K-means
Precision, recall error rate FPR, accuracy hit and miss rate. | There is a significance difference between K-means and BPNN. BPNN model has higher accuracy comparing with K-means. BPNN accuracy = 93.1%. K-means accuracy = 79.9%. | Real credit card data/European cardholders | Comparing the effect of combing these two models together so as to optimise the accuracy. | A2 | LR | Accuracy, recall, precision | The model reached high performance using imbalanced dataset. L-BFGS is 0.980. Lib-linear is 0.9816. Newton-CG is 0.9812. Sag is 0.997. Saga is 0.996. | Real data/European cardholders | NA |
A3 | CNN BILSTM | Confusion matrix, accuracy, precision, recall | The proposed model (CNN-BI-LSTM-ATTENTION) achieved high accuracy in fraud detecting. Adding attention layer enhances performance. Accuracy is 95%. | IEEE-CIS fraud detection from | NA |
A4 | ANN | Accuracy Precision Recall | The ANN proposed model is best suited for detecting fraud. The accuracy around 100%. | NA | Combining this algorithm with other algorithms. |
A5 | HMM | NA | Applying HMM model to detect credit card fraud would be successful. | NA | NA |
A6 | RF SMOTE | Sensitivity Specificity Precision F-measure Accuracy Misclassification rate, ROC | The model showed high performance. When using RF the large number of datasets can be processed automatically. Quick RF classifier accuracy with imbalanced dataset is 98%. Quick RF Classifier accuracy with balanced dataset is 99%. | Real-world data/UCSD FICO/2009 | NA |
A7 | ADA boost majority balloting NB, QDA, LR, DT, RF, NN, KNN, and SVM. | -Accuracy -Matthews correlation coefficient (MCC) | Results showed that using bulk balloting technique achieves high accuracy in detecting fraud. NB: 0.9458. QDA: 0.9544. LR:0.9913 DT: 0.9837. RF: 0.9869. NN:0.971 KNN: 0.9718. SVM: 0.8526. | Genuine world MasterCard data set. | Procedures be stretched out to the internet becoming acquainted with designs. |
A8 | K-means RF, J48 SVM | Accuracy | Results showed that RF is better on global dataset with 92.1% accuracy. K-means: 85.6%. RF: 92.1%. J48 DT: 89.3%. SVM: 89.9%. | Two types of data: Global/Bank. User dataset | For this model, the transaction time is required. |
A9 | FraudMiner RUSBoost Bagged KNN SVM | Sensitivity False alarm rate Balanced Classification rate, MCC | This model showed great performance with catch rate 85.3% and MCC of 0.83. | Public dataset/Provided by FISCO/UCSD | NA |
A10 | Autoencoder LR | Confusion matrix Accuracy Recall F1-score Precision | Results showed that proposed model can detect fraud transaction between 64%, 79%, and 91%. This model is better than LR (57%) with unbalanced dataset. The model solved data balancing problem. : accuracy is 97.23. Recall is 0.90. Precision is 0.06. The F1-score is 0.12. While results on accuracy is 99.91. Recall is 0.57. Precision is 0.93 and F1-score is 0.71. | Real dataset from ULB | Compare the performance of this model with other classification algorithms. |
A11 | LR, KNN | Confusion matrix Accuracy Sensitivity Error rate | The LR-based model is the best comparing with KNN and voting classifier. Accuracy is 97.2%. Sensitivity is 97%. Error rate is 2.8%. | Real dataset/European cardholders | Proposed model suffers in the response time. |
A12 | LSTM | Accuracy Loss rate Execution time | Results showed great performance of LSTM comparing with Autoencoder. Model accuracy is 99.95%. | Real dataset/European cardholders | Calculate timing and location of fraud |
A13 | LR, MLP, XGBoost, K-fold cross, RF, Bagging Gradient Boosting, Voting, KNN SVM, GNB. | Accuracy Confusion matrix | MLP achieved highest accuracy comparing with 15 algorithms. The accuracy is 98% | Real dataset/European cardholders | Further research of MLP to increase the detection performance. |
A14 | LR RF. XG CatBoost (CB), | F1-score AUC Savings | Results showed that the CatBoost obtained the best savings with 0.7158 alone. When applying SMOTE the savings is 0.971. When applying SMOTE and BMR, the saving is 0.9762. XGBoost achieved the best saving 0.757 when applying BMR without the SMOTE. XG + BMR: F1-score is (0.2890). AUC is (0.9699). Savings is (0.7570). CB + SMOTE + BMR: F1-score is (0.8250). AUC is (0.9999). Savings is (0.9762). | Real dataset/European cardholders | Using another dataset. Also testing XG and CB |
A15 | LR, RF KNN DT | Accuracy Precision Recall | The results show that RF achieved highest performance. RF: accuracy (95.19%), precision (0.9794), recall (0.9226). | Real dataset/Europeans cardholders | Other data balancing techniques be explored. |
A16 | SVM RUSBoost LR, MLP, DT, KNN, AdaBoost, RF | Accuracy Precision Specificity F1-score AUPR, ROC | The results showed that CtRUSBoost outperformed other algorithms. Results scores on three dataset: A, B, and C. sensitivity (96.30), specificity (85.60), precision (94.20), F1-score (88.60). Sensitivity (99.60), specificity (98.70), precision (95.70), F1-score (97.60). Dataset C: sensitivity 100), specificity (99.80), precision (99.30), F1-score (99.60). | Three datasets from (A, B, C) | Customized the model and adding new algorithms. |
A17 | Social spider optimisation (SSO), ant colony optimisation (ACO), ANN | Sensitivity Specificity Accuracy F-score Kappa | The model SSO-ANN achieved high performance with 93.20% accuracy on Germane dataset, and 92.82% on Kaggle dataset. | Benchmark dataset. Kaggle dataset | Improving the model by using clustering techniques. |
A18 | Deep ensemble algorithm (DEAL). CNN. DNN. MLP, Auto encoder. SVM, LR | Mean absolute error (MAE) Fraud catching rate (FCR) Accuracy | DEAL model obtained high performance in detecting fraud. Model accuracy is 99.81% | Real dataset/Europeans cardholders | Using AI and IoT in cloud computing |
A19 | SVM, KNN, ANN | Confusion matrix, accuracy, precision, recall | ANN provides high accuracy in detecting fraud comparing with the unsupervised algorithms. | Real dataset/Europeans cardholders | Na |
A20 | DT IFDTC4.5 intuitionistic fuzzy logic | Accuracy, sensitivity, false positive rate, specificity | IFDTC4.5 outperforms existing techniques. The model able to detect fraud efficiently. However, still the frauds cannot be eliminated by 100%. | Singaporean bank and one similar synthetic data set. | Add multi factor authentication using the biometrics like iris, voice . |
A21 | NB, DT, RF, CNN | Precision, Recall, Accuracy | Algorithms like NB, DT, RF and CNN are used. These algorithms are used as single models. Then these are used as hybrid models using majority voting technique. Adaptive boost also used in the model. | Publicly available credit card data set. | This model will extend to online model. |
A22 | SVM, NB, KNN, RF | Accuracy Sensitivity Specificity Precision | Results showed that RF performs better than other algorithms. Applying sampling approach will improve the performance. NB: 97.80%. SVM: 97%. KNN: 46.98%. RF: 98.23%. | Real dataset/European cardholders/ULB | Using huge dataset instead of sampling techniques |
A23 | Generative adversarial networks GANs | AUC AUPRC Recall F1-score Precision | The results show that applying Wasserstein-GAN will improve detecting fraudulent transactions comparing with traditional GAN. WCGAN model achieves: AUC is 0.948. AUPRC is 0.717. Recall is 0.6420. Precision is 0.852. F1-score is 0.710. | NA | NA |
A24 | LR, KNN, RF, NB, MLP, AdaBoost, pipeling | Accuracy Precision Recall, F1-score | The results showed that applying pipeling can improve the model’s performance. Accuracy: 00.99%. Precision: 0.84. Recall: 0.86. F1-score: 0.85. | Real dataset/European cardholders | NA |
A25 | GNB, LR, DT, RF | Accuracy Recall Precision F1-score, MSE | The result showed that DT algorithm is the best with an accuracy: 0.999. Recall: 0.782. Precision: 0.766. F1-score: 0.774. MSE : 0.0008 | Real dataset/European cardholders/ULB | NA |
A26 | RNN | Accuracy, recall, precision F1-score, MSE | The result showed that RNN model is capable in detecting fraud. The accuracy is 99.87%. MSE is 0.01. F1-score is 0.99. | Synthetic dataset and real dataset | NA |
A27 | DT, NB, SVM AdaBoost | Accuracy Sensitivity Specificity Precision ROC, F1-measure | The results showed that applying Boosting with DT outperforms other methods. The model obtained highest accuracy of 98.3%. F measure is 93.98%. Using boosting techniques improve the performance. | Real dataset/Europeans cardholders | NA |
A28 | DT,SVM, k-means Optimal resampling strategy, C4.5 DT AdaBoost | Accuracy Sensitivity Cost sensitive | The suggested model obtained high performance with 96.59% accuracy and 67.52% sensitivity. | Real dataset/CB bank/Brazilian bank | Compare this model with other models |
A29 | LSTM | MSE MAE, RMSE | Results showed that the LSTM model achieves perfect performance. AUC: 0.995. MSE: 0.0035. MAE:0.0065 | From the Kaggle website. | Further study of other types of RNN technique. |
A30 | SMOTE, LOF, isolation forest, SVM, LR, DT, RF | Accuracy Precision MCC | LR, DT and RF are the best algorithms. The better parameter to deal with unbalanced data is MCC. Classifiers performing better when using SOMTE. RF: accuracy (0.9998), precision (0.9996), MCC (0.9996). DT: accuracy (0.9708), precision, (0.9814), MCC (0.9420). LR: accuracy (0.9718), precision, (0.9831), MCC (0.9438). | Real dataset/Europeans cardholders/Kaggle | NA |
A31 | Autoencoders | NA | The results showed that Autoencoders model most promising for detecting fraud in credit card. | Real data/European cardholders | Using balanced dataset and unhidden features. |
A32 | NB using robust scaling | Accuracy, Precision, Recall Sensitivity AUC score F1-score | The result shows NB which used Robust Scaleris showed improvements in predicting and detecting fraud in credit card. Accuracy: 97.78%. Precision: 99.79%. Recall: 97.78. F1-score 98.71. AUC: 95.73. | Real dataset/Europeans cardholders/Kaggle | NA |
A33 | NB, RF, DT, MLP | Precision Recall, F-measure Specificity | The result showed that the amount-based profiling both MLP and RF obtained high improvement. This model boost fraud detection. | Dataset from 35 banks in Turkey | The high number of false positive needs further study. |
A34 | CNN DAE MLP | Precision Recall AUC Confusion matrix ROC curves | Results showed that DNN is capable in fraud detection. MLP2OH128H918 obtained an alert reduction rate. Threshold/D (0:1) of 35.16% when capturing 91.79% fraud cases. The rate of misclassification is 8.21%. Threshold/D (0:2) of 41.47% when capturing 87.75% fraud cases. Misclassification rate is 12.25%. | Dataset from a Spanish organisation. | NA |
A35 | DCNN, RNN, SVM, LR, RF. | Accuracy | Proposed model obtained accuracy of 99% in detecting fraud in credit card in time duration of 45 seconds. | Real dataset/Europeans cardholders | Applying the fraud location and timing calculation. |
A36 | 3DCNN, Spatial-temporal attention-based graph network (STAGN) | AUC Precision recall | The suggested model showed a high performance in detecting fraud in credit card. The model is effective and accurate. | Real-world data (Commercial bank) | Builds a real-time detection system. |
A37 | Bi-LSTM-autoencoder and isolation forest | Accuracy Confusion matrix | The suggested hybrid model contains Bi-LSTM Autoencoder and the isolation forest with unbalanced data. This model obtained the highest detection rate with 87% | Real dataset/Europeans cardholders | NA |
A38 | KNN, DT, RF LR, NB | Confusion matrix recall/sensitivity precision time | Hybrid classifier/combination of supervised classifiers which worked better than any other single classifier. KNN + DT: Sensitivity: 85.63%. Precision: 86.90%. KNN + LR: Sensitivity: 57%. Precision: 85.55%. KNN + RF: Sensitivity: 82%. Precision: 95.89%. KNN + NB: Sensitivity: 58%. Precision: 80.57% | Real dataset/Europeans cardholders/Kaggle | Use unsupervised combined classifier for batter result and use more classifier. |
A39 | LR, RF, DT, KNN | Accuracy, specificity, precision, sensitivity | The accuracy of LR is 94.9%, DT accuracy is 91.9%, and RF accuracy is 92.9%. KNN has a 93.9% success rate. Despite LR was more accurate, majority of this algorithm under fit. Thus, KNN is the best technique. | Real dataset/Europeans cardholders/Kaggle | NA |
A40 | ANN Harmony search algorithm (HSA) | Accuracy, recall SM calculation confusion matrix | The suggested model NNHS provides a solution using HAS for ANN. The best accuracy achieved is 86. Recall is 87. | German dataset available at the UCI website | NA |
A41 | HMM, SMOTE DBSCAN | Precision Recall F1-score | Proposed approach (SMOTE + DBSCAN + HMM) performed relatively better for all the various hidden states. | Simulated mobile based transactions | NA |
A42 | Deep reinforcement. Resampling SMOTE and ADASYN | Accuracy Precision Sensitivity Specificity | The proposed model of ML with two resampling techniques and DRL is reliable. SMOTE and ADASYN are used to resampling dataset. The proposed system obtained high accuracy with 99%. RF and XGBoost are the best techniques. | Real dataset/Europeans cardholders/Kaggle | Extend dataset. Applying new ML and DL algorithms |
A43 | HMM | Accuracy | The model is very efficient and showed the importance in learning spending behaviour. The accuracy is 80%. | NA | NA |
A44 | K-means. PCA T-SNE SOM | Accuracy | The model obtained an accuracy of 90%. The results were vary as the initialization of the weight of nodes SOM grid is done by randomly records or patterns. | Statlog Australian dataset. | Trying different iterations and store weights of SOM |
A45 | KNN, RF, NB | Accuracy | KNN showed the highest accuracy than the RF algorithm and NB. | Real-world dataset | More ML supervised algorithm can be added. |
A46 | DCNN space invariant ANN | Accuracy | The results showed that proposed robust SIANN (RSIANN) is outperformed other techniques. The accuracy is 85%. SVM accuracy is: 0.77. RF accuracy is: 0.72. NB accuracy is: 0.70. DCNN accuracy is 0.82. | NA | Using kernels technique also using pre trained CNN. |
A47 | SVM, NB, DT | Accuracy | Results showed that the new system will reduce the frauds which are happening while transactions. | NA | NA |
A48 | SVM, GNB, DT | Execution times | The proposed model using fusion of detection algorithms and AI. Support Vector Classifier take less time. SVC obtained solution with less time. 0.191343 ms. | Real data/European cardholders | Using other datasets also applying other algorithms |
A49 | RF | Accuracy | The result showed that RF obtained high performance. However, the speed will suffer. On the other hand, SVM suffer from unbalanced data. The SVM obtained good performance. | NA | NA |
A50 | NB, DT, RF, LR AdaBoost Gradient Boost XGBoost | Accuracy Recall Precision Confusion matrix | Results showed that XGBoost is the best boosting technique in predicting fraud. The accuracy is 100%. F1-score is 0.88. NB classifier: 95.6%. DT classifier: 90.0%. RF classifier: 97.7%. LR: 98.3%. AdaBoost: 99.9%. Gradient boost: 99.9%. XGBoost: 100%. | Real dataset/Europeans cardholders/Kaggle | NA |
A51 | RF, DT LR, LOF Isolation forest | F1-scores Precision Recall | Results showed that isolation forest obtained better efficiency. RF: 95.5%. DT: 94.3%. LR: 90%. Isolation forest: 99.77%. Local outlier factor: 99.69%. | Real dataset/Europeans cardholders | Using NN for training the system, to obtain better accuracy. |
A52 | Semi-supervised learning. AutoEncoders | Precision Recall F1-score | The results show that using semi-supervised technique is efficient to detect fraud. Accuracy is 0.98%. | Real dataset/Europeans cardholders | Investigate the intelligent dependent attributes. |
A53 | Autoencoders | Accuracy Precision F1-score Sensitivity | The proposed model obtained high performance. SSAE+LDA model showed significant improvement comparing with other research on same dataset. Accuracy is 90%, F1-score is 90%, precision is 91%, sensitivity is 90%. | Real dataset/UCI | Study effect of optimizers, stacking diverse autoencoders |
A54 | Light gradient boosting. RF | Accuracy AUC | This study only used to identify the fraudulent user. The results show that light gradient boosting obtained great performance with a total recall rate of 99%. | Real dataset/Europeans cardholders | Further study on how to judge fraud ring based on relation map. |
A55 | XGBoosting Neural network | Accuracy Precision, F1-score Recall, ROC, AUC | Results indicated that XGBoosting performs better when comparing with other ensemble models. XGB AUS is 0.778 | Consumer’s dataset/Taiwan. | NA |
A56 | MLP LR, SVM. Gradient descent algorithms. | Accuracy | Results showed that proposed model performs good comparing with LR and SVM. MLP Accuracy: 0.9990 LR Accuracy: 0.9723 SVM Accuracy: 0.9345 | NA | A dependent variable with numerous classifications can be used. |
A57 | GAN | Accuracy Precision | The model obtained an improved sensitivity. GAN model can training of small dataset. GAN Accuracy: 0.99962. Precision: 0.9583. | Real dataset/Europeans cardholders | Develop a strategy to reduce the decreasing in specificity to minimum |
A58 | Ensemble learning approach RNN, FFNN LSTM, GRU | Recall Precision F1-score | Results showed that proposed model based on LSTM with ensemble GRU on two datasets outperforms other models. The new model is efficient in term of realtime. | -Real dataset/Europeans cardholders. -Brazilian bank | Develop new Model to take advantage of deep encoder and decoder. |
A59 | CatBoost XGBoost Stochastic gradient boosting | Precision Recall Confusion matrix | Results showed that the CatBoost is the best comparing with XGBoost and SGB boosting. CatBoost accuracy is 0.921. Recall is 1.00. XGBoost accuracy is 0.914. Recall is 0.99. SGB accuracy is 0.907. Recall is 0.97. | NA | New models using supervised and unsupervised. |
A60 | LR, ANN SVM, RF Boosted Tree | Kolmogorov-Smirnov Formula. FDR | The new model using boosted tree shows best performance in fraud detection. FDR = 49.83% | Real dataset/government agency/USA | Some data and fields such as time, day point of sale should be added. |
A61 | NB, RF LR, SVM | AUC Precision Recall | NB technique shows high performance comparing with other techniques. Accuracy is 80.4%. Area under curve is 96.3% | Real dataset/Europeans cardholders | Develop another model for sampling imbalanced data. |
A62 | Isolation forest | Precision-recall curve (AUCPR) AUC | The proposed model demonstrate the efficiency in fraud detection, observed to be 98.72%, which indicates a significantly better approach than other techniques. | Real dataset/Europeans cardholders/Kaggle | Financial institutions must make available data set. Thus, outcome will be more efficient. |
A63 | UQ techniques: MCD EMCD | Confusion matrix UAcc, USen USpe, UPre | The suggested model using UQ provide high performance in predicting fraud. Ensemble technique is efficient in fraud prediction. MCD: UAcc (0.82) Ensemble: UAcc (0.85). EMCD: UAcc (0.84) | Publicly available dataset/Vesta corporation | The quality of final uncertainty estimates should be improved. |
A64 | RF, LR, DT, GNB combination with ensemble. | Matthews correlation coefficient (MCC) | The accuracy of all the five models is 100% & even the MCC score is +1 for the models been evaluated. | Real dataset/Europeans cardholders | NA |
A65 | DT augmented with regression analysis. | Accuracy Confusion matrix | The results showed that new model successfully verified the injected intrusions. Accuracy is 81.6% with 18.4% misclassification error. | Dataset from the UCI repository | NA |
A66 | SOM ANN | Accuracy | Using hybrid model of SOM and ANN achieved high performance compared to use ANN or SOM alone. | Dataset from the UCI repository | Creating a NN with some optimization technique. |
A67 | LR, RF, and CatBoost | Accuracy Precision Recall | The result showed that model of RF with CatBoost provides efficient accuracy. RF technique has the most elevated incentive than the LR and CatBoost algorithm.. RF: Accuracy (99.95). CatBoost: Accuracy (99.93). LR: Accuracy (99.88). | Real dataset/Europeans cardholders/Kaggle | NA |
A68 | Deep forest XGBoost AE, gcForest | Accuracy Precision, Recall Confusion matrix | The proposed model showed high performance in detecting card fraud. | Dataset from China’s bank. | NA |
A69 | GAN, variational automatic coding (VAE) | Accuracy F-measure Precision | The model showed that VAE-based oversampling performs better than the normal DNN and synthetic minority over sampling technique as it can solve the imbalanced problem. | Real dataset/Europeans cardholders | Improving the model recall rate |
A70 | C4.5 DT, NB Bagging ensemble | Accuracy Precision, Recall | The model shows that bagging with C4.5 DT is the best algorithm with rate of 1,000 for class 0.0825 for class 1. | Real dataset/Europeans cardholders | NA |
A71 | Fuzzy rough nearest neighbor (FRNN) SMO, LR, MLP, NB, IBK, RF | Positive predictive value (PPV). F-measure Specificity PPV, F-measure | The results showed that the suggested model provided significant results. The rate of detection is 84.90, AUC is 0.8555/Australian dataset. While 76.30% detection rate with 0.679 AUC/German dataset. | Australian dataset/German dataset | Other ensemble techniques should be considered. |
A72 | NB, DT, (LMT, J48,) Rules classifier Lazy classifier Meta classifier | Accuracy Recall Precision F1-score | The result showed that applying LMT algorithm to classification fraud is better than other techniques. LMT model obtained 82.08% accuracy. | Client’s data in Taiwan. Data available on: . | Further study to find out new algorithms with higher voting. |
A73 | Feature maps and GANs SVM CNN | AUC score ROC Confusion matrix | Results showed that the suggested model is applicable to test datasets and less time is required for learning. SVM obtained better detection. However, learning time exceeds other models when dataset increase. CNN-based model needs long time. SOMTE performance is effective. | Machine learning group ULB. Kaggle. | Change on oversampling techniques in the suggested model. |
A74 | CNN,SVM, RF isolation forest Autoencoder | Accuracy Precision | ML models have been implemented for classification purpose. Achieved competitive accuracy in CNN model. CNN: Accuracy (99.51). | Real dataset/Europeans cardholders | Predict fraud in real-time. Applying service on the cloud platform. |
A75 | LR, NB, KNN | Accuracy, Recall Specificity Sensitivity F-measure Precision | Results showed that LR showed optimal performance. It is getting high accuracy of 95%. NB accuracy is 91%. KNN accuracy is 75%. LR showed better sensitivity, precision, specificity, and F-measure. | Real dataset/Europeans cardholders/Kaggle | NA |
A76 | Isolate forest and local outlier factor (LOF) algorithms | Accuracy Precision Recall F-measure | The result showed that local outlier factor achieved high accuracy with 97%. Isolation forest accuracy is 76% | Real dataset/Europeans cardholders/Kaggle | NA |
A77 | RF, DT | Accuracy Sensitivity Specificity Precision | The result showed that this model is accurate on large dataset with 98.6% accuracy. RF provides high performance, however, it needs many training data. | Dataset from product reviews on credit card transaction. | Develop AI/ML/DL techniques |
A78 | Multiple classifiers system (MCS). NB, C4.5, KNN, ANN, SVM. | TPR TNR Accuracy | Results showed that the suggested model can tackle the unbalanced class distribution and overlapping class samples. The proposed model obtained high TPR, which is 0.840 and 0.930 accuracy. TNR is 0.955. | Dataset1: ULB Dataset2: credit cardholders/Taiwan bank | Considering combining the DL algorithms for promising detection results. |
A79 | KNN, SVM, LR HYBRID NB-RF XGB | Accuracy, recall, precision, TPR, FPR, | Results showed that all proposed models are superior in performance. Staking classifier using LR as meta classifier is most promising then SVM, LR, KNN and HNB-RF. Stacking classifier accuracy is 0.95. RF accuracy is 0.94. | Real dataset/Europeans cardholders/Kaggle | Applying Voting classifier. |
A80 | Hybrid models using AdaBoost and majority voting, NB, SVM | MCC | Results showed that the majority voting obtained high accuracy. The best MCC score is 0.823. | A publicly available data set/Turkish bank. | Applying online learning models so we enable efficient fraud detection. |
A81 | DT, LR, Shallow NN. Challenger model: DL model with ensemble. | AUROC K–S statistics alert rate, recall precision | Results showed that after testing off-line and post-line, operate the FDS with DL model. This shown +3.8% improvement of recall. The hybrid ensemble model perform well in detecting fraud. | Dataset from company/South Korea | NA |
A82 | LR, NB, AdaBoost, and voting classifier | Accuracy, recall, precision, sensitivity F1-score | Results showed a good accuracy for NB: 91.41%. LR: 94.51%. AdaBoost: 95.67%. Voting: 94.69%. | Real dataset/Europeans cardholders/Kaggle | A hybrid classification method will be designed. |
A83 | Ensembles of classifiers based on DT, XGBoost and LightGBM. | Accuracy, precision, recall AUC Confusion matrix | The result showed that the ensemble of models allowed to detect maximum 85.7% of fraud. Accuracy is 79‒85%. | Real dataset/Europeans cardholders/Kaggle | NA |
A84 | AdaBoost voting KNN, greater part casting ballot techniques. | MCC | The results showed that perfect MCC score achieved when using AdaBoost and greater part casting a ballot. Commotion from 10% to 30% included with data. The model yielded best MCC of 0.942. | Informational index from a Turkish bank | NA |
A85 | RF, KNN, NB, SVM | Accuracy | The result shows that RF has the highest accuracy of detection of fraud. RF accuracy is: 0.9996. | NA | Seeking information from advanced technologies. |
A86 | ANN, BBN | Confusion matrix | Result showed a Bayesian Network is more accurate than the NB Classifier. This is disturbed with using the fact of conditional dependence between the attributes in Bayesian Network, but it requires more difficult to calculation and as training process. | Real dataset/Europeans cardholders/Kaggle | NA |
A87 | KNN, NB, LR | Accuracy, sensitivity, specificity, | The result showed that KNN performed high performance of matrices except accuracy. | Real data/European cardholders | NA |
A88 | LR, RF, SVM | Accuracy, precision, F1-score, recall | Compression between LR, RF and SVM is performed and the accuracy of LR is 77.97%, RF is 81.79% and SVM is 65.16. So, RF is better than the SVM and LR. | Real dataset/UCI | NA |
A89 | LR, RF, XGBoost, ANN, isolation forest, PCA with SVM. | Accuracy, sensitivity, specificity, MCC precision, BCR | Results show that RF and XGBoost provided better result than other models. The accuracy of XGBoost is 0.9951. RF accuracy is 0.9955. | Mobile money transactions published on Kaggle. | Combined ANN with genetic algorithm to enhance accuracy. |
A90 | SVM, GA Cuckoo search Particle swarm | Accuracy Precision Recall | The results showed that Linear kernel function is the best. Accuracy is 91.56%. Radial basis used to enhance kernel accuracy. The accuracy improved from 42.86 to 98.05%. Overall, PSO-SVM better than CS-SVM and GA-SVM. | Data from law enforcement department in China | Look for new algorithms to optimize SVM |
A91 | AE-PRE Bootstrap aggregating Bagging | Accuracy TPR, TNR FPR ROC curve AUC, MCC | The result shows that AE-PRF is efficient when dataset is unbalanced. AE-PRF obtained high performance in accuracy. | Real dataset/Europeans cardholders/Kaggle | Improve AE-PRF model with adding fine-tuning the hyperparameters of AE and RF models. |
A92 | Multi-perspective HMMs | PR-AUC | The results showed that HMM model is powerful in detecting fraud. | Real dataset/Belgian | Combine LSTM with HMM-base features |
A93 | C5.0, SVM, ANN NB, BBN, LR, KNN, artificial immune systems (AIS). | Accuracy Recall Precision | The results showed that C5.0, SVM, and ANN are performing well with imbalanced classification problem. Even these techniques improve the classifier’s performance in fraud, high number of fraud cases continue undetected. | Two dataset available at | Develop new model with big data driven ecosystem. |
A94 | Hybrid model: DT, SVM, ANN genetic algorithm (GA). | F-score Accuracy Recall | The results showed that the suggested hybrid model obtained high accuracy with 93.5% comparing with ANN, SVM, and DT. The hybrid model applied GA outperform other techniques. | Realworld dataset from financial institution | Real-life test for the suggested model |
A95 | DT, RF, KNN, LR K-means, DBSCAN, MLP, NB, XGBoost Gradient boost | Accuracy Precision Recall F1-score | The result showed that RF yielded perfect performance result with accuracy 99.995. RF is suitable for large datasets. | Real dataset/Europeans cardholders/Kaggle | NA |
A96 | Local outlier factor. Isolation forest | Precision Accuracy | The results showed that the model reached over than 99.6% accuracy. Precision at 28%. When fed more data in the model, the precision raised to 33%. | Dataset from German bank in 2006. | Adding more algorithms. Using more dataset. |
A97 | KNN, PCA, SMOTE | Recall Precision F1-score | The results showed that the suggested model performed well. For KNN: Precision 98.32. F-score 97.44%. For Time subset when using the misclassified instance, precision is 100% and F-score is 98.24%. | Real dataset/Europeans cardholders/Kaggle | Know how PCA can affect the performance of a dataset. |
A98 | KNN, DT, LR RF, XGBoost | Accuracy F1-score Precision Recall, AUC-ROC | The results show that the XGBoost and DT outruns all other algorithms in detecting fraud. | Real dataset/Europeans cardholders/Kaggle | Study on other ML algorithms and various forms of stacked classifiers. |
A99 | Outlier detection DT, RF and NN | Precision Recall ROC Confusion matrix | The results showed that RF is the most precise and accurate technique. However, it takes long time to train. NN is the next best algorithm. DT is the least accurate. In term of time efficiency and computational resource utilization the NN is the best technique. | Real dataset/Europeans cardholders/Kaggle | NA |
A100 | NB, C4.5 DT, and bagging ensemble learner. | Precision Recall PRC | The result showed that the performance is between 99.9% and 100%. The best classifier is C4.5 DT with 94.1% precision and 78.9% recall. The acceptable performance is bagging ensemble with 91.6% precision and 80.7% recall. As for the worst performance, it is the NB classifier with precision of 65.6% and a recall of 81%. | Real dataset/Europeans cardholders/Kaggle | Other classifiers will be used and applied to a set of local data that will be collected from banks in Iraq. |
A101 | Autoencoders MLP, KNN and LR | Accuracy Precision Recall F1-score | Results showed that the suggested model maintains a good performance. It outperforms the systems based on either different classifiers or variants of autoencoder. It establishes the efficiency of proposed two stage model. Proposed method accuracy is 0.9994. Precision is 0.8534. F1-score is 0.8265. | Dataset from ULB machine learning group on Kaggle. | Proposed two stage model can be tuned to handle stream data. The model can be trained on a batch of transactions. |
A102 | LOF, AdaBoost, RF, isolation forest, DT, KNN, HMM, GA, ANN, NB, LR | Accuracy Confusion matrix | Results showed that the local outlier factor accuracy is greater than other algorithms. Local outlier factor accuracy is: 0.898. | Real data/European cardholders | NA |
A103 | RF | Accuracy | The results showed that RF performs better with large dataset. The accuracy is 99.9%. The SVM algorithm can be used instead of RF. However, SVM still suffers from the imbalanced dataset. | NA | Privacy preserving techniques can be applied in distributed environment. |
A104 | RF | Accuracy F1-score, Precision, Recall | The result showed that the RF performed better comparing with DT and NB. The suggested model showed better accuracy on huge dataset. | Real dataset/100,000 cardholders | Applying semi-supervised technique |
A105 | Oversampling with SMOTE SVM, LR, DT, RF | Accuracy Precision Recall, F1-score | Results showed that when using SMOTE technique, the model works better in predicting fraudulent. RF and DT provided best performance. | Real dataset/Europeans cardholders | Building a real-time solution to detect fraud. |
A106 | RF AdaBoost oversampling ADASYN | Accuracy Recall Precision F1-score | This research examines various existing credit card fraud systems using ML approaches. Despite the fact that RF produces outstanding results on tiny sets of data, there are still certain problems, such as data imbalance. RF accuracy is: 0.999. | Real dataset/Europeans cardholders/Kaggle | Using large amount of data. More pre-processing procedures. |
A107 | Autoencoder neural network DAE | Recall Accuracy | The results showed the DAE improves classification accuracy of minority class of imbalanced datasets. Proposed model increases accuracy of minority class. When threshold equal to 0.6, model achieves best performance with 97.93%. | Real dataset/Europeans cardholders/Kaggle | Dimensionality reduction of high-dimensional data needs further research. |
A108 | ANN with LR | Accuracy, Precision and Recall | The results show that the model is very good. Accuracy achieved of 0.9948, the recall is 0.8639 and precision of 0.2134. | Real data/European cardholders | NA |
A109 | GAORF | Accuracy Confusion matrix | The results showed that using real and genetic algorithm optimised RF models. The model has good improvement and bringing down misclassifications. | Commercial bank in Nigeria | NA |
A110 | 2DCNN, 1DCNN LSTM, NLP SMOTE | Accuracy F-score Precision Recall | The result showed that using CNN and LSTM yielded better performance. LSTM (50 blocks) was the highest with F1-score of 84.85%. Sampling techniques applied to solve imbalanced dataset and improve model performance. | Real dataset/Europeans cardholders/Kaggle | Hyperparameters to build DL techniques to improve performance. |
A111 | ANN, RF, GBM RUS, SMOTE DBSMOTE SMOTEENN | F1-score Recall Precision Accuracy | The result showed that using sampling techniques enhanced the detecting of fraud in credit card. Recall obtained with SMOTE by DRF classifier is 0.81 which is the best. Precision is 0.86. Staked ensemble shown promise in detecting fraud. | Real dataset/Europeans cardholders/Kaggle | Using other sampling techniques. Applying unsupervised and semi- supervised techniques. |
A112 | Local outlier factor, LR, RF, DT isolation forest | Accuracy MCC | The result showed that the LR, SVM obtained higher accuracy. SVM accuracy is 0.9987. LR accuracy is 0.9990. One-class SVM applied in this study. | Real dataset/Europeans cardholders | NA |
A113 | ANN.LR, DT, RF and XGBoost | Accuracy Precision Recall F1-score | Results showed that ANN and XGBoost performed a high performance. ANN achieved a 99% accuracy. | -Real dataset/Europeans cardholders. -Synthetic dataset | Use more real world datasets. |
A114 | NB, SVM AdaBoost | MCC Accuracy | The results showed that boosting technique achieved a good accuracy. The best MCC score is 0.823. | Real world dataset. | Extend the model to online learning model. |
A115 | KNN, LR, SVM, RF, DT, XGB, OCSVM, AE, RBM, GAN | AUROC FPR TPR | The results showed that applying supervised approach such as, RF and XGB achieved better performance. XGB obtained 0.989 AUROC. RF obtained 0.988 AUROC. Unsupervised techniques RBM achieved the best performance with 0.961 AUROC. | Real dataset/Europeans cardholders/Kaggle | Focuses on new GAN model |
A116 | RF | Accuracy Sensitivity Specificity Precision | The result showed that building multiple DT achieved good performance with 98.6% accuracy. | Real dataset/Europeans cardholders/Kaggle | NA |
A117 | IBk, IB1, KStar, RandomCommittee, and RandomTree AdaBoost | Accuracy Precision Recall | The results showed that the best accuracy achieved by Bagging, Rotation Forest, Random SubSpace, Random Committee, LMT, and REPTree. The IBK, IB1, RandomCommittee, KStar, and RandomTree obtained good accuracy. And can detect fraud 348 (35.27%), 354 (40.97%), 396 (45.83%), 397 (45.94%), and 399 (46.18%) respectively. | UCSD—FICO dataset. | NA |
A118 | Spectral-clustering hybrid of GA trained modular NN. | Sensitivity Specificity Accuracy | Results showed that hybrid model is efficient in detecting fraud. The model obtained sensitivity of 90%, specificity of 19% and prediction accuracy of 74% with improvement rate of 12% for data inclusion. | Dataset from banks/Africa and Nigeria. | NA |
A119 | ANN, SA-ANN HTM-CLA DRNN, LSTM | Accuracy | Results showed that the HTM-CLA offered a realistic features. HTM-CLA with SA-ANN achieved good performance. The maximum accuracy obtained from SA-ANN. | Real dataset/Australia Real dataset/German | Reduce computational burden in HTM-CLA technique |
A120 | Isolation forest KNN, DT, LR, RF | Sensitivity time and precision | The result showed that KNN sensitivity is better than DT. However, DT needs less time to detect fraud. DT is the best model. | Real data/European cardholders | NA |
A121 | LR, DT, RF, NB, ANN | Accuracy Recall Precision | Results showed that the accuracy is 94.84% when using LR. 91.62% when using NB and 92.88% when using DT. ANN obtained better accuracy of 98.69%. ANN is the best. | Real dataset/Europeans cardholders | NA |
A122 | KNN, DT, SVM, LR, RF XGBoost | Accuracy F1-score Confusion matrix | The result showed that KNN model is the best comparing with other techniques. Accuracy is 99.95%. F1-score is 85.71%. | Real dataset/Europeans cardholders | Using other resampling and applying DL techniques. |
A123 | Hybrid architecture involving the optimization of the particles swarm (PSO) SVM | Accuracy F1-score Confusion matrix | The PSO algorithm is used to select characteristics and the SVM is used for the iterative development of the feature selection. Results shown that a minimum of functionalities is extracted by the suggested PSOSVM. The PSO-SVM algorithm is an optimal preparatory instrument for enhancing feature selection optimisation. Accuracy for German dataset: with SVM: 78.69. PSOSVM: 89.42. Accuracy for Australian Dataset: with SVM: 78.84.PSOSVM: 89.27. | German credit card datasets. Australian credit cards | NA |
A124 | Stacking AdaBoost majority voting LR, DT, RF | Accuracy | The result showed that the suggested model provided better fraud detection. The boosted stacking performs better than others. Boosted Staking accuracy is 94.5% | Real dataset/Europeans cardholders/Kaggle | NA |
A125 | Neo4j, PageRank, RF, DT KNN, SVM, MLP, LOF, isolation forest | Accuracy MCC, F1-score Recall, Precision ROC, AUC,AUPR | The result showed that significant improvement in performance metrics of DT. LOF yielded a better result with 99.54% accuracy and recall 83.39%. When using PageRank graph feature. RF accuracy is 99.47%. | Synthetic dataset/BankSim | Other graph algorithms to extract feature and DL should be studied further. |
A126 | Autoencoder, RBM | Recall, Precision AUC | The result showed the AE and RBM can make AUC more accurate. AE based camera and H2O applied. | Real dataset/Europeans cardholders | NA |
A127 | AdaBoost Majority vote MLP, SVM LOR, HS | MCC metrics | The result showed that the hybrid model of majority voting provided good accuracy. The model achieved great location rate 98% with 0.1%. Perfect MCC score when using AdaBoost and Majority voting. | Real dataset/Europeans cardholders/Kaggle | Examined other internet study models |
A128 | AE, one-class SVM and robust Mahalanobis outlier detection | Precision Error rate MSE | Results showed that the advantage of robust Mahalanobis is that does not need label for training. The performance of the three models was vary. To get vision about performance of models the available labels used for model performance evaluations. | Real dataset from international corporation | Global and local outlier, cardholder behaviour need to be considered. |
A129 | AdaBoost, NB, RT Majority voting DT, GBM, NN, SVM, Spark ML | MCC | The results showed that the hybrid model of NB, SVM, and DL techniques obtained an ideal MCC score 0.823. | Public real dataset/bank | Expand to internet learning |
A130 | SVM-RFE Hyper-parameters Optimization SMOTE | Accuracy Precision Recall, Specificity F-score | Results showed that the proposed model is high effective and obtained the best accuracy with 99%. | Real dataset/Europeans cardholders/Kaggle | Using more complex datasets |
A131 | RNN SMOTE Tomek LSTM, BLSTM GRU, BGRU | Accuracy Recall, Precision AUC | Results showed that BGRU achieved the best accuracy 97.16%, then BLSTM with 96.04%. | Real dataset/Europeans cardholders/Kaggle | Focuses on the behavior of customer. |
A132 | WOA SMOTE BPNN | Accuracy | The result showed that the WOA and SMOTE obtained more efficient than BPNN. | Real dataset/Europeans cardholders | NA |
A133 | NB, SVM, RF | Accuracy | The results showed that the RF is the best technique with accuracy of 100%. | Real data/European cardholders | NA |
A134 | RF, SVM, LOF isolation forest | Accuracy Precision Recall | The result showed that the RF obtained 99.92 accuracy. RF performed better comparing with other techniques. | Real dataset/Europeans cardholders | Improve dataset and add other algorithms to the suggested model |
A135 | K-means C5.0 DT Hadoop and Spark | Accuracy ROC AUC | The results showed that the spark-based IHA hybrid model obtained 94% accuracy. It is suitable for detect fraud. | Public domain | Applying this model to other fields |
A136 | SVM Undersampling techniques | Accuracy Precision Recall | Results showed that the new model improves the performance. Accuracy is 99.9%. SVM obtained best precision with 89.5%. | Real dataset/Europeans cardholders | NA |
A137 | Isolation forest | Accuracy | The results showed that the isolation forest obtained accuracy with 99.87. | Professional survey organizations. | Using hybrid techniques and AI |
A138 | RF | Accuracy | The results showed that RF using feedback and delayed supervised sample is better than other techniques. RF accuracy is 0.962. | NA | Applying semi-supervised techniques |
A139 | SVM, KNN AdaBoost PSOS RIG | Accuracy Precision Recall F-measure | The results point out that PSOS technique is the best feature optimisation technique. This technique enhanced the accuracy from 82.90% to 85.51%. PSOS technique gives more performance. | Australian financial dataset. | Extend the model by using hybrid techniques |
A140 | AdaBoost majority voting, NB, SVM, DL | MCC | The results showed that Majority voting obtained a high accuracy and best MCC score with 0.823. | Public realworld data set | Extend to online learning model |
A141 | RF, NN | Accuracy Precision Recall, F-measure | The result showed that RF obtained accuracy with 90%. RF is suitable technique. | Real-life B2C dataset | Thr RF itself needs improvement. |
A142 | NB, RF, DT, GBT, DS, ANN, RT, MLP, LIR, LOR, SVM | Accuracy ACC MCC | The results showed that the best AUC obtained is 0.937 from GBT using aggregated features. Aggregated features improve the models performance. | Public data sets. Benchmark databases. | Further evaluation of this models using different datasets. |
A143 | HOBA DBN, RNN, CNN BPNN, SVM, RF | Accuracy Precision, Recall F1-measure | The results showed that the DBN with HOBA variable obtained better performance. Using DL techniques and HOBA feature engineering improve the performance. | Real-world dataset/bank in China | Build real-time model. Build a combination model of ML and DL |
A144 | AE GAN | Precision Recall F1-measure | The result shows that the DAEGAN model achieved best performance. AUC is 0.958. Recall is 0.815. AUPRC is 0.805. DAEGAN improves accuracy. | Real dataset/Europeans cardholders | Improve the model |
A145 | Isolation forest | AUCPR F1-score Precision, Recall ROC-AUC | The result showed that the model achieved good performance. AUCPR is better than ROC-AUC in describing performance. Precision is 0.807. Recall 0.763. F1-score is 0.784. ROC-AUC is 0.973. AUCPR is 0.759. | Real-life dataset from ULB. Kaggle. | NA |
A146 | SVM, LOF isolation forest SVM | Accuracy Precision F1-score, Recall | The results point out that isolation forest with LOF model very fast and accurate. The accuracy is 99.74%, SVM obtained 45.84%. LOF achieved 99.66%. | NA | NA |
A147 | SVM, K-means AdaBoost | Recall, Accuracy | The result showed that SVM and AdaBoost obtained high performance. | Dataset from a bank. | NA |
A148 | Deep auto-encoder | Accuracy Precision, Recall AUC-ROC Curve | The results showed that the algorithm is perfect and gave high performance 98.8% acceptance rate. The proposed algorithm can be used for any Binary classification task. | Real dataset/Europeans cardholders | NA |
A149 | NN | Accuracy | The result showed that the suggested model can be integrated with mobile apps to detect fraud. Model obtained excellent accuracy with 99.75%. | Real dataset/Europeans cardholders | NA |
A150 | RF, DT, SVM, GNB LR | Accuracy | The result showed that the DT provided better performance. However, speed still suffer. | NA | Using other ML and DL techniques |
A151 | Isolation forest LOF | Recall, Precision F1-score | The isolation forest obtained accuracy with 99.72%. With number of errors 71. LOF accuracy is 99.62% and number of errors 107. Isolation forest is better in detecting fraud. | Real dataset/Europeans cardholders | Using NN technique |
A152 | DT, RF, HMM, NN | Accuracy false alarm rate, MCC | The results point out that the RF obtained high performance with 0.999% accuracy in fraud detection. | Real dataset/UCI | NA |
A153 | TVIWDA SVM WFSVM | Accuracy Precision, Recall F1-score | The result showed that using TVIWDA with WFSVM improved the accuracy of detection. The suggested system obtained 97.82% accuracy. Precision is 92.62%. | German credit card dataset. | Solving the imbalanced data problem |
A154 | Oversampling pre-processing technique SAS. RF, KNN, DT, LR | Accuracy | The study proposed 4 models to detect credit card fraud. The result showed that the RF and KNN are overfitting. Thus, only the DT and LR have been compared. The best performing model is a LR. Result shows that LR with stepwise splitting rules has outperformed the DT with only 0.6% error rate. | Real dataset/Europeans cardholders/Kaggle | Use different sampling technique such as undersampling, SMOTE or roughly balancing to compare the result. |
A155 | RF, DNN | Accuracy | The results showed that the RF perform perfect with large number of data. RF accuracy is 0.999. | NA | NA |
A156 | KNN, LR | Accuracy Precision Recall, F-measure | The result shows that the KNN technique is achieved best result. Precision is 0.95. Recall is 0.72. F1-score is 0.82. | Real dataset/Europeans cardholders | NA |
A157 | SMOTE MLP, KNN, SVM OSE, NN, GAN | Accuracy F1-score | The results point out that the model using stacking classifier which combines GAN-improved MLP with SVM and KNN. OSE is preferred because of its ability to harness the abilities of MLP which works better in finding hidden patterns. The accuracy of OSE is 99.8% | Real dataset/Europeans cardholders/Kaggle | Apply weighted voting and boosting algorithms |
A158 | Aggrandized RF | Accuracy Precision Recall, F-measure Sensitivity Specificity | The result showed that the aggrandized random forest is obtained high accuracy with 0.9972% for balanced data. And 0.9995% for imbalanced data. RF is the best technique in detecting fraud. | NA | NA |
A159 | RF, ANN, SVM, LR, tree classifier gradient boosting | Accuracy Precision Recall F1-score | Results showed that the RF algorithm demonstrate an accuracy percentage with 95.988%. SVM accuracy is 93.228%. LR accuracy is 92.89%. NB accuracy is 91.2%. DT accuracy is 90.9%. GBM accuracy is 93.99%. | ULB dataset from Kaggle | Apply other ML techniques |
A160 | SVM, NB, KNN focal loss XGBoost W-CEL, LR | Accuracy Precision Recall MCC | The result showed that the suggested model achieved accuracy with 100%. Precision is 0.97. Recall is 0.56. MC is 0.72 using extreme imbalanced dataset. When using mild balanced dataset, the accuracy is 99%. 0.88 precision. 0.87 recall. 0.89 MCC. The suggested model is not working well when using extreme dataset. XGBoost improves model performance. | ULB dataset from Kaggle | Solve the imbalanced dataset problem |
A161 | HMM | NA | The study provided a method to find out the spending behaviour of cardholder, then find out the observation symbols so that help in estimating the model performance. | NA | NA |
A162 | LOF, K-means isolation forest | Precision Recall F1-score | The result shows that proposed model provided an accuracy with 98%. K-means clustering, isolation forest and LOF. | Real dataset/Europeans cardholders | NA |
A163 | KNN, LR, RF XGBoost extreme gradient boost | Precision ROC-AUC | As the XGBoost is showing more accuracy than other models. Out of these algorithms, XGBoost model is preferable over the RF model and LR model. | Real data/European cardholders | RF model would be improved |
A164 | AdaSyn, ROS, RUS, Tomeklinks AIIKNN, Tomek SMOTE+ENN, AdaBoost, KNN, RF, SVM, eXtreme XGBoost, LR | Accuracy Precision Recall K-fold AUC-ROC Execution time | The result showed that oversampling followed by undersampling performs well for ensemble classification models. AIIKNN, SMTN, and RUS are performing well. SVM and KNN achieved perfect results. Best precision provided by oversampling followed by undersampling methods in conjunction with RF. NB classifier was the least. | Machine learning Group ULB. Kaggle. | NA |
A165 | RF, SVM, ANN. | Accuracy | The result showed that ANN produced high accuracy then RF then SVM. | NA | Using more techniques. |
A166 | KNN, isolation forest, local outlier factor | Accuracy Recall score | Results showed that all algorithms achieved 95.0% accuracy. Isolation forest had high accuracy and K-means produced the low accuracy. LR and vanilla LR gave great accuracy. | Real dataset/Europeans cardholders | Implement an autoencoder or SVM. |
A167 | LIGHTGBM AdaBoost, RF | Accuracy, precision and recall | The results showed that AdaBoost provided the highest result with 0.9613. In term of precision, Light BGM produces the highest result with 0.986. AdaBoost provided the highest recall with 0.889. | Real dataset/Europeans cardholders/Kaggle | Adding more parameters. |
A168 | OLightGBM RFLR SVM, DT, KNN NB | Accuracy Recall Precision F1-measure | The results highlight the importance of adopting an efficient parameter optimization strategy for enhancing the predictive performance. The proposed model outperformed other techniques with accuracy 98.40%. AUC is 92.88%. Precision is 97.34%. F1-score is 56.95%. | Real dataset/Europeans cardholders/Kaggle | NA |
A169 | RF, Apache Kafka | True positive rate (TPR), TNR, recall, precision accuracy | Using Apache Kafka to consume the transactions from the transaction record and publish them in real time. This project is using Cassandra as the storage layer. This proposed system offers the user maximum security and precision. | Data from the file system to the Cassandra database. | NA |
A170 | Autoencoder RBM Federate learning | Accuracy ROC, Recall Precision | The results showed that the average accuracy of Autoencoder is 94% and RBM is 88%. AUC achieved a result of 0.94. | Real dataset/Europeans cardholders | NA |
A171 | RF | Accuracy Recall, Precision F1-score | The result showed that RF obtains good performance on small dataset. Some problems with imbalanced dataset. RF accuracy is 0.9632. Precision is 0.894. Recall is 0.85. F1-score is 0.871. | Real dataset/Europeans cardholders | Improve RF itself |
A172 | RF, LR, DT, KNN NB, Undersampling and oversampling techniques. | Accuracy Sensitivity Specificity Precision Matthews’s co-relation | Results showed that LR is the best algorithm. The proposed classifier NN and LR outperform DT. LR accuracy is 0.9699. | Real dataset/Europeans cardholders/Kaggle | NA |
A173 | Local outlier factor, LOF, INFLO, and AVF | Accuracy Recall Precision | The results showed that using LOF, INFLO, and AFV resulted in the highest level of LOF. 96% accuracy, 98% recall, and 93% precision. | World website | Trying other algorithms. |
A174 | LR, DT, SVM, NB, RF, KNN | Accuracy Precision Recall | The result showed that using RF obtained best accuracy of 99.947%, precision is 76%, and recall is 92.68%. | Real dataset/Europeans cardholders | ANN can be used to construct new classification techniques. |
A175 | Deep learning based fraud detection model (DLFD) | Accuracy Precision Recall | DL model is constructed for the prediction process using Keras. Comparison with existing models indicate high performance in detecting fraud. Detection rate is 8.7%. DLFD accuracy/0.997. Precision/0.929. Recall/0.795. | BankSim dataset was used for analysis of performance. | Improving the TPR levels and also on handling the concept drift. |
A176 | ANN | Accuracy | The result showed that ANN is successful in fraud detection. Accuracy is 98%. However, ANN faced problems when training on huge datasets. | Dataset from company/South Africa | NA |
A177 | ANN, GA, LR, SMOTE | Accuracy Precision Recall, F1-score | The results showed that the ANN with genetic algorithm obtained accurate results. The accuracy is 99.83%. Precision is 50.70%. Recall is 97.27%. F1-score is 66.66%. | Real dataset/Europeans cardholders | NA |
A178 | SVM, fuzzy association rules (FAR). Gradient recurrent unit | NA | The results showed that the proposed framework provided significant contribution. The framework allow to detect abnormal transaction. | NA | Implementation and evaluation the framework. |
A179 | Hybrid ensemble-based. Boosting and bagging, RF, LR | MCC, Precision Recall Detection rate Accuracy | Results showed that the model is efficient in detecting fraud. MCC is 1.00. The false positive rate is 0.00235. False negative rate is 0.0003048. The detection rate is 0.9918. Accuracy is 0.9996. MCC is 0.9959. | Brazilian bank data and UCSD-FICO data | NA |
A180 | Particle swarm optimization (PSO). NN | Accuracy Precision Recall | Results showed that performance of PSO is very high with 99.9% accuracy. | Real dataset/European cardholders | Focus on solving imbalanced. |
A181 | LR, RF Under sampling and oversampling | Confusion matrix, precision, F1-score, Roc-AUC | RF precision is 0.93. F1-score is 0.85. The oversampling, under sampling of data for accuracy of classifiers is promising. Oversampling technique gave better fraud prediction results as compared to random under sampling. | Real dataset/European cardholders | NN and using combination of HMM or KNN to achieve better in fraud detection. |
Overall performance estimation of ML/DL model in credit fraud detection
This section addresses RQ3, which concerns the estimate of ML/DL model performance. Accuracy of estimation is the primary performance indicator for ML/DL models. This question focuses on the following features of estimating accuracy; performance metric, accuracy value, and dataset. As the construction of ML/DL models is dependent on the dataset, we examined the data sources of ML/DL models in the reviewed article. In addition, we found a number of datasets utilised in the experiments of associated article. This review articles employs two sets of datasets; real-word data set and synthetic dataset. The dataset utilised most frequently in the reviewed article is a real-word dataset. In addition, 154 research article employed real-world datasets, eight utilised synthetic datasets, and 19 did not specify the dataset source.
Evaluation metrics were used to calculate ML/DL model performance. Confusion matrix provides output matrix that characterises the model’s overall effectiveness. ML/DL model’s accuracy is compared using confusion matrix sensitivity and specificity, F-score, precision, receiver operating characteristic (ROC), and area under precision recall area (AUPR).
In this review, a number of different performance indicators have been used in addition to accuracy. As shown in Table C1 , we found 177 article that clearly presented the performance metrics of the proposed models. Four article did not mention the performance metrics. We discovered that 177 of reviewed article mentioned the performance indicators of their suggested models. However, four reviewed article did not mention the performance metrics. In this review, accuracy, recall, precision, and F-score were often employed as performance indicators. Accuracy is the proportion of test set records that were properly categorised transaction to fraudulent or non-fraudulent. The ration of true positives to all positives is referred to as precision. The proportion of fraudulent transactions that we correctly detected as fraudulent compared to the total number of fraudulent transactions would be the precision. Recall is percentage of all correctly classified predictions made by an algorithm. In addition, the value of F1 provides a single score that is proportionate to both recall and precision. Full two-dimensional area under the entire ROC curve is measured by AUC. One of the best indicators for analysing the effectiveness of credit card fraud detection is the ROC curve. The classification’s quality is measured by MCC. Because it covers true positive, true negatives, false positive, and false negatives, it is a balanced metric. MCC utilised in 13 reviewed article.
In addition, 30 of the 181 studies employed only a single performance metric, with the majority of these article using only accuracy (24) article, MCC (five) article, and execution time (one) article. Using single performance metric is insufficient for determining the quality of ML/DL model. However, article such as 43 and 74 utilised more than five performance indicators to represent the performance of their ML/DL model. In addition, a number of reviewed article give computational performance measurements as well as performance metrics. The length of time the model took to complete the assigned task is called execution time. To ascertain how long the model takes to detect fraud, the execution time is calculated. As a result, we guarantee that the model successfully achieves its goal. Execution time employed in Alghofaili, Albattah & Rassam (2020) , Devi, Thangavel & Anbhazhagan (2019) , Singh, Ranjan & Tiwari (2021) . The loss rate function compares actual and expected training output to speed up learning. Loss rate employed in article ( Alghofaili, Albattah & Rassam, 2020 ). Test of the effect of cost sensitive wrapping of Bayes minimal risk (BMR) applied in article ( Almhaithawi, Jafar & Aljnidi, 2020 ) as a cost-saving measure. Balanced accuracy (BCR) combines the matrices of sensitivity and specificity to produce a balanced outcome. BCR presented in article ( Layek, 2020 ). In ( Arun & Venkatachalapathy, 2020 ) Kappa assesse the predication performance of the classifier model. Few article ( Arya & Sastry, 2020 ; Bandyopadhyay et al., 2021 ; Bandyopadhyay & Dutta, 2020 ; Benchaji, Douzi & El Ouahidi, 2021 ; Rezapour, 2019 ) introduced mean square error (MSE) assessment metrics, mean absolute error (MAE), and root mean square error (RMSE). Table C1 shows the proposed ML/DL model along with performance and datasets.
Trend of research
To answer RQ4, we examine the trend of the reviewed article. In addition, we compare the models created over the three years to determine and evaluate which techniques recently garnered more attention. This also assist, to identify the gaps so that future research will be able to address them in their own work. First, we examined the distribution of the chosen article by the publication year. In year 2019 (47 articles), 2020 (70 articles), and 2021 (64 articles). Significant difference existed between the years 2019 and 2020, the number of published articles for credit card fraud detection increased (23 articles). However, there was no notable difference between 2020 and 2021 (six articles). Fig. 2 demonstrates this comparison.
In response to RQ1, we demonstrated that 110 distinct ML models, 34 distinct DL models, and 39 models that combine ML and DL have been utilised by researchers. RF, LR, and SVM are the most commonly employed ML approaches. ANN, AUE, and LSTM are the most utilised DL approaches. In addition, we observed increased interest in combining ML and DL models.
In our review, we count the various learning-based credit card cyber fraud detection techniques applied in the reviewed article to answer RQ2. From this review we found that the most common technique among the reviewed article is the use of supervised algorithm. Supervised algorithms applied in 74% of the reviewed article. A total of 12% of the reviewed article utilised unsupervised techniques. A total of 12% used supervised and unsupervised techniques. A total of 2% applied semi-supervised technique. A total of 1% used reinforcement technique. For the RQ3, we listed the performance metrics that each research article applied. We discovered that 24 out of 181 reviewed article utilised accuracy as their only key performance metric. We also found a number of datasets that utilised in the reviewed article. Majority of the reviewed article using real-world datasets. A total of 154 research article applied real-world data, eight article used synthetic data, and 19 did not mention the source.
In RQ4, we identified research gaps by investigating unexplored or infrequently studied algorithms. In addition, we found supervised learning as the most prevalent learning technique and SMOTE as the most prevalent oversampling technique. The majority of researchers focused on supervised techniques such as LR, RF, SVM, and NN.
Combination techniques that employ multiple algorithms are becoming increasingly prevalent in the detection of cyber fraud. Detecting cyber fraud in credit card increasingly involves the use of DL. DL techniques utilised 34 times in the reviewed article, whereas 39 of the reviewed article applied a combination of DL and ML techniques for credit card cyber fraud detection. DL is advantageous for fraud detection since it solves the difficulty of recognising unexpected and sophisticated fraud patterns. Moreover, as the number of fraud cases to be recognised is relatively limited, DL may be effective. DL have garnered the most attention and had the most success in combating cyberthreats recently. Due to its ability to minimise overfitting and discover underlying fraud tendencies. Moreover, the capacity to handle massive datasets.
For supervised learning algorithms to predict future credit card transaction, each observation must have a label. Given that there is no classification for these observations, this could be a problem when trying to identify fraudulent transactions. Additionally, since fraudsters constantly alter their behaviour, it is challenging to develop a supervised learning model for a given transaction. The normal class is often the only one that unsupervised algorithms need labels for, and they can predict future observations based on deviations from the normal data. Future research should give more attention to unsupervised and semi supervised techniques, which can yield new insights. In addition, paying more attention to DL techniques such as CNN, RNN, and LSTM, we recommend that further research may be conducted on ML techniques, especially semi-supervised and unsupervised techniques in order to improve ML model performance. In addition, performing additional research on DL techniques is needed. As a result of the unavailability of a balanced dataset and the shortage of datasets, financial institutions are encouraged to make the essential dataset available, so that research outputs will be more effective and qualitative.
To detect cyber fraud in credit card, supervised, unsupervised, and semi-supervised ML/DL techniques applied in the reviewed article. Figure 4 displays that 74% of the reviewed article utilised supervised techniques. As a result, it is the most common technique used in the reviewed article. In addition, according to the reviewed article, classification and regression techniques been always of interest. On the other hand, 12% of selected articles applied unsupervised techniques, 12% of selected articles applied both supervised and unsupervised techniques, while 2% articles applied semi supervised techniques, and 1% articles applied reinforcement learning. A growing trend in this field is the use of ensemble techniques that capitalise on the benefits of several classification methods. The use of ensemble methods increased in 2020 and 2021 comparing with 2019. The other interesting finding is that DL approaches have attracted considerable interest during 2019 to 2021. The number of research articles that used DL techniques as single technique or combined with other ML techniques in 2019 is 15 articles, in 2020, 30 articles, and in 2021, 28 articles. It appears that the popularity of DL algorithms has increased.
The countries that published research on utilising ML/DL techniques to detect credit card cyber fraud is growing over time. In 2021, Ghana, Romania, Taiwan, and Vietnam are among the new countries that made an effort in detecting cyber fraud. India is the pioneer when it comes to the publication of ML/DL studies. Figure 5 depicts the number of article published by country and year (2019, 2020, and 2021).

Gap analysis and the future direction
The most effective way for determining the approaches that are most appropriate for this research problem is to categorise the ML/DL algorithms used in detecting cyber fraud in credit card. Additionally, it is beneficial to determine why particular tactics were chosen. Supervised algorithms have always been of interest, as 74% of the reviewed articles have been used supervised algorithms, with the most commonly used being RF then LR then SVM. Unsupervised learning algorithms also applied in 12% articles with the most commonly used being Isolation forest. However, it is interesting that only 12% of the 181 reviewed studies utilised unsupervised learning techniques. Semi-supervised approach employed in 2% of the reviewed articles. It appears that semi-supervised and unsupervised learning techniques may be researched further. According to reviewed articles ( Choubey & Gautam, 2020 ; More et al., 2021 ; Muaz, Jayabalan & Thiruchelvam, 2020 ; Shirgave et al., 2019 ), unsupervised or semi-supervised learning techniques such as one-SVM, isolation forest, and K-means clustering should be utilised more in credit card fraud detection.
In the three years, DL techniques have been examined increasingly frequently. Utilising DL to get greater accuracy and efficient performance. By applying DL techniques, new fraudulent patterns can be recognised and system can respond flexibly to complex data patterns. Thus, for efficient credit card fraud detection, researchers are encouraged to conduct additional study on DL techniques. Several studies such as ( Benchaji, Douzi & El Ouahidi, 2021 ; Jonnalagadda, Gupta & Sen, 2019 ; Kalid et al., 2020 ) suggested further study of DL techniques for detection in credit card. Moreover, as each ML/DL technique has its own limitations, it is necessary to consider combining the ML and DL algorithms for promising detection results. Several article such as ( Agarwal, 2021 ; Dang et al., 2021 ; Gamini et al., 2021 ; Kalid et al., 2020 ; Singh & Jain, 2019 ) suggested combinations of DL methods and traditional ML methods to cyber fraud detection from an unbalanced data and enhance the accuracy.
Several reviewed article cited the lack of the dataset as the limitation of their work. According to Meenu et al. (2020) , the research outcomes will be more effective and of higher quality if the financial institutions make the crucial data set of various fraudulent actions available. As a result, one of the key problems in many studies is the lack of data. Limitations on the availability of the data could be overcome if there is a vital data set of diverse fraudulent activities across nations. Maniraj et al. (2019) noted that when dataset size increase, algorithm precision also increases. It appears that adding additional data will undoubtedly increase the model’s ability to detect fraud and decrease the number of false positives. The banks themselves must formally support this. The study ( Seera et al., 2021 ) proposed conducting further evaluation of their generated model with real data from diverse regions.
Additionally, the datasets are significantly skewed, which is a problem. Numerous studies attempted to develop a model that could perform properly with data that is highly skewed. Several articles ( Balne, Singh & Yada, 2020 ; Ojugo & Nwankwo, 2021 ; Shekar & Ramakrisha, 2021 ; Voican, 2021 ; Vengatesan et al., 2020 ), unbalanced data was applied, and balancing the dataset using sampling techniques such as oversampling or undersampling is left as future work. Several articles ( Ahirwar, Sharma & Bano, 2020 ; Almhaithawi, Jafar & Aljnidi, 2020 ; Manlangit, Azam & Shanmugam, 2019 ) applied oversampling techniques.
Undersampling techniques have been applied in several article ( Amusan et al., 2021 ; Ata & Hazim, 2020 ; Muaz, Jayabalan & Thiruchelvam, 2020 ; Rezapour, 2019 ; Zhang, Bhandari & Black, 2020 ). In Amusan et al. (2021) , a random undersampling technique was used, and the study recommended that other balancing data techniques be explored. One reviewed article ( Ata & Hazim, 2020 ) applied an undersampling technique. However, the study recommends adopting the suggested model by using massive dataset instead of using sampling technique. In addition, some articles such as Trisanto et al. (2021) and Singh, Ranjan & Tiwari (2021) applied undersampling techniques and oversampling techniques.
Oversampling technique such as SMOTE, ADASYN, DBSMOTE, and SMOTEEN have been used. Undersampling techniques such as random undersampling (RUS) has been applied. In light of this, future studies should consider applying alternative oversampling techniques, such as borderline-SMOTE and borderline oversampling with SVM, as well as undersampling techniques. In addition to fraud location, an algorithm to determine the timing of the fraud is required ( Alghofaili, Albattah & Rassam, 2020 ; Chen & Lai, 2021 ). In addition, an algorithm can be developed to predict fraudulent transactions in a real-time and deploying the service on various cloud platforms to make it easily accessible and reliable ( Ingole et al., 2021 ).
Limitation of the review
Our review is restricted to journal article published in 2019, 2020, and 2021 that apply ML/DL techniques. By using our methodology in the early stages, we eliminated several irrelevant article. This assured that the selected article met the requirements for our review. Even though we searched the most prominent digital libraries for the article, there may be more digital libraries having relevant research article that were not included for this study. The snowballing method used to include relevant article that excluded during automatic searching in order to address this limitation. In addition, as it is probable that while looking for the keywords, we would have missed some synonyms. Hence, we also analysed the search terms and keywords for recognised collection of research works. We restricted our search to only English-language articles. This creates a language bias, as there may be article in this field of study written in other languages.
Conclusions
This review studied cyber fraud detection in credit card using ML/DL techniques. We examined ML/DL models from the perspectives of ML/DL technique type, ML/DL performance estimation, and the learning-based fraud detection. The study focused on relevant studies that were published in 2019, 2020, and 2021. In order to address the four research questions posed in this study, we reviewed 181 research article. In our review, we have provided a detailed analysis of ML/DL techniques and their function in credit card cyber fraud detection and also offered recommendations for selecting the most suitable techniques for detecting cyber fraud. The study also includes the trends of research, gaps, future direction, and limitations in detecting cyber fraud in credit cards. We believe that this comprehensive review enables researchers and banking industry to develop innovation systems for cyber fraud detection.
On the basis of this analysis, we suggest that more research may be conducted on semi-supervised learning and unsupervised learning techniques. Based on our review, we recommend that DL techniques might be further researched for credit card cyber fraud detection. Researchers are encouraged to conduct further research on integrating the ML/DL algorithms for effective detection outcomes. In addition, researchers are advised to use both oversampling and undersampling techniques because the datasets are extremely skewed. Furthermore, we recommend researchers to mention dataset sources and performance metrics employed to present the outcomes. Banks are also encouraged to make available dataset of different fraudulent activities across nation for further research.
Funding Statement
The authors received no funding for this work.
Additional Information and Declarations
The authors declare that they have no competing interests.
Eyad Abdel Latif Marazqah Btoush conceived and designed the experiments, performed the experiments, analyzed the data, prepared figures and/or tables, authored or reviewed drafts of the article, and approved the final draft.
Xujuan Zhou conceived and designed the experiments, prepared figures and/or tables, authored or reviewed drafts of the article, and approved the final draft.
Raj Gururajan conceived and designed the experiments, performed the experiments, prepared figures and/or tables, authored or reviewed drafts of the article, and approved the final draft.
Ka Ching Chan conceived and designed the experiments, analyzed the data, prepared figures and/or tables, authored or reviewed drafts of the article, and approved the final draft.
Rohan Genrich conceived and designed the experiments, prepared figures and/or tables, authored or reviewed drafts of the article, and approved the final draft.
Prema Sankaran conceived and designed the experiments, prepared figures and/or tables, authored or reviewed drafts of the article, and approved the final draft.
Watch CBS News
We may receive commissions from some links to products on this page. Promotions are subject to availability and retailer terms.
How to find the best credit card debt forgiveness programs
By Angelica Leicht
Edited By Matt Richardson
July 25, 2024 / 4:23 PM EDT / CBS News
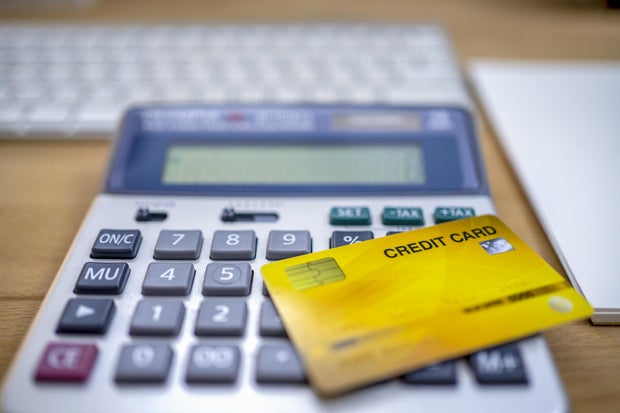
If you aren't paying off your credit card balances in full each month, you may be putting yourself in a precarious financial position. After all, the average credit card rate has increased significantly over the last couple of years, climbing from 16.65% in 2022 to nearly 23% currently. That's an uptick of about 36% in just two years.
So, if you're carrying a balance from month to month right now, chances are that you're dealing with a hefty amount of interest on what you owe. That alone can make it tough to balance your credit card payments with your other financial obligations. And, if your credit card balances continue to grow over time, there could be a point when it's tough to make the minimum payments on your cards.
Should that happen, the good news is that there are debt relief solutions , like credit card debt forgiveness , that can help. When you enroll in a credit card debt forgiveness program, a debt relief company negotiates with your creditors to try and reduce what you owe in return for a lump-sum payment. That can be a good path to eliminating card debt , but it's important to find the right program first.
See which credit card debt forgiveness options are available to you now .
These steps can help you determine what the best credit card debt forgiveness programs are for you:
Research reputable debt relief companies
Start by researching what well-established debt relief companies offer credit card debt forgiveness programs in your area. It can help to look for organizations accredited by the American Fair Credit Council (AFCC) or the International Association of Professional Debt Arbitrators (IAPDA). These accreditations indicate that the companies adhere to industry best practices and ethical standards.
It's also important to make sure that any debt relief company you're considering is properly licensed to operate in your state. You can check with your state's attorney general's office or consumer protection agency for this information.
Start comparing the top debt relief companies and learn more about your options today .
Check reviews and ratings
Read reviews from previous clients on independent review sites like the Better Business Bureau (BBB), Trustpilot or Consumer Affairs. And, during the process, be sure to pay attention to both positive and negative feedback. That way, you can get a balanced view of each company's performance and reputation.
Take advantage of free consultations
Most reputable debt relief companies offer free initial consultations, so be sure to take advantage of the opportunity to speak with experts from multiple companies. That way, you can ensure you're getting the best possible service and terms .
During the consultation process, ask each company about their programs, fees and approach to debt settlement. By comparing these factors you can better determine which companies make the most sense for you. Be sure to also ask each company about their success rates in negotiating with creditors. While past performance doesn't guarantee future results, it can give you an idea of the company's effectiveness.
Consider other factors, like creditor relationships and suppor t
It can also help to look for companies that offer personalized debt forgiveness plans tailored to your specific financial situation. The ability to adjust your plan as your circumstances change can be crucial for long-term success.
You should also weigh whether the debt forgiveness programs have established relationships with creditors, which could potentially lead to more favorable negotiations. While this shouldn't be the sole deciding factor, it could be beneficial if a company has a good track record with your specific creditors.
Evaluate the level of support and communication each debt forgiveness program offers as well. Do they provide a dedicated account manager? How often will you receive updates on your progress? And, what channels are available for you to reach out with questions or concerns?
Be on the lookout for red flags
You may want to rule out any companies that make unrealistic promises or use high-pressure sales tactics . Legitimate debt relief firms will be transparent about the risks and limitations of their programs, so be cautious of any company that:
- Guarantees to settle all your debts for a specific percentage
- Promises to settle your debts extremely quickly (e.g., in a few months)
- Asks for upfront fees before any debts are settled
- Claims their program has no negative impact on your credit score
The bottom line
If you want to find the best credit card debt forgiveness program, you'll need to do some research and take numerous factors into consideration. After all, while these programs can offer relief for those struggling with overwhelming debt, they're not without risks and drawbacks. But by thoroughly vetting potential debt relief companies, understanding the process and considering all your options, you can make an informed decision about whether debt forgiveness is the right choice for your financial situation — and which program makes the most sense for you.
Angelica Leicht is senior editor for Managing Your Money, where she writes and edits articles on a range of personal finance topics. Angelica previously held editing roles at The Simple Dollar, Interest, HousingWire and other financial publications.
More from CBS News
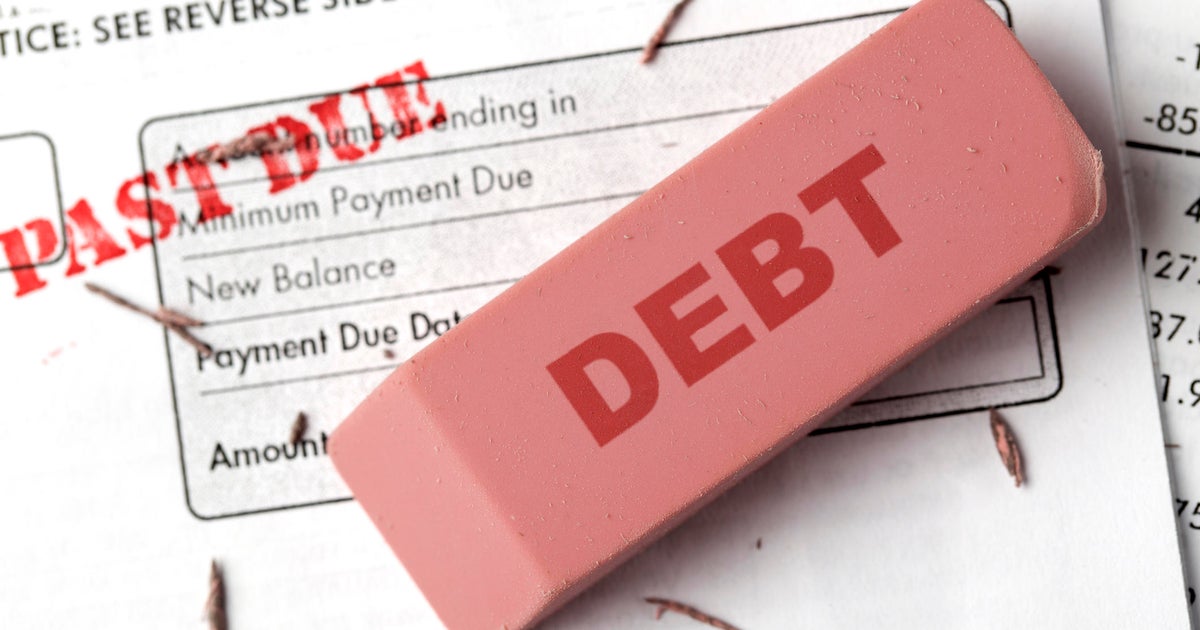
Credit card delinquencies hit a 12-year high. 4 ways to resolve yours now
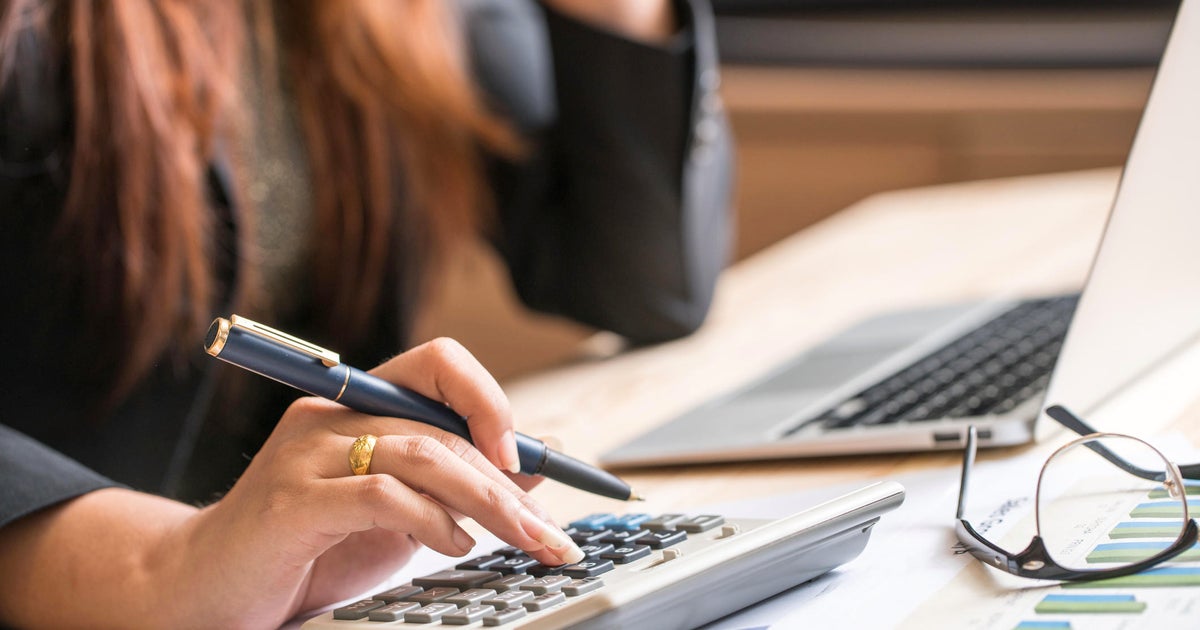
How much does a $80,000 HELOC cost monthly?
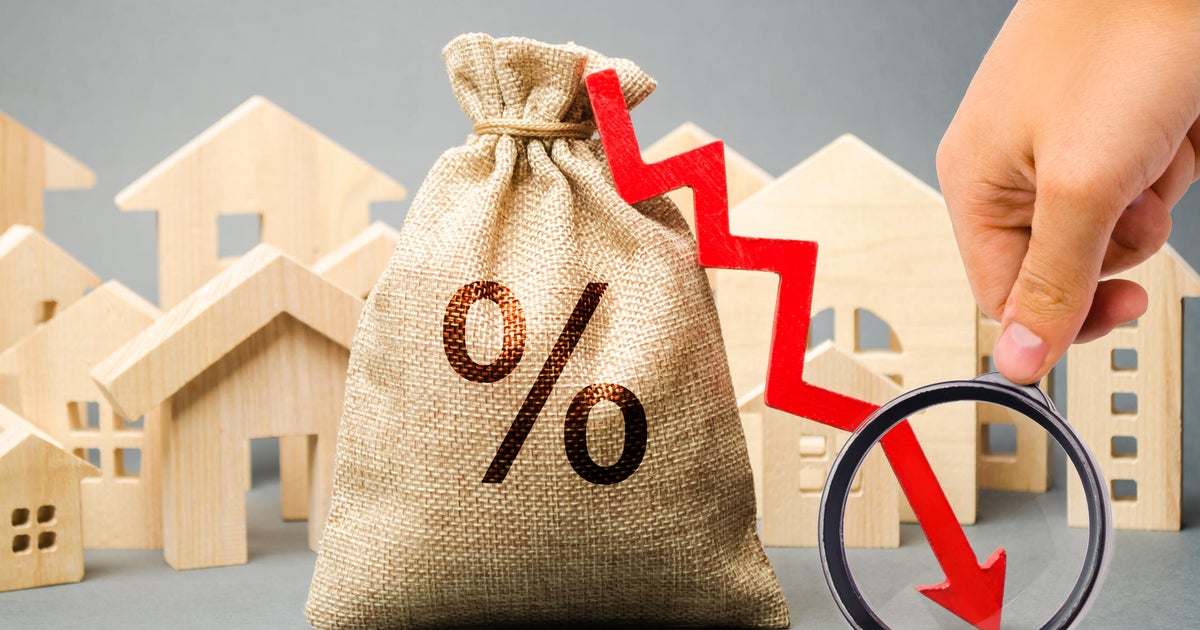
3 ways mortgage interest rates could drop this August
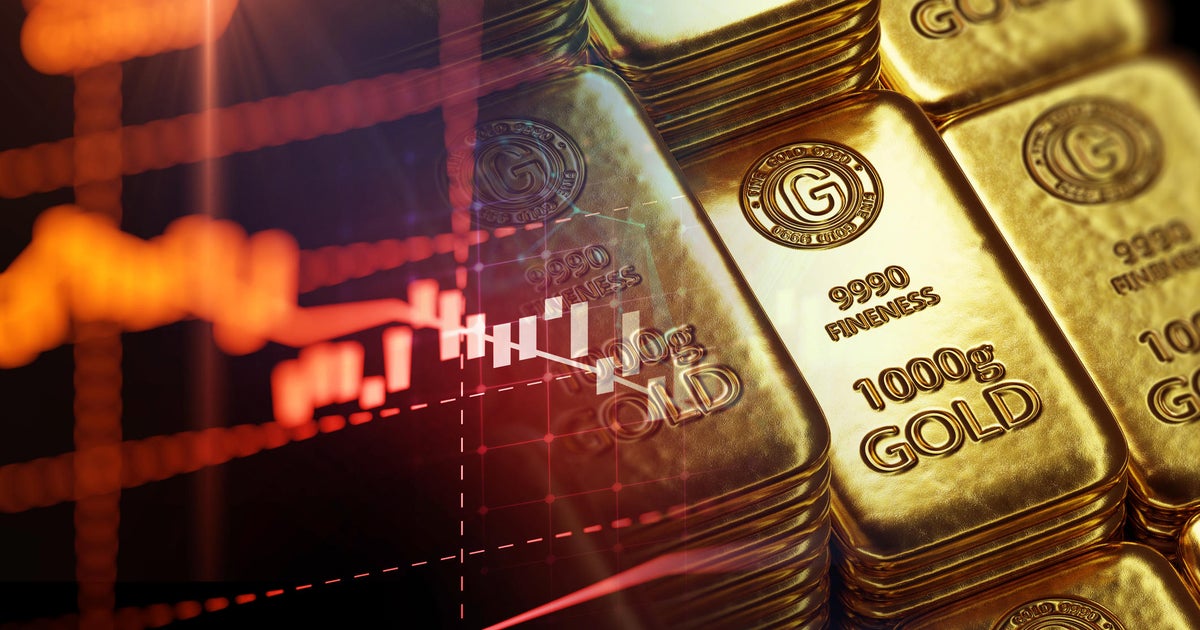
Gold prices are falling. 5 smart moves to make now
This device is too small
If you're on a Galaxy Fold, consider unfolding your phone or viewing it in full screen to best optimize your experience.
How Many Americans Have a Perfect Credit Score?
Published on July 25, 2024
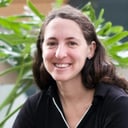
By: Christy Bieber
- Just 1.31% of Americans have a perfect credit score.
- The average credit score is 714.
- Getting your score up to the very good range is sufficient to qualify for the best borrowing rates.
There are different formulas for assigning a credit score. However, FICO is the most commonly used credit scoring formula. FICO® Scores fall on a scale of 300 to 850, with higher scores awarded to those with a better credit history.
A score of 850 is considered to be a perfect score on this scale, but very few people have earned an 850 score or even come close to it.
Having perfect credit is very rare
According to credit score research from The Motley Fool Ascent, just 1.31% of all Americans who have a FICO® Score have earned a perfect 850. However, the rate of people with perfect credit does vary significantly by age.
The table below shows which demographic groups are most likely to have achieved perfection.
Scores by generation | Percent of 850 FICO® Scores |
Gen Z | 0.1% |
Millennials | 4.1% |
Gen X | 22.4% |
Baby boomer | 59.4% |
Silent | 14.0% |
As you can see, older Americans make up a higher percentage of people with perfect credit than their younger counterparts. That's because it's really hard to get a perfect score. You need:
- A good mix of different kinds of credit. That's why people with 850 scores have more credit cards than the average person and higher personal loan balances, as well.
- No delinquencies. Delayed payments can send your score plummeting. While the average American has 1.8 delinquent payments, those with perfect scores have none.
- A lower credit utilization ratio. Using a small percentage of your credit is important, which is why people with perfect scores have lower credit card balances.
A long average age of credit and limited inquiries are also important if you want that coveted 850, as short credit histories and too many inquiries count against you.
Do you need a perfect 850 credit score?
With so few people having perfect credit, you may feel as if achieving a score of 850 is hopeless. The good news is, you don't have to in order to be able to get the best rates and terms from creditors and in order to be able to get approved for the most competitive credit cards .
A credit score between 670 and 739 is considered to be good, while a score of 740 to 799 is classified as very good, and a score of 800 to 850 is considered to be exceptional. Since the average score among all Americans is 714, most people don't have a lot to worry about. They're well within the good credit range, so they shouldn't have too hard a time borrowing.
Of course, it doesn't hurt to try for a perfect 850 if you want. After all, maintaining good credit habits can help you out, as can a high credit score, so you can only benefit from making responsible choices to try to earn a perfect score. No one is ever going to regret paying their bills on time or not carrying large balances on their credit cards, even if their score only ends up in the 750 range.
Just don't be discouraged if you don't achieve 850 -- especially if you're young, since some factors like average credit age can be out of your control. Keep working on improving your personal score, check your credit often to be sure there are no problems, and rest assured if you're above 670, you're probably doing fine. And if you aren't at that level yet, just keep making those on-time payments and whittling away debt and you'll get there.
Alert: our top-rated cash back card now has 0% intro APR until 2025
This credit card is not just good – it’s so exceptional that our experts use it personally. It features a lengthy 0% intro APR period, a cash back rate of up to 5%, and all somehow for no annual fee! Click here to read our full review for free and apply in just 2 minutes.
Our Research Expert
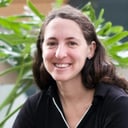
Christy Bieber is a full-time personal finance and legal writer with 15 years of experience. She has a JD from UCLA and is a former college instructor.
Share this page
We're firm believers in the Golden Rule, which is why editorial opinions are ours alone and have not been previously reviewed, approved, or endorsed by included advertisers. The Ascent, a Motley Fool service, does not cover all offers on the market. The Ascent has a dedicated team of editors and analysts focused on personal finance, and they follow the same set of publishing standards and editorial integrity while maintaining professional separation from the analysts and editors on other Motley Fool brands.
Related Articles
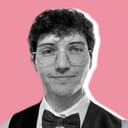
By: Cole Tretheway | Published on June 7, 2024
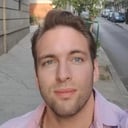
By: Lyle Daly | Published on June 5, 2024
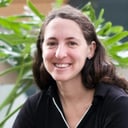
By: Christy Bieber | Published on June 5, 2024
By: Lyle Daly | Published on June 4, 2024
The Ascent is a Motley Fool service that rates and reviews essential products for your everyday money matters.
Copyright © 2018 - 2024 The Ascent. All rights reserved.
Advertisement
Supported by
Why Paper Checks Refuse to Die
It’s hard to avoid hassle — or fraud — when you’re required to pay with paper and ink. Here’s why checks persist and why some people don’t mind.

By Ron Lieber
Ron Lieber read several hundred tips from Your Money newsletter subscribers while reporting this column.
Target stopped accepting personal checks as a form of payment this month, which might inspire the following question: What took so long?
Check fraud has more than doubled in recent years, and it costs at least a dollar for businesses to process each check they receive. Plenty of young adults have never even written one.
But if you haven’t used a check in years and consider it a badge of honor, that may say a lot about where you live and what you pay for. In many industries, checks continue to be a popular form of payment, and sometimes they are required.
According to consumer survey data from the Federal Reserve Bank of Atlanta, which tracks the percentage of payments that consumers make by check, the following industries receive the most check payments: Contractors, like electricians and plumbers, get 25 percent of their payments by check. Charitable and religious organizations are next at 22 percent. Landlords, government taxing authorities and professional-service firms also receive double-digit percentages of their payments by check.
There are many pockets of commerce in which checks are required. Readers of Your Money newsletter wrote in to complain about having to write checks for a number of things, like homeowners’ association dues and haircuts, along with dog shows and the occasional long-term care insurance policy.
Some people actually like it this way, though they cite many different reasons. Feelings play a role, as do fees. Fear does, too.
We are having trouble retrieving the article content.
Please enable JavaScript in your browser settings.
Thank you for your patience while we verify access. If you are in Reader mode please exit and log into your Times account, or subscribe for all of The Times.
Thank you for your patience while we verify access.
Already a subscriber? Log in .
Want all of The Times? Subscribe .

IMAGES
VIDEO
COMMENTS
Figure 1 shows how the average U.S. consumer's credit card limit and debt varied significantly from 2000-2014. From 2000-2008, the average credit card limit increased by approximately 40 percent, from around $10,000 to a peak of $14,000. During 2009, overall limits collapsed rapidly before recovering slightly in 2012.
Objective: This paper aims at sectoral analysis of the credit card industry in India by considering top three credit card issuers i.e., HDFC bank, SBI Cards, and ICICI Bank. Methodology: In order ...
Revolving Credit. This paper investigates the implication of bank revolving credit in the form of credit card loans as a channel of monetary policy targeting the federal funds rate since 1980. Credit cards have become increasingly popular and a necessity for many transactions and purchases in the United States.
Abstract. Credit card frauds are easy and friendly targets. E-commerce and many other online sites have increased the online payment modes, increasing the risk for online frauds. Increase in fraud rates, researchers started using different machine learning methods to detect and analyse frauds in online transactions.
The work in Al-Hashedi and Magalingam (2021) covers research papers on financial fraud in general from 2009 to 2019 inclusive. It mainly discusses works based on data mining techniques and classifies the literature based on range of factors, including publication year, publisher, method used, and research area (credit fraud, cryptocurrency ...
The model was trained on a real credit card dataset and the customer behavioural scores are analysed using classical measures such as accuracy, Area Under the Curve, Brier score, Kolmogorov-Smirnov test, and H-measure. ... Therefore, the research of this paper is motivated by the necessity of automatically scoring the customer's behaviour ...
Research in the area of consumer credit card abundance of literature in the business, psychology, and public policy fields. 1960s, the work revolved around descriptive characteristics and evolved as scholars probed deeper by investigating ... Since the first paper on consumer credit cards was published in 1969, researchers have attempted to ...
Consumers prefer credit cards due to uncertainty when carrying cash (Khare et al., 2012) or special discounts from famous brands (Dali et al., 2015). They use credit cards as a source of revolving credit with long grace period (Chahal et al., 2014; Khare et al., 2012). They can even withdraw cash by credit cards as required (Chahal et al., 2014).
Credit cards are central to the financial lives of over 175 million American consumers. Over the last few years and through 2019, the credit card market, the largest U.S. consumer lending market measured by number of users, continued to grow in almost all measures until suddenly reversing course in March 2020.
Many researchers have investigated the consumer's attitude towards using credit cards. However, how the different attributes contribute to credit card usage attitude is not evident. Thus, the main theoretical contribution of this study is to examine the importance and performance of a set of variables that explain the attitude towards using credit cards. It provides essential inputs to ...
1.3 "A Research Paper on Credit Card Fraud Detection" The proposed model involves pre-processing the credit card transaction data and then apply- ing various
1 Introduction. In this paper, we report results from the fi rst field experiment to examine the impact of. credit cards on spending, a quest ion of great interest for economics, law and public ...
When the research is based on big data analytics, there will be a huge volume of data which can be implemented in Apache Hadoop, Spark, etc. Tensorflow, H2O, Pytorch, Keras, etc. are the libraries imported in the application of deep learning. ... Artikis, A., et al.: A prototype for credit card fraud management: industry paper. In: Proceedings ...
1. Introduction. Both the issuance of credit cards and the scale of credit have increased steadily in recent years. According to data from the People's Bank of China, at the end of 2020, the number of credit cards issued totaled 778 million, and the credit balance of credit cards was 7.91 trillion yuan.
As credit card becomes the most popular payment mode particularly in the online sector, the fraudulent activities using credit card payment technologies are rapidly increasing as a result. For this end, it is obligatory for financial institutions to continuously improve their fraud detection systems to reduce huge losses. The purpose of this paper is to develop a novel system for credit card ...
According to the U.S. Credit Card Statistics in 2021, 70.2% of consumers have at least one credit card, and 14% have at least ten. Moreover, the number of credit card accounts increased by 2.5% year-over-year, implying that credit cards have become a primary and vital payment method in modern societies.
Compulsive buying behaviour and credit card could have a powerful effect on consumers' financial stability. Further, in place of comprehending credit card usage and compulsive buying, this study correlates them with wealth attitudes such as power-prestige, financial knowledge and retention time.
card statistics 2021) the number of people using credit cards around the world was 2.8 billion in 2019, in addition 70% of those users own a single card at least. Reports of Credit card fraud in the US rose by 44.7% from 271,927 in 2019 to 393,207 reports in 2020. There are two kinds of credit card fraud, the first one is by having a credit
The recent advances of e-commerce and e-payment systems have sparked an increase in financial fraud cases such as credit card fraud. It is therefore crucial to implement mechanisms that can detect the credit card fraud. Features of credit card frauds play important role when machine learning is used for credit card fraud detection, and they must be chosen properly. This paper proposes a ...
Using a large sample of credit bureau data, this paper documents a tight link between available credit (the limit) and credit card debt, and then it offers a model-based interpretation of this linkage. Credit limits change frequently for individuals, increase rapidly on average as people age, and show large changes over the business cycle.
Since the first paper on consumer credit cards was published in 1969, researchers have attempted to develop a demographic profile of credit card consumers. The demographic characteristics most often used were age (22 studies) and gender (20 studies), followed by income (11 studies) and education (5 studies).
The review investigates the present status of research on detecting cyber fraud in credit card and addresses our research questions. The methodology begins with a description of the data sources, the search strategy, the inclusion and exclusion criteria, as well as the quantity of research article selected from the different databases. ...
Credit cards, a combination of payment card and personal consumption credit, are widely used in around the world. Starting with a relationship between vendors and consumers, as well as a need to buy rst and pay later, Franklin National Bank in New York, the USA, issued rst-ever fi fi credit cards to market in 1951.
Using unique credit bureau data, which tracks consumers from initial entry to subsequent participation in the credit card market, we document significant gender-based sorting across credit card products: women are 35% more likely than men to open a retail store credit card as opposed to a general-purpose credit card when they first
In particular, make sure to pay credit cards and loans on time, because these payments get reported on your credit history. Have at least one credit card that you use regularly. To build credit ...
After all, the average credit card rate has increased significantly over the last couple of years, climbing from 16.65% in 2022 to nearly 23% currently. That's an uptick of about 36% in just two ...
Review our list of the best credit cards for Costco to find out more. Alert: our top-rated cash back card now has 0% intro APR until 2025 This credit card is not just good - it's so ...
When you use your credit cards to pay for everyday purchases, you can earn points, miles, or cash back that can save you money. Alert: our top-rated cash back card now has 0% intro APR until 2025
According to credit score research from The Motley Fool Ascent, just 1.31% of all Americans who have a FICO® Score have earned a perfect 850. However, the rate of people with perfect credit does ...
In Queen Anne's County in Maryland, the service charge for processing a credit-card payment is a 2.95 percent flat fee. If you want to use what the county calls E-checks to pay directly from ...