading with updated algorithms on neural networks with content-based features.
Adamson et al. ( 2014 ) proposed an automatic essay grading system which is a statistical-based approach in this they retrieved features like POS, Character count, Word count, Sentence count, Miss spelled words, n-gram representation of words to prepare essay vector. They formed a matrix with these all vectors in that they applied LSA to give a score to each essay. It is a statistical approach that doesn’t consider the semantics of the essay. The accuracy they got when compared to the human rater score with the system is 0.532.
Cummins et al. ( 2016 ). Proposed Timed Aggregate Perceptron vector model to give ranking to all the essays, and later they converted the rank algorithm to predict the score of the essay. The model trained with features like Word unigrams, bigrams, POS, Essay length, grammatical relation, Max word length, sentence length. It is multi-task learning, gives ranking to the essays, and predicts the score for the essay. The performance evaluated through QWK is 0.69, a substantial agreement between the human rater and the system.
Sultan et al. ( 2016 ). Proposed a Ridge regression model to find short answer scoring with Question Demoting. Question Demoting is the new concept included in the essay's final assessment to eliminate duplicate words from the essay. The extracted features are Text Similarity, which is the similarity between the student response and reference answer. Question Demoting is the number of repeats in a student response. With inverse document frequency, they assigned term weight. The sentence length Ratio is the number of words in the student response, is another feature. With these features, the Ridge regression model was used, and the accuracy they got 0.887.
Contreras et al. ( 2018 ). Proposed Ontology based on text mining in this model has given a score for essays in phases. In phase-I, they generated ontologies with ontoGen and SVM to find the concept and similarity in the essay. In phase II from ontologies, they retrieved features like essay length, word counts, correctness, vocabulary, and types of word used, domain information. After retrieving statistical data, they used a linear regression model to find the score of the essay. The accuracy score is the average of 0.5.
Darwish and Mohamed ( 2020 ) proposed the fusion of fuzzy Ontology with LSA. They retrieve two types of features, like syntax features and semantic features. In syntax features, they found Lexical Analysis with tokens, and they construct a parse tree. If the parse tree is broken, the essay is inconsistent—a separate grade assigned to the essay concerning syntax features. The semantic features are like similarity analysis, Spatial Data Analysis. Similarity analysis is to find duplicate sentences—Spatial Data Analysis for finding Euclid distance between the center and part. Later they combine syntax features and morphological features score for the final score. The accuracy they achieved with the multiple linear regression model is 0.77, mostly on statistical features.
Süzen Neslihan et al. ( 2020 ) proposed a text mining approach for short answer grading. First, their comparing model answers with student response by calculating the distance between two sentences. By comparing the model answer with student response, they find the essay's completeness and provide feedback. In this approach, model vocabulary plays a vital role in grading, and with this model vocabulary, the grade will be assigned to the student's response and provides feedback. The correlation between the student answer to model answer is 0.81.
3.4.2 Classification based Models
Persing and Ng ( 2013 ) used a support vector machine to score the essay. The features extracted are OS, N-gram, and semantic text to train the model and identified the keywords from the essay to give the final score.
Sakaguchi et al. ( 2015 ) proposed two methods: response-based and reference-based. In response-based scoring, the extracted features are response length, n-gram model, and syntactic elements to train the support vector regression model. In reference-based scoring, features such as sentence similarity using word2vec is used to find the cosine similarity of the sentences that is the final score of the response. First, the scores were discovered individually and later combined two features to find a final score. This system gave a remarkable increase in performance by combining the scores.
Mathias and Bhattacharyya ( 2018a ; b ) Proposed Automated Essay Grading Dataset with Essay Attribute Scores. The first concept features selection depends on the essay type. So the common attributes are Content, Organization, Word Choice, Sentence Fluency, Conventions. In this system, each attribute is scored individually, with the strength of each attribute identified. The model they used is a random forest classifier to assign scores to individual attributes. The accuracy they got with QWK is 0.74 for prompt 1 of the ASAS dataset ( https://www.kaggle.com/c/asap-sas/ ).
Ke et al. ( 2019 ) used a support vector machine to find the response score. In this method, features like Agreeability, Specificity, Clarity, Relevance to prompt, Conciseness, Eloquence, Confidence, Direction of development, Justification of opinion, and Justification of importance. First, the individual parameter score obtained was later combined with all scores to give a final response score. The features are used in the neural network to find whether the sentence is relevant to the topic or not.
Salim et al. ( 2019 ) proposed an XGBoost Machine Learning classifier to assess the essays. The algorithm trained on features like word count, POS, parse tree depth, and coherence in the articles with sentence similarity percentage; cohesion and coherence are considered for training. And they implemented K-fold cross-validation for a result the average accuracy after specific validations is 68.12.
3.4.3 Neural network models
Shehab et al. ( 2016 ) proposed a neural network method that used learning vector quantization to train human scored essays. After training, the network can provide a score to the ungraded essays. First, we should process the essay to remove Spell checking and then perform preprocessing steps like Document Tokenization, stop word removal, Stemming, and submit it to the neural network. Finally, the model will provide feedback on the essay, whether it is relevant to the topic. And the correlation coefficient between human rater and system score is 0.7665.
Kopparapu and De ( 2016 ) proposed the Automatic Ranking of Essays using Structural and Semantic Features. This approach constructed a super essay with all the responses. Next, ranking for a student essay is done based on the super-essay. The structural and semantic features derived helps to obtain the scores. In a paragraph, 15 Structural features like an average number of sentences, the average length of sentences, and the count of words, nouns, verbs, adjectives, etc., are used to obtain a syntactic score. A similarity score is used as semantic features to calculate the overall score.
Dong and Zhang ( 2016 ) proposed a hierarchical CNN model. The model builds two layers with word embedding to represents the words as the first layer. The second layer is a word convolution layer with max-pooling to find word vectors. The next layer is a sentence-level convolution layer with max-pooling to find the sentence's content and synonyms. A fully connected dense layer produces an output score for an essay. The accuracy with the hierarchical CNN model resulted in an average QWK of 0.754.
Taghipour and Ng ( 2016 ) proposed a first neural approach for essay scoring build in which convolution and recurrent neural network concepts help in scoring an essay. The network uses a lookup table with the one-hot representation of the word vector of an essay. The final efficiency of the network model with LSTM resulted in an average QWK of 0.708.
Dong et al. ( 2017 ). Proposed an Attention-based scoring system with CNN + LSTM to score an essay. For CNN, the input parameters were character embedding and word embedding, and it has attention pooling layers and used NLTK to obtain word and character embedding. The output gives a sentence vector, which provides sentence weight. After CNN, it will have an LSTM layer with an attention pooling layer, and this final layer results in the final score of the responses. The average QWK score is 0.764.
Riordan et al. ( 2017 ) proposed a neural network with CNN and LSTM layers. Word embedding, given as input to a neural network. An LSTM network layer will retrieve the window features and delivers them to the aggregation layer. The aggregation layer is a superficial layer that takes a correct window of words and gives successive layers to predict the answer's sore. The accuracy of the neural network resulted in a QWK of 0.90.
Zhao et al. ( 2017 ) proposed a new concept called Memory-Augmented Neural network with four layers, input representation layer, memory addressing layer, memory reading layer, and output layer. An input layer represents all essays in a vector form based on essay length. After converting the word vector, the memory addressing layer takes a sample of the essay and weighs all the terms. The memory reading layer takes the input from memory addressing segment and finds the content to finalize the score. Finally, the output layer will provide the final score of the essay. The accuracy of essay scores is 0.78, which is far better than the LSTM neural network.
Mathias and Bhattacharyya ( 2018a ; b ) proposed deep learning networks using LSTM with the CNN layer and GloVe pre-trained word embeddings. For this, they retrieved features like Sentence count essays, word count per sentence, Number of OOVs in the sentence, Language model score, and the text's perplexity. The network predicted the goodness scores of each essay. The higher the goodness scores, means higher the rank and vice versa.
Nguyen and Dery ( 2016 ). Proposed Neural Networks for Automated Essay Grading. In this method, a single layer bi-directional LSTM accepting word vector as input. Glove vectors used in this method resulted in an accuracy of 90%.
Ruseti et al. ( 2018 ) proposed a recurrent neural network that is capable of memorizing the text and generate a summary of an essay. The Bi-GRU network with the max-pooling layer molded on the word embedding of each document. It will provide scoring to the essay by comparing it with a summary of the essay from another Bi-GRU network. The result obtained an accuracy of 0.55.
Wang et al. ( 2018a ; b ) proposed an automatic scoring system with the bi-LSTM recurrent neural network model and retrieved the features using the word2vec technique. This method generated word embeddings from the essay words using the skip-gram model. And later, word embedding is used to train the neural network to find the final score. The softmax layer in LSTM obtains the importance of each word. This method used a QWK score of 0.83%.
Dasgupta et al. ( 2018 ) proposed a technique for essay scoring with augmenting textual qualitative Features. It extracted three types of linguistic, cognitive, and psychological features associated with a text document. The linguistic features are Part of Speech (POS), Universal Dependency relations, Structural Well-formedness, Lexical Diversity, Sentence Cohesion, Causality, and Informativeness of the text. The psychological features derived from the Linguistic Information and Word Count (LIWC) tool. They implemented a convolution recurrent neural network that takes input as word embedding and sentence vector, retrieved from the GloVe word vector. And the second layer is the Convolution Layer to find local features. The next layer is the recurrent neural network (LSTM) to find corresponding of the text. The accuracy of this method resulted in an average QWK of 0.764.
Liang et al. ( 2018 ) proposed a symmetrical neural network AES model with Bi-LSTM. They are extracting features from sample essays and student essays and preparing an embedding layer as input. The embedding layer output is transfer to the convolution layer from that LSTM will be trained. Hear the LSRM model has self-features extraction layer, which will find the essay's coherence. The average QWK score of SBLSTMA is 0.801.
Liu et al. ( 2019 ) proposed two-stage learning. In the first stage, they are assigning a score based on semantic data from the essay. The second stage scoring is based on some handcrafted features like grammar correction, essay length, number of sentences, etc. The average score of the two stages is 0.709.
Pedro Uria Rodriguez et al. ( 2019 ) proposed a sequence-to-sequence learning model for automatic essay scoring. They used BERT (Bidirectional Encoder Representations from Transformers), which extracts the semantics from a sentence from both directions. And XLnet sequence to sequence learning model to extract features like the next sentence in an essay. With this pre-trained model, they attained coherence from the essay to give the final score. The average QWK score of the model is 75.5.
Xia et al. ( 2019 ) proposed a two-layer Bi-directional LSTM neural network for the scoring of essays. The features extracted with word2vec to train the LSTM and accuracy of the model in an average of QWK is 0.870.
Kumar et al. ( 2019 ) Proposed an AutoSAS for short answer scoring. It used pre-trained Word2Vec and Doc2Vec models trained on Google News corpus and Wikipedia dump, respectively, to retrieve the features. First, they tagged every word POS and they found weighted words from the response. It also found prompt overlap to observe how the answer is relevant to the topic, and they defined lexical overlaps like noun overlap, argument overlap, and content overlap. This method used some statistical features like word frequency, difficulty, diversity, number of unique words in each response, type-token ratio, statistics of the sentence, word length, and logical operator-based features. This method uses a random forest model to train the dataset. The data set has sample responses with their associated score. The model will retrieve the features from both responses like graded and ungraded short answers with questions. The accuracy of AutoSAS with QWK is 0.78. It will work on any topics like Science, Arts, Biology, and English.
Jiaqi Lun et al. ( 2020 ) proposed an automatic short answer scoring with BERT. In this with a reference answer comparing student responses and assigning scores. The data augmentation is done with a neural network and with one correct answer from the dataset classifying reaming responses as correct or incorrect.
Zhu and Sun ( 2020 ) proposed a multimodal Machine Learning approach for automated essay scoring. First, they count the grammar score with the spaCy library and numerical count as the number of words and sentences with the same library. With this input, they trained a single and Bi LSTM neural network for finding the final score. For the LSTM model, they prepared sentence vectors with GloVe and word embedding with NLTK. Bi-LSTM will check each sentence in both directions to find semantic from the essay. The average QWK score with multiple models is 0.70.
3.4.4 Ontology based approach
Mohler et al. ( 2011 ) proposed a graph-based method to find semantic similarity in short answer scoring. For the ranking of answers, they used the support vector regression model. The bag of words is the main feature extracted in the system.
Ramachandran et al. ( 2015 ) also proposed a graph-based approach to find lexical based semantics. Identified phrase patterns and text patterns are the features to train a random forest regression model to score the essays. The accuracy of the model in a QWK is 0.78.
Zupanc et al. ( 2017 ) proposed sentence similarity networks to find the essay's score. Ajetunmobi and Daramola ( 2017 ) recommended an ontology-based information extraction approach and domain-based ontology to find the score.
3.4.5 Speech response scoring
Automatic scoring is in two ways one is text-based scoring, other is speech-based scoring. This paper discussed text-based scoring and its challenges, and now we cover speech scoring and common points between text and speech-based scoring. Evanini and Wang ( 2013 ), Worked on speech scoring of non-native school students, extracted features with speech ratter, and trained a linear regression model, concluding that accuracy varies based on voice pitching. Loukina et al. ( 2015 ) worked on feature selection from speech data and trained SVM. Malinin et al. ( 2016 ) used neural network models to train the data. Loukina et al. ( 2017 ). Proposed speech and text-based automatic scoring. Extracted text-based features, speech-based features and trained a deep neural network for speech-based scoring. They extracted 33 types of features based on acoustic signals. Malinin et al. ( 2017 ). Wu Xixin et al. ( 2020 ) Worked on deep neural networks for spoken language assessment. Incorporated different types of models and tested them. Ramanarayanan et al. ( 2017 ) worked on feature extraction methods and extracted punctuation, fluency, and stress and trained different Machine Learning models for scoring. Knill et al. ( 2018 ). Worked on Automatic speech recognizer and its errors how its impacts the speech assessment.
3.4.5.1 The state of the art
This section provides an overview of the existing AES systems with a comparative study w. r. t models, features applied, datasets, and evaluation metrics used for building the automated essay grading systems. We divided all 62 papers into two sets of the first set of review papers in Table 5 with a comparative study of the AES systems.
3.4.6 Comparison of all approaches
In our study, we divided major AES approaches into three categories. Regression models, classification models, and neural network models. The regression models failed to find cohesion and coherence from the essay because it trained on BoW(Bag of Words) features. In processing data from input to output, the regression models are less complicated than neural networks. There are unable to find many intricate patterns from the essay and unable to find sentence connectivity. If we train the model with BoW features in the neural network approach, the model never considers the essay's coherence and coherence.
First, to train a Machine Learning algorithm with essays, all the essays are converted to vector form. We can form a vector with BoW and Word2vec, TF-IDF. The BoW and Word2vec vector representation of essays represented in Table 6 . The vector representation of BoW with TF-IDF is not incorporating the essays semantic, and it’s just statistical learning from a given vector. Word2vec vector comprises semantic of essay in a unidirectional way.
In BoW, the vector contains the frequency of word occurrences in the essay. The vector represents 1 and more based on the happenings of words in the essay and 0 for not present. So, in BoW, the vector does not maintain the relationship with adjacent words; it’s just for single words. In word2vec, the vector represents the relationship between words with other words and sentences prompt in multiple dimensional ways. But word2vec prepares vectors in a unidirectional way, not in a bidirectional way; word2vec fails to find semantic vectors when a word has two meanings, and the meaning depends on adjacent words. Table 7 represents a comparison of Machine Learning models and features extracting methods.
In AES, cohesion and coherence will check the content of the essay concerning the essay prompt these can be extracted from essay in the vector from. Two more parameters are there to access an essay is completeness and feedback. Completeness will check whether student’s response is sufficient or not though the student wrote correctly. Table 8 represents all four parameters comparison for essay grading. Table 9 illustrates comparison of all approaches based on various features like grammar, spelling, organization of essay, relevance.
3.5 What are the challenges/limitations in the current research?
From our study and results discussed in the previous sections, many researchers worked on automated essay scoring systems with numerous techniques. We have statistical methods, classification methods, and neural network approaches to evaluate the essay automatically. The main goal of the automated essay grading system is to reduce human effort and improve consistency.
The vast majority of essay scoring systems are dealing with the efficiency of the algorithm. But there are many challenges in automated essay grading systems. One should assess the essay by following parameters like the relevance of the content to the prompt, development of ideas, Cohesion, Coherence, and domain knowledge.
No model works on the relevance of content, which means whether student response or explanation is relevant to the given prompt or not if it is relevant to how much it is appropriate, and there is no discussion about the cohesion and coherence of the essays. All researches concentrated on extracting the features using some NLP libraries, trained their models, and testing the results. But there is no explanation in the essay evaluation system about consistency and completeness, But Palma and Atkinson ( 2018 ) explained coherence-based essay evaluation. And Zupanc and Bosnic ( 2014 ) also used the word coherence to evaluate essays. And they found consistency with latent semantic analysis (LSA) for finding coherence from essays, but the dictionary meaning of coherence is "The quality of being logical and consistent."
Another limitation is there is no domain knowledge-based evaluation of essays using Machine Learning models. For example, the meaning of a cell is different from biology to physics. Many Machine Learning models extract features with WordVec and GloVec; these NLP libraries cannot convert the words into vectors when they have two or more meanings.
3.5.1 Other challenges that influence the Automated Essay Scoring Systems.
All these approaches worked to improve the QWK score of their models. But QWK will not assess the model in terms of features extraction and constructed irrelevant answers. The QWK is not evaluating models whether the model is correctly assessing the answer or not. There are many challenges concerning students' responses to the Automatic scoring system. Like in evaluating approach, no model has examined how to evaluate the constructed irrelevant and adversarial answers. Especially the black box type of approaches like deep learning models provides more options to the students to bluff the automated scoring systems.
The Machine Learning models that work on statistical features are very vulnerable. Based on Powers et al. ( 2001 ) and Bejar Isaac et al. ( 2014 ), the E-rater was failed on Constructed Irrelevant Responses Strategy (CIRS). From the study of Bejar et al. ( 2013 ), Higgins and Heilman ( 2014 ), observed that when student response contain irrelevant content or shell language concurring to prompt will influence the final score of essays in an automated scoring system.
In deep learning approaches, most of the models automatically read the essay's features, and some methods work on word-based embedding and other character-based embedding features. From the study of Riordan Brain et al. ( 2019 ), The character-based embedding systems do not prioritize spelling correction. However, it is influencing the final score of the essay. From the study of Horbach and Zesch ( 2019 ), Various factors are influencing AES systems. For example, there are data set size, prompt type, answer length, training set, and human scorers for content-based scoring.
Ding et al. ( 2020 ) reviewed that the automated scoring system is vulnerable when a student response contains more words from prompt, like prompt vocabulary repeated in the response. Parekh et al. ( 2020 ) and Kumar et al. ( 2020 ) tested various neural network models of AES by iteratively adding important words, deleting unimportant words, shuffle the words, and repeating sentences in an essay and found that no change in the final score of essays. These neural network models failed to recognize common sense in adversaries' essays and give more options for the students to bluff the automated systems.
Other than NLP and ML techniques for AES. From Wresch ( 1993 ) to Madnani and Cahill ( 2018 ). discussed the complexity of AES systems, standards need to be followed. Like assessment rubrics to test subject knowledge, irrelevant responses, and ethical aspects of an algorithm like measuring the fairness of student response.
Fairness is an essential factor for automated systems. For example, in AES, fairness can be measure in an agreement between human score to machine score. Besides this, From Loukina et al. ( 2019 ), the fairness standards include overall score accuracy, overall score differences, and condition score differences between human and system scores. In addition, scoring different responses in the prospect of constructive relevant and irrelevant will improve fairness.
Madnani et al. ( 2017a ; b ). Discussed the fairness of AES systems for constructed responses and presented RMS open-source tool for detecting biases in the models. With this, one can change fairness standards according to their analysis of fairness.
From Berzak et al.'s ( 2018 ) approach, behavior factors are a significant challenge in automated scoring systems. That helps to find language proficiency, word characteristics (essential words from the text), predict the critical patterns from the text, find related sentences in an essay, and give a more accurate score.
Rupp ( 2018 ), has discussed the designing, evaluating, and deployment methodologies for AES systems. They provided notable characteristics of AES systems for deployment. They are like model performance, evaluation metrics for a model, threshold values, dynamically updated models, and framework.
First, we should check the model performance on different datasets and parameters for operational deployment. Selecting Evaluation metrics for AES models are like QWK, correlation coefficient, or sometimes both. Kelley and Preacher ( 2012 ) have discussed three categories of threshold values: marginal, borderline, and acceptable. The values can be varied based on data size, model performance, type of model (single scoring, multiple scoring models). Once a model is deployed and evaluates millions of responses every time for optimal responses, we need a dynamically updated model based on prompt and data. Finally, framework designing of AES model, hear a framework contains prompts where test-takers can write the responses. One can design two frameworks: a single scoring model for a single methodology and multiple scoring models for multiple concepts. When we deploy multiple scoring models, each prompt could be trained separately, or we can provide generalized models for all prompts with this accuracy may vary, and it is challenging.
4 Synthesis
Our Systematic literature review on the automated essay grading system first collected 542 papers with selected keywords from various databases. After inclusion and exclusion criteria, we left with 139 articles; on these selected papers, we applied Quality assessment criteria with two reviewers, and finally, we selected 62 writings for final review.
Our observations on automated essay grading systems from 2010 to 2020 are as followed:
The implementation techniques of automated essay grading systems are classified into four buckets; there are 1. regression models 2. Classification models 3. Neural networks 4. Ontology-based methodology, but using neural networks, the researchers are more accurate than other techniques, and all the methods state of the art provided in Table 3 .
The majority of the regression and classification models on essay scoring used statistical features to find the final score. It means the systems or models trained on such parameters as word count, sentence count, etc. though the parameters extracted from the essay, the algorithm are not directly training on essays. The algorithms trained on some numbers obtained from the essay and hear if numbers matched the composition will get a good score; otherwise, the rating is less. In these models, the evaluation process is entirely on numbers, irrespective of the essay. So, there is a lot of chance to miss the coherence, relevance of the essay if we train our algorithm on statistical parameters.
In the neural network approach, the models trained on Bag of Words (BoW) features. The BoW feature is missing the relationship between a word to word and the semantic meaning of the sentence. E.g., Sentence 1: John killed bob. Sentence 2: bob killed John. In these two sentences, the BoW is "John," "killed," "bob."
In the Word2Vec library, if we are prepared a word vector from an essay in a unidirectional way, the vector will have a dependency with other words and finds the semantic relationship with other words. But if a word has two or more meanings like "Bank loan" and "River Bank," hear bank has two implications, and its adjacent words decide the sentence meaning; in this case, Word2Vec is not finding the real meaning of the word from the sentence.
The features extracted from essays in the essay scoring system are classified into 3 type's features like statistical features, style-based features, and content-based features, which are explained in RQ2 and Table 3 . But statistical features, are playing a significant role in some systems and negligible in some systems. In Shehab et al. ( 2016 ); Cummins et al. ( 2016 ). Dong et al. ( 2017 ). Dong and Zhang ( 2016 ). Mathias and Bhattacharyya ( 2018a ; b ) Systems the assessment is entirely on statistical and style-based features they have not retrieved any content-based features. And in other systems that extract content from the essays, the role of statistical features is for only preprocessing essays but not included in the final grading.
In AES systems, coherence is the main feature to be considered while evaluating essays. The actual meaning of coherence is to stick together. That is the logical connection of sentences (local level coherence) and paragraphs (global level coherence) in a story. Without coherence, all sentences in a paragraph are independent and meaningless. In an Essay, coherence is a significant feature that is explaining everything in a flow and its meaning. It is a powerful feature in AES system to find the semantics of essay. With coherence, one can assess whether all sentences are connected in a flow and all paragraphs are related to justify the prompt. Retrieving the coherence level from an essay is a critical task for all researchers in AES systems.
In automatic essay grading systems, the assessment of essays concerning content is critical. That will give the actual score for the student. Most of the researches used statistical features like sentence length, word count, number of sentences, etc. But according to collected results, 32% of the systems used content-based features for the essay scoring. Example papers which are on content-based assessment are Taghipour and Ng ( 2016 ); Persing and Ng ( 2013 ); Wang et al. ( 2018a , 2018b ); Zhao et al. ( 2017 ); Kopparapu and De ( 2016 ), Kumar et al. ( 2019 ); Mathias and Bhattacharyya ( 2018a ; b ); Mohler and Mihalcea ( 2009 ) are used content and statistical-based features. The results are shown in Fig. 3 . And mainly the content-based features extracted with word2vec NLP library, but word2vec is capable of capturing the context of a word in a document, semantic and syntactic similarity, relation with other terms, but word2vec is capable of capturing the context word in a uni-direction either left or right. If a word has multiple meanings, there is a chance of missing the context in the essay. After analyzing all the papers, we found that content-based assessment is a qualitative assessment of essays.
On the other hand, Horbach and Zesch ( 2019 ); Riordan Brain et al. ( 2019 ); Ding et al. ( 2020 ); Kumar et al. ( 2020 ) proved that neural network models are vulnerable when a student response contains constructed irrelevant, adversarial answers. And a student can easily bluff an automated scoring system by submitting different responses like repeating sentences and repeating prompt words in an essay. From Loukina et al. ( 2019 ), and Madnani et al. ( 2017b ). The fairness of an algorithm is an essential factor to be considered in AES systems.
While talking about speech assessment, the data set contains audios of duration up to one minute. Feature extraction techniques are entirely different from text assessment, and accuracy varies based on speaking fluency, pitching, male to female voice and boy to adult voice. But the training algorithms are the same for text and speech assessment.
Once an AES system evaluates essays and short answers accurately in all directions, there is a massive demand for automated systems in the educational and related world. Now AES systems are deployed in GRE, TOEFL exams; other than these, we can deploy AES systems in massive open online courses like Coursera(“ https://coursera.org/learn//machine-learning//exam ”), NPTEL ( https://swayam.gov.in/explorer ), etc. still they are assessing student performance with multiple-choice questions. In another perspective, AES systems can be deployed in information retrieval systems like Quora, stack overflow, etc., to check whether the retrieved response is appropriate to the question or not and can give ranking to the retrieved answers.
5 Conclusion and future work
As per our Systematic literature review, we studied 62 papers. There exist significant challenges for researchers in implementing automated essay grading systems. Several researchers are working rigorously on building a robust AES system despite its difficulty in solving this problem. All evaluating methods are not evaluated based on coherence, relevance, completeness, feedback, and knowledge-based. And 90% of essay grading systems are used Kaggle ASAP (2012) dataset, which has general essays from students and not required any domain knowledge, so there is a need for domain-specific essay datasets to train and test. Feature extraction is with NLTK, WordVec, and GloVec NLP libraries; these libraries have many limitations while converting a sentence into vector form. Apart from feature extraction and training Machine Learning models, no system is accessing the essay's completeness. No system provides feedback to the student response and not retrieving coherence vectors from the essay—another perspective the constructive irrelevant and adversarial student responses still questioning AES systems.
Our proposed research work will go on the content-based assessment of essays with domain knowledge and find a score for the essays with internal and external consistency. And we will create a new dataset concerning one domain. And another area in which we can improve is the feature extraction techniques.
This study includes only four digital databases for study selection may miss some functional studies on the topic. However, we hope that we covered most of the significant studies as we manually collected some papers published in useful journals.
Adamson, A., Lamb, A., & December, R. M. (2014). Automated Essay Grading.
Ajay HB, Tillett PI, Page EB (1973) Analysis of essays by computer (AEC-II) (No. 8-0102). Washington, DC: U.S. Department of Health, Education, and Welfare, Office of Education, National Center for Educational Research and Development
Ajetunmobi SA, Daramola O (2017) Ontology-based information extraction for subject-focussed automatic essay evaluation. In: 2017 International Conference on Computing Networking and Informatics (ICCNI) p 1–6. IEEE
Alva-Manchego F, et al. (2019) EASSE: Easier Automatic Sentence Simplification Evaluation.” ArXiv abs/1908.04567 (2019): n. pag
Bailey S, Meurers D (2008) Diagnosing meaning errors in short answers to reading comprehension questions. In: Proceedings of the Third Workshop on Innovative Use of NLP for Building Educational Applications (Columbus), p 107–115
Basu S, Jacobs C, Vanderwende L (2013) Powergrading: a clustering approach to amplify human effort for short answer grading. Trans Assoc Comput Linguist (TACL) 1:391–402
Article Google Scholar
Bejar, I. I., Flor, M., Futagi, Y., & Ramineni, C. (2014). On the vulnerability of automated scoring to construct-irrelevant response strategies (CIRS): An illustration. Assessing Writing, 22, 48-59.
Bejar I, et al. (2013) Length of Textual Response as a Construct-Irrelevant Response Strategy: The Case of Shell Language. Research Report. ETS RR-13-07.” ETS Research Report Series (2013): n. pag
Berzak Y, et al. (2018) “Assessing Language Proficiency from Eye Movements in Reading.” ArXiv abs/1804.07329 (2018): n. pag
Blanchard D, Tetreault J, Higgins D, Cahill A, Chodorow M (2013) TOEFL11: A corpus of non-native English. ETS Research Report Series, 2013(2):i–15, 2013
Blood, I. (2011). Automated essay scoring: a literature review. Studies in Applied Linguistics and TESOL, 11(2).
Burrows S, Gurevych I, Stein B (2015) The eras and trends of automatic short answer grading. Int J Artif Intell Educ 25:60–117. https://doi.org/10.1007/s40593-014-0026-8
Cader, A. (2020, July). The Potential for the Use of Deep Neural Networks in e-Learning Student Evaluation with New Data Augmentation Method. In International Conference on Artificial Intelligence in Education (pp. 37–42). Springer, Cham.
Cai C (2019) Automatic essay scoring with recurrent neural network. In: Proceedings of the 3rd International Conference on High Performance Compilation, Computing and Communications (2019): n. pag.
Chen M, Li X (2018) "Relevance-Based Automated Essay Scoring via Hierarchical Recurrent Model. In: 2018 International Conference on Asian Language Processing (IALP), Bandung, Indonesia, 2018, p 378–383, doi: https://doi.org/10.1109/IALP.2018.8629256
Chen Z, Zhou Y (2019) "Research on Automatic Essay Scoring of Composition Based on CNN and OR. In: 2019 2nd International Conference on Artificial Intelligence and Big Data (ICAIBD), Chengdu, China, p 13–18, doi: https://doi.org/10.1109/ICAIBD.2019.8837007
Contreras JO, Hilles SM, Abubakar ZB (2018) Automated essay scoring with ontology based on text mining and NLTK tools. In: 2018 International Conference on Smart Computing and Electronic Enterprise (ICSCEE), 1-6
Correnti R, Matsumura LC, Hamilton L, Wang E (2013) Assessing students’ skills at writing analytically in response to texts. Elem Sch J 114(2):142–177
Cummins, R., Zhang, M., & Briscoe, E. (2016, August). Constrained multi-task learning for automated essay scoring. Association for Computational Linguistics.
Darwish SM, Mohamed SK (2020) Automated essay evaluation based on fusion of fuzzy ontology and latent semantic analysis. In: Hassanien A, Azar A, Gaber T, Bhatnagar RF, Tolba M (eds) The International Conference on Advanced Machine Learning Technologies and Applications
Dasgupta T, Naskar A, Dey L, Saha R (2018) Augmenting textual qualitative features in deep convolution recurrent neural network for automatic essay scoring. In: Proceedings of the 5th Workshop on Natural Language Processing Techniques for Educational Applications p 93–102
Ding Y, et al. (2020) "Don’t take “nswvtnvakgxpm” for an answer–The surprising vulnerability of automatic content scoring systems to adversarial input." In: Proceedings of the 28th International Conference on Computational Linguistics
Dong F, Zhang Y (2016) Automatic features for essay scoring–an empirical study. In: Proceedings of the 2016 Conference on Empirical Methods in Natural Language Processing p 1072–1077
Dong F, Zhang Y, Yang J (2017) Attention-based recurrent convolutional neural network for automatic essay scoring. In: Proceedings of the 21st Conference on Computational Natural Language Learning (CoNLL 2017) p 153–162
Dzikovska M, Nielsen R, Brew C, Leacock C, Gi ampiccolo D, Bentivogli L, Clark P, Dagan I, Dang HT (2013a) Semeval-2013 task 7: The joint student response analysis and 8th recognizing textual entailment challenge
Dzikovska MO, Nielsen R, Brew C, Leacock C, Giampiccolo D, Bentivogli L, Clark P, Dagan I, Trang Dang H (2013b) SemEval-2013 Task 7: The Joint Student Response Analysis and 8th Recognizing Textual Entailment Challenge. *SEM 2013: The First Joint Conference on Lexical and Computational Semantics
Educational Testing Service (2008) CriterionSM online writing evaluation service. Retrieved from http://www.ets.org/s/criterion/pdf/9286_CriterionBrochure.pdf .
Evanini, K., & Wang, X. (2013, August). Automated speech scoring for non-native middle school students with multiple task types. In INTERSPEECH (pp. 2435–2439).
Foltz PW, Laham D, Landauer TK (1999) The Intelligent Essay Assessor: Applications to Educational Technology. Interactive Multimedia Electronic Journal of Computer-Enhanced Learning, 1, 2, http://imej.wfu.edu/articles/1999/2/04/ index.asp
Granger, S., Dagneaux, E., Meunier, F., & Paquot, M. (Eds.). (2009). International corpus of learner English. Louvain-la-Neuve: Presses universitaires de Louvain.
Higgins, D., & Heilman, M. (2014). Managing what we can measure: Quantifying the susceptibility of automated scoring systems to gaming behavior. Educational Measurement: Issues and Practice, 33(3), 36–46.
Horbach A, Zesch T (2019) The influence of variance in learner answers on automatic content scoring. Front Educ 4:28. https://doi.org/10.3389/feduc.2019.00028
https://www.coursera.org/learn/machine-learning/exam/7pytE/linear-regression-with-multiple-variables/attempt
Hussein, M. A., Hassan, H., & Nassef, M. (2019). Automated language essay scoring systems: A literature review. PeerJ Computer Science, 5, e208.
Ke Z, Ng V (2019) “Automated essay scoring: a survey of the state of the art.” IJCAI
Ke, Z., Inamdar, H., Lin, H., & Ng, V. (2019, July). Give me more feedback II: Annotating thesis strength and related attributes in student essays. In Proceedings of the 57th Annual Meeting of the Association for Computational Linguistics (pp. 3994-4004).
Kelley K, Preacher KJ (2012) On effect size. Psychol Methods 17(2):137–152
Kitchenham B, Brereton OP, Budgen D, Turner M, Bailey J, Linkman S (2009) Systematic literature reviews in software engineering–a systematic literature review. Inf Softw Technol 51(1):7–15
Klebanov, B. B., & Madnani, N. (2020, July). Automated evaluation of writing–50 years and counting. In Proceedings of the 58th Annual Meeting of the Association for Computational Linguistics (pp. 7796–7810).
Knill K, Gales M, Kyriakopoulos K, et al. (4 more authors) (2018) Impact of ASR performance on free speaking language assessment. In: Interspeech 2018.02–06 Sep 2018, Hyderabad, India. International Speech Communication Association (ISCA)
Kopparapu SK, De A (2016) Automatic ranking of essays using structural and semantic features. In: 2016 International Conference on Advances in Computing, Communications and Informatics (ICACCI), p 519–523
Kumar, Y., Aggarwal, S., Mahata, D., Shah, R. R., Kumaraguru, P., & Zimmermann, R. (2019, July). Get it scored using autosas—an automated system for scoring short answers. In Proceedings of the AAAI Conference on Artificial Intelligence (Vol. 33, No. 01, pp. 9662–9669).
Kumar Y, et al. (2020) “Calling out bluff: attacking the robustness of automatic scoring systems with simple adversarial testing.” ArXiv abs/2007.06796
Li X, Chen M, Nie J, Liu Z, Feng Z, Cai Y (2018) Coherence-Based Automated Essay Scoring Using Self-attention. In: Sun M, Liu T, Wang X, Liu Z, Liu Y (eds) Chinese Computational Linguistics and Natural Language Processing Based on Naturally Annotated Big Data. CCL 2018, NLP-NABD 2018. Lecture Notes in Computer Science, vol 11221. Springer, Cham. https://doi.org/10.1007/978-3-030-01716-3_32
Liang G, On B, Jeong D, Kim H, Choi G (2018) Automated essay scoring: a siamese bidirectional LSTM neural network architecture. Symmetry 10:682
Liua, H., Yeb, Y., & Wu, M. (2018, April). Ensemble Learning on Scoring Student Essay. In 2018 International Conference on Management and Education, Humanities and Social Sciences (MEHSS 2018). Atlantis Press.
Liu J, Xu Y, Zhao L (2019) Automated Essay Scoring based on Two-Stage Learning. ArXiv, abs/1901.07744
Loukina A, et al. (2015) Feature selection for automated speech scoring.” BEA@NAACL-HLT
Loukina A, et al. (2017) “Speech- and Text-driven Features for Automated Scoring of English-Speaking Tasks.” SCNLP@EMNLP 2017
Loukina A, et al. (2019) The many dimensions of algorithmic fairness in educational applications. BEA@ACL
Lun J, Zhu J, Tang Y, Yang M (2020) Multiple data augmentation strategies for improving performance on automatic short answer scoring. In: Proceedings of the AAAI Conference on Artificial Intelligence, 34(09): 13389-13396
Madnani, N., & Cahill, A. (2018, August). Automated scoring: Beyond natural language processing. In Proceedings of the 27th International Conference on Computational Linguistics (pp. 1099–1109).
Madnani N, et al. (2017b) “Building better open-source tools to support fairness in automated scoring.” EthNLP@EACL
Malinin A, et al. (2016) “Off-topic response detection for spontaneous spoken english assessment.” ACL
Malinin A, et al. (2017) “Incorporating uncertainty into deep learning for spoken language assessment.” ACL
Mathias S, Bhattacharyya P (2018a) Thank “Goodness”! A Way to Measure Style in Student Essays. In: Proceedings of the 5th Workshop on Natural Language Processing Techniques for Educational Applications p 35–41
Mathias S, Bhattacharyya P (2018b) ASAP++: Enriching the ASAP automated essay grading dataset with essay attribute scores. In: Proceedings of the Eleventh International Conference on Language Resources and Evaluation (LREC 2018).
Mikolov T, et al. (2013) “Efficient Estimation of Word Representations in Vector Space.” ICLR
Mohler M, Mihalcea R (2009) Text-to-text semantic similarity for automatic short answer grading. In: Proceedings of the 12th Conference of the European Chapter of the ACL (EACL 2009) p 567–575
Mohler M, Bunescu R, Mihalcea R (2011) Learning to grade short answer questions using semantic similarity measures and dependency graph alignments. In: Proceedings of the 49th annual meeting of the association for computational linguistics: Human language technologies p 752–762
Muangkammuen P, Fukumoto F (2020) Multi-task Learning for Automated Essay Scoring with Sentiment Analysis. In: Proceedings of the 1st Conference of the Asia-Pacific Chapter of the Association for Computational Linguistics and the 10th International Joint Conference on Natural Language Processing: Student Research Workshop p 116–123
Nguyen, H., & Dery, L. (2016). Neural networks for automated essay grading. CS224d Stanford Reports, 1–11.
Palma D, Atkinson J (2018) Coherence-based automatic essay assessment. IEEE Intell Syst 33(5):26–36
Parekh S, et al (2020) My Teacher Thinks the World Is Flat! Interpreting Automatic Essay Scoring Mechanism.” ArXiv abs/2012.13872 (2020): n. pag
Pennington, J., Socher, R., & Manning, C. D. (2014, October). Glove: Global vectors for word representation. In Proceedings of the 2014 conference on empirical methods in natural language processing (EMNLP) (pp. 1532–1543).
Persing I, Ng V (2013) Modeling thesis clarity in student essays. In:Proceedings of the 51st Annual Meeting of the Association for Computational Linguistics (Volume 1: Long Papers) p 260–269
Powers DE, Burstein JC, Chodorow M, Fowles ME, Kukich K (2001) Stumping E-Rater: challenging the validity of automated essay scoring. ETS Res Rep Ser 2001(1):i–44
Google Scholar
Powers, D. E., Burstein, J. C., Chodorow, M., Fowles, M. E., & Kukich, K. (2002). Stumping e-rater: challenging the validity of automated essay scoring. Computers in Human Behavior, 18(2), 103–134.
Ramachandran L, Cheng J, Foltz P (2015) Identifying patterns for short answer scoring using graph-based lexico-semantic text matching. In: Proceedings of the Tenth Workshop on Innovative Use of NLP for Building Educational Applications p 97–106
Ramanarayanan V, et al. (2017) “Human and Automated Scoring of Fluency, Pronunciation and Intonation During Human-Machine Spoken Dialog Interactions.” INTERSPEECH
Riordan B, Horbach A, Cahill A, Zesch T, Lee C (2017) Investigating neural architectures for short answer scoring. In: Proceedings of the 12th Workshop on Innovative Use of NLP for Building Educational Applications p 159–168
Riordan B, Flor M, Pugh R (2019) "How to account for misspellings: Quantifying the benefit of character representations in neural content scoring models."In: Proceedings of the Fourteenth Workshop on Innovative Use of NLP for Building Educational Applications
Rodriguez P, Jafari A, Ormerod CM (2019) Language models and Automated Essay Scoring. ArXiv, abs/1909.09482
Rudner, L. M., & Liang, T. (2002). Automated essay scoring using Bayes' theorem. The Journal of Technology, Learning and Assessment, 1(2).
Rudner, L. M., Garcia, V., & Welch, C. (2006). An evaluation of IntelliMetric™ essay scoring system. The Journal of Technology, Learning and Assessment, 4(4).
Rupp A (2018) Designing, evaluating, and deploying automated scoring systems with validity in mind: methodological design decisions. Appl Meas Educ 31:191–214
Ruseti S, Dascalu M, Johnson AM, McNamara DS, Balyan R, McCarthy KS, Trausan-Matu S (2018) Scoring summaries using recurrent neural networks. In: International Conference on Intelligent Tutoring Systems p 191–201. Springer, Cham
Sakaguchi K, Heilman M, Madnani N (2015) Effective feature integration for automated short answer scoring. In: Proceedings of the 2015 conference of the North American Chapter of the association for computational linguistics: Human language technologies p 1049–1054
Salim, Y., Stevanus, V., Barlian, E., Sari, A. C., & Suhartono, D. (2019, December). Automated English Digital Essay Grader Using Machine Learning. In 2019 IEEE International Conference on Engineering, Technology and Education (TALE) (pp. 1–6). IEEE.
Shehab A, Elhoseny M, Hassanien AE (2016) A hybrid scheme for Automated Essay Grading based on LVQ and NLP techniques. In: 12th International Computer Engineering Conference (ICENCO), Cairo, 2016, p 65-70
Shermis MD, Mzumara HR, Olson J, Harrington S (2001) On-line grading of student essays: PEG goes on the World Wide Web. Assess Eval High Educ 26(3):247–259
Stab C, Gurevych I (2014) Identifying argumentative discourse structures in persuasive essays. In: Proceedings of the 2014 Conference on Empirical Methods in Natural Language Processing (EMNLP) p 46–56
Sultan MA, Salazar C, Sumner T (2016) Fast and easy short answer grading with high accuracy. In: Proceedings of the 2016 Conference of the North American Chapter of the Association for Computational Linguistics: Human Language Technologies p 1070–1075
Süzen, N., Gorban, A. N., Levesley, J., & Mirkes, E. M. (2020). Automatic short answer grading and feedback using text mining methods. Procedia Computer Science, 169, 726–743.
Taghipour K, Ng HT (2016) A neural approach to automated essay scoring. In: Proceedings of the 2016 conference on empirical methods in natural language processing p 1882–1891
Tashu TM (2020) "Off-Topic Essay Detection Using C-BGRU Siamese. In: 2020 IEEE 14th International Conference on Semantic Computing (ICSC), San Diego, CA, USA, p 221–225, doi: https://doi.org/10.1109/ICSC.2020.00046
Tashu TM, Horváth T (2019) A layered approach to automatic essay evaluation using word-embedding. In: McLaren B, Reilly R, Zvacek S, Uhomoibhi J (eds) Computer Supported Education. CSEDU 2018. Communications in Computer and Information Science, vol 1022. Springer, Cham
Tashu TM, Horváth T (2020) Semantic-Based Feedback Recommendation for Automatic Essay Evaluation. In: Bi Y, Bhatia R, Kapoor S (eds) Intelligent Systems and Applications. IntelliSys 2019. Advances in Intelligent Systems and Computing, vol 1038. Springer, Cham
Uto M, Okano M (2020) Robust Neural Automated Essay Scoring Using Item Response Theory. In: Bittencourt I, Cukurova M, Muldner K, Luckin R, Millán E (eds) Artificial Intelligence in Education. AIED 2020. Lecture Notes in Computer Science, vol 12163. Springer, Cham
Wang Z, Liu J, Dong R (2018a) Intelligent Auto-grading System. In: 2018 5th IEEE International Conference on Cloud Computing and Intelligence Systems (CCIS) p 430–435. IEEE.
Wang Y, et al. (2018b) “Automatic Essay Scoring Incorporating Rating Schema via Reinforcement Learning.” EMNLP
Zhu W, Sun Y (2020) Automated essay scoring system using multi-model Machine Learning, david c. wyld et al. (eds): mlnlp, bdiot, itccma, csity, dtmn, aifz, sigpro
Wresch W (1993) The Imminence of Grading Essays by Computer-25 Years Later. Comput Compos 10:45–58
Wu, X., Knill, K., Gales, M., & Malinin, A. (2020). Ensemble approaches for uncertainty in spoken language assessment.
Xia L, Liu J, Zhang Z (2019) Automatic Essay Scoring Model Based on Two-Layer Bi-directional Long-Short Term Memory Network. In: Proceedings of the 2019 3rd International Conference on Computer Science and Artificial Intelligence p 133–137
Yannakoudakis H, Briscoe T, Medlock B (2011) A new dataset and method for automatically grading ESOL texts. In: Proceedings of the 49th annual meeting of the association for computational linguistics: human language technologies p 180–189
Zhao S, Zhang Y, Xiong X, Botelho A, Heffernan N (2017) A memory-augmented neural model for automated grading. In: Proceedings of the Fourth (2017) ACM Conference on Learning@ Scale p 189–192
Zupanc K, Bosnic Z (2014) Automated essay evaluation augmented with semantic coherence measures. In: 2014 IEEE International Conference on Data Mining p 1133–1138. IEEE.
Zupanc K, Savić M, Bosnić Z, Ivanović M (2017) Evaluating coherence of essays using sentence-similarity networks. In: Proceedings of the 18th International Conference on Computer Systems and Technologies p 65–72
Dzikovska, M. O., Nielsen, R., & Brew, C. (2012, June). Towards effective tutorial feedback for explanation questions: A dataset and baselines. In Proceedings of the 2012 Conference of the North American Chapter of the Association for Computational Linguistics: Human Language Technologies (pp. 200-210).
Kumar, N., & Dey, L. (2013, November). Automatic Quality Assessment of documents with application to essay grading. In 2013 12th Mexican International Conference on Artificial Intelligence (pp. 216–222). IEEE.
Wu, S. H., & Shih, W. F. (2018, July). A short answer grading system in chinese by support vector approach. In Proceedings of the 5th Workshop on Natural Language Processing Techniques for Educational Applications (pp. 125-129).
Agung Putri Ratna, A., Lalita Luhurkinanti, D., Ibrahim I., Husna D., Dewi Purnamasari P. (2018). Automatic Essay Grading System for Japanese Language Examination Using Winnowing Algorithm, 2018 International Seminar on Application for Technology of Information and Communication, 2018, pp. 565–569. https://doi.org/10.1109/ISEMANTIC.2018.8549789 .
Sharma A., & Jayagopi D. B. (2018). Automated Grading of Handwritten Essays 2018 16th International Conference on Frontiers in Handwriting Recognition (ICFHR), 2018, pp 279–284. https://doi.org/10.1109/ICFHR-2018.2018.00056
Download references
Not Applicable.
Author information
Authors and affiliations.
School of Computer Science and Artificial Intelligence, SR University, Warangal, TS, India
Dadi Ramesh
Research Scholar, JNTU, Hyderabad, India
Department of Information Technology, JNTUH College of Engineering, Nachupally, Kondagattu, Jagtial, TS, India
Suresh Kumar Sanampudi
You can also search for this author in PubMed Google Scholar
Corresponding author
Correspondence to Dadi Ramesh .
Additional information
Publisher's note.
Springer Nature remains neutral with regard to jurisdictional claims in published maps and institutional affiliations.

Supplementary Information
Below is the link to the electronic supplementary material.
Supplementary file1 (XLSX 80 KB)
Rights and permissions.
Reprints and permissions
About this article
Ramesh, D., Sanampudi, S.K. An automated essay scoring systems: a systematic literature review. Artif Intell Rev 55 , 2495–2527 (2022). https://doi.org/10.1007/s10462-021-10068-2
Download citation
Published : 23 September 2021
Issue Date : March 2022
DOI : https://doi.org/10.1007/s10462-021-10068-2
Share this article
Anyone you share the following link with will be able to read this content:
Sorry, a shareable link is not currently available for this article.
Provided by the Springer Nature SharedIt content-sharing initiative
- Short answer scoring
- Essay grading
- Natural language processing
- Deep learning
- Find a journal
- Publish with us
- Track your research
A Study on Web Based Online Examination System
International Conference on Recent Trends in Artificial Intelligence, IOT, Smart Cities & Applications (ICAISC-2020)
3 Pages Posted: 24 Sep 2020
Anjali Choubey
Department of Computer Science & Engineering, Chaibasa Engineering College, Jharkhand, India
Avinash Kumar
Ayush ranjan behra, anil raj kisku, asha rabidas, beas bhadra.
Date Written: May 27, 2020
Online examination system is a web-based examination system where examinations are given online. Either through the internet or intranet using computer system. The main goal of this online examination system is to effectively evaluate the student thoroughly through a totally automated system that not only reduce the required time but also obtain fast and accurate results. The main objective of our software is to efficiently evaluate the candidate thoroughly through a fully automated system there is no need of paper and pen. The user can write exam without going to the exam centre. Also the website will provide a good.
Keywords: Online Exam, Offline Exam, Efficiency, Accuracy, Assesment
Suggested Citation: Suggested Citation
Anjali Choubey (Contact Author)
Department of computer science & engineering, chaibasa engineering college, jharkhand, india ( email ), do you have a job opening that you would like to promote on ssrn, paper statistics, related ejournals, pedagogy ejournal.
Subscribe to this fee journal for more curated articles on this topic
Applied Computing eJournal
Software engineering ejournal, computer science education ejournal, electrical engineering ejournal, engineering education ejournal, materials science education ejournal.

An official website of the United States government
The .gov means it’s official. Federal government websites often end in .gov or .mil. Before sharing sensitive information, make sure you’re on a federal government site.
The site is secure. The https:// ensures that you are connecting to the official website and that any information you provide is encrypted and transmitted securely.
- Publications
- Account settings
Preview improvements coming to the PMC website in October 2024. Learn More or Try it out now .
- Advanced Search
- Journal List
- Elsevier - PMC COVID-19 Collection

A systematic review of online examinations: A pedagogical innovation for scalable authentication and integrity
Kerryn butler-henderson.
a College of Health and Medicine, University of Tasmania, Locked Bag 1322, Launceston, Tasmania, 7250, Australia
Joseph Crawford
b Academic Division, University of Tasmania, Locked Bag 1322, Launceston, Tasmania, 7250, Australia
Digitization and automation across all industries has resulted in improvements in efficiencies and effectiveness to systems and process, and the higher education sector is not immune. Online learning, e-learning, electronic teaching tools, and digital assessments are not innovations. However, there has been limited implementation of online invigilated examinations in many countries. This paper provides a brief background on online examinations, followed by the results of a systematic review on the topic to explore the challenges and opportunities. We follow on with an explication of results from thirty-six papers, exploring nine key themes: student perceptions, student performance, anxiety, cheating, staff perceptions, authentication and security, interface design, and technology issues. While the literature on online examinations is growing, there is still a dearth of discussion at the pedagogical and governance levels.
- • There is a lack of score variation between examination modalities.
- • Online exams offer various methods for mitigating cheating.
- • There is a favorable ratings for online examinations by students.
- • Staff preferred online examinations for their ease of completion and logistics.
- • The interface of a system continues to be an enabler or barrier of online exams.
1. Introduction
Learning and teaching is transforming away from the conventional lecture theatre designed to seat 100 to 10,000 passive students towards more active learning environments. In our current climate, this is exacerbated by COVID-19 responses ( Crawford et al., 2020 ), where thousands of students are involved in online adaptions of face-to-face examinations (e.g. online Zoom rooms with all microphones and videos locked on). This evolution has grown from the need to recognize that students now rarely study exclusively and have commitments that conflict with their University life (e.g. work, family, social obligations). Students have more diverse digitally capability ( Margaryan et al., 2011 ) and higher age and gender diversity ( Eagly & Sczesny, 2009 ; Schwalb & Sedlacek, 1990 ). Continual change of the demographic and profile of students creates a challenge for scholars seeking to develop a student experience that demonstrates quality and maintains financial and academic viability ( Gross et al., 2013 ; Hainline et al., 2010 ).
Universities are developing extensive online offerings to grow their international loads and facilitate the massification of higher learning. These protocols, informed by growing policy targets to educate a larger quantity of graduates (e.g. Kemp, 1999 ; Reiko, 2001 ), have challenged traditional university models of fully on-campus student attendance. The development of online examination software has offered a systematic and technological alternative to the end-of-course summative examination designed for final authentication and testing of student knowledge retention, application, and extension. As a result of the COVID-19 pandemic, the initial response in higher education across many countries was to postpone examinations ( Crawford et al., 2020 ). However, as the pandemic continued, the need to move to either an online examination format or alternative assessment became more urgent.
This paper is a timely exploration of the contemporary literature related to online examinations in the university setting, with the hopes to consolidate information on this relatively new pedagogy in higher education. This paper begins with a brief background of traditional examinations, as the assumptions applied in many online examination environments build on the techniques and assumptions of the traditional face-to-face gymnasium-housed invigilated examinations. This is followed by a summary of the systematic review method, including search strategy, procedure, quality review, analysis, and summary of the sample.
Print-based educational examinations designed to test knowledge have existed for hundreds of years. The New York State Education Department has “the oldest educational testing service in the United States” and has been delivering entrance examinations since 1865 ( Johnson, 2009 , p. 1; NYSED, 2012 ). In pre-Revolution Russia, it was not possible to obtain a diploma to enter university without passing a high-stakes graduation examinations ( Karp, 2007 ). These high school examinations assessed and assured learning of students in rigid and high-security conditions. Under traditional classroom conditions, these were likely a reasonable practice to validate knowledge. The discussion of authenticating learning was not a consideration at this stage, as students were face to face only. For many high school jurisdictions, these are designed to strengthen the accountability of teachers and assess student performance ( Mueller & Colley, 2015 ).
In tertiary education, the use of an end-of-course summative examination as a form of validating knowledge has been informed significantly by accreditation bodies and streamlined financially viable assessment options. The American Bar Association has required a final course examination to remain accredited ( Sheppard, 1996 ). Law examinations typically contained brief didactic questions focused on assessing rote memory through to problem-based assessment to evaluate students’ ability to apply knowledge ( Sheppard, 1996 ). In accredited courses, there are significant parallels. Alternatives to traditional gymnasium-sized classroom paper-and-pencil invigilated examinations have been developed with educators recognizing the limitations associated with single-point summative examinations ( Butt, 2018 ).
The objective structured clinical examinations (OSCE) incorporate multiple workstations with students performing specific practical tasks from physical examinations on mannequins to short-answer written responses to scenarios ( Turner & Dankoski, 2008 ). The OSCE has parallels with the patient simulation examination used in some medical schools ( Botezatu et al., 2010 ). Portfolios assess and demonstrate learning over a whole course and for extracurricular learning ( Wasley, 2008 ).
The inclusion of online examinations, e-examinations, and bring-your-own-device models have offered alternatives to the large-scale examination rooms with paper-and-pencil invigilated examinations. Each of these offer new opportunities for the inclusion of innovative pedagogies and assessment where examinations are considered necessary. Further, some research indicates online examinations are able to discern a true pass from a true fail with a high level of accuracy ( Ardid et al., 2015 ), yet there is no systematic consolidation of the literature. We believe this timely review is critical for the progression of the field in first stepping back and consolidating the existing practices to support dissemination and further innovation. The pursuit of such systems may be to provide formative feedback and to assess learning outcomes, but a dominant rationale for final examinations is to authenticate learning. That is, to ensure the student whose name is on the student register, is the student who is completing the assessed work. The development of digitalized examination pilot studies and case studies are becoming an expected norm with universities developing responses to a growing online curriculum offering (e.g. Al-Hakeem & Abdulrahman, 2017 ; Alzu'bi, 2015 ; Anderson et al., 2005 ; Fluck et al., 2009 ; Fluck et al., 2017 ; Fluck, 2019 ; Seow & Soong, 2014 ; Sindre & Vegendla, 2015 ; Steel et al., 2019 ; Wibowo et al., 2016 ).
As many scholars highlight, cheating is a common component of the contemporary student experience ( Jordan, 2001 ; Rettinger & Kramer, 2009 ) despite that it should not be. Some are theorizing responses to the inevitability of cheating from developing student capacity for integrity ( Crawford, 2015 ; Wright, 2011 ) to enhancing detection of cheating ( Dawson & Sutherland-Smith, 2018 , 2019 ) and legislation to ban contract cheating ( Amigud & Dawson, 2020 ). We see value in the pursuit of methods that can support integrity in student assessment, including during rapid changes to the curriculum. The objective of this paper is to summarize the current evidence on online examination methods, and scholarly responses to authentication of learning and the mitigation of cheating, within the confines of assessment that enables learning and student wellbeing. We scope out preparation for examinations (e.g. Nguyen & Henderson, 2020 ) to enable focus on the online exam setting specifically.
2. Material and methods
2.1. search strategy.
To address the objective of this paper, a systematic literature review was undertaken, following the PRISMA approach for article selection ( Moher et al., 2009 ). The keyword string was developed incorporating the U.S. National Library of Medicine (2019) MeSH (Medical Subject Headings) terms: [(“online” OR “electronic” OR “digital”) AND (“exam*” OR “test”) AND (“university” OR “educat*” OR “teach” OR “school” OR “college”)]. The following databases were queried: A + Education (Informit), ERIC (EBSCO), Education Database (ProQuest), Education Research Complete (EBSCO), Educational Research Abstracts Online (Taylor & Francis), Informit, and Scopus. These search phrases will enable the collection of a broad range of literature on online examinations as well as terms often used synonymously, such as e-examination/eExams and BYOD (bring-your-own-device) examinations. The eligibility criteria included peer-reviewed journal articles or full conference papers on online examinations in the university sector, published between 2009 and 2018, available in English. As other sources (e.g. dissertations) are not peer-reviewed, and we aimed to identify rigorous best practice literature, we excluded these. We subsequently conducted a general search in Google Scholar and found no additional results. All records returned from the search were extracted and imported into the Covidence® online software by the first author.
2.2. Selection procedure and quality assessment
The online Covidence® software facilitated article selection following the PRISMA approach. Each of the 1906 titles and abstracts were double-screened by the authors based on the eligibility criteria. We also excluded non-higher education examinations, given the context around student demographics is often considerably different than vocational education, primary and high schools. Where there was discordance between the authors on a title or abstract inclusion or exclusion, consensus discussions were undertaken. The screening reduced the volume of papers significantly because numerous papers related to a different education context or involved online or digital forms of medical examinations. Next, the full-text for selected abstracts were double-reviewed, with discordance managed through a consensus discussion. The papers selected following the double full-text review were accepted for this review. Each accepted paper was reviewed for quality using the MMAT system ( Hong et al., 2018 ) and the scores were calculated as high, medium, or low quality based on the matrix ( Hong et al., 2018 ). A summary of this assessment is presented in Table 1 .
Summary of article characteristics.
First Author | Year | Country | Method | Participants | Theme | QAS |
---|---|---|---|---|---|---|
AbdelKarim | Saudi, Jordan, Malaysia | Survey | 119 students | Student perception, interface design | Medium | |
Abumansour | Saudi | Description | NA | Authentication and security | Low | |
Aisyah | Indonesia | Description | NA | Authentication and security | Low | |
Attia | Saudi | Survey | 34 students | Student perception, anxiety | High | |
Böhmer | Germany | Survey | 17 students | Student perception, student performance | Medium | |
Chao | Taiwan | Survey | 25 students | Authentication and security | Medium | |
Chebrolu | India | Description | NA | Authentication and security | Low | |
Chen | China | Exam data analysis | Not provided | Student performance | Medium | |
Chytrý | Czech Republic | Exam data analysis | 115 students | Student performance | High | |
Daffin | USA | Exam data analysis | 1694 students | Student performance | High | |
2016 | Australia | Description | NA | Cheating | Low | |
Ellis | UK | Survey, exam data analysis | >120 students | Student performance | Medium | |
Gehringer | USA | Survey | 85 staff and 315 students | Cheating, administration | Medium | |
Gold | USA | Exam data analysis | 1800 students | Student performance | Medium | |
Guillen-Ganez | Spain | Exam data analysis | 70 students | Authentication and security | Medium | |
HearnMoore | USA | Description, exam data analysis | Not provided | Cheating | Medium | |
Hylton | Jamaica | Survey, exam data analysis | 350 students | Cheating | High | |
Kolagari | Iran | Test Anxiety Scale | 39 students | Anxiety | High | |
Kolski | USA | Test Anxiety Scale, exam data analysis, interviews | 238 students | Anxiety | High | |
Kumar | USA | Problem analysis | 2 staff | Anxiety | High | |
Li | USA | Exam data analysis | 9 students | Cheating | High | |
Matthiasdottir | Iceland | Survey | 183 students | Student perceptions, anxiety | Medium | |
Mitra | USA | Interviews, survey | 5 staff; 30 students | Cheating, administration | Medium | |
Mohanna | Saudi | Exam data analysis | 127 students | Student performance, technical issues | High | |
Oz | Turkey | Exam data analysis | 97 students | Student performance | High | |
Pagram | Australia | Interviews, survey | Interviews: 4 students, 2 staff; Survey: 6 students | Student perceptions, academic perceptions, anxiety | Medium | |
Park | USA | Survey | 37 students | Student perception | Medium | |
Patel | Saudi | Exam data analysis | 180 students | Student performance | High | |
Petrović | Croatia | Exam data analysis | 591 students | Cheating | Medium | |
Rios | USA | Exam data analysis, survey | 1126 students | Student performance, student perceptions, Authentication and security (under user friendliness) | High | |
Rodchua | USA | Description | NA | Cheating | Low | |
Schmidt | USA | Survey | 49 students | Student performance, academic perception, student perception, anxiety, tech issues | High | |
Stowell | USA | Test Anxiety Scale, exam data analysis | 69 students | Anxiety | High | |
Sullivan | USA | Exam data analysis, survey | 178 students | Cheating | Medium | |
Williams | Singapore | Survey | 91 students | Student perception, cheating | Medium | |
Yong-Sheng | China | Description | NA | Authentication and security | Low |
QAS, quality assessment score.
2.3. Thematic analysis
Following the process described by Braun and Clarke (2006) , an inductive thematic approach was undertaken to identify common themes identified in each article. This process involves six stages: data familiarization, data coding, theme searching, theme review, defining themes, and naming themes. Familiarization with the literature was achieved during the screening, full-text, and quality review process by triple exposure to works. The named authors then inductively coded half the manuscripts each. The research team consolidated the data together to identify themes. Upon final agreement of themes and their definitions, the write-up was split among the team with subsequent review and revision of ideas in themes through independent and collaborative writing and reviewing ( Creswell & Miller, 2000 ; Lincoln & Guba, 1985 ). This resulted in nine final themes, each discussed in-depth during the discussion.
There were thirty-six (36) articles identified that met the eligibility criteria and were selected following the PRISMA approach, as shown in Fig. 1 .

PRISMA results.
3.1. Characteristics of selected articles
The selected articles are from a wide range of discipline areas and countries. Table 1 summarizes the characteristics of the selected articles. The United States of America held a vast majority (14, 38.9%) of the publications on online examinations, followed by Saudi Arabia (4, 11.1%), China (2, 5.6%), and Australia (2, 5.6%). When aggregated at the region-level, there was an equality of papers from North America and Asia (14, 38.9% each), with Europe (6, 16.7%) and Oceania (2, 5.6%) least represented in the selection of articles. There has been considerable growth in publications in the past five years, concerning online examinations. Publications between the years 2009 and 2015 represented a third (12, 33.3%) of the total number of selected papers. The majority (24, 66.7%) of papers were published in the last three years. Papers that described a system but did not include empirical evidence scored a low-quality rank as they did not meet many of the criteria that relate to the evaluation of a system.
When examining the types of papers, the majority (30, 83.3%) were empirical research, with the remainder commentary papers (6, 16.7%). Of the empirical research papers, three-quarters of the paper reported a quantitative study design (32, 88.9%) compared to two (5.6%) qualitative study designs and two (5.6%) that used a mixed method. For quantitative studies, there was a range between nine and 1800 student participants ( x ̄ = 291.62) across 26 studies, and a range between two and 85 staff participants ( x ̄ = 30.67) in one study. The most common quantitative methods were self-administered surveys and analysis of numerical examination student grades (38% each). Qualitative and mixed methods studies only adopted interviews (6%). Only one qualitative study reported a sample of students ( n = 4), with two qualitative studies reporting a sample of staff ( n = 2, n = 5).
3.2. Student perceptions
Today's students prefer online examinations compared to paper exams ([68.75% preference of online over paper-based examinations: Attia, 2014 ; 56–62.5%: Böhmer et al., 2018 ; no percentage: ( Schmidt, Ralph & Buskirk, 2009 ); 92%: Matthíasdóttir & Arnalds, 2016 ; no percentage: Pagram et al., 2018 ; 51%: Park, 2017 ; 84%: Schmidt, Ralph & Williams & Wong, 2009 ). Two reasons provided for the preference is the increased speed and ease of editing responses ( Pagram et al., 2018 ), with one study finding two-thirds (67%) of students reported a positive experience in online examination environment ( Matthíasdóttir & Arnalds, 2016 ). Students believe online examinations allows a more authentic assessment experience ( Williams & Wong, 2009 ), with 78 percent of students reporting consistencies between the online environment and their future real-world environment ( Matthíasdóttir & Arnalds, 2016 ).
Students perceive the online examinations saves time (75.0% of students surveyed) and is more economical (87.5%) than paper examinations ( Attia, 2014 ). It provides greater flexibility for completing examinations ( Schmidt et al., 2009 ) with faster access to remote student papers (87.5%) and students trust the result of online over paper-based examinations (78.1%: Attia, 2014 ). The majority of students (59.4%: Attia, 2014 ; 55.5%: Pagram et al., 2018 ) perceive that the online examination environment makes it easier to cheat. More than half (56.25%) of students believe that a lack of information communication and technology (ICT) skill do not adversely affect performance in online examinations ( Attia, 2014 ). Nearly a quarter (23%) of students reported ( Abdel Karim & Shukur, 2016 ) the most preferred font face (type) was Arial, a font also recommended by Vision Australia (2014) in their guidelines for online and print inclusive design and legibility considerations. Nearly all (87%) students preferred black text color on a white background color (87%). With regards to onscreen time counters, a countdown counter was the most preferred option (42%) compared to a traditional analogue clock (30%) or an ascending counter (22%). Many systems allow students to set their preferred remaining time reminder or alert, including 15 min remaining (35% students preferred), 5 min remaining (26%), mid-examination (15%) or 30 min remaining (13%).
3.3. Student performance
Several studies in the sample referred to a lack of score variation between the results of examination across different administration methods. For example, student performance did not have significant difference in final examination scores across online and traditional examination modalities ( Gold & Mozes-Carmel, 2017 ). This is reinforced by a test of validity and reliability of computer-based and paper-based assessment that demonstrated no significant difference ( Oz & Ozturan, 2018 ), and equality of grades identified across the two modalities ( Stowell & Bennett, 2010 ).
When considering student perceptions, of the studies documented in our sample, there tended to be favorable ratings of online examinations. In a small sample of 34 postgraduate students, the respondents had positive perceptions towards online learning assessments (67.4%). The students also believed it contributed to improved learning and feedback (67.4%), and 77 percent had favorable attitudes towards online assessment ( Attia, 2014 ). In a pre-examination survey, students indicated they preferred to type than to write, felt more confident about the examination, and had limited issues with software and hardware ( Pagram, 2018 ). With the same sample in a post-examination survey, within the design and technology examination, students felt the software and hardware were simple to use, yet many students did not feel at ease from their use of an e-examination.
Rios and Liu (2017) compared proctored and non-proctored online examinations across several aspects, including test-taking behavior. Their study did not identify any difference in the test-taking behavior of students between the two environments. There was no significant difference between omitted items and not-reached items. Furthermore, with regards to rapid guessing, there was no significant difference. A negligible difference existed for students aged older than thirty-five years, yet gender was a nonsignificant factor.
3.4. Anxiety
Scholars have an increasing awareness of the role that test anxiety has in reducing student success in online learning environments ( Kolski & Weible, 2018 ). The manuscripts identified by the literature scan, identified inconsistencies of results for the effect that examination modalities have on student test anxiety. A study of 69 psychology undergraduates identified that students who typically experienced high anxiety in traditional test environments had lower anxiety levels when completing an online examination ( Stowell & Bennett, 2010 ). In a quasi-experimental study ( n = 38 nursing students), when baseline anxiety is controlled, students in computer-based examinations had higher degrees of test anxiety.
In 34 postgraduate student interviews, only three opposed online assessment based on perceived lack of technical skill (e.g. typing; Attia, 2014 ). Around two-thirds of participants identified some form of fear-based on internet disconnection, electricity, slow typing, or family disturbances at home. A 37 participant Community College study used proximal indicators (e.g. lip licking and biting, furrowed eyebrows, and seat squirming) to assess the rate of test anxiety in webcam-based examination proctoring ( Kolski & Weible, 2018 ). Teacher strategies to reduce anxiety in their students include enabling students to consider, review, and acknowledge their anxieties ( Kolski & Weible, 2018 ). Responses such as students writing of their anxiety, or responding to multiple-choice questionnaire on test anxiety, reduced anxiety. Students in the test group and provided anxiety items or expressive writing exercises, performed better ( Kumar, 2014 ).
3.5. Cheating
Cheating was the most prevalent area among all the themes identified. Cheating in asynchronous, objective, and online assessments is argued by some to be at unconscionable levels ( Sullivan, 2016 ). In one survey, 73.6 percent of students felt it was easier to cheat on online examinations than regular examinations ( Aisyah et al., 2018 ). This is perhaps because students are monitored in paper and pencil examinations, compared to online examinations where greater control of variables is required to mitigate cheating. Some instructors have used randomized examination batteries to minimize cheating potential through peer-to-peer sharing ( Schmidt et al., 2009 ).
Scholars identify various methods for mitigating cheating. Identifying the test taker, preventing examination theft, unauthorized use of textbook/notes, preparing a set-up for online examination, unauthorized student access to a test bank, preventing the use of devices (e.g. phone, Bluetooth, and calculators), limiting access to other people during the examination, equitable access to equipment, identifying computer crashes, inconsistency of method for proctoring ( Hearn Moore et al., 2017 ). In another, the issue for solving cheating is social as well as technological. While technology is considered the current norm for reducing cheating, these tools have been mostly ineffective ( Sullivan, 2016 ). Access to multiple question banks through effective quiz design and delivery is a mechanism to reduce the propensity to cheat, by reducing the stakes through multiple delivery attempts ( Sullivan, 2016 ). Question and answer randomization, continuous question development, multiple examination versions, open book options, time stamps, and diversity in question formats, sequences, types, and frequency are used to manage the perception and potential for cheating. In the study with MBA students, perception of the ability to cheat seemed to be critical for the development of a safe online examination environment ( Sullivan, 2016 ).
Dawson (2016) in a review of bring-your-own-device examinations including:
- • Copying contents of USB to a hard drive to make a copy of the digital examination available to others,
- • Use of a virtual machine to maintain access to standard applications on their device,
- • USB keyboard hacks to allow easy access to other documents (e.g. personal notes),
- • Modifying software to maintain complete control of their own device, and
- • A cold boot attack to maintain a copy of the examination.
The research on cheating has focused mainly on technical challenges (e.g. hardware to support cheating), rather than ethical and social issues (e.g. behavioral development to curb future cheating behaviors). The latter has been researched in more depth in traditional assessment methods (e.g. Wright, 2015 ). In a study on Massive Open Online Courses (MOOCs), motivations for students to engage in optional learning stemmed from knowledge, work, convenience, and personal interest ( Shapiro et al., 2017 ). This provides possible opportunities for future research to consider behavioral elements for responding to cheating, rather than institutional punitive arrangements.
3.6. Staff perception
Schmidt et al. (2009) also examined the perceptions of academics with regards to online examination. Academics reported that their biggest concern with using online examinations is the potential for cheating. There was a perception that students may get assistance during an examination. The reliability of the technology is the second more critical concern of academic staff. This includes concerns about internet connectivity as well as computer or software issues. The third concern is related to ease of use, both for the academic and for students. Academics want a system that is easy and quick to create, manage and mark examinations, and students can use with proficient ICT skills ( Schmidt et al., 2009 ). Furthermore, staff reported in a different study that marking digital work was easier and preferred it over paper examinations because of the reduction in paper ( Pagram et al., 2018 ). They believe preference should be given to using university machines instead of the student using their computer, mainly due to issues around operating system compatibility and data loss.
3.7. Authentication and security
Authentication was recognized as a significant issue for examination. Some scholars indicate that the primary reason for requiring physical attendance to proctored examinations is to validate and authenticate the student taking the assessment ( Chao et al., 2012 ). Importantly, the validity of online proctored examination administration procedures is argued as lower than proctored on-campus examinations ( Rios & Liu, 2017 ). Most responses to online examinations use bring-your-own-device models where laptops are brought to traditional lecture theatres, use of software on personal devices in any location desired, or use of prescribed devices in a classroom setting. The primary goal of each is to balance the authentication of students and maintain the integrity and value of achieving learning outcomes.
In a review of current authentication options ( AbuMansoor, 2017 ), the use of fingerprint reading, streaming media, and follow-up identifications were used to authenticate small cohorts of students. Some learning management systems (LMS) have developed subsidiary products (e.g. Weaver within Moodle) to support authentication processes. Some biometric software uses different levels to authenticate keystrokes for motor controls, stylometry for linguistics, application behavior for semantics, capture to physical or behavioral samples, extraction of unique data, comparison of distance measures, and recording decision-making. Development of online examinations should be oriented towards the same theory of open book examinations.
A series of models are proposed in our literature sample. AbuMansoor (2017) propose to use a series of processes into place to develop examinations that minimize cheating (e.g. question batteries), deploying authentication techniques (e.g. keystrokes and fingerprints), and conduct posthoc assessments to search for cheating. The Aisyah et al. (2018) model identifies two perspectives to conceptualize authentication systems: examinee and admin. From the examinee perspective, points of authentication at the pre-, intra-, and post-examination periods. From the administrative perspective, accessing photographic authentication from pre- and intra-examination periods can be used to validate the examinee. The open book open web (OBOW: Mohanna & Patel, 2016 ) model uses the application of authentic assessment to place the learner in the role of a decision-maker and expert witness, with validation by avoiding any question that could have a generic answer.
The Smart Authenticated Fast Exams (SAFE: Chebrolu et al., 2017 ) model uses application focus (e.g. continuously tracking focus of examinee), logging (phone state, phone identification, and Wi-Fi status), visual password (a password that is visually presented but not easily communicated without photograph), Bluetooth neighborhood logging (to check for nearby devices), ID checks, digitally signed application, random device swap, and the avoidance of ‘bring your own device’ models. The online comprehensive examination (OCE) was used in a National Board Dental Examination to test knowledge in a home environment with 200 multiple choice questions, and the ability to take the test multiple times for formative knowledge development.
Some scholars recommend online synchronous assessments as an alternative to traditional proctored examinations while maintaining the ability to manually authenticate ( Chao et al., 2012 ). In these assessments: quizzes are designed to test factual knowledge, practice for procedural, essay for conceptual, and oral for metacognitive knowledge. A ‘cyber face-to-face’ element is required to enable the validation of students.
3.8. Interface design
The interface of a system will impact on whether a student perceives the environment to be an enabler or barrier for online examinations. Abdel Karim and Shukur (2016) summarized the potential interface design features that emerged from a systematic review of the literature on this topic, as shown in Table 2 . The incorporation of navigation tools has also been identified by students and staff as an essential design feature ( Rios & Liu, 2017 ), as is an auto-save functionality ( Pagram et al., 2018 ).
Potential interface design features ( Abdel Karim & Shukur, 2016 ).
Interface design features | Recommended values | Description |
---|---|---|
Font size | 10, 12, 14, 18, 22, and 26 points | Font size has a significant effect on objective and subjective readability and comprehensibility. |
Font face (type) | Andale Mono, Arial, Arial Black, Comic Sans Ms, Courier New, Georgia, Impact, Times New Roman, Trebuchet Ms, Verdana, and Tahoma | Reading efficiency and reading time are important aspects related to the font type and size. |
Font style | Regular, Italic, Bold, and Bold Italic | |
Text and background colour | Either: | Text and background colour affect text readability and colours, with greater contrast ratio generally lead to greater readability. |
Time counter | Countdown timer, ascending counter and traditional clock | Online examination systems should display the time counter on the screen until the examination time has ended. |
Alert | 5 min (M) remain, 15 M remain, 30 M remain, Mid-exam and No alert | An alert can be used to give attention about remaining examination time. |
3.9. Technology issues
None of the studies that included technological problems in its design reported any issues ( Böhmer et al., 2018 ; Matthíasdóttir & Arnalds, 2016 ; Schmidt et al., 2009 ). One study stated that 5 percent of students reported some problem ranging from a slow system through to the system not working well with the computer operating system, however, the authors stated no technical problems that resulted in the inability to complete the examination were reported ( Matthíasdóttir & Arnalds, 2016 ). In a separate study, students reported that they would prefer to use university technology to complete the examination due to distrust of the system working with their home computer or laptop operating system or the fear of losing data during the examination ( Pagram et al., 2018 ). While the study did not report any problems loading on desktop machines, some student laptops from their workplace had firewalls, and as such had to load the system from a USB.
4. Discussion
This systematic literature review sought to assess the current state of literature concerning online examinations and its equivalents. For most students, online learning environments created a system more supportive of their wellbeing, personal lives, and learning performance. Staff preferred online examinations for their workload implications and ease of completion, and basic evaluation of print-based examination logistics could identify some substantial ongoing cost savings. Not all staff and students preferred the idea of online test environments, yet studies that considered age and gender identified only negligible differences ( Rios & Liu, 2017 ).
While the literature on online examinations is growing, there is still a dearth of discussion at the pedagogical and governance levels. Our review and new familiarity with papers led us to point researchers in two principal directions: accreditation and authenticity. We acknowledge that there are many possible pathways to consider, with reference to the consistency of application, the validity and reliability of online examinations, and whether online examinations enable better measurement and greater student success. There are also opportunities to synthesize online examination literature with other innovative digital pedagogical devices. For example, immersive learning environments ( Herrington et al., 2007 ), mobile technologies ( Jahnke & Liebscher, 2020 ); social media ( Giannikas, 2020 ), and web 2.0 technologies ( Bennett et al., 2012 ). The literature examined acknowledges key elements of the underlying needs for online examinations from student, academic, and technical perspectives. This has included the need for online examinations need to accessible, need to be able to distinguish a true pass from a true fail, secure, minimize opportunities for cheating, accurately authenticates the student, reduce marking time, and designed to be agile in software or technological failure.
We turn attention now to areas of need in future research, and focus on accreditation and authenticity over these alternates given there is a real need for more research prior to synthesis of knowledge on the latter pathways.
4.1. The accreditation question
The influence of external accreditation bodies was named frequently and ominously among the sample group, but lacked clarity surrounding exact parameters and expectations. Rios (2017, p. 231) identified a specific measure was used “for accreditation purposes”. Hylton et al. (2016 , p. 54) specified that the US Department of Education requires “appropriate procedures or technology are implemented” to authentic distance students. Gehringer and Peddycord (2013) empirically found that online/open-web examinations provided more significant data for accreditation. Underlying university decisions to use face-to-face invigilated examination settings is to enable authentication of learning – a requirement of many governing bodies globally. The continual refinement of rules has enabled a degree of assurance that students are who they say they are.
Nevertheless, sophisticated networks have been established globally to support direct student cheating from completing quick assessments and calculators with secret search engine capability through to full completion of a course inclusive of attending on-campus invigilated examinations. The authentication process in invigilated examinations does not typically account for distance students who have a forged student identification card to enable a contract service to complete their examinations. Under the requirement assure authentication of learning, invigilated examinations will require revision to meet contemporary environments. The inclusion of a broader range of big data from keystroke patterns, linguistics analysis, and whole-of-student analytics over a student lifecycle is necessary to identify areas of risk from the institutional perspective. Where a student has a significantly different method of typing or sentence structure, it is necessary to review.
An experimental study on the detection of cheating in a psychology unit found teachers could detect cheating 62 percent of the time ( Dawson & Sutherland-Smith, 2017 ). Automated algorithms could be used to support the pre-identification of this process, given lecturers and professors are unlikely to be explicitly coding for cheating propensity when grading multiple hundreds of papers on the same topic. Future scholars should be considering the innate differences that exist among test-taking behaviors that could be codified to create pattern recognition software. Even in traditional invigilated examinations, the use of linguistics and handwriting evaluations could be used for cheating identification.
4.2. Authentic assessments and examinations
The literature identified in the sample discussed with limited depth the role of authentic assessment in examinations. The evolution of pedagogy and teaching principles (e.g. constructive alignment; Biggs, 1996 ) have paved the way for revised approaches to assessment and student learning. In the case of invigilated examinations, universities have been far slower to progress innovative solutions despite growing evidence that students prefer the flexibility and opportunities afforded by digitalizing exams. University commitments to the development of authentic assessment environments will require a radical revision of current examination practice to incorporate real-life learning processes and unstructured problem-solving ( Williams & Wong, 2009 ). While traditional examinations may be influenced by financial efficacy, accreditation, and authentication pressures, there are upward pressures from student demand, student success, and student wellbeing to create more authentic learning opportunities.
The online examination setting offers greater connectivity to the kinds of environments graduates will be expected to engage in on a regular basis. The development of time management skills to plan times to complete a fixed time examination is reflected in the business student's need to pitch and present at certain times of the day to corporate stakeholders, or a dentist maintaining a specific time allotment for the extraction of a tooth. The completion of a self-regulated task online with tangible performance outcomes is reflected in many roles from lawyer briefs on time-sensitive court cases to high school teacher completions of student reports at the end of a calendar year. Future practitioner implementation and evaluation should be focused on embedding authenticity into the examination setting, and future researchers should seek to understand better the parameters by which online examinations can create authentic learning experiences for students. In some cases, the inclusion of examinations may not be appropriate; and in these cases, they should be progressively extracted from the curriculum.
4.3. Where to next?
As institutions begin to provide higher learning flexibility to students with digital and blended offerings, there is scholarly need to consider the efficacy of the examination environment associated with these settings. Home computers and high-speed internet are becoming commonplace ( Rainie & Horrigan, 2005 ), recognizing that such an assumption has implications for student equity. As Warschauer (2007 , p. 41) puts it, “the future of learning is digital”. Our ability as educators will be in seeking to understand how we can create high impact learning opportunities while responding to an era of digitalization. Research considering digital fluency in students will be pivotal ( Crawford & Butler-Henderson, 2020 ). Important too, is the scholarly imperative to examine the implementation barriers and successes associated with online examinations in higher education institutions given the lack of clear cross-institutional case studies. There is also a symbiotic question that requires addressing by scholars in our field, beginning with understanding how online examinations can enable higher education, and likewise how higher education can shape and inform the implementation and delivery of online examinations.
4.4. Limitations
This study adopted a rigorous PRISMA method for preliminary identification of papers for inclusion, the MMAT protocol for identifying the quality of papers, and an inductive thematic analysis for analyzing papers included. These processes respond directly to limitations of subjectivity and assurance of breadth and depth of literature. However, the systematic literature review method limits the papers included by the search criteria used. While we opted for a broad set of terms, it is possible we missed papers that would typically have been identified in other manual and critical identification processes. The lack of research published provided a substantial opportunity to develop a systematic literature review to summarize the state of the evidence, but the availability of data limits each comment. A meta-analysis on quantitative research in this area of study would be complicated because of the lack of replication. Indeed, our ability to unpack which institutions currently use online examinations (and variants thereof) relied on scholars publishing on such implementations; many of which have not. The findings of this systematic literature review are also limited by the lack of replication in this infant field. The systematic literature review was, in our opinions, the most appropriate method to summarize the current state of literature despite the above limitations and provides a strong foundation for an evidence-based future of online examinations. We also acknowledge the deep connection that this research may have in relation to the contemporary COVID-19 climate in higher education, with many universities opting for online forms of examinations to support physically distanced education and emergency remote teaching. There were 138 publications on broad learning and teaching topics during the first half of 2020 ( Butler-Henderson et al., 2020 ). Future research may consider how this has changed or influenced the nature of rapid innovation for online examinations.
5. Conclusion
This systematic literature review considered the contemporary literature on online examinations and their equivalents. We discussed student, staff, and technological research as it was identified in our sample. The dominant focus of the literature is still oriented on preliminary evaluations of implementation. These include what processes changed at a technological level, and how students and staff rated their preferences. There were some early attempts to explore the effect of online examinations on student wellbeing and student performance, along with how the changes affect the ability for staff to achieve.
Higher education needs this succinct summary of the literature on online examinations to understand the barriers and how they can be overcome, encouraging greater uptake of online examinations in tertiary education. One of the largest barriers is perceptions of using online examinations. Once students have experienced online examinations, there is a preference for this format due to its ease of use. The literature reported student performance did not have significant difference in final examination scores across online and traditional examination modalities. Student anxiety decreased once they had used the online examination software. This information needs to be provided to students to change students’ perceptions and decrease anxiety when implementing an online examination system. Similarly, the information summarized in this paper needs to be provided to staff, such as the data related to cheating, reliability of the technology, ease of use, and reduction in time for establishing and marking examinations. When selecting a system, institutions should seek one that includes biometrics with a high level of precision, such as user authentication, and movement, sound, and keystroke monitoring (reporting deviations so the recording can be reviewed). These features reduce the need for online examinations to be invigilated. Other system features should include locking the system or browser, cloud-based technology so local updates are not required, and an interface design that makes using the online examination intuitive. Institutions should also consider how it will address technological failures and digital disparities, such as literacy and access to technology.
We recognize the need for substantially more evidence surrounding the post-implementation stages of online examinations. The current use of online examinations across disciplines, institutions, and countries needs to be examined to understand the successes and gaps. Beyond questions of ‘do students prefer online or on-campus exams’, serious questions of how student mental wellbeing, employability, and achievement of learning outcomes can be improved as a result of an online examination pedagogy is critical. In conjunction is the need to break down the facets and types of digitally enhanced examinations (e.g. online, e-examination, BYOD examinations, and similar) and compare each of these for their respective efficacy in enabling student success against institutional implications. While this paper was only able to capture the literature that does exist, we believe the next stage of literature needs to consider broader implications than immediate student perceptions toward the achievement of institutional strategic imperatives that may include student wellbeing, student success, student retention, financial viability, staff enrichment, and student employability.
Author statement
Both authors Kerryn Butler-Henderson and Joseph Crawford contributed to the design of this study, literature searches, data abstraction and cleaning, data analysis, and development of this manuscript. All contributions were equal.
This research did not receive any specific grant from funding agencies in the public, commercial, or not-for-profit sectors.
- Abdel Karim N., Shukur Z. Proposed features of an online examination interface design and its optimal values. Computers in Human Behavior. 2016; 64 :414–422. doi: 10.1016/j.chb.2016.07.013. [ CrossRef ] [ Google Scholar ]
- AbuMansour H. 2017 IEEE/ACS 14th international conference on computer systems and applications (AICCSA) 2017. Proposed bio-authentication system for question bank in learning management systems; pp. 489–494. [ CrossRef ] [ Google Scholar ]
- Aisyah S., Bandung Y., Subekti L.B. 2018 international conference on information technology systems and innovation (ICITSI) 2018. Development of continuous authentication system on android-based online exam application; pp. 171–176. [ CrossRef ] [ Google Scholar ]
- Al-Hakeem M.S., Abdulrahman M.S. Developing a new e-exam platform to enhance the university academic examinations: The case of Lebanese French University. International Journal of Modern Education and Computer Science. 2017; 9 (5):9. doi: 10.5815/ijmecs.2017.05.02. [ CrossRef ] [ Google Scholar ]
- Alzu'bi M. Proceedings of conference of the international journal of arts & sciences. 2015. The effect of using electronic exams on students' achievement and test takers' motivation in an English 101 course; pp. 207–215. [ Google Scholar ]
- Amigud A., Dawson P. The law and the outlaw: is legal prohibition a viable solution to the contract cheating problem? Assessment & Evaluation in Higher Education. 2020; 45 (1):98–108. doi: 10.1080/02602938.2019.1612851. [ CrossRef ] [ Google Scholar ]
- Anderson H.M., Cain J., Bird E. Online course evaluations: Review of literature and a pilot study. American Journal of Pharmaceutical Education. 2005; 69 (1):34–43. doi: 10.5688/aj690105. [ CrossRef ] [ Google Scholar ]
- Ardid M., Gómez-Tejedor J.A., Meseguer-Dueñas J.M., Riera J., Vidaurre A. Online exams for blended assessment. Study of different application methodologies. Computers & Education. 2015; 81 :296–303. doi: 10.1016/j.compedu.2014.10.010. [ CrossRef ] [ Google Scholar ]
- Attia M. Postgraduate students' perceptions toward online assessment: The case of the faculty of education, Umm Al-Qura university. In: Wiseman A., Alromi N., Alshumrani S., editors. Education for a knowledge society in Arabian Gulf countries. Emerald Group Publishing Limited; Bingley, United Kingdom: 2014. pp. 151–173. [ CrossRef ] [ Google Scholar ]
- Bennett S., Bishop A., Dalgarno B., Waycott J., Kennedy G. Implementing web 2.0 technologies in higher education: A collective case study. Computers & Education. 2012; 59 (2):524–534. [ Google Scholar ]
- Biggs J. Enhancing teaching through constructive alignment. Higher Education. 1996; 32 (3):347–364. doi: 10.1007/bf00138871. [ CrossRef ] [ Google Scholar ]
- Böhmer C., Feldmann N., Ibsen M. 2018 IEEE global engineering education conference (EDUCON) 2018. E-exams in engineering education—online testing of engineering competencies: Experiences and lessons learned; pp. 571–576. [ CrossRef ] [ Google Scholar ]
- Botezatu M., Hult H., Tessma M.K., Fors U.G. Virtual patient simulation for learning and assessment: Superior results in comparison with regular course exams. Medical Teacher. 2010; 32 (10):845–850. doi: 10.3109/01421591003695287. [ PubMed ] [ CrossRef ] [ Google Scholar ]
- Braun V., Clarke V. Using thematic analysis in psychology. Qualitative Research in Psychology. 2006; 3 (2):77–101. doi: 10.1191/1478088706qp063oa. [ CrossRef ] [ Google Scholar ]
- Butler-Henderson K., Crawford J., Rudolph J., Lalani K., Sabu K.M. COVID-19 in Higher Education Literature Database (CHELD V1): An open access systematic literature review database with coding rules. Journal of Applied Learning and Teaching. 2020; 3 (2) doi: 10.37074/jalt.2020.3.2.11. Advanced Online Publication. [ CrossRef ] [ Google Scholar ]
- Butt A. Quantification of influences on student perceptions of group work. Journal of University Teaching and Learning Practice. 2018; 15 (5) [ Google Scholar ]
- Chao K.J., Hung I.C., Chen N.S. On the design of online synchronous assessments in a synchronous cyber classroom. Journal of Computer Assisted Learning. 2012; 28 (4):379–395. doi: 10.1111/j.1365-2729.2011.00463.x. [ CrossRef ] [ Google Scholar ]
- Chebrolu K., Raman B., Dommeti V.C., Boddu A.V., Zacharia K., Babu A., Chandan P. Proceedings of the 2017 ACM SIGCSE technical symposium on computer science education. 2017. Safe: Smart authenticated Fast exams for student evaluation in classrooms; pp. 117–122. [ CrossRef ] [ Google Scholar ]
- Chen Q. Proceedings of ACM turing celebration conference-China. 2018. An application of online exam in discrete mathematics course; pp. 91–95. [ CrossRef ] [ Google Scholar ]
- Chytrý V., Nováková A., Rícan J., Simonová I. 2018 international symposium on educational technology (ISET) 2018. Comparative analysis of online and printed form of testing in scientific reasoning and metacognitive monitoring; pp. 13–17. [ CrossRef ] [ Google Scholar ]
- Crawford J. University of Tasmania, Australia: Honours Dissertation; 2015. Authentic leadership in student leaders: An empirical study in an Australian university. [ Google Scholar ]
- Crawford J., Butler-Henderson K. Digitally empowered workers and authentic leaders: The capabilities required for digital services. In: Sandhu K., editor. Leadership, management, and adoption techniques for digital service innovation. IGI Global; Hershey, Pennsylvania: 2020. pp. 103–124. [ CrossRef ] [ Google Scholar ]
- Crawford J., Butler-Henderson K., Rudolph J., Malkawi B., Glowatz M., Burton R., Magni P., Lam S. COVID-19: 20 countries' higher education intra-period digital pedagogy responses. Journal of Applied Teaching and Learning. 2020; 3 (1):9–28. doi: 10.37074/jalt.2020.3.1.7. [ CrossRef ] [ Google Scholar ]
- Creswell J., Miller D. Determining validity in qualitative inquiry. Theory into Practice. 2000; 39 (3):124–130. doi: 10.1207/s15430421tip3903_2. [ CrossRef ] [ Google Scholar ]
- Daffin L., Jr., Jones A. Comparing student performance on proctored and non-proctored exams in online psychology courses. Online Learning. 2018; 22 (1):131–145. doi: 10.24059/olj.v22i1.1079. [ CrossRef ] [ Google Scholar ]
- Dawson P. Five ways to hack and cheat with bring‐your‐own‐device electronic examinations. British Journal of Educational Technology. 2016; 47 (4):592–600. doi: 10.1111/bjet.12246. [ CrossRef ] [ Google Scholar ]
- Dawson P., Sutherland-Smith W. Can markers detect contract cheating? Results from a pilot study. Assessment & Evaluation in Higher Education. 2018; 43 (2):286–293. doi: 10.1080/02602938.2017.1336746. [ CrossRef ] [ Google Scholar ]
- Dawson P., Sutherland-Smith W. Can training improve marker accuracy at detecting contract cheating? A multi-disciplinary pre-post study. Assessment & Evaluation in Higher Education. 2019; 44 (5):715–725. doi: 10.1080/02602938.2018.1531109. [ CrossRef ] [ Google Scholar ]
- Eagly A., Sczesny S. Stereotypes about women, men, and leaders: Have times changed? In: Barreto M., Ryan M.K., Schmitt M.T., editors. Psychology of women book series. The glass ceiling in the 21st century: Understanding barriers to gender equality. American Psychological Association; 2009. pp. 21–47. [ CrossRef ] [ Google Scholar ]
- Ellis S., Barber J. Expanding and personalizing feedback in online assessment: A case study in a school of pharmacy. Practitioner Research in Higher Education. 2016; 10 (1):121–129. [ Google Scholar ]
- Fluck A. An international review of eExam technologies and impact. Computers & Education. 2019; 132 :1–15. doi: 10.1016/j.compedu.2018.12.008. [ CrossRef ] [ Google Scholar ]
- Fluck A., Adebayo O.S., Abdulhamid S.I.M. Secure e-examination systems compared: Case studies from two countries. Journal of Information Technology Education: Innovations in Practice. 2017; 16 :107–125. doi: 10.28945/3705. [ CrossRef ] [ Google Scholar ]
- Fluck A., Pullen D., Harper C. Case study of a computer based examination system. Australasian Journal of Educational Technology. 2009; 25 (4):509–533. doi: 10.14742/ajet.1126. [ CrossRef ] [ Google Scholar ]
- Gehringer E., Peddycord B., III Experience with online and open-web exams. Journal of Instructional Research. 2013; 2 :10–18. doi: 10.9743/jir.2013.2.12. [ CrossRef ] [ Google Scholar ]
- Giannikas C. Facebook in tertiary education: The impact of social media in e-learning. Journal of University Teaching and Learning Practice. 2020; 17 (1):3. [ Google Scholar ]
- Gold S.S., Mozes-Carmel A. A comparison of online vs. proctored final exams in online classes. Journal of Educational Technology. 2009; 6 (1):76–81. doi: 10.26634/jet.6.1.212. [ CrossRef ] [ Google Scholar ]
- Gross J., Torres V., Zerquera D. Financial aid and attainment among students in a state with changing demographics. Research in Higher Education. 2013; 54 (4):383–406. doi: 10.1007/s11162-012-9276-1. [ CrossRef ] [ Google Scholar ]
- Guillén-Gámez F.D., García-Magariño I., Bravo J., Plaza I. Exploring the influence of facial verification software on student academic performance in online learning environments. International Journal of Engineering Education. 2015; 31 (6A):1622–1628. [ Google Scholar ]
- Hainline L., Gaines M., Feather C.L., Padilla E., Terry E. Changing students, faculty, and institutions in the twenty-first century. Peer Review. 2010; 12 (3):7–10. [ Google Scholar ]
- Hearn Moore P., Head J.D., Griffin R.B. Impeding students' efforts to cheat in online classes. Journal of Learning in Higher Education. 2017; 13 (1):9–23. [ Google Scholar ]
- Herrington J., Reeves T.C., Oliver R. Immersive learning technologies: Realism and online authentic learning. Journal of Computing in Higher Education. 2007; 19 (1):80–99. [ Google Scholar ]
- Hong Q.N., Fàbregues S., Bartlett G., Boardman F., Cargo M., Dagenais P., Gagnon M.P., Griffiths F., Nicolau B., O'Cathain A., Rousseau M.C., Vedel I., Pluye P. The mixed methods appraisal tool (MMAT) version 2018 for information professionals and researchers. Education for Information. 2018; 34 (4):285–291. doi: 10.3233/EFI-180221. [ CrossRef ] [ Google Scholar ]
- Hylton K., Levy Y., Dringus L.P. Utilizing webcam-based proctoring to deter misconduct in online exams. Computers & Education. 2016; 92 :53–63. doi: 10.1016/j.compedu.2015.10.002. [ CrossRef ] [ Google Scholar ]
- Jahnke I., Liebscher J. Three types of integrated course designs for using mobile technologies to support creativity in higher education. Computers & Education. 2020; 146 doi: 10.1016/j.compedu.2019.103782. Advanced Online Publication. [ CrossRef ] [ Google Scholar ]
- Johnson C. 2009. History of New York state regents exams. Unpublished manuscript. [ Google Scholar ]
- Jordan A. College student cheating: The role of motivation, perceived norms, attitudes, and knowledge of institutional policy. Ethics & Behavior. 2001; 11 (3):233–247. doi: 10.1207/s15327019eb1103_3. [ CrossRef ] [ Google Scholar ]
- Karp A. Exams in algebra in Russia: Toward a history of high stakes testing. International Journal for the History of Mathematics Education. 2007; 2 (1):39–57. [ Google Scholar ]
- Kemp D. Australian Government Printing Service; Canberra: 1999. Knowledge and innovation: A policy statement on research and research training. [ Google Scholar ]
- Kolagari S., Modanloo M., Rahmati R., Sabzi Z., Ataee A.J. The effect of computer-based tests on nursing students' test anxiety: A quasi-experimental study. Acta Informatica Medica. 2018; 26 (2):115. doi: 10.5455/aim.2018.26.115-118. [ PMC free article ] [ PubMed ] [ CrossRef ] [ Google Scholar ]
- Kolski T., Weible J. Examining the relationship between student test anxiety and webcam based exam proctoring. Online Journal of Distance Learning Administration. 2018; 21 (3):1–15. [ Google Scholar ]
- Kumar A. 2014 IEEE Frontiers in Education Conference (FIE) Proceedings. 2014. Test anxiety and online testing: A study; pp. 1–6. [ CrossRef ] [ Google Scholar ]
- Li X., Chang K.M., Yuan Y., Hauptmann A. Proceedings of the 18th ACM conference on computer supported cooperative work & social computing. 2015. Massive open online proctor: Protecting the credibility of MOOCs certificates; pp. 1129–1137. [ CrossRef ] [ Google Scholar ]
- Lincoln Y., Guba E. Sage Publications; California: 1985. Naturalistic inquiry. [ Google Scholar ]
- Margaryan A., Littlejohn A., Vojt G. Are digital natives a myth or reality? University students' use of digital technologies. Computers & Education. 2011; 56 (2):429–440. doi: 10.1016/j.compedu.2010.09.004. [ CrossRef ] [ Google Scholar ]
- Matthíasdóttir Á., Arnalds H. Proceedings of the 17th international conference on computer systems and technologies 2016. 2016. e-assessment: students' point of view; pp. 369–374. [ CrossRef ] [ Google Scholar ]
- Mitra S., Gofman M. Proceedings of the twenty-second americas conference on information systems (28) 2016. Towards greater integrity in online exams. [ Google Scholar ]
- Mohanna K., Patel A. 2015 fifth international conference on e-learning. 2015. Overview of open book-open web exam over blackboard under e-Learning system; pp. 396–402. [ CrossRef ] [ Google Scholar ]
- Moher D., Liberati A., Tetzlaff J., Altman D.G. Preferred reporting items for systematic reviews and meta-analyses: The PRISMA statement. Annals of Internal Medicine. 2009; 151 (4) doi: 10.7326/0003-4819-151-4-200908180-00135. 264-249. [ PubMed ] [ CrossRef ] [ Google Scholar ]
- Mueller R.G., Colley L.M. An evaluation of the impact of end-of-course exams and ACT-QualityCore on US history instruction in a Kentucky high school. Journal of Social Studies Research. 2015; 39 (2):95–106. doi: 10.1016/j.jssr.2014.07.002. [ CrossRef ] [ Google Scholar ]
- Nguyen H., Henderson A. Can the reading load Be engaging? Connecting the instrumental, critical and aesthetic in academic reading for student learning. Journal of University Teaching and Learning Practice. 2020; 17 (2):6. [ Google Scholar ]
- NYSED . 2012. History of regent examinations: 1865 – 1987. Office of state assessment. http://www.p12.nysed.gov/assessment/hsgen/archive/rehistory.htm [ Google Scholar ]
- Oz H., Ozturan T. Computer-based and paper-based testing: Does the test administration mode influence the reliability and validity of achievement tests? Journal of Language and Linguistic Studies. 2018; 14 (1):67. [ Google Scholar ]
- Pagram J., Cooper M., Jin H., Campbell A. Tales from the exam room: Trialing an e-exam system for computer education and design and technology students. Education Sciences. 2018; 8 (4):188. doi: 10.3390/educsci8040188. [ CrossRef ] [ Google Scholar ]
- Park S. Proceedings of the 21st world multi-conference on systemics, cybernetics and informatics. WMSCI 2017; 2017. Online exams as a formative learning tool in health science education; pp. 281–282. [ Google Scholar ]
- Patel A.A., Amanullah M., Mohanna K., Afaq S. Third international conference on e-technologies and networks for development. ICeND2014; 2014. E-exams under e-learning system: Evaluation of onscreen distraction by first year medical students in relation to on-paper exams; pp. 116–126. [ CrossRef ] [ Google Scholar ]
- Petrović J., Vitas D., Pale P. 2017 international symposium ELMAR. 2017. Experiences with supervised vs. unsupervised online knowledge assessments in formal education; pp. 255–258. [ CrossRef ] [ Google Scholar ]
- Rainie L., Horrigan J. Pew Internet & American Life Project; Washington, DC: 2005. A decade of adoption: How the internet has woven itself into American life. [ Google Scholar ]
- Reiko Y. University reform in the post-massification era in Japan: Analysis of government education policy for the 21st century. Higher Education Policy. 2001; 14 (4):277–291. doi: 10.1016/s0952-8733(01)00022-8. [ CrossRef ] [ Google Scholar ]
- Rettinger D.A., Kramer Y. Situational and personal causes of student cheating. Research in Higher Education. 2009; 50 (3):293–313. doi: 10.1007/s11162-008-9116-5. [ CrossRef ] [ Google Scholar ]
- Rios J.A., Liu O.L. Online proctored versus unproctored low-stakes internet test administration: Is there differential test-taking behavior and performance? American Journal of Distance Education. 2017; 31 (4):226–241. doi: 10.1080/08923647.2017.1258628. [ CrossRef ] [ Google Scholar ]
- Rodchua S., Yiadom-Boakye G., Woolsey R. Student verification system for online assessments: Bolstering quality and integrity of distance learning. Journal of Industrial Technology. 2011; 27 (3) [ Google Scholar ]
- Schmidt S.M., Ralph D.L., Buskirk B. Utilizing online exams: A case study. Journal of College Teaching & Learning (TLC) 2009; 6 (8) doi: 10.19030/tlc.v6i8.1108. [ CrossRef ] [ Google Scholar ]
- Schwalb S.J., Sedlacek W.E. Have college students' attitudes toward older people changed. Journal of College Student Development. 1990; 31 (2):125–132. [ Google Scholar ]
- Seow T., Soong S. Proceedings of the australasian society for computers in learning in tertiary education, Dunedin. 2014. Students' perceptions of BYOD open-book examinations in a large class: A pilot study; pp. 604–608. [ Google Scholar ]
- Sheppard S. An informal history of how law schools evaluate students, with a predictable emphasis on law school final exams. UMKC Law Review. 1996; 65 :657. [ Google Scholar ]
- Sindre G., Vegendla A. NIK: Norsk Informatikkonferanse (n.p.) 2015, November. E-exams and exam process improvement. [ Google Scholar ]
- Steel A., Moses L.B., Laurens J., Brady C. Use of e-exams in high stakes law school examinations: Student and staff reactions. Legal Education Review. 2019; 29 (1):1. [ Google Scholar ]
- Stowell J.R., Bennett D. Effects of online testing on student exam performance and test anxiety. Journal of Educational Computing Research. 2010; 42 (2):161–171. doi: 10.2190/ec.42.2.b. [ CrossRef ] [ Google Scholar ]
- Sullivan D.P. An integrated approach to preempt cheating on asynchronous, objective, online assessments in graduate business classes. Online Learning. 2016; 20 (3):195–209. doi: 10.24059/olj.v20i3.650. [ CrossRef ] [ Google Scholar ]
- Turner J.L., Dankoski M.E. Objective structured clinical exams: A critical review. Family Medicine. 2008; 40 (8):574–578. [ PubMed ] [ Google Scholar ]
- US National Library of Medicine . 2019. Medical subject headings. https://www.nlm.nih.gov/mesh/meshhome.html [ Google Scholar ]
- Vision Australia . 2014. Online and print inclusive design and legibility considerations. Vision Australia. https://www.visionaustralia.org/services/digital-access/blog/12-03-2014/online-and-print-inclusive-design-and-legibility-considerations [ Google Scholar ]
- Warschauer M. The paradoxical future of digital learning. Learning Inquiry. 2007; 1 (1):41–49. doi: 10.1007/s11519-007-0001-5. [ CrossRef ] [ Google Scholar ]
- Wibowo S., Grandhi S., Chugh R., Sawir E. A pilot study of an electronic exam system at an Australian University. Journal of Educational Technology Systems. 2016; 45 (1):5–33. doi: 10.1177/0047239516646746. [ CrossRef ] [ Google Scholar ]
- Williams J.B., Wong A. The efficacy of final examinations: A comparative study of closed‐book, invigilated exams and open‐book, open‐web exams. British Journal of Educational Technology. 2009; 40 (2):227–236. doi: 10.1111/j.1467-8535.2008.00929.x. [ CrossRef ] [ Google Scholar ]
- Wright T.A. Distinguished Scholar Invited Essay: Reflections on the role of character in business education and student leadership development. Journal of Leadership & Organizational Studies. 2015; 22 (3):253–264. doi: 10.1177/1548051815578950. [ CrossRef ] [ Google Scholar ]
- Yong-Sheng Z., Xiu-Mei F., Ai-Qin B. 2015 7th international conference on information technology in medicine and education (ITME) 2015. The research and design of online examination system; pp. 687–691. [ CrossRef ] [ Google Scholar ]

IMAGES
COMMENTS
This systematic literature review sought to assess the current state of literature concerning online examinations and its equivalents. For most students, online learning environments created a system more supportive of their wellbeing, personal lives, and learning performance.
In this article, a Systematic Literature Review (SLR) of online examination is performed to select and analyze 53 studies published during the last five years (i.e. Jan 2016 to July 2020).
During the online assessment, evaluation of the learning outcomes presents challenges mainly due to academic dishonesty among students that may lead to unfair evaluations. This systematic review examines the research on online assessment security involving studies completed between 2016 and 2021.
Finally, the participation of countries in online exam research is investigated. Key factors for the global adoption of online exams are identified and compared with major online exams features. This facilitates the selection of right online exam system for a particular country on the basis of existing E-learning infrastructure and overall cost.
Below is a literature review on online examination systems with references: Online examination systems have gained prominence in recent years due to the increasing demand for flexible and convenient assessment methods.
However, to the best of our knowledge, there is a lack of systematic review of the literature addressing online exams from students' perspectives and summarizing the findings regarding students' acceptance and/or preferences of online exams.
The online examination system is a digital platform that evaluates students in a hassle-free way. The entire examination process is simplified, and exams are taken from anywhere, anytime. Eventually, with online examinations becoming the new normal, protecting the integrity of exam and exam data are becoming key areas of concern too.
Finally, the participation of countries in online exam research is investigated. Key factors for the global adoption of online exams are identi ed and compared with major online exams features. This facilitates the selection of right online exam system for a par- ticular country on the basis of existing E-learning infrastructure and overall cost.
This paper aims to provide a systematic literature review (SLR) on automated essay grading systems. An SLR is an Evidence-based systematic review to summarize the existing research. It critically evaluates and integrates all relevant studies' findings and addresses the research domain's specific research questions.
2. Literature Review Online examination system allows an institute to arrange, conduct, and manage examinations via an online environment. It assists the inspector with reducing the work of leading exam, checking answer sheets, and producing result [ 14 ]. So, online exams have gained a lot of popularity in the past few years. Okada et al. [ 15] pointed out that although a lot of young ...
Finally, the participation of countries in online exam research is investigated. Key factors for the global adoption of online exams are identified and investigated. This facilitates the selection of right online exam system for a particular country on the basis of existing E-learning infrastructure and overall cost.
The purpose of this study was to assess, through a systematic review, students' views about online examination practices in universities. Examination of students learning is a key component in ...
However, as the pandemic spread, it became more urgent to switch to an online exam format or another type of evaluation. In an effort to assemble knowledge about this newly developed methodology in higher learning, this study explores recent literature relevant to online exams in a university environment.
Online examination system is a web-based examination system where examinations are given online. Either through the internet or intranet using computer system.
This systematic literature review sought to assess the current state of literature concerning online examinations and its equivalents. For most students, online learning environments created a system more supportive of their wellbeing, personal lives, and learning performance.
This study was made to gather literacy studies related to the use of online testing system. It is intended to evaluate the factors that attract users to utilize online testing system.
ticipation of countries in online exam research is investigated. Key factors for the global adoption of online exams are identi ed and compared with major online exams features. This facilitates the selection of right online exam system for a particular country on the basis of existing E-learning infrastructure and overall cost.
The Online examination or assessment system is the method of organizing the exams which doesn't require any kind of a piece of paper or a pen. Exam organizers or examiners can use online examination platforms to conduct examinations using the internet for candidates which are not able to showing up physically.
This systematic literature review sought to assess the current state of literature concerning online examinations and its equivalents. For most students, online learning environments created a system more supportive of their wellbeing, personal lives, and learning performance.
The scientific literature on bifacial solar photovoltaic system design, modelling, performance, and application is the subject of the systematic literature review. The data and information are derived from studies and reports conducted around the world from 2010 to 2022.