- DOI: 10.4236/OALIB.1107423
- Corpus ID: 236395944

A Literature Review of Academic Performance, an Insight into Factors and their Influences on Academic Outcomes of Students at Senior High Schools
- E. Brew , Benjamin Nketiah , R. Koranteng
- Published in OALib 1 June 2021
24 Citations
Key influences on students' academic success: insights from scholarly research, relationship between teachers’ attitude towards curriculum and students’ academic achievement at secondary school level, exploring college academic performance of k to12 it and non-it-related strands to reduce academic deficiencies, an assessment of teacher motivation in determining academic performance of secondary school learners in kenya, parental involvement and students' engagement in modular learning environments, factors affecting learners’ academic performance in selected districts, division of gingoog city, the influence of social support on academic performance: the mediating role of cognitive engagement, teaching performance, student self-efficacy and creativity fostering teacher: a structural model on academic success of students, exploring the impact of quality education management on pupils’ academic performance. a case study of basic schools in ghana, relationship between digital capabilities and academic performance: the mediating effect of self-efficacy, 77 references, library use and students academic achievement: implication for counseling, what factors determine academic achievement in high achieving undergraduate medical students a qualitative study, students’ views about secondary school science lessons: the role of practical work, factors affecting female students' academic achievement at bahir dar university (special issue : study results of the africa-asia university dialogue for educational development network second phase), effects of availability and use of laboratories on students performance in science subjects in community secondary schools, e-textbooks usage by students at andrews university: a study of attitudes, perceptions, and behaviors, students’ failure in academic environment☆, e‐textbooks and students’ learning experiences, homework type, parental occupational status and academic performance of primary school pupils in english and mathematics in ijebu north local government, ogun state, nigeria, textbook research in mathematics education: development status and directions, related papers.
Showing 1 through 3 of 0 Related Papers

An official website of the United States government
The .gov means it’s official. Federal government websites often end in .gov or .mil. Before sharing sensitive information, make sure you’re on a federal government site.
The site is secure. The https:// ensures that you are connecting to the official website and that any information you provide is encrypted and transmitted securely.
- Publications
- Account settings
Preview improvements coming to the PMC website in October 2024. Learn More or Try it out now .
- Advanced Search
- Journal List
- Springer Nature - PMC COVID-19 Collection

Analysing the Impact of Social Media on Students’ Academic Performance: A Comparative Study of Extraversion and Introversion Personality
Sourabh sharma.
International Management Institute (IMI), Bhubaneswar, India
Ramesh Behl
Associated data.
Complete data and material is available to support transparency.
The advent of technology in education has seen a revolutionary change in the teaching–learning process. Social media is one such invention which has a major impact on students’ academic performance. This research analyzed the impact of social media on the academic performance of extraversion and introversion personality students. Further, the comparative study between these two personalities will be analysed on education level (postgraduate and undergraduate) and gender (male and female). The research was initiated by identifying the factors of social media impacting students’ academic performance. Thereafter, the scale was developed, validated and tested for reliability in the Indian context. Data were collected from 408 students segregated into 202 males and 206 females. Two hundred and thirty-four students are enrolled in postgraduation courses, whereas 174 are registered in the undergraduate programme. One-way ANOVA has been employed to compare the extraversion and introversion students of different education levels and gender. A significant difference is identified between extraversion and introversion students for the impact of social media on their academic performance.
Introduction
Social Networking Sites (SNS) gained instant popularity just after the invention and expansion of the Internet. Today, these sites are used the most to communicate and spread the message. The population on these social networking sites (SNS) has increased exponentially. Social networking sites (SNS) in general are called social media (Boyd & Ellison, 2008 ). Social media (SM) is used extensively to share content, initiate discussion, promote businesses and gain advantages over traditional media. Technology plays a vital role to make SM more robust by reducing security threats and increasing reliability (Stergiou et al., 2018 ).
As of January 2022, more than 4.95 billion people are using the Internet worldwide, and around 4.62 billion are active SM users (Johnson, 2022 ). In India, the number of Internet users was 680 million by January 2022, and there were 487 million active social media users (Basuray, 2022 ). According to Statista Research Department ( 2022 ), in India, SM is dominated by two social media sites, i.e. YouTube and Facebook. YouTube has 467 million users followed by Facebook with 329 million users.
Although almost all age groups are using SM platforms to interact and communicate with their known community (Whiting & Williams, 2013 ), it has been found that social media sites are more popular among youngsters and specifically among students. They use SM for personal as well as academic activities extensively (Laura et al., 2017 ). Other than SM, from the last two years, several online platforms such as Microsoft Teams, Zoom and Google Meet are preferred to organize any kind of virtual meetings, webinars and online classes. These platforms were used worldwide to share and disseminate knowledge across the defined user community during the pandemic. Social media sites such as Facebook, YouTube, Instagram, WhatsApp and blogs are comparatively more open and used to communicate with public and/or private groups. Earlier these social media platforms were used only to connect with friends and family, but gradually these platforms became one of the essential learning tools for students (Park et al., 2009 ). To enhance the teaching–learning process, these social media sites are explored by all types of learning communities (Dzogbenuku et al., 2019 ). SM when used in academics has both advantages and disadvantages. Social media helps to improve academic performance, but it may also distract the students from studies and indulge them in other non-academic activities (Alshuaibi et al., 2018 ).
Here, it is important to understand that the personality traits of students, their education level and gender are critical constructs to determine academic performance. There are different personality traits of an individual such as openness, conscientiousness, extraversion and introversion, agreeableness and neuroticism (McCrae & Costa, 1987 ). This cross-functional research is an attempt to study the impact of social media on the academic performance of students while using extraversion and introversion personality traits, education levels and gender as moderating variables.
Literature Review
There has been a drastic change in the internet world due to the invention of social media sites in the last ten years. People of all age groups now share their stories, feelings, videos, pictures and all kinds of public stuff on social media platforms exponentially (Asur & Huberman, 2010 ). Youth, particularly from the age group of 16–24, embraced social media sites to connect with their friends and family, exchange information and showcase their social status (Boyd & Ellison, 2008 ). Social media sites have many advantages when used in academics. The fun element of social media sites always helps students to be connected with peers and teachers to gain knowledge (Amin et al., 2016 ). Social media also enhances the communication between teachers and students as this are no ambiguity and miscommunication from social media which eventually improves the academic performance of the students (Oueder & Abousaber, 2018 ).
When social media is used for educational purposes, it may improve academic performance, but some associated challenges also come along with it (Rithika & Selvaraj, 2013 ). If social media is incorporated into academics, students try to also use it for non-academic discussions (Arnold & Paulus, 2010 ). The primary reason for such distraction is its design as it is designed to be a social networking tool (Qiu et al., 2013 ). According to Englander et al. ( 2010 ), the usage of social media in academics has more disadvantages than advantages. Social media severely impacts the academic performance of a student. The addiction to social media is found more among the students of higher studies which ruins the academic excellence of an individual (Nalwa & Anand, 2003 ). Among the social media users, Facebook users’ academic performance was worse than the nonusers or users of any other social media network. Facebook was found to be the major distraction among students (Kirschner & Karpinski, 2010 ). However, other studies report contrary findings and argued that students benefited from chatting (Jain et al., 2012 ), as it improves their vocabulary and writing skills (Yunus & Salehi, 2012 ). Social media can be used either to excel in academics or to devastate academics. It all depends on the way it is used by the students. The good or bad use of social media in academics is the users’ decision because both the options are open to the students (Landry, 2014 ).
Kaplan and Haenlein ( 2010 ) defined social media as user-generated content shared on web 2.0. They have also classified social media into six categories:
- Social Networking Sites: Facebook, Twitter, LinkedIn and Instagram are the social networking sites where a user may create their profile and invite their friends to join. Users may communicate with each other by sharing common content.
- Blogging Sites: Blogging sites are individual web pages where users may communicate and share their knowledge with the audience.
- Content Communities and Groups: YouTube and Slideshare are examples of content communities where people may share media files such as pictures, audio and video and PPT presentations.
- Gaming Sites: Users may virtually participate and enjoy the virtual games.
- Virtual Worlds: During COVID-19, this type of social media was used the most. In the virtual world, users meet with each other at some decided virtual place and can do the pre-decided things together. For example, the teacher may decide on a virtual place of meeting, and students may connect there and continue their learning.
- Collaborative Content Sites: Wikipedia is an example of a collaborative content site. It permits many users to work on the same project. Users have all rights to edit and add the new content to the published project.
Massive open online courses (MOOCs) are in trend since 2020 due to the COVID-19 pandemic (Raja & Kallarakal, 2020 ). MOOCs courses are generally free, and anyone may enrol for them online. Many renowned institutions have their online courses on MOOCs platform which provides a flexible learning opportunity to the students. Students find them useful to enhance their knowledge base and also in career development. Many standalone universities have collaborated with the MOOCs platform and included these courses in their curriculum (Chen, 2013 ).
Security and privacy are the two major concerns associated with social media. Teachers are quite apprehensive in using social media for knowledge sharing due to the same concerns (Fedock et al., 2019 ). It was found that around 72% teachers were reluctant to use social media platforms due to integrity issues and around 63% teachers confirmed that security needs to be tightened before using social media in the classroom (Surface et al., 2014 ). Proper training on security and privacy, to use social media platforms in academics, is needed for students and teachers (Bhatnagar & Pry, 2020 ).
The personality traits of a student also play a significant role in deciding the impact of social media on students’ academic performance. Personality is a dynamic organization which simplifies the way a person behaves in a situation (Phares, 1991 ). Human behaviour has further been described by many renowned researchers. According to Lubinski ( 2000 ), human behaviour may be divided into five factors, i.e. cognitive abilities, personality, social attitudes, psychological interests and psychopathology. These personality traits are very important characteristics of a human being and play a substantial role in work commitment (Macey & Schneider, 2008 ). Goldberg ( 1993 ) elaborated on five dimensions of personality which are commonly known as the Big Five personality traits. The traits are “openness vs. cautious”; “extraversion vs. introversion”; “agreeableness vs. rational”; “conscientiousness vs. careless”; and “neuroticism vs. resilient”.
It has been found that among all personality traits, the “extraversion vs. introversion” personality trait has a greater impact on students’ academic performance (Costa & McCrae, 1999 ). Extrovert students are outgoing, talkative and assertive (Chamorro et al., 2003 ). They are positive thinkers and comfortable working in a crowd. Introvert students are reserved and quiet. They prefer to be isolated and work in silos (Bidjerano & Dai, 2007 ). So, in the present study, we have considered only the “extraversion vs. introversion” personality trait. This study is going to analyse the impact of social media platforms on students’ academic performance by taking the personality trait of extraversion and introversion as moderating variables along with their education level and gender.
Research Gap
Past research by Choney ( 2010 ), Karpinski and Duberstein ( 2009 ), Khan ( 2009 ) and Kubey et al. ( 2001 ) was done mostly in developed countries to analyse the impact of social media on the students’ academic performance, effect of social media on adolescence, and addictiveness of social media in students. There are no published research studies where the impact of social media was studied on students’ academic performance by taking their personality traits, education level and gender all three together into consideration. So, in the present study, the impact of social media will be evaluated on students’ academic performance by taking their personality traits (extraversion and introversion), education level (undergraduate and postgraduate) and gender (male and female) as moderating variables.
Objectives of the Study
Based on the literature review and research gap, the following research objectives have been defined:
- To identify the elements of social media impacting student's academic performance and to develop a suitable scale
- To test the validity and reliability of the scale
- To analyse the impact of social media on students’ academic performance using extraversion and introversion personality trait, education level and gender as moderating variables
Research Methodology
Sampling technique.
Convenience sampling was used for data collection. An online google form was floated to collect the responses from 408 male and female university students of undergraduation and postgraduation streams.
Objective 1 To identify the elements of social media impacting student's academic performance and to develop a suitable scale.
A structured questionnaire was employed to collect the responses from 408 students of undergraduate and postgraduate streams. The questionnaire was segregated into three sections. In section one, demographic details such as gender, age and education stream were defined. Section two contained the author’s self-developed 16-item scale related to the impact of social media on the academic performance of students. The third section had a standardized scale developed by John and Srivastava ( 1999 ) of the Big Five personality model.
Demographics
There were 408 respondents (students) of different education levels consisting of 202 males (49.5%) and 206 females (50.5%). Most of the respondents (87%) were from the age group of 17–25 years. 234 respondents (57.4) were enrolled on postgraduation courses, whereas 174 respondents (42.6) were registered in the undergraduate programme. The result further elaborates that WhatsApp with 88.6% and YouTube with 82.9% are the top two commonly used platforms followed by Instagram with 76.7% and Facebook with 62.3% of students. 65% of students stated that Google doc is a quite useful and important application in academics for document creation and information dissemination.
Validity and Reliability of Scale
Objective 2 Scale validity and reliability.
Exploratory factor analysis (EFA) and Cronbach’s alpha test were used to investigate construct validity and reliability, respectively.
The author’s self-designed scale of ‘social media impacting students’ academic performance’ consisting of 16 items was validated using exploratory factor analysis. The principle component method with varimax rotation was applied to decrease the multicollinearity within the items. The initial eigenvalue was set to be greater than 1.0 (Field, 2005 ). Kaiser–Meyer–Olkin (KMO) with 0.795 and Bartlett’s test of sphericity having significant values of 0.000 demonstrated the appropriateness of using exploratory factor analysis.
The result of exploratory factor analysis and Cronbach’s alpha is shown in Table Table1. 1 . According to Sharma and Behl ( 2020 ), “High loading on the same factor and no substantial cross-loading confirms convergent and discriminant validity respectively”.
Exploratory factor analysis and Cronbach’s alpha for the self-developed scale of “Social media impact on academic performance”
Factors | Items retained in factor analysis | Factor loading |
---|---|---|
Accelerating impact | ||
My grades are improving with the help of study materials shared on social media platforms | Yes | 0.918 |
For expressing our thoughts, social media platforms are the best means | Yes | 0.913 |
Our teachers share assignments and class activities on social media platforms which eventually help us in managing our academics better | Yes | 0.820 |
Academic discussions on public/private groups accelerate my understanding of the topics | Yes | 0.562 |
Deteriorating impact | ||
My academic performance negatively affected due to unlimited use of social media | Yes | 0.814 |
Distraction from studies is more when social media is added to academics | Yes | 0.808 |
My grades have deteriorated since I am engaged on these social platforms | Yes | 0.780 |
Addiction to social networking sites, affecting my academic performance | Yes | 0.761 |
I have observed mood swings and irresponsible behaviour due to social media posts | Yes | 0.631 |
Social media prospects | ||
Social media sites increase employment prospects | Yes | 0.715 |
I use social networking sites (SNS) to spread and share knowledge with my classmate | Yes | 0.686 |
Massive Open Online Courses (MOOCs) help me in the self-learning mode | Yes | 0.679 |
I use materials obtained from social media sites to complement what has been taught in the class | Yes | 0.634 |
Social media challenges | ||
Cyberbullying on social media platforms makes me anxious | Yes | 0.834 |
Privacy and security on social networking sites are the biggest challenges in academics | Yes | 0.736 |
Social media is a barrier for me to being engaged in face-to-face communication | Yes | 0.528 |
The self-developed scale was segregated into four factors, namely “Accelerating Impact”, “Deteriorating Impact”, “Social Media Prospects” and “Social Media Challenges”.
The first factor, i.e. “Accelerating Impact”, contains items related to positive impact of social media on students’ academic performance. Items in this construct determine the social media contribution in the grade improvement, communication and knowledge sharing. The second factor “Deteriorating Impact” describes the items which have a negative influence of social media on students’ academic performance. Items such as addiction to social media and distraction from studies are an integral part of this factor. “Social Media Prospects” talk about the opportunities created by social media for students’ communities. The last factor “Social Media Challenges” deals with security and privacy issues created by social media sites and the threat of cyberbullying which is rampant in academics.
The personality trait of an individual always influences the social media usage pattern. Therefore, the impact of social media on the academic performance of students may also change with their personality traits. To measure the personality traits, the Big Five personality model was used. This model consists of five personality traits, i.e. “openness vs. cautious”; “extraversion vs. introversion”; “agreeableness vs. rational”; “conscientiousness vs. careless”; and “neuroticism vs. resilient”. To remain focussed on the scope of the study, only a single personality trait, i.e. “extraversion vs. introversion” with 6 items was considered for analysis. A reliability test of this existing scale using Cronbach’s alpha was conducted. Prior to the reliability test, reverse scoring applicable to the associated items was also calculated. Table Table2 2 shows the reliability score, i.e. 0.829.
Cronbach’s alpha test for the scale of extraversion vs. introversion personality traits
Personality traits | Cronbach’s alpha value |
---|---|
I see myself as someone who is talkative | 0.829 |
I see myself as someone who is reserved and quiet | |
I see myself as someone who is full of energy and enthusiasm | |
I see myself as someone who has an assertive personality | |
I see myself as someone who is sometimes shy, self-conscious | |
I see myself as someone who is outgoing, sociable |
Objective 3 To analyse the impact of social media on students’ academic performance using extraversion and introversion personality traits, education level and gender as moderating variables.
The research model shown in Fig. 1 helps in addressing the above objective.

Social media factors impacting academic performances of extraversion and introversion personality traits of students at different education levels and gender
As mentioned in Fig. 1 , four dependent factors (Accelerating Impact, Deteriorating Impact, Social Media Prospects and Social Media Challenges) were derived from EFA and used for analysing the impact of social media on the academic performance of students having extraversion and introversion personality traits at different education levels and gender.
Students having a greater average score (more than three on a scale of five) for all personality items mentioned in Table Table2 2 are considered to be having extraversion personality or else introversion personality. From the valid dataset of 408 students, 226 students (55.4%) had extraversion personality trait and 182 (44.6%) had introversion personality trait. The one-way ANOVA analysis was employed to determine the impact of social media on academic performance for all three moderators, i.e. personality traits (Extraversion vs. Introversion), education levels (Undergraduate and Postgraduate) and gender (Male and Female). If the sig. value for the result is > = 0.05, we may accept the null hypothesis, i.e. there is no significant difference between extraversion and introversion personality students for the moderators; otherwise, null hypothesis is rejected which means there is a significant difference for the moderators.
Table Table3 3 shows the comparison of the accelerating impact of social media on the academic performance of all students having extraversion and introversion personality traits. It also shows a comparative analysis on education level and gender for these two personality traits of students. In the first comparison of extraversion and introversion students, the sig. value is 0.001, which indicates that there is a significant difference among extraversion and introversion students for the “Accelerating Impact” of social media on academic performance. Here, 3.781 is the mean value for introversion students which is higher than the mean value 3.495 of extraversion students. It clearly specifies that the accelerating impact of social media is more prominent in the students having introversion personality traits. Introversion students experienced social media as the best tool to express thoughts and improve academic grades. The result is also consistent with the previous studies where introvert students are perceived to use social media to improve their academic performance (Amichai-Hamburger et al., 2002 ; Voorn & Kommers, 2013 ). Further at the education level, there was a significant difference in postgraduate as well as undergraduate students for the accelerating impact of social media on the academic performance among students with extraversion and introversion, and introverts seem to get better use of social media. The gender-wise significant difference was also analysed between extraversion and introversion personalities. Female introversion students were found to gain more of an accelerating impact of social media on their academic performance.
One-way ANOVA: determining “Accelerating Impact” among extraversion and introversion personality traits students at different education levels and genders
Factor | Group | Mean | SD | Stat | Sig. | |
---|---|---|---|---|---|---|
Accelerating impact | Extraversion | 226 | 3.495 | 0.8912 | 11.68 | 0.001 |
Introversion | 182 | 3.781 | 0.7997 | |||
Accelerating impact | Extraversion | 129 | 3.643 | 0.741 | 7.388 | 0.007 |
Introversion | 105 | 3.901 | 0.7081 | |||
Accelerating impact | Extraversion | 99 | 3.292 | 1.033 | 5.102 | 0.025 |
Introversion | 77 | 3.621 | 0.8862 | |||
Accelerating impact | Extraversion | 115 | 3.578 | 0.9519 | 0.049 | 0.825 |
Introversion | 87 | 3.604 | 0.7651 | |||
Accelerating impact | Extraversion | 111 | 3.418 | 0.8921 | 23.079 | 0 |
Introversion | 95 | 3.964 | 0.7377 |
Significant at the 0.05 level
Like Table Table3, 3 , the first section of Table Table4 4 compares the deteriorating impact of social media on the academic performance of all students having extraversion and introversion personality traits. Here, the sig. value 0.383 indicates no significant difference among extraversion and introversion students for the “Deteriorating Impact” of social media on academic performance. The mean values show the moderating deteriorating impact of social media on the academic performance of extraversion and introversion personality students. Unlimited use of social media due to the addiction is causing a distraction in academic performance, but the overall impact is not on the higher side. Further, at the education level, the sig. values 0.423 and 0.682 of postgraduate and undergraduate students, respectively, show no significant difference between extraversion and introversion students with respect to “Deteriorating Impact of Social Media Sites”. The mean values again represent the moderate impact. Gender-wise, male students have no difference between the two personality traits, but at the same time, female students have a significant difference in the deteriorating impact, and it is more on extroverted female students.
One-way ANOVA: Examining “Deteriorating Impact” among extraversion and introversion personality traits students at different education levels and genders
Factor | Group | Mean | SD | Stat | Sig. | |
---|---|---|---|---|---|---|
Deteriorating impact | Extraversion | 226 | 2.535 | 0.969 | 0.764 | 0.383 |
Introversion | 182 | 2.615 | 0.852 | |||
Deteriorating impact | Extraversion | 129 | 2.547 | 0.9436 | 0.645 | 0.423 |
Introversion | 105 | 2.642 | 0.8342 | |||
Deteriorating impact | Extraversion | 97 | 2.52 | 1.0065 | 0.168 | 0.682 |
Introversion | 77 | 2.579 | 0.8799 | |||
Deteriorating impact | Extraversion | 115 | 2.722 | 0.9233 | 0.598 | 0.44 |
Introversion | 87 | 2.621 | 0.9155 | |||
Deteriorating impact | Extraversion | 111 | 2.611 | 0.7943 | 4.545 | 0.034 |
Introversion | 95 | 2.342 | 0.9814 |
The significant value, i.e. 0.82, in Table Table5 5 represents no significant difference between extraversion and introversion personality students for the social media prospects. The higher mean value of both personality students indicates that they are utilizing the opportunities of social media in the most appropriate manner. It seems that all the students are using social media for possible employment prospects, gaining knowledge by attending MOOCs courses and transferring knowledge among other classmates. At the education level, postgraduation students have no significant difference between extraversion and introversion for the social media prospects, but at the undergraduate level, there is a significant difference among both the personalities, and by looking at mean values, extroverted students gain more from the social media prospects. Gender-wise comparison of extraversion and introversion personality students found no significant difference in the social media prospects for male as well as female students.
One-way ANOVA: Examining “Social Media Prospects” among extraversion and introversion personality traits students at different education levels and genders
Factor | Group | Mean | SD | Stat | Sig. | |
---|---|---|---|---|---|---|
Social media opportunities | Extraversion | 226 | 3.704 | 0.716 | 3.031 | 0.082 |
Introversion | 182 | 3.574 | 0.782 | |||
Social media prospects | Extraversion | 129 | 3.893 | 0.6356 | 0.086 | 0.77 |
Introversion | 105 | 3.869 | 0.6308 | |||
Social media prospects | Extraversion | 97 | 3.451 | 0.7418 | 5.717 | 0.018 |
Introversion | 77 | 3.172 | 0.7919 | |||
Social media prospects | Extraversion | 115 | 3.713 | 0.655 | 1.487 | 0.224 |
Introversion | 87 | 3.589 | 0.7887 | |||
Social media prospects | Extraversion | 111 | 3.694 | 0.7773 | 1.499 | 0.222 |
Introversion | 95 | 3.561 | 0.7793 |
Table Table6 6 shows the comparison of the social media challenges of all students having extraversion and introversion personality traits. It is also doing a comparative analysis on education level and gender for these two personality traits of students. All sig. values in Table Table6 6 represent no significant difference between extraversion and introversion personality students for social media challenges. Even at the education level and gender-wise comparison of the two personalities, no significant difference is derived. The higher mean values indicate that the threat of cyberbullying, security and privacy is the main concern areas for extraversion and introversion personality students. Cyberbullying is seen to be more particularly among female students (Snell & Englander, 2010 ).
One-way ANOVA: Examining “Social Media Challenges” among extraversion and introversion personality traits students at different education levels and genders
Factor | Group | Mean | SD | Stat | Sig. | |
---|---|---|---|---|---|---|
Social media challenges | Extraversion | 226 | 3.273 | 0.889 | 0.707 | 0.401 |
Introversion | 182 | 3.2 | 0.857 | |||
Social media challenges | Extraversion | 129 | 3.375 | 0.874 | 2.067 | 0.152 |
Introversion | 105 | 3.21 | 0.8737 | |||
Social media challenges | Extraversion | 97 | 3.136 | 0.8946 | 0.134 | 0.714 |
Introversion | 77 | 3.186 | 0.8386 | |||
Social media challenges | Extraversion | 115 | 3.322 | 0.8353 | 0.398 | 0.529 |
Introversion | 87 | 3.245 | 0.8767 | |||
Social media challenges | Extraversion | 111 | 3.222 | 0.9421 | 0.263 | 0.608 |
Introversion | 95 | 3.158 | 0.8405 |
The use of social media sites in academics is becoming popular among students and teachers. The improvement or deterioration in academic performance is influenced by the personality traits of an individual. This study has tried to analyse the impact of social media on the academic performance of extraversion and introversion personality students. This study has identified four factors of social media which have an impact on academic performance. These factors are: accelerating impact of social media; deteriorating impact of social media; social media prospects; and social media challenges.
Each of these factors has been used for comparative analysis of students having extraversion and introversion personality traits. Their education level and gender have also been used to understand the detailed impact between these two personality types. In the overall comparison, it has been discovered that both personalities (extraversion and introversion) have a significant difference for only one factor, i.e. “Accelerating Impact of Social Media Sites” where students with introversion benefited the most. At the education level, i.e. postgraduate and undergraduate, there was a significant difference between extraversion and introversion personalities for the first factor which is the accelerating impact of social media. Here, the introversion students were found to benefit in postgraduate as well as undergraduate courses. For the factors of deteriorating impact and social media challenges, there was no significant difference between extraversion and introversion personality type at the different education levels.
Surprisingly, for the first factor, i.e. the accelerating impact of social media, in gender-wise comparison, no significant difference was found between extraversion and introversion male students. Whereas a significant difference was found in female students. The same was the result for the second factor, i.e. deteriorating impact of social media of male and female students. For social media prospects and social media challenges, no significant difference was identified between extraversion and introversion students of any gender.
Findings and Implications
The personality trait of a student plays a vital role in analysing the impact of social media on their academic performance. The present study was designed to find the difference between extraversion and introversion personality types in students for four identified factors of social media and their impact on students’ academic performance. The education level and gender were also added to make it more comprehensive. The implications of this study are useful for institutions, students, teachers and policymakers.
This study will help the institutions to identify the right mix of social media based on the personality, education level and gender of the students. For example, technological challenges are faced by all students. It is important for the institutions to identify the challenges such as cyberbullying, security and privacy issues and accordingly frame the training sessions for all undergraduate and postgraduate students. These training sessions will help students with extraversion and introversion to come out from possible technological hassles and will create a healthy ecosystem (Okereke & Oghenetega, 2014 ).
Students will also benefit from this study as they will be conscious of the possible pros and cons that exist because of social media usage and its association with students’ academic performance. This learning may help students to enhance their academic performance with the right use of social media sites. The in-depth knowledge of all social media platforms and their association with academics should be elucidated to the students so that they may explore the social media opportunities in an optimum manner. Social media challenges also need to be made known to the students to improve upon and overcome with time (Boateng & Amankwaa, 2016 ).
Teachers are required to design the curriculum by understanding the learning style of students with extraversion and introversion personality type. Innovation and customization in teaching style are important for the holistic development of students and to satisfy the urge for academic requirements. Teachers should also guide the students about the adverse impacts of each social media platform, so that these can be minimized. Students should also be guided to reduce the time limit of using social media (Owusu-Acheaw & Larson, 2015 ).
Policymakers are also required to understand the challenges faced by the students while using social media in academics. All possible threats can be managed by defining and implementing transparent and proactive policies. As social media sites are open in nature, security and privacy are the two major concerns. The Government of India should take a strong stand to control all big social media companies so that they may fulfil the necessary compliances related to students’ security and privacy (Kumar & Pradhan, 2018 ).
The overall result of these comparisons gives a better insight and deep understanding of the significant differences between students with extraversion and introversion personality type towards different social media factors and their impact on students’ academic performance. Students’ behaviour according to their education level and gender for extraversion and introversion personalities has also been explored.
Limitation and Future Scope of Research
Due to COVID restrictions, a convenient sampling technique was used for data collection which may create some response biases where the students of introversion personality traits may have intentionally described themselves as extroversion personalities and vice versa. This study also creates scope for future research. In the Big Five personality model, there are four other personality traits which are not considered in the present study. There is an opportunity to also use cross-personality comparisons for the different social media parameters. The other demographic variables such as age and place may also be explored in future research.
Author contributions
All authors contributed to the study conception and design. Material preparation, data collection and analysis were performed by Dr. SS and Prof. RB. The first draft of the manuscript was written by Dr. SS, and all authors commented on previous versions of the manuscript. All authors read and approved the final manuscript.
No funds, grants, or other support was received.
Availability of data and material
Declarations.
The authors declare that they have no conflict of interest.
All procedures performed in studies involving human participants were in accordance with the ethical standards of the institutional and/or national research committee and with the 1964 Helsinki Declaration and its later amendments or comparable ethical standards.
Verbal informed consent was obtained from the participants.
Verbal consent is obtained for publication
Publisher's Note
Springer Nature remains neutral with regard to jurisdictional claims in published maps and institutional affiliations.
Contributor Information
Sourabh Sharma, Email: ni.ude.hbimi@hbaruos .
Ramesh Behl, Email: ude.imi@lhebr .
- Alshuaibi M, Ibrahim S, Ibrahim AAS, Mohd F, Shamsudin DA, Arshad. Use of social media, student engagement, and academic performance of business students in Malaysia. International Journal of Educational Management. 2018; 32 (4):625–640. [ Google Scholar ]
- Amichai-Hamburger Y, Wainapel G, Fox S. "On the Internet no one knows I'm an introvert": Extroversion, neuroticism, and Internet interaction. Cyberpsychology & Behaviour. 2002; 5 (2):125–128. doi: 10.1089/109493102753770507. [ PubMed ] [ CrossRef ] [ Google Scholar ]
- Amin Z, Mansoor A, Hussain SR, Hashmat F. Impact of social media of student’s academic performance. International Journal of Business and Management Invention. 2016; 5 (4):22–29. [ Google Scholar ]
- Arnold N, Paulus T. Using a social networking site for experiential learning: Appropriating, lurking, modeling and community building. The Internet and Higher Education. 2010; 13 (4):188–196. doi: 10.1016/j.iheduc.2010.04.002. [ CrossRef ] [ Google Scholar ]
- Asur, S., & Huberman, B. A. (2010, August). Predicting the future with social media. In 2010 IEEE/WIC/ACM international conference on web intelligence and intelligent agent technology (Vol. 1, pp. 492–499). IEEE.
- Basuray, T. (2022). Social media usage in India: Statistics & facts. https://www.statista.com/topics/5113/social-media-usage-in-india/#topicHeader__wrapper .
- Bhatnagar N, Pry M. Student attitudes, awareness, and perceptions of personal privacy and cybersecurity in the use of social media: An initial study. Information Systems Education Journal. 2020; 18 (1):48–58. [ Google Scholar ]
- Bidjerano T, Dai DY. The relationship between the big-five model of personality and self-regulated learning strategies. Learning and Individual Differences. 2007; 17 (1):69–81. doi: 10.1016/j.lindif.2007.02.001. [ CrossRef ] [ Google Scholar ]
- Boateng R, Amankwaa A. The impact of social media on student academic life in higher education. Global Journal of Human-Social Science. 2016; 16 (4):1–8. [ Google Scholar ]
- Boyd DM, Ellison NB. Social Network Sites: Definition, history and scholarship. Journal of Computer-Mediatied Communication. 2008; 13 :210–230. doi: 10.1111/j.1083-6101.2007.00393.x. [ CrossRef ] [ Google Scholar ]
- Chamorro Premuzic T, Furnham A. Personality predicts academic performance: Evidence from two longitudinal university samples. Journal of Research in Personality. 2003; 37 (4):319–338. doi: 10.1016/S0092-6566(02)00578-0. [ CrossRef ] [ Google Scholar ]
- Chen, J. (2013). Opportunities and challenges of MOOCs: perspectives from Asia.
- Choney, S. (2010) Facebook Use Can Lower Grades by 20 Percent, Study Says. http://www.msnbc.com/id/39038581/ns.technology_and_science-tech_and_gadgets/
- Costa PT, McCRAE RR. A five-factor theory of personality. The Five-Factor Model of Personality: Theoretical Perspectives. 1999; 2 :51–87. [ Google Scholar ]
- Dzogbenuku RK, George AK, Kumi DK. Social media and student performance: The moderating role of ICT knowledge. Journal of Information, Communication and Ethics in Society. 2019; 18 (2):197–219. doi: 10.1108/JICES-08-2019-0092. [ CrossRef ] [ Google Scholar ]
- Englander F, Terregrossa RA, Wang Z. Internet use among college students: Tool or toy? Educational Review. 2010; 62 (1):85–96. doi: 10.1080/00131910903519793. [ CrossRef ] [ Google Scholar ]
- Fedock BC, McCartney M, Neeley D. Online adjunct higher education teachers’ perceptions of using social media sites as instructional approaches. Journal of Research in Innovative Teaching & Learning. 2019; 12 (3):222–235. doi: 10.1108/JRIT-02-2018-0005. [ CrossRef ] [ Google Scholar ]
- Field AP. Discovering statistics using SPSS. 2. Sage Publications; 2005. [ Google Scholar ]
- Goldberg LR. The structure of phenotypic personality traits. American Psychologist. 1993; 48 (1):26–34. doi: 10.1037/0003-066X.48.1.26. [ PubMed ] [ CrossRef ] [ Google Scholar ]
- Jain N, Verma A, Verma R, Tiwari P. Going social: The impact of social networking in promoting education. International Journal of Computer Science. 2012; 9 (1):483–485. [ Google Scholar ]
- John OP, Srivastava S. The Big Five trait taxonomy: History, measurement, and theoretical perspectives. Handbook of Personality: Theory and Research. 1999; 2 (1999):102–138. [ Google Scholar ]
- Johnson, J. (2022). Worldwide digital population as of January 2022. https://www.statista.com/statistics/617136/digital-population-worldwide/ .
- Kaplan AM, Haenlein M. Users of the world, unite! The Challenges and Opportunities of Social Media. Business Horizons. 2010; 53 (1):59–68. doi: 10.1016/j.bushor.2009.09.003. [ CrossRef ] [ Google Scholar ]
- Karpinski, A. C., & Duberstein, A. (2009). A description of Facebook use and academic performance among undergraduate and graduate students. In Annual Meeting of the American Educational Research Association, San Diego, CA (pp. 5–10).
- Khan, U (2009). Facebook student underachieve in exams. Daily Telegraph. http://www.telegraph.co.uk/educationnews/5145243/Facebook-students-underachieve-in-exams.html
- Kirschner PA, Karpinski AC. Facebook® and academic performance. Computers in Human Behavior. 2010; 26 (6):1237–1245. doi: 10.1016/j.chb.2010.03.024. [ CrossRef ] [ Google Scholar ]
- Kubey RW, Lavin MJ, Barrows JR. Internet use and collegiate academic performance decrements: Early findings. Journal of Communication. 2001; 51 (2):366–382. doi: 10.1111/j.1460-2466.2001.tb02885.x. [ CrossRef ] [ Google Scholar ]
- Kumar, V., & Pradhan, P. (2018). Trust management issues in social-media marketing. In Social Media Marketing: Breakthroughs in Research and Practice (pp. 714–732). IGI Global.
- Landry, T. (2014). How social media has changed us: The good and the bad. Retrieved from .
- Laura R, Jose B, Braojos J. From traditional education technologies to student satisfaction in Management education: A theory of the role of social media applications. Information & Management. 2017; 54 (8):1059–1071. doi: 10.1016/j.im.2017.06.002. [ CrossRef ] [ Google Scholar ]
- Lubinski D. Scientific and social significance of assessing individual differences:“Sinking shafts at a few critical points” Annual Review of Psychology. 2000; 51 (1):405–444. doi: 10.1146/annurev.psych.51.1.405. [ PubMed ] [ CrossRef ] [ Google Scholar ]
- Macey WH, Schneider B. The meaning of employee engagement. Industrial and Organizational Psychology. 2008; 1 :3–30. doi: 10.1111/j.1754-9434.2007.0002.x. [ CrossRef ] [ Google Scholar ]
- McCrae RR, Costa PT. Validation of the five-factor model of personality across instruments and observers. Journal of Personality and Social Psychology. 1987; 52 (1):81. doi: 10.1037/0022-3514.52.1.81. [ PubMed ] [ CrossRef ] [ Google Scholar ]
- Nalwa K, Anand AP. Internet addiction in students: A cause of concern. Cyberpsychology & Behavior. 2003; 6 (6):653–656. doi: 10.1089/109493103322725441. [ PubMed ] [ CrossRef ] [ Google Scholar ]
- Okereke CE, Oghenetega LU. The impact of social media on the academic performance of university students in Nigeria. Journal of Education and Practice. 2014; 5 (33):21–24. [ Google Scholar ]
- Oueder M, Abousaber I. A Study on the Impact of Social Media Usage on Student Academic Performance: University of Tabuk an Example. American Academic Scientific Research Journal for Engineering, Technology, and Sciences. 2018; 40 (1):77–88. [ Google Scholar ]
- Owusu-Acheaw M, Larson AG. Use of social media and its impact on academic performance of tertiary institution students: A study of students of Koforidua Polytechnic, Ghana. Journal of Education and Practice. 2015; 6 (6):94–101. [ Google Scholar ]
- Park N, Kee KF, Valenzuela S. Being immersed in social networking environment: Facebook groups, uses and gratifications, and social outcomes. Cyberpsychology & Behavior. 2009; 12 (6):729–733. doi: 10.1089/cpb.2009.0003. [ PubMed ] [ CrossRef ] [ Google Scholar ]
- Phares EJ. Introduction to psychology. 3. Harper Collins; 1991. [ Google Scholar ]
- Qiu L, Lin H, Leung AKY. Cultural differences and switching of in-group sharing behavior between an American (Facebook) and a Chinese (Renren) social networking site. Journal of Cross-Cultural Psychology. 2013; 44 (1):106–121. doi: 10.1177/0022022111434597. [ CrossRef ] [ Google Scholar ]
- Raja, M. A. S., & Kallarakal, T. K. (2020). “COVID-19 and students perception about MOOCs” a case of Indian higher educational institutions. Interactive Technology and Smart Education .
- Rithika M, Selvaraj S. Impact of social media on students’ academic performance. International Journal of Logistics & Supply Chain Management Perspectives. 2013; 2 (4):636–640. [ Google Scholar ]
- Sharma, S., & Behl, R. (2020). Strategic Alignment of Information Technology in Public and Private Organizations in India: A Comparative Study. Global Business Review , 1–18.
- Snell, P. A., & Englander, E. (2010). Cyberbullying victimization and behaviors among girls: Applying research findings in the field. Journal of Social Sciences .
- Statista Research Department. (2022). Countries with the most Facebook users 2022. https://www.statista.com/statistics/268136/top-15-countries-based-on-number-of-facebook-users/
- Stergiou C, Psannis KE, Kim BG, Gupta B. Secure integration of IoT and cloud computing. Future Generation Computer Systems. 2018; 78 :964–975. doi: 10.1016/j.future.2016.11.031. [ CrossRef ] [ Google Scholar ]
- Surface JL, Stader D, Armenta A. Educator sexual misconduct and nondisclosure agreements: Policy guidance from missouri’s amy hestir student protection act. Educational Leadership Faculty Publications; 2014. [ Google Scholar ]
- Voorn RJ, Kommers PA. Social media and higher education: Introversion and collaborative learning from the student’s perspective. International Journal of Social Media and Interactive Learning Environments. 2013; 1 (1):59–73. doi: 10.1504/IJSMILE.2013.051650. [ CrossRef ] [ Google Scholar ]
- Whiting A, Williams D. Why people use social media: A uses and gratifications approach. Qualitative Market Research: An International Journal. 2013; 16 (4):362–369. doi: 10.1108/QMR-06-2013-0041. [ CrossRef ] [ Google Scholar ]
- Yunus M, Salehi H. The effectiveness of Facebook groups on teaching and improving writing: students’ perceptions. International Journal of Education and Information Technologies. 2012; 6 :87–96. [ Google Scholar ]
Publication
The Impacts of Teacher Expectations on Student Outcomes
A practitioner's literature review.
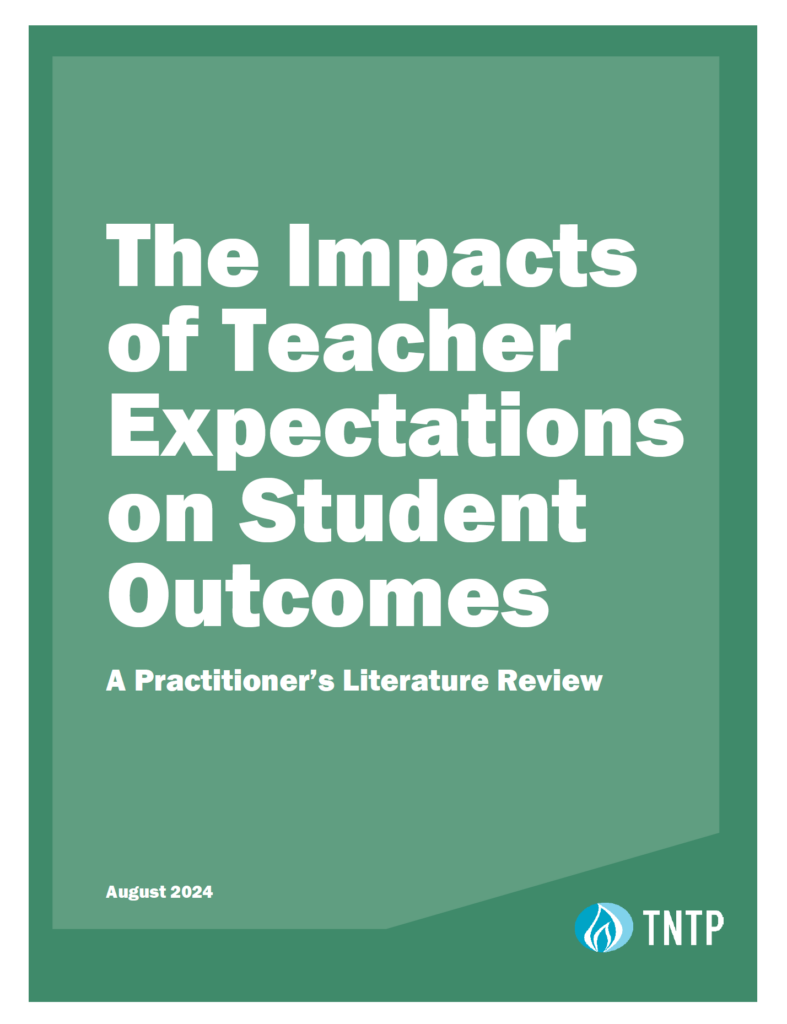
Research has shown that teacher beliefs shape classroom dynamics, influence student performance, and drive achievement disparities, especially across racial and socioeconomic lines. However, interventions can help mitigate these beliefs and empower teachers to raise expectations for all students, creating growth-focused, inclusive environments. This resource is a research base that focuses on equitable student outcomes through an exploration of teacher expectations and their profound impact on student success.
Related Topics
- Curriculum and Instruction
- Leadership and Development
Stay in the Know
Sign up for updates on our latest research, insights, and high-impact work.
" * " indicates required fields
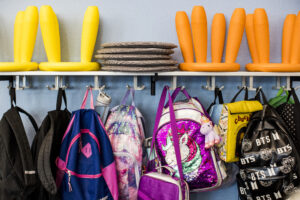
Case Study | May 14, 2024
Good to Great en Puerto Rico
TNTP ofreció desarrollo profesional a docentes de Español de nivel elemental en San Juan, Puerto Rico.
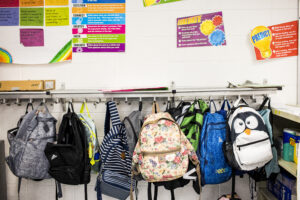
From Good to Great in Puerto Rico
TNTP helped transform teacher mindsets through a Spanish language arts professional development intensive for K-6 educators in San Juan, Puerto Rico.
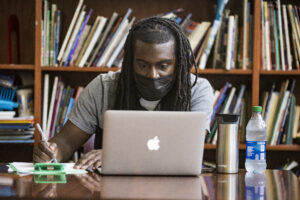
Blog Post | May 8, 2024
Innovative Educator Prep Program Centers HQIM and Research-Based Teaching Methods
A community college sees increased enrollment in training programs for new teachers and facilitates social mobility by embedding HQIM in their curricula.
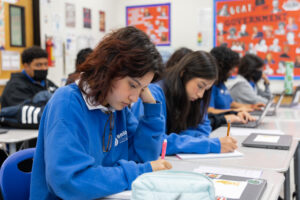
Blog Post | April 29, 2024
How This High School Network Built a System of Support for Multilingual Learners
Ednovate, a network of six high schools in California, coordinates support for multilingual learners by prioritizing their input and involving leadership.
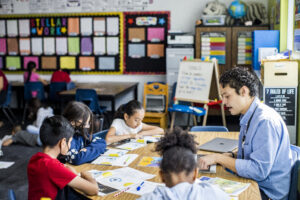
Blog Post | April 23, 2024
Ask Them to Stay: Data-Backed Teacher Retention Strategies
TNTP’s Insight surveys show that strategies for retaining teachers can be low-cost and as simple as offering positive feedback and asking them to stay.
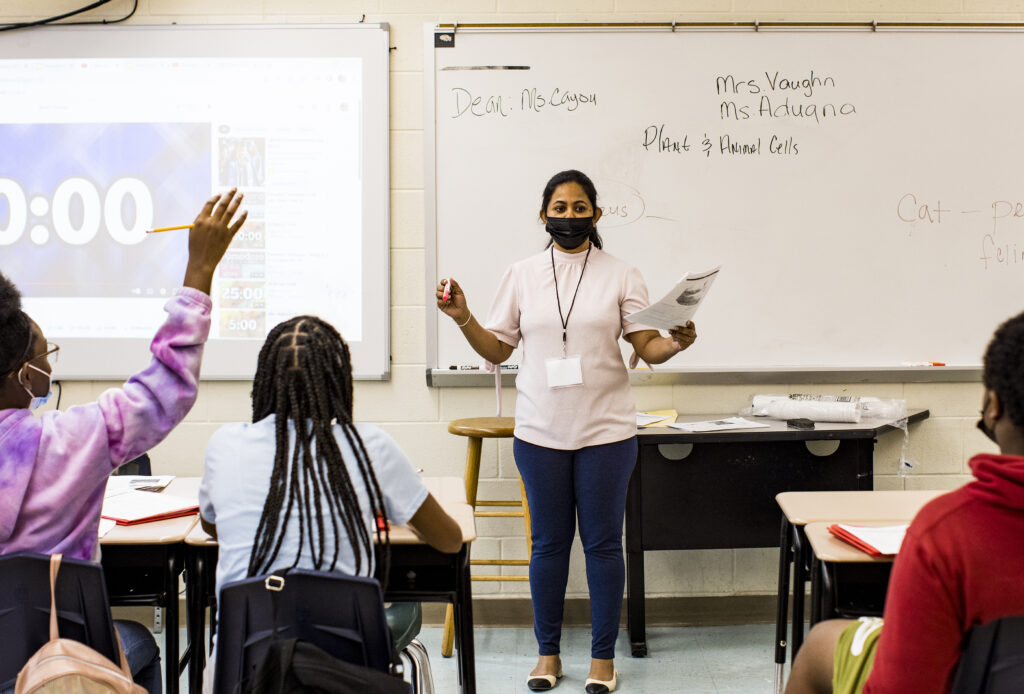
Imali Ariyarathne, seventh-grade teacher at Langston Hughes Academy, introduces her students to the captivating world of science.
TNTP is the nation’s leading research, policy, and consulting organization dedicated to transforming America’s public education system, so that every generation thrives.
Today, we work side-by-side with educators, system leaders, and communities across 39 states and over 6,000 districts nationwide to reach ambitious goals for student success.
Yet the possibilities we imagine push far beyond the walls of school and the education field alone. We are catalyzing a movement across sectors to create multiple pathways for young people to achieve academic, economic, and social mobility.
Academia.edu no longer supports Internet Explorer.
To browse Academia.edu and the wider internet faster and more securely, please take a few seconds to upgrade your browser .
Enter the email address you signed up with and we'll email you a reset link.
- We're Hiring!
- Help Center
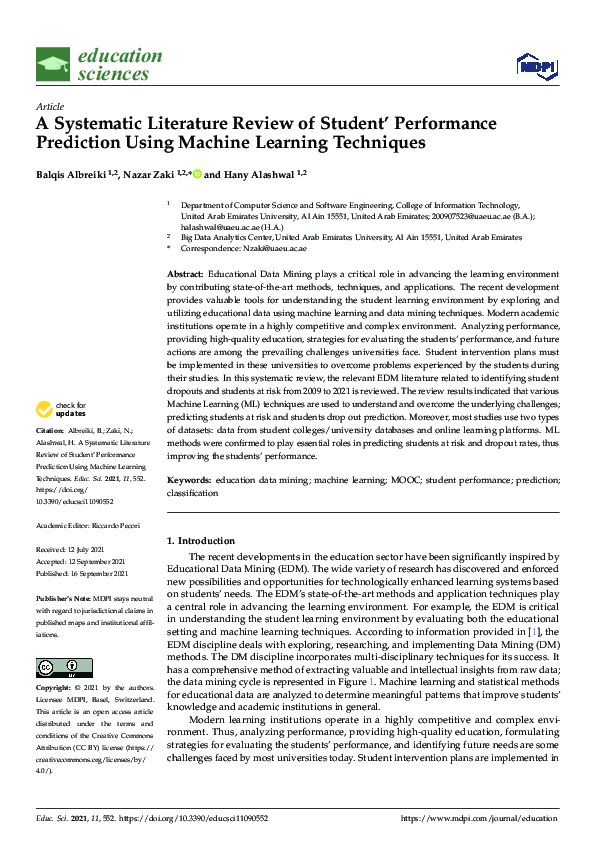
A Systematic Literature Review of Student’ Performance Prediction Using Machine Learning Techniques

2021, Education Sciences
Educational Data Mining plays a critical role in advancing the learning environment by contributing state-of-the-art methods, techniques, and applications. The recent development provides valuable tools for understanding the student learning environment by exploring and utilizing educational data using machine learning and data mining techniques. Modern academic institutions operate in a highly competitive and complex environment. Analyzing performance, providing high-quality education, strategies for evaluating the students’ performance, and future actions are among the prevailing challenges universities face. Student intervention plans must be implemented in these universities to overcome problems experienced by the students during their studies. In this systematic review, the relevant EDM literature related to identifying student dropouts and students at risk from 2009 to 2021 is reviewed. The review results indicated that various Machine Learning (ML) techniques are used to unde...
Related Papers
Education and Information Technologies
Sitalakshmi Venkatraman
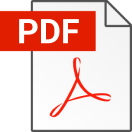
International Journal of Emerging Technologies in Learning (iJET)
khalid oqaidi
Using machine learning to predict students’ dropout in higher education institutions and programs has proven to be effective in many use cases. In an approach based on machine learning algorithms to detect students at risk of dropout, there are three main factors: the choice of features likely to influence a partial or total stop of the student, the choice of the algorithm to implement a prediction model, and the choice of the evaluation metrics to monitor and assess the credibility of the results. This paper aims to provide a diagnosis of machine learning techniques used to detect students’ dropout in higher education programs, a critical analysis of the limitations of the models proposed in the literature, as well as the major contribution of this arti-cle is to present recommendations that may resolve the lack of global model that can be generalized in all the higher education institutions at least in the same country or in the same university.
International Journal of Computer Applications Technology and Research
James AFRIFA
The advancement in Information Technology makes it easier and cheaper to collect large amounts of data, but if this data is not further analyzed, it remains only huge amounts of data. These large amounts of data set have motivated research and development in various fields to extract meaningful information with a view of analyzing it to solve complex problem. With new methods and techniques, data can be analyze and be of great advantage. Data mining and machine learning are two computing disciplines that enable analysis of large data sets using different techniques. This paper gave an overview of several applications using these disciplines in education, with focus on student’s academic performance prediction. Early prediction of students’ performance is useful in taking early action of improving learning outcome. The perfect methods for this are machine learning and data mining. This paper also discusses special use of data mining in education, called educational data mining. Educa...
Technology, Knowledge and Learning
Ayuba Bappah
International Journal of Information and Communication Technology Education
Hoda Abdel Hafez
Learning data analytics improves the learning field in higher education using educational data for extracting useful patterns and making better decisions. Identifying potential at-risk students may help instructors and academic guidance to improve the students' performance and the achievement of learning outcomes. The aim of this research study is to predict at early phases the student's failure in a particular course using the standards-based grading. Several machine learning techniques were implemented to predict the student failure based on support vector machine, multilayer perceptron, naïve bayes, and decision tree. The results on each technique show the ability of machine learning algorithms to predict the student failure accurately after the third week and before the course dropout week. This study provides a strong knowledge for student performance in all courses. It also provides faculty members the ability to help at-risk students by focusing on them and providing necessary support to improve their performance and avoid failure.
Applied Sciences
Boran Sekeroglu
Improving the quality, developing and implementing systems that can provide advantages to students, and predicting students’ success during the term, at the end of the term, or in the future are some of the primary aims of education. Due to its unique ability to create relationships and obtain accurate results, artificial intelligence and machine learning are tools used in this field to achieve the expected goals. However, the diversity of studies and the differences in their content create confusion and reduce their ability to pioneer future studies. In this study, we performed a systematic literature review of student performance prediction studies in three different databases between 2010 and 2020. The results are presented as percentages by categorizing them as either model, dataset, validation, evaluation, or aims. The common points and differences in the studies are determined, and critical gaps and possible remedies are presented. The results and identified gaps could be elim...
IJCSIS Vol 18 No. 3 March 2020 Issue
Journal of Computer Science IJCSIS
Predicting students’ academic performance is of great concern for both students and educational institutions. Drawing on the prediction of learning outcome, lecturers and institutional administrators can facilitate students’ learning plan by making adjustments to the lectures and curriculum. Academic performance of students can depend on various factors such as academic progress, personal characteristics and behaviors relating to learning activities. This paper investigate the machine learning techniques to predict the final Grade Point Average of students based on personal characteristics (comprising gender and living location), university entry scores, gap year, and their academic performance of the first and second year. The data is collected by combining data from a survey of graduate students of three different years and data from the student management information system of the university. The result proved the correlation between the aforementioned factors and the academic performance of students and it also revealed the most suitable technique for student performance prediction by empirical study. Keywords: student academic performance, machine learning, data mining.
IJESRT Journal
Students' academic performance is perilous for educational institutions because tactical programs can be prearranged in cultivating or maintaining enactment of the students for the duration of their period of studies in the institutions. The upsurge of student's dropout rate in higher education is one of the significant problems in most organizations. The unearthing of hidden information from the educational data system by the operative process of data mining technique to investigate factors affecting student waster can lead to a healthier academic planning and administration to moderate students drop out frequency, as well as can apprise cherished information for outcome making of policy makers to mend the quality of higher educational system. In this paper, we consider issues of factors affecting students' dropout rate, discussed about different techniques of data mining, machine learning which will predict the student performance index and what the parameters are which affects the accuracy of prediction model.
IEEE Access
David Asirvatham
International Journal of Advanced Computer Science and Applications
Loading Preview
Sorry, preview is currently unavailable. You can download the paper by clicking the button above.
RELATED PAPERS
MALAYSIAN JOURNAL OF COMPUTING
Moshood Alarape
Social Indicators Research
Simone Di Zio
SSRN Electronic Journal
Kerstin Schneider
INTERNATIONAL JOURNAL OF COMPUTERS & TECHNOLOGY
Williamjeet Singh
Computational Intelligence and Neuroscience
Abdulsalam Mustafa
IRJET Journal
sarah alturki
chinhoyi university of technology
Raphael Muchovo
Sunil Bhutada
Anais do XXXII Simpósio Brasileiro de Informática na Educação (SBIE 2021)
Norton Roman
Carlos Preciado
Kanna Velusamy
Physical Science & Biophysics Journal
emmanuel ukekwe

RELATED TOPICS
- We're Hiring!
- Help Center
- Find new research papers in:
- Health Sciences
- Earth Sciences
- Cognitive Science
- Mathematics
- Computer Science
- Academia ©2024
Information
- Author Services
Initiatives
You are accessing a machine-readable page. In order to be human-readable, please install an RSS reader.
All articles published by MDPI are made immediately available worldwide under an open access license. No special permission is required to reuse all or part of the article published by MDPI, including figures and tables. For articles published under an open access Creative Common CC BY license, any part of the article may be reused without permission provided that the original article is clearly cited. For more information, please refer to https://www.mdpi.com/openaccess .
Feature papers represent the most advanced research with significant potential for high impact in the field. A Feature Paper should be a substantial original Article that involves several techniques or approaches, provides an outlook for future research directions and describes possible research applications.
Feature papers are submitted upon individual invitation or recommendation by the scientific editors and must receive positive feedback from the reviewers.
Editor’s Choice articles are based on recommendations by the scientific editors of MDPI journals from around the world. Editors select a small number of articles recently published in the journal that they believe will be particularly interesting to readers, or important in the respective research area. The aim is to provide a snapshot of some of the most exciting work published in the various research areas of the journal.
Original Submission Date Received: .
- Active Journals
- Find a Journal
- Proceedings Series
- For Authors
- For Reviewers
- For Editors
- For Librarians
- For Publishers
- For Societies
- For Conference Organizers
- Open Access Policy
- Institutional Open Access Program
- Special Issues Guidelines
- Editorial Process
- Research and Publication Ethics
- Article Processing Charges
- Testimonials
- Preprints.org
- SciProfiles
- Encyclopedia
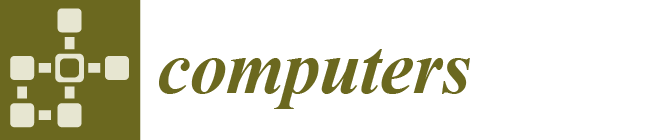
Article Menu

- Subscribe SciFeed
- Recommended Articles
- Author Biographies
- Google Scholar
- on Google Scholar
- Table of Contents
Find support for a specific problem in the support section of our website.
Please let us know what you think of our products and services.
Visit our dedicated information section to learn more about MDPI.
JSmol Viewer
Predicting student performance in introductory programming courses.
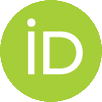
1. Introduction
2. methodology, 2.1. datasets.
- Questionnaires
- Academic Records
- Personal Data
2.2. Evaluation Metrics
- Accuracy-is the most common evaluation metric. It works as an indicator of the model’s overall performance by determining the percentage of correctly classified instances [ 38 ].
- Precision-is an extremely useful metric, in situations where false positives are more important than false negatives because it allows us to evaluate the model and determine what percentage of instances classified as positive are positive [ 38 ].
- Recall-is a metric that can be very useful when false negatives are more important than false positives, as it allows us to calculate the percentage of true positive instances that have been correctly classified [ 38 ].
- F1-Score-is created when the precision and recall metrics are both critical. This metric combines precision and recall through a harmonic mean, so by maximizing the value of the F1-Score, we simultaneously maximize the precision and recall [ 38 ].
- Specificity-is the metric that allows calculating the percentage of truly negative instances that have been classified as negative; in other words, it assesses the ability of the model to correctly identify negative cases [ 38 ].
- Sensitivity-is the metric that allows calculating the percentage of truly positive instances that have been classified as positive [ 38 ].
- Root Mean Square Error (RMSE)-is commonly used to evaluate model performance. This metric measures the average squared difference between the values predicted by the model and the actual values. The lower the RMSE value, the better the model’s performance [ 38 ].
3. Related Work
4.1. models, 4.2. most-used algorithms, 4.3. most-used evaluation metrics, 4.4. most-used dataset, 4.5. datasets analysis, 4.6. algorithms with best results, 4.7. deep learning approach, 4.8. challenges and gaps.
- Data Quality Assurance
- Selection of the Algorithms
- Evaluation Metrics Application
5. Discussion
6. threats to validity, 7. future research directions.
- Use of deep learning techniques
- Integration of various data sources
8. Conclusions
Author contributions, institutional review board statement, informed consent statement, data availability statement, conflicts of interest.
- Watson, C.; Li, F.W. Failure rates in introductory programming revisited. In Proceedings of the 2014 Innovation and Technology in Computer Science Education Conference, Uppsala, Sweden, 21–25 June 2014. [ Google Scholar ] [ CrossRef ]
- Bennedsen, J.; Caspersen, M.E. Failure rates in introductory programming. ACM Inroads 2019 , 10 , 30–36. [ Google Scholar ] [ CrossRef ]
- Lahtinen, E.; Ala-Mutka, K.; Järvinen, H.-M. A study of the difficulties of novice programmers—12 years later. ACM SIGCSE Bull. 2005 , 37 , 14–18. [ Google Scholar ] [ CrossRef ]
- Qian, Y.; Lehman, J. Students’ misconceptions and other difficulties in introductory programming. ACM Trans. Comput. Educ. 2017 , 18 , 1–24. [ Google Scholar ] [ CrossRef ]
- Gomes, A.; Mendes, A.J.N. Learning to program-difficulties and solutions. In Proceedings of the International Conference on Engineering Education—ICEE 2007, Coimbra, Portugal, 3–7 September 2007. [ Google Scholar ]
- Jenkins, T. On The Difficulty of Learning To Program. In Proceedings of the 3rd Annual LTSN-ICS Conference, Loughborough University, Loughborough, UK, 27–29 August 2002. [ Google Scholar ]
- Bennedsen, J.; Caspersen, M.E. Abstraction ability as an indicator of success for learning object-oriented programming? ACM SIGCSE Bull. 2006 , 38 , 39–43. [ Google Scholar ] [ CrossRef ]
- Byrne, P.; Lyons, G. The effect of student attributes on success in programming. ACM SIGCSE Bull. 2001 , 33 , 49–52. [ Google Scholar ] [ CrossRef ]
- Luxton-Reilly, A.; Simon; Albluwi, I.; Becker, B.A.; Giannakos, M.; Kumar, A.N.; Ott, L.; Paterson, J.; Scott, M.J.; Sheard, J.; et al. Introductory programming: A systematic literature review. In Proceedings of the ITiCSE ′18: 23rd Annual ACM Conference on Innovation and Technology in Computer Science Education, Larnaca, Cyprus, 2–4 July 2018. [ Google Scholar ]
- Gomes, A.; Mendes, A. A study on student’s characteristics and programming learning. In Proceedings of the ED-MEDIA 2008—World Conference on Educational Multimedia, Hypermedia & Telecommunications, Vienna, Austria, 30 June 2008. [ Google Scholar ]
- Gomes, A.; Mendes, A. A teacher’s view about introductory programming teaching and learning: Difficulties, strategies and motivations. In Proceedings of the 2014 IEEE Frontiers in Education Conference (FIE), Madrid, Spain, 22–25 October 2014. [ Google Scholar ] [ CrossRef ]
- Tomai, E.; Reilly, C.F. The impact of math preparedness on introductory programming (CS1) success (abstract only). In Proceedings of the SIGCSE ′14: The 45th ACM Technical Symposium on Computer Science Education, Atlanta, GA, USA, 5–8 March 2014. [ Google Scholar ] [ CrossRef ]
- Lishinski, A.; Yadav, A.; Enbody, R.; Good, J. The influence of problem solving abilities on students’ performance on different assessment tasks in CS1. In Proceedings of the SIGCSE ′16: The 47th ACM Technical Symposium on Computing Science Education, Memphis, TN, USA, 2–5 March 2016; pp. 329–334. [ Google Scholar ] [ CrossRef ]
- Jokhan, A.; Chand, A.A.; Singh, V.; Mamun, K.A. Increased Digital Resource Consumption in Higher Educational Institutions and the Artificial Intelligence Role in Informing Decisions Related to Student Performance. Sustainability 2022 , 14 , 2377. [ Google Scholar ] [ CrossRef ]
- Sobral, S.R. Strategies on Teaching Introducing to Programming in Higher Education. In Advances in Intelligent Systems and Computing ; Springer: Cham, Switzerland, 2021. [ Google Scholar ] [ CrossRef ]
- Gomes, A.; Mendes, A.J.; Marcelino, M.J. Computer Science Education Research—An overview and some proposals. In Innovative Teaching Strategies and New Learning Paradigms in Computer Programming ; Queiroz, R., Ed.; IGI-Global: Hershey, PA, USA, 2015; pp. 1–29. [ Google Scholar ]
- Köhler, J.; Hidalgo, L.; Jara, J.L. Predicting Students’ Outcome in an Introductory Programming Course: Leveraging the Student Background. Appl. Sci. 2023 , 13 , 11994. [ Google Scholar ] [ CrossRef ]
- Liu, Y.; Fan, S.; Xu, S.; Sajjanhar, A.; Yeom, S.; Wei, Y. Predicting Student Performance Using Clickstream Data and Machine Learning. Educ. Sci. 2023 , 13 , 17. [ Google Scholar ] [ CrossRef ]
- Quille, K.; Bergin, S. CS1: How will they do? How can we help? A decade of research and practice. Comput. Sci. Educ. 2019 , 29 , 254–282. [ Google Scholar ] [ CrossRef ]
- Shahiri, A.M.; Husain, W.; Rashid, N.A. A Review on Predicting Student’s Performance Using Data Mining Techniques. Procedia Comput. Sci. 2015 , 72 , 414–422. [ Google Scholar ] [ CrossRef ]
- Alhothali, A.; Albsisi, M.; Assalahi, H.; Aldosemani, T. Predicting Student Outcomes in Online Courses Using Machine Learning Techniques: A Review. Sustainability 2022 , 14 , 6199. [ Google Scholar ] [ CrossRef ]
- Silva, C.; Fonseca, J. Educational data mining: A literature review. In Advances in Intelligent Systems and Computing ; Springer: Cham, Switzerland, 2017. [ Google Scholar ] [ CrossRef ]
- Bachhal, P.; Ahuja, S.; Gargrish, S. Educational Data Mining: A Review. J. Physics Conf. Ser. 2021 , 1950 , 012022. [ Google Scholar ] [ CrossRef ]
- Scherer, R.; Siddiq, F.; Viveros, B.S. The cognitive benefits of learning computer programming: A meta-analysis of transfer effects. J. Educ. Psychol. 2019 , 111 , 764–792. [ Google Scholar ] [ CrossRef ]
- Sobral, S.; Oliveira, C. Predicting Students’ Performance in Introductory Programming Courses: A Literature Review. In Proceedings of the 15th International Technology, Education and Development Conference, Online, 8–9 March 2021; pp. 7402–7412. [ Google Scholar ]
- Kitchenham, B. Procedures for Performing Systematic Reviews ; Keele University: Keele, UK; National ICT Australia: Sydney, Australia, 2004; Volume 33. [ Google Scholar ]
- Silva, M.; Shaffer, E.G.; Nytko, N.; Amos, J.R. A case study of early performance prediction and intervention in a computer science course. In Proceedings of the ASEE Annual Conference and Exposition, Conference Proceedings, Virtual Online, June 2020. [ Google Scholar ] [ CrossRef ]
- Jamjoom, M.; Alabdulkreem, E.; Hadjouni, M.; Karim, F.; Qarh, M. Early prediction for at-risk students in an introductory programming course based on student self-efficacy. Informatica 2021 , 45 , 1–9. [ Google Scholar ] [ CrossRef ]
- Sivasakthi, M.; Pandiyan, M. Machine Learning Algorithms to Predict Students’ Programming Performance: A comparative Study. J. Univ. Shanghai Sci. Technol. 2022 , 24 , 1–8. [ Google Scholar ]
- Ahadi, A.; Lister, R.; Haapala, H.; Vihavainen, A. Exploring machine learning methods to automatically identify students in need of assistance. In Proceedings of the ICER ′15: International Computing Education Research Conference, Omaha, NE, USA, 9–13 July 2015. [ Google Scholar ]
- Đambić, G.; Krajcar, M.; Bele, D. Machine learning model for early detection of higher education students that need additional attention in introductory programming courses. Int. J. Digit. Technol. Econ. 2016 , 1 , 1–11. [ Google Scholar ]
- Khan, I.; Al Sadiri, A.; Ahmad, A.R.; Jabeur, N. Tracking student performance in introductory programming by means of machine learning. In Proceedings of the 2019 4th MEC International Conference on Big Data and Smart City (ICBDSC), Muscat, Oman, 15–16 January 2019. [ Google Scholar ] [ CrossRef ]
- Veerasamy, A.K.; D’Souza, D.; Apiola, M.-V.; Laakso, M.-J.; Salakoski, T. Using early assessment performance as early warning signs to identify at-risk students in programming courses. In Proceedings of the 2020 IEEE Frontiers in Education Conference (FIE), Uppsala, Sweden, 21–24 October 2020. [ Google Scholar ] [ CrossRef ]
- Cooper, C. Using Machine Learning to Identify At-risk Students in an Introductory Programming Course at a Two-year Public College. Adv. Artif. Intell. Mach. Learn. 2022 , 2 , 407–421. [ Google Scholar ] [ CrossRef ]
- Shen, G.; Yang, S.; Huang, Z.; Yu, Y.; Li, X. The prediction of programming performance using student profiles. Educ. Inf. Technol. 2023 , 28 , 725–740. [ Google Scholar ] [ CrossRef ]
- Sunday, K.; Ocheja, P.; Hussain, S.; Oyelere, S.S.; Samson, B.O.; Agbo, F.J. Analyzing student performance in programming education using classification techniques. Int. J. Emerg. Technol. Learn. (iJET) 2020 , 15 , 127–144. [ Google Scholar ] [ CrossRef ]
- Sivasakthi, M. Classification and prediction based data mining algorithms to predict students’ introductory programming performance. In Proceedings of the 2017 International Conference on Inventive Computing and Informatics (ICICI), Coimbatore, India, 23–24 November 2017; pp. 346–350. [ Google Scholar ]
- Naser, M.Z.; Alavi, A.H. Error Metrics and Performance Fitness Indicators for Artificial Intelligence and Machine Learning in Engineering and Sciences. Arch. Struct. Constr. 2021 , 3 , 499–517. [ Google Scholar ] [ CrossRef ]
- Sessions, V.; Valtorta, M. The effects of data quality on machine learning algorithms. In Proceedings of the 2006 International Conference on Information Quality, ICIQ 2006, Cambridge, MA, USA, 10–12 November 2006. [ Google Scholar ]
- Realinho, V.; Machado, J.; Baptista, L.; Martins, M.V. Predicting Student Dropout and Academic Success. Data 2022 , 7 , 146. [ Google Scholar ] [ CrossRef ]
- Sala, R.; Zambetti, M.; Pirola, F.; Pinto, R. How to select a suitable machine learning algorithm: A feature-based, scope-oriented selection framework. In Proceedings of the Summer School “Francesco Turco”, Palermo, Italy, 12–14 September 2018. [ Google Scholar ]
- Liu, Y.; Zhou, Y.; Wen, S.; Tang, C. A Strategy on Selecting Performance Metrics for Classifier Evaluation. Int. J. Mob. Comput. Multimedia Commun. 2014 , 6 , 20–35. [ Google Scholar ] [ CrossRef ]
Click here to enlarge figure
Datasets | Papers |
---|---|
Questionnaires | [ , , ] |
Academic records | [ , , , , , , , , ] |
Personal data | [ , , , , ] |
Dataset | Algorithm | Metric | Value |
---|---|---|---|
Academic records Questionnaires | Gradient Boosting | Accuracy | 82% |
Recall | 57% | ||
Precision | 56% | ||
Specificity | 88% |
Dataset | Algorithm | Metric | Value |
---|---|---|---|
Academic records Questionnaires | Decision Tree | Accuracy | 99.18% |
Precision | 100% | ||
Recall | 98.7% | ||
KNN | Accuracy | 98.36% | |
Precision | 99.3% | ||
Recall | 100% | ||
Naïve Bayes | Accuracy | 96.72% | |
Precision | 97.4% | ||
Recall | 97.4% | ||
SVM | Accuracy | 99.18% | |
Precision | 100% | ||
Recall | 98.7% |
Dataset | Algorithm | Metric | Value |
---|---|---|---|
Academic records Personal data | ADTree | Accuracy | 83% |
Bayesian Network | Accuracy | 76% | |
Conjunctive Rule | Accuracy | 86% | |
Decision Stump | Accuracy | 90% | |
Decision Table | Accuracy | 76% | |
J48 | Accuracy | 89% | |
Naïve Bayes | Accuracy | 86% | |
PART | Accuracy | 76% | |
Random Forest | Accuracy | 90% |
Dataset | Algorithm | Metric | Value |
---|---|---|---|
Academic records | Logistic Regression | Precision | 67% |
Recall | 92% | ||
F1-Score | 77% |
Dataset | Algorithm | Metric | Value |
---|---|---|---|
Academic records Personal data | Decision Table | Accuracy | 83% |
F1-Score | 79% | ||
J48 | Accuracy | 88% | |
F1-Score | 88% | ||
Naïve Bayes | Accuracy | 84% | |
F1-Score | 83% |
Dataset | Algorithm | Metric | Value |
---|---|---|---|
Academic records | Random Forest | Accuracy | 60% |
Sensitivity | 77% |
Dataset | Algorithm | Metric | Value |
---|---|---|---|
Academic records Personal data | PNN | Accuracy | 91% |
Dataset | Algorithm | Metric | Value |
---|---|---|---|
Questionnaires | Decision Tree | Accuracy | 85.10% |
Precision | 86.57% | ||
Recall | 84.89% | ||
F1-Score | 85.72% | ||
KNN | Accuracy | 84.28% | |
Precision | 85.82% | ||
Recall | 83.46% | ||
F1-Score | 84.62% | ||
Naïve Bayes | Accuracy | 91.02% | |
Precision | 92.21% | ||
Recall | 90% | ||
F1-Score | 91.09% | ||
Random Forest | Accuracy | 87.55% | |
Precision | 86.06% | ||
Recall | 88.60% | ||
F1-Score | 87.31% | ||
SVM | Accuracy | 88.77% | |
Precision | 89.79% | ||
Recall | 88% | ||
F1-Score | 88.88% |
Dataset | Algorithm | Metric | Value |
---|---|---|---|
Personal data | J48 | Accuracy | 92.03% |
Multilayer Perceptron | Accuracy | 93.23% | |
Naïve Bayes | Accuracy | 84.46% | |
REPTree | Accuracy | 91.03% | |
Sequential Minimal Optimization | Accuracy | 90.03% |
Dataset | Algorithm | Metric | Value |
---|---|---|---|
Academic records Personal data | DNN | RMSE | 12.68 |
SVM | RMSE | 14.07 |
Dataset | Algorithm | Metric | Value |
---|---|---|---|
Academic records | ID3 | Accuracy | 85.4% |
Precision | 84.8% | ||
Recall | 86% | ||
F1-Score | 85% | ||
J48 | Accuracy | 87% | |
Precision | 80% | ||
Recall | 87% | ||
F1-Score | 82.8% |
Algorithms | Papers |
---|---|
ADTree | [ ] |
Bayesian Network | [ ] |
Conjunctive Rule | [ ] |
Decision Stump | [ ] |
Decision Table | [ , ] |
Decision Tree | [ , ] |
DNN | [ ] |
Gradient Boosting | [ ] |
ID3 | [ ] |
J48 | [ , , , ] |
KNN | [ , ] |
Logistic Regression | [ ] |
Multilayer Perceptron | [ ] |
Naïve Bayes | [ , , , , ] |
PART | [ ] |
PNN | [ ] |
Random Forest | [ , , ] |
REPTree | [ ] |
Sequential Minimal Optimization | [ ] |
SVM | [ , , ] |
The statements, opinions and data contained in all publications are solely those of the individual author(s) and contributor(s) and not of MDPI and/or the editor(s). MDPI and/or the editor(s) disclaim responsibility for any injury to people or property resulting from any ideas, methods, instructions or products referred to in the content. |
Share and Cite
Pires, J.P.J.; Brito Correia, F.; Gomes, A.; Borges, A.R.; Bernardino, J. Predicting Student Performance in Introductory Programming Courses. Computers 2024 , 13 , 219. https://doi.org/10.3390/computers13090219
Pires JPJ, Brito Correia F, Gomes A, Borges AR, Bernardino J. Predicting Student Performance in Introductory Programming Courses. Computers . 2024; 13(9):219. https://doi.org/10.3390/computers13090219
Pires, João P. J., Fernanda Brito Correia, Anabela Gomes, Ana Rosa Borges, and Jorge Bernardino. 2024. "Predicting Student Performance in Introductory Programming Courses" Computers 13, no. 9: 219. https://doi.org/10.3390/computers13090219
Article Metrics
Article access statistics, further information, mdpi initiatives, follow mdpi.
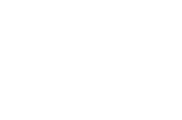
Subscribe to receive issue release notifications and newsletters from MDPI journals
Development and validation of the high school students’ Mathematics Discourse Feedback Skills Scale (MDFSS)
- Published: 07 September 2024
Cite this article
- Hao Chen 1 , 2 ,
- Sanyi Tang 3 ,
- Shang Zhang 1 ,
- Jie Xu 1 &
- Guangsheng Wang 3
This study aimed to develop an instrument for assessing high school students’ mathematics discourse feedback skills (MDFS) in order to measure their feedback literacy performance in mathematics. First, the researcher constructed a theoretical framework of MDFS, including comparative analysis, expressing communication, mathematical reasoning, monitor and adjust, diagnostic evaluation, and implementation capacity, through literature review, and designed the mathematics discourse feedback skills scale (MDFSS) questions accordingly. Subsequently, 9 experts conducted two rounds of content validity tests on the theoretical framework and scale questions, while 32 high school student volunteers conducted surface validity tests. Then, 273 high school students participated in the item analysis of the scale. Ultimately, 1681 high school students assessed the structural validity of the scale. In these assessments, exploratory factor analysis was conducted on 841 high school students, and confirmatory factor analysis with first-order and second-order models was conducted on 840 students. The study also conducted reliability, validity, and measurement invariance tests on the survey questionnaire. Based on the results of these analyses, the researcher confirmed that the final version of the scale consisted of 24 items. The results of the study indicated that the scale provided a valid evidence for measuring the MDFS of high school students. The study is of great significance to academic and educational practice, as it not only deepens the research on student feedback literacy in mathematics, but also provides a valuable reference tool for improving the academic quality of mathematics among high school students in China and other Asian countries.
This is a preview of subscription content, log in via an institution to check access.
Access this article
Subscribe and save.
- Get 10 units per month
- Download Article/Chapter or eBook
- 1 Unit = 1 Article or 1 Chapter
- Cancel anytime
Price includes VAT (Russian Federation)
Instant access to the full article PDF.
Rent this article via DeepDyve
Institutional subscriptions
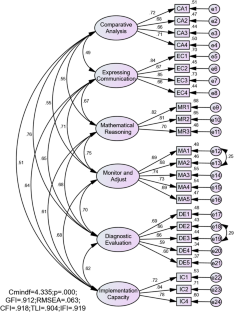
Data availability
The data generated during the current study are not publicly available since they constitute an excerpt of research in progress. Data are available from the corresponding author on reasonable request.
Al-Nuaimi, M. N., & Al-Emran, M. (2024). Development and validation of ICT unethical behavior scale among undergraduate students. Current Psychology, 43 (10), 8760–8776. https://doi.org/10.1007/s12144-023-05038-6
Article Google Scholar
Attard, C., Edwards-Groves, C., & Grootenboer, P. (2018). Dialogic practices in the mathematics classroom. In J. Hunter, P. Perger, & L. Darragh (Eds.), Making waves, opening spaces (Proceedings of the 41st annual conference of the Mathematics Education Research Group of Australasia) (pp. 122–129). MERGA
Boekaerts, M., & Corno, L. (2005). Self-regulation in the classroom: A perspective on assessment and intervention. Applied Psychology, 54 (2), 199–231. https://doi.org/10.1111/j.1464-0597.2005.00205.x
Carless, D., & Boud, D. (2018). The development of student feedback literacy: Enabling uptake of feedback. Assessment & Evaluation in Higher Education, 43 (8), 1315–1325. https://doi.org/10.1080/02602938.2018.1463354
Carless, D. (2019). Feedback loops and the longer-term: towards feedback spirals. Assessment & evaluation in Higher Education, 44 (5), 705–714. https://doi.org/10.1080/02602938.2018.1531108
Carless, D. (2020). Longitudinal perspectives on students’ experiences of feedback: A need for teacher-student partnerships. Higher Education Research and Development, 39 (3), 425–438. https://doi.org/10.1080/07294360.2019.1684455
Cheung, G. W., & Rensvold, R. B. (2002). Evaluating goodnessof-fit indexes for testing measurement invariance. Structural Equation Modeling, 9 (2), 233–255. https://doi.org/10.1207/S15328007SEM0902_5
Chong, S. W. (2021). Reconsidering student feedbackliteracy from an ecological perspective. Assessment and Evaluation in Higher Education, 46 (1), 92–104. https://doi.org/10.1080/02602938.2020.1730765
Cortez, C. P., Osenar-Rosqueta, A. M. F., & Prudente, M. S. (2023). Cooperative-flipped classroom under online modality: En hancing students’ mathematics achievement and critical thinking attitude. International Journal of Educational Research, 120 ,. https://doi.org/10.1016/j.ijer.2023.102213
DeKleijn, R. A. M. (2023). Supporting student and teacher feedback literacy: an instructional model for student feedback processes. Assessment & Evaluation in Higher Education, 48 (2), 186–200. https://doi.org/10.1080/02602938.2021.1967283
DeVellis, R. F. (2003). Scale development: Theory and applications (2nd ed.). Sage.
Fornell, C., & Larcker, D. F. (1981). Evaluating structural equation models with unobservable variables and measurement error. Journal of Marketing Research, 18 , 39–50. https://doi.org/10.2307/3151312
Goldman, Z. W. (2018). Communicating competently in graduate school: a conditional process analysis using self-regulation. Western Journal of Communication, 83 (1), 75–93. https://doi.org/10.1080/10570314.2017.1416488
Hattie, J., & Timperley, H. (2007). The power of feedback. Review of Educational Research, 77 (1), 81–112. https://doi.org/10.3102/003465430298487
Hair, J. F., Black, W. C., Babin, B. J., & Anderson, R. E. (2010). Multivariate data analysis (7th ed.). Prentice-Hall.
Harks, B., Rakoczy, K., Hattie, J., Besser, M., & Klieme, E. (2014). The effects of feedback on achievement, interest and self-evaluation: the roleof feedback’s perceived usefulness. Educational Psychology, 34 (3), 269–290. https://doi.org/10.1080/01443410.2013.785384
Hemmi, K., & Ryve, A. (2015). Effective mathematics teaching in Finnish and Swedish teacher education discourses. Journal of Mathematics Teacher Education, 18 , 501–521. https://doi.org/10.1007/s10857-014-9293-4
Heron, M., Donaghue, H., & Balloo, K. (2023). Observational feedback literacy: designing post observation feedback for learning. Teaching in Higher Education . https://doi.org/10.1080/13562517.2023.2191786
Hu, X. (2023). Research on the evaluation and cultivation of senior high school students’ science critical thinking. Graduate Dissertation.
Ilhan, A., Pocan, S., & Gemcioglu, M. (2022). The effect of mathematics class commitment and anxiety on mathematics success: A path analysis study. Education and Urban Society, 54 (2), 186–204. https://doi.org/10.1177/00131245211028621
Jeannotte, D., & Kieran, C. (2017). A conceptual model of mathematical reasoning for school mathematics. Educational Studies in Mathematics,96 (1). https://doi.org/10.1007/s10649-017-9761-8 .
Kamid, K., Kurniawan, D., & Rahman, A. (2022). A comparativestudy: Students’ process skills and students’ analytical thinking with learningmodels. Jurnal Penelitian dan Evaluasi Pendidikan, 26 (2), 233–250. https://doi.org/10.21831/pep.v26i2.53326
Ketonen, L., Nieminen, P., & Hähkiöniemi, M. (2020). The Development of Secondary Students’ Feedback Literacy: Peer Assessment as an Intervention. The Journal of Educational Research, 113 (6), 407–417. https://doi.org/10.1080/00220671.2020.1835794
Kiemer, K., Groschner, A., Pehmer, A.-K., & Seidel, T. (2015). Effects of a Classroom Discourse Intervention on Teachers’ Practice and Students’ Motivation to Learn Mathematics and Science. Learning and Instruction, 35 , 94–103. https://doi.org/10.1016/j.learninstruc.2014.10.003
Kline, R. B. (2016). Principles and practice of structural equation modeling (4th Ed). Guilford Press.
Knuth, E., & Peressini, D. (2001). Unpacking the nature of discourse in mathematics classrooms. Mathematics Teaching in the Middle SchoOl, 6 (5), 320–325. https://doi.org/10.2307/41180959
Koyama, M. (2019). Issues of mathematics curriculum in Japan: changing curriculum policies and developing curriculum frameworks for mathematics. In C. Vistro-Yu & T. Toh (Eds.), School mathematics curricula: mathematics education– an Asian perspective (pp. 171–187). Springer. https://doi.org/10.1007/978-981-13-6312-2_9 .
Li, F., & Han, Y. (2021). Student feedback literacy in L2 disciplinary writing: insights from international graduate students at a UK university. Assessment & Evaluation in Higher Education, 47 (2), 198–212. https://doi.org/10.1080/02602938.2021.1908957
Malecka, B., Boud, D., & Carless, D. (2020). Eliciting, processing and enacting feedback: mechanisms for embedding student feedback literacy within the curriculum. Teaching in Higher Education, 27 (7), 908–922. https://doi.org/10.1080/13562517.2020.1754784
Malecka, B., Boud, D., Tai, J., & Ajjawi, R. (2022). Navigating feedback practices across learning contexts: implications for feedback literacy. Assessment & Evaluation in Higher Education, 47 (8), 1330–1344. https://doi.org/10.1080/02602938.2022.2041544
Merenda, P. F. (1997). A guide to the proper use of factor analysis in the conduct and reporting of research: Pitfalls to avoid. Measurement and Evaluation in Counseling and Development, 30 (3), 156–154.
Molloy, E., Boud, D., & Henderson, M. (2020). Developing a learning-centred framework for feedback literacy. Assessment & Evaluation in Higher Education, 45 (4), 527–540. https://doi.org/10.1080/02602938.2019.1667955
MOEPRC. (2020). Curriculum standard for general high school mathematics (2017 edition revised in 2020) . People’s Education Press.
Nicol, D. (2021). The Power of Internal Feedback: Exploiting Natural Comparison Processes. Assessment & Evaluation in Higher Education, 46 (5), 756–778. https://doi.org/10.1080/02602938.2020.1823314
Nieminen, J. H., & Carless, D. (2022). Feedback literacy: a critical review of an emerging concept. Higher Education, 85 , 1381–1400. https://doi.org/10.1007/s10734-022-00895-9
Rahmatika, D., & Waluya, S. B. (2023). Students’ mathematical reasoning ability viewed from self-regulated learning in the missouri mathematics project learning with open-ended approach. Unnes Journal of Mathematics Education, 12 (1), 84–92. https://doi.org/10.15294/ujme.v12i1.66188
Santos, L., & Semana, S. (2015). Developing mathematicswritten communication through expository writing supported by assessmentstrategies. Educational Studies in Mathematics, 88 (1), 65–87. https://doi.org/10.1007/s10649-014-9557-z
Sfard, A. (2008). Thinking as communicating: Human development, the growth of discourses, and mathematizing . Cambridge University Press.
Book Google Scholar
Sfard, A. (2012). Introduction: Developing mathematical discourse - some insights from communicational research. International Journal of Educational Research, 51–52 , 1–9. https://doi.org/10.1016/j.ijer.2011.12.013
Si, S. X., & Cullen, J. B. (1998). Response categories andpotential cultural bias: Effects of an explicit middle point in cross-culturalsurveys. International Journal of Organizational Analysis, 6 (3), 218–230. https://doi.org/10.1108/eb028885
Song, B. K. (2022). Bifactor modelling of the psychological constructs of learner feedback literacy: conceptions of feedback, feedback trust and self-efficacy. Assessment & Evaluation in Higher Education, 47 (8), 1444–1457. https://doi.org/10.1080/02602938.2022.2042187
Steen-Utheima, A., & Wittek, A. L. (2017). Dialogic feedback and potentialities for student learning. Learning, Culture and Social Interaction, 15 , 18–30. https://doi.org/10.1016/j.lcsi.2017.06.002
Tai, J., Ajjawi, R., Boud, D., Dawson, P., & Panadero, E. (2018). Developing evaluative judgement:enabling students to make decisions about the quality of work. High Educ, 76 (3), 467–481. https://doi.org/10.1007/s10734-017-0220-3
Temple, C., & Doerr, H. (2012). Developing fluency in the mathematical register through conversation in a tenth-grade classroom. Educational Studies in Mathematics, 81 (3), 287–306. https://doi.org/10.1007/s10649-012-9398-6
Vani, M. F., Lucibello, K. M., & Sabiston, C. M. (2024). Development and validation of the body-related embarrassment scale (BREM). Current Psychology, 43 (11), 10467–10483. https://doi.org/10.1007/s12144-023-05183-y
Vygotsky, L. S. (1987). Zone of proximal development. In M. Cole, V. John-Steiner, S. Scribner, & E. Souberman (Eds.), Mind in society: The development of higher psychological processes (pp. 52–91). Harvard University Press.
Google Scholar
Wang, M., Fredricks, J. A., Ye, F., Hofkens, T. L., & Linn, J. S. (2016). The math and science engagement scales: Scale development, validation, and psychometric properties. Learning and Instruction, 43 , 16–26. https://doi.org/10.1016/j.learninstruc.2016.01.008
Wong, K. Y., Koyama, M., & Lee, K. H. (2014). Mathematics curriculum policies: a framework with case studies from Japan, Korea, and Singapore. In Y. Li & G. Lappan (Eds.), Mathematics curriculum in school education. advances in mathematics education . Springer. https://doi.org/10.1007/978-94-007-7560-2_5
Chapter Google Scholar
Wu, X., Zhang, Y., Wu, R., et al. (2022). A comparative study on cognitive diagnostic assessment of mathematical key competencies and learning trajectories. Current Psychology, 41 , 7854–7866. https://doi.org/10.1007/s12144-020-01230-0
Xu, B., Lu, X., Yang, X., & Bao, J. (2022). Mathematicians’, mathematics educators’, and mathematics teachers’ professional conceptions of the school learning of mathematical modelling in China. ZDM Mathematics Education, 54 , 679–691. https://doi.org/10.1007/s11858-022-01356-4
Yang, L., Chen, Y., Li, X., & Li, T. (2017). The relationship between parenting styles and self monitoring learning of the senior high school students-The mediating effects of core self-evaluation. Journal of Zhejiang University (Science Edition) , 44 (2), 214–220.
Zhang, J., Gao, M., Holmes, W., Mavrikis, M., & Ma, N. (2019). Interaction patterns in exploratory learning environments for mathematics: a sequential analysis of feedback and external representations in Chinese schools. Interactive Learning Environments, 29 (7), 1211–1228. https://doi.org/10.1080/10494820.2019.1620290
Zhan, Y. (2022). Developing and validating a student feedback literacy scale. Assessment & Evaluation in Higher Education, 47 (7), 1087–1100. https://doi.org/10.1080/02602938.2021.2001430
Download references
Acknowledgements
The authors would like to thank all those who participated in this study. They are also grateful to Professor Robert A. Cheke from the UK for language editing, and to the Editor-in-Chief, Associate Editors, and anonymous reviewers for their valuable comments on this paper.
This study is supported by the National Natural Science Foundation of China (NSFC: 12031010).
Author information
Authors and affiliations.
Faculty of Education, Shaanxi Normal University, 710062, Xi’an, People’s Republic of China
Hao Chen, Shang Zhang & Jie Xu
Affiliated Secondary School, Xizang Minzu University, 712082, Xianyang, People’s Republic of China
School of Mathematics and Statistics, Shaanxi Normal University, 710119, Xi’an, People’s Republic of China
Sanyi Tang & Guangsheng Wang
You can also search for this author in PubMed Google Scholar
Contributions
Hao Chen drafted the manuscript. Sanyi Tang and Guangsheng Wang served as the research advisor. Guangsheng Wang and Hao Chen contributed significantly to the conception, the data analysis, and manuscript revision. Shang Zhang and Jie Xu collected the data and worked as writer’s assistant. All authors contributed to the article and approved the submitted version.
Corresponding author
Correspondence to Guangsheng Wang .
Ethics declarations
Ethical approval.
This study gained ethical approval from the Ethics Committee of the Faculty of Education, Shaanxi Normal University in China.
Informed consent
All participants in the study provided informed consent.
Conflict of interest
No potential conflict of interest was reported by the authors.
Additional information
Publisher’s note.
Springer Nature remains neutral with regard to jurisdictional claims in published maps and institutional affiliations.
Rights and permissions
Springer Nature or its licensor (e.g. a society or other partner) holds exclusive rights to this article under a publishing agreement with the author(s) or other rightsholder(s); author self-archiving of the accepted manuscript version of this article is solely governed by the terms of such publishing agreement and applicable law.
Reprints and permissions
About this article
Chen, H., Tang, S., Zhang, S. et al. Development and validation of the high school students’ Mathematics Discourse Feedback Skills Scale (MDFSS). Curr Psychol (2024). https://doi.org/10.1007/s12144-024-06578-1
Download citation
Accepted : 15 August 2024
Published : 07 September 2024
DOI : https://doi.org/10.1007/s12144-024-06578-1
Share this article
Anyone you share the following link with will be able to read this content:
Sorry, a shareable link is not currently available for this article.
Provided by the Springer Nature SharedIt content-sharing initiative
- High school students
- Mathematics discourse
- Discourse feedback
- Feedback literacy
- Skill scales
- Find a journal
- Publish with us
- Track your research

COMMENTS
A Literature Review of Academic Performance, an Insight into Factors and their Influences on Academic Outcomes of Students at Senior High Schools January 2021 Open Access Library Journal 08(06):1-14
This review investigates the application of different techniques of data mining and machine learning to; Predict the performance of students at risk in academic institutions. Determine and predict students' dropout from on-going courses. Evaluate students' performance based on dynamic and static data.
Factors Affecting Students' Academic Performance: A review
When students reflect on relationships between formal academic knowledge and concrete learning experiences, a deeper understanding develops (Ghanizadeh, 2017).Studies have shown that experiential learning environments that provide opportunities for reflection enhance students' academic success and performance (Dyment and O'Connell, 2011; Peltier et al., 2005) and increase examination ...
This paper provides a systematic literature review (SLR) of 347 papers, focusing on the: indicators of academic performance, relation between student part-time work and academic performance, and drivers of students' decision to work. ... This paper's contributions lie in systematising positive and negative impacts of student work on ...
Educational Data Mining plays a critical role in advancing the learning environment by contributing state-of-the-art methods, techniques, and applications. The recent development provides valuable tools for understanding the student learning environment by exploring and utilizing educational data using machine learning and data mining techniques. Modern academic institutions operate in a ...
The review results indicated that various Machine Learning (ML) techniques are used to understand and overcome the underlying challenges; predicting students at risk and students drop out prediction.
Moreover, research in predicting student performance seeks to determine interrelated features and to identify the underlying reasons why certain features work better than others. This working group report presents a systematic literature review of work in the area of predicting student performance.
Predicting Academic Performance: A Systematic Literature ...
To our understanding, this is the first systematic review focusing on student performance in the online setting, and this work will help teachers and institutions develop an understanding of what drives academic performance of online students so that they can create the appropriate e-environment for e-teaching and e-learning. ... The literature ...
The good academic performance of students at the Senior High School is of paramount importance in every educational system. Meanwhile, numerous factors influence the academic performance of students and have been researched, but many problems persist. A literature review in this area would provide the gaps and areas that need more research and will go a long way to curb the situation.
In this study, we performed a systematic literature review of student performance prediction studies in three different databases between 2010 and 2020. The results are presented as percentages by categorizing them as either model, dataset, validation, evaluation, or aims. The common points and differences in the studies are determined, and ...
Determinants of poor academic performance among ...
The effects of attendance and high school GPA on student ...
This review presents a meta-analysis of performance influencing factors identified by the researchers. It also discusses the existing student performance prediction studies in the context of their aim and time of prediction. It seems that the literature on student performance prediction after course commencement is rich.
Researchers agree that teachers are one of the most important school-based resources in determining students' future academic success and lifetime outcomes (Chetty et al. 2014; Rivkin et al. 2005; Rockoff 2004). As a consequence, there has been a strong emphasis on improving teacher effectiveness as a means to enhancing student learning.
This study performs a comprehensive literature review of student performance prediction using EDM techniques, including various research from 2002 to 2021. Our study is aimed to provide a ...
Analysing the Impact of Social Media on Students ...
A Practitioner's Literature Review August 28, 2024. Launch the full report Research has shown that teacher beliefs shape classroom dynamics, influence student performance, and drive achievement disparities, especially across racial and socioeconomic lines. However, interventions can help mitigate these beliefs and empower teachers to raise ...
Literature Survey on Student's Performance Prediction in Education using Data Mining Techniques 42 2. Research Questions Formation and Search Strategy for Literature Review The main purpose of literature survey is to find out new techniques to work on the old data set and then find out some new information form that.
Literature Review On Student Performance. This chapter elaborates the relation of previous research study with the objectives in this research. The important parameters, guidelines, quotes or findings from earlier researches are mentioned. According to U.S. Department of Education, student performance defined as academic progress of a single ...
In another review by Kotsiantis, Sotiris B. [23] proposed a decision support system for a tutor to predict students' performance. This review considers student demographic data, e-learning system logs, academic data, and admission information. The dataset is comprised of 354 student's data having 17 attributes each.
Student performance prediction attempts to forecast a student's grade before enrolling in a course or completing an exam. The goal of this paper is to present a systematic literature review on ...
The importance of accurately predicting student performance in education, especially in the challenging curricular unit of Introductory Programming, cannot be overstated. As institutions struggle with high failure rates and look for solutions to improve the learning experience, the need for effective prediction methods becomes critical. This study aims to conduct a systematic review of the ...
This study aimed to develop an instrument for assessing high school students' mathematics discourse feedback skills (MDFS) in order to measure their feedback literacy performance in mathematics. First, the researcher constructed a theoretical framework of MDFS, including comparative analysis, expressing communication, mathematical reasoning, monitor and adjust, diagnostic evaluation, and ...
integrating the use of artificial intelligence (ai) to promote physical activity: the effects on lifestyle and academic performance of university students. a literature review integrazione dell ...