Academia.edu no longer supports Internet Explorer.
To browse Academia.edu and the wider internet faster and more securely, please take a few seconds to upgrade your browser .
Enter the email address you signed up with and we'll email you a reset link.
- We're Hiring!
- Help Center
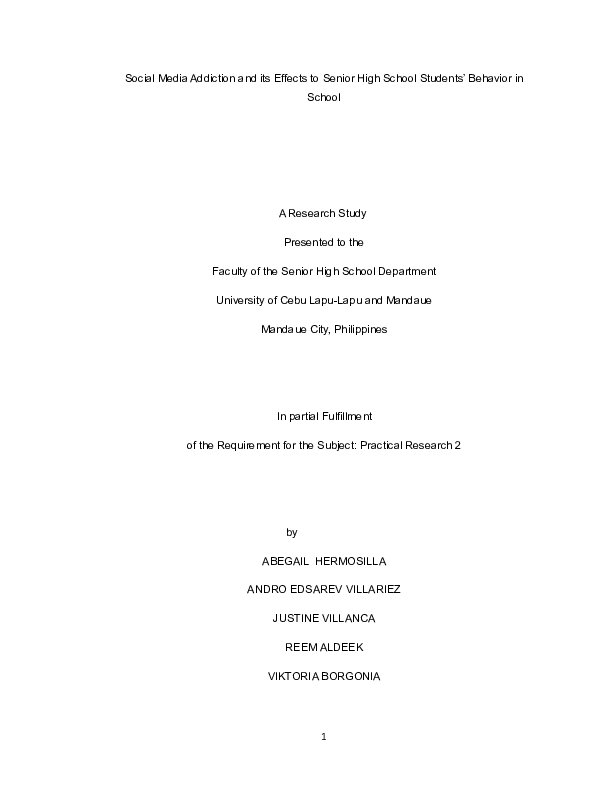
Download Free PDF

Social Media Addiction and its Effects to Senior High School Students' Behavior in School A Research Study
Related papers
Enough (or maybe not so much) has been said about social media and its adverse consequences on humans, particularly the young ones. Yet, the concept is inexhaustible, it remains elusive as we are in a constant flux from one existing social platform to a new and more advanced, sophisticated social platform. Our aim, therefore is to look critically into the adverse effects of excessive use of social media on our growing teens especially in our contemporary era. The question is not whether social media is good or bad but how much good can be derived from it and how much efforts we are putting to curb the bad, negative effects of social media. To begin, what does it mean to be social? What is media? To be social means relating to society or its organization, to interact, mingle and contribute to the betterment of such society or community as the case may be. Media, on the other hand, are the communication outlets or tools used to store and deliver information or data. Thus, when we put these two words together, we have what is called social media. What is worthy of note in this exercise is that social media go beyond the popular Facebook, Twitter, WhatsApp and the likes. What then is social media? What is social media? According to Investopedia, an online resource, "social media is a computer-based technology that facilitates the sharing of ideas, thoughts, and information through the building of virtual networks and communities." By design, social media is Internet-based and gives users quick electronic communication of content. Content includes personal information, documents, videos, and photos. Users engage with social media via a computer, tablet, or smartphone via web-based software or applications.
The Island - Sunday News Paper, 2018
Social media is an internet medium through which people or communities connect. Common reasons for social networking are building and maintaining relationships, expressing beliefs and ideas, sharing information, or even combating boredom. Communication can be conducted by communities or groups interacting and sharing information. The most commonly used global social networking sites are Facebook, Twitter, Myspace, and LinkedIn. They have become increasingly popular, and social media has become a vital daily routine for most teenagers. most renowned social network, Facebook, launched in 2004; it currently has over one billion active users and is still growing.
Social media has become an increasingly important part of all cultures around the World. Twitter, Facebook, LinkedIn, Multiply and other social media sites have swept the nation and have come to dominate the millennial generation way of thinking and behaving. Social media allows interactive dialogue and social interaction with many people who in this day spend a lot of great deal online such as working, leisure, entertaining, and so on. Many cannot deny that using internet is helped to find the great deal of information and spend less time to do so. Until today there are still surprisingly few studies conducted on the subject matter of symptoms of Internet addiction and what can and cannot be classified as such, making this so called pathological sickness debatable. Many claimed that social media is merely a channel or medium that leads to other addictions. Therefore, many researchers have been working on the benefit of using internet. However, in this study the researcher searched ...
Social media's impact on youth is creating additional challenges and opportunities. Social Networking sites provide a platform for discussion on burning issues that has been overlooked in today's scenario. The impact of social networking sites in the changing mind-set of the youth. It was survey type research and data was collected through the questionnaire. 300 sampled youth fill the questionnaire; non-random sampling technique was applied to select sample units. The main objectives were as (1) To analyze the influence of social media on youth social life (2) To assess the beneficial and preferred form of social media for youth (3) To evaluate the attitude of youth towards social media and measure the spending time on social media (4) To recommend some measure for proper use of social media in right direction to inform and educate the people. Collected data was analyzed in term of frequency, percentage, and mean score of statements. Following were main findings Majority of the respondents shows the agreements with these influences of social media. Respondents opine Facebook as their favorite social media form, and then the like Skype as second popular form of social media, the primary place for them, 46 percent responded connect social media in educational institution computer labs, mainstream responded as informative links share, respondents Face main problem during use of social are unwanted messages, social media is beneficial for youth in the field of education, social media deteriorating social norms, social media is affecting negatively on study of youth. Social media promotes unethical pictures, video clips and images among youth, anti-religious post and links create hatred among peoples of different communities, Negative use of social media is deteriorating the relationship among the countries, social media is playing a key role to create political awareness among youth. Introduction Social media is most recent form of media and having many features and characteristics. It have many facilities on same channel like as communicating ,texting, images sharing , audio and video sharing , fast publishing, linking with all over world, direct connecting. it is also cheapest fast access to the world so it is very important for all age of peoples. Its use is increasing day by day with high rate in all over the
Journal of Education Technology in Health Sciences, 2023
Abstract Utilizing the technology made our life very easier and brought the globe in our hand which has got both pros and cons. Young generation is more of techno oriented than the values that makes them to be depending on the social medias easily that affects the domains of health. A study was conducted to assess the Social media addiction among the paramedical students. Quantitative research approach with non experimental, descriptive research design was used. Non probability convenient sampling technique was used to select 140 para medical students who fulfills the inclusion criteria. Self administered structured questionnaire was used. Modified social media addiction likert scale was used with 20 items. Findings of the study shows that vast majority (103(74%)) of the students were addicted to the social media. To conclude, it is the high time for the policy-makers to restrict on this and make provision to improve the interaction skills. Keywords: Social media addiction, Social interactions.
Loading Preview
Sorry, preview is currently unavailable. You can download the paper by clicking the button above.
European Journal of Molecular & Clinical Medicine, 2020
Research Journey, 2019
Science Insights, 2017
Journal of information and communication convergence engineering, 2014
IRJET, 2021
isara solutions, 2023
www.ijiemr.org, 2023
International Journal of All Research Education & Scientific Methods
AJIT-e: Online Academic Journal of Information Technology, 2019
Journal of Medical Science And clinical Research, 2018
International Journal of E-Business Research
Zenodo (CERN European Organization for Nuclear Research), 2022
- We're Hiring!
- Help Center
- Find new research papers in:
- Health Sciences
- Earth Sciences
- Cognitive Science
- Mathematics
- Computer Science
- Academia ©2024
- DOI: 10.6007/ijarbss/v14-i1/20536
- Corpus ID: 267046239
Social Media Addiction and Academic Performance: A Bibliometric Analysis Approach
- Olayiwola Alfa Abdullahi , Mahadi Bahari , +1 author M. H. Abu Yazid
- Published in International Journal of… 10 January 2024
- Education, Computer Science, Psychology
One Citation
Social media use and academic performance among students at pahang’s public higher education institutions: a correlation study, 26 references, social media addiction and its impact on college students' academic performance: the mediating role of stress, effects of social media on psychological wellbeing and academic performance among university students in sindh, implications of social media addiction on academic performance among generation z student-athletes during covid-19 lockdown, social media addiction: its impact, mediation, and intervention, the relationship between social networking addiction and academic performance in iranian students of medical sciences: a cross-sectional study, impact of social media on students' academic performance & generation gap: a study of public sector university in punjab, the relations among social media addiction, self-esteem, and life satisfaction in university students, investigating social media usage and addiction levels among undergraduates in university of ibadan, nigeria.
- Highly Influential
Understanding students’ behavior in online social networks: a systematic literature review
Technology addictions and technostress: an examination of the u.s. and china, related papers.
Showing 1 through 3 of 0 Related Papers

Search Query Search
Social media addiction: Its impact, mediation, and intervention
Vol.13, no.1 (2019).
Yubo Hou Dan Xiong Tonglin Jiang Lily Song Qi Wang
https://doi.org/10.5817/CP2019-1-4
Download Citation
- Author biographies
- Additional information
This research examined the relations of social media addiction to college students' mental health and academic performance, investigated the role of self-esteem as a mediator for the relations, and further tested the effectiveness of an intervention in reducing social media addiction and its potential adverse outcomes. In Study 1, we used a survey method with a sample of college students ( N = 232) and found that social media addiction was negatively associated with the students' mental health and academic performance and that the relation between social media addiction and mental health was mediated by self-esteem. In Study 2, we developed and tested a two-stage self-help intervention program. We recruited a sample of college students ( N = 38) who met criteria for social media addiction to receive the intervention. Results showed that the intervention was effective in reducing the students’ social media addiction and improving their mental health and academic efficiency. The current studies yielded original findings that contribute to the empirical database on social media addiction and that have important theoretical and practical implications.
Peking University, China
Yubo Hou is an associate professor at Peking University's School of Psychological and Cognitive Sciences and Beijing Key Laboratory of Behavior and Mental Health, as well as a core member of the Center for Cultural Psychology at Tsinghua University. His major research interests include organizational behavior, personality and social psychology, social media, and cultural psychology. He is well-known for his cross-cultural research on the thinking styles of Chinese and Western populations. His current work focuses on behavioral problems and Confucian style of coping among Chinese adults, and the influence of social media on psychological wellbeing. Hou holds a BSc in Psychology from Zhejiang University and a Ph.D. in Social Psychology from Peking University.
Southwest University, China, Peking University, China
Dan Xiong, Assistant Professor, Faculty of Psychology, Southwest University
Tonglin Jiang
Peking university, china the university of hong kong, hong kong.
Tonglin Jiang, Ph.D candidate, Department of Psychology, The University of Hong Kong.
Lily Song, Ph.D candidate, Institute of Psychology, Chinese Academy of Science.
Cornell University, The USA
Qi Wang is a professor and department chair in Human Development at Cornell University. Her research integrates developmental, cognitive, and sociocultural perspectives to examine the mechanisms underlying the development of a variety of social-cognitive skills, including autobiographical memory, self, future thinking, and emotion knowledge. She has undertaken extensive studies to examine how cultural beliefs and goals influence social cognitive representations and processes by affecting information processing at the level of the individual and by shaping social practices between individuals. In addition, she has conducted studies to examine the impact of Internet technology as a cultural force unique to our time on cognitive functioning and well-being. A graduate of Peking University, China, Qi Wang earned a Ph.D. in psychology in 2000 at Harvard University. She has received many honors and awards and has over one hundred and fifty publications in scientific journals and in volumes of collected works. Her single-authored book, The Autobiographical Self in Time and Culture (2013, Oxford University Press), is regarded as the definitive work on culture and autobiographical memory.
Al-Menayes, J. J. (2014). The relationship between mobile social media use and academic performance in university students. New Media and Mass Communication, 25, 23–29.
Al-Menayes, J. J. (2015). Social media use, engagement and addiction as predictors of academic performance. International Journal of Psychological Studies, 7, 86-94. http://dx.doi.org/10.5539/ijps.v7n4p86
Alabi, O. F. (2012). A survey of Facebook addiction level among selected Nigerian University undergraduates. New Media and Mass Communication, 10, 70–80. Andreassen, C. S. (2015). Online social network site addiction: A comprehensive review. Current Addiction Reports, 2, 175–184. https://doi.org/10.1007/s40429-015-0056-9
Andreassen, C. S., & Pallesen, S. (2014). Social network site addiction-an overview. Current Pharmaceutical Design, 20, 4053–4061. https://doi.org/10.2174/13816128113199990616
Andreassen, C. S., Pallesen, S., & Griffiths, M. D. (2017). The relationship between addictive use of social media, narcissism, and self-esteem: Findings from a large national survey. Addictive Behaviors, 64, 287–293. https://doi.org/10.1016/j.addbeh.2016.03.006
Andreassen, C. S., Torsheim, T., Brunborg, G. S., & Pallesen, S. (2012). Development of a Facebook addiction scale. Psychological Reports, 110, 501–517. https://doi.org/10.2466/02.09.18.PR0.110.2.501-517
Balakrishnan, V., & Shamim, A. (2013). Malaysian Facebookers: Motives and addictive behaviours unraveled. Computers in Human Behavior, 29, 1342–1349. https://doi.org/10.1016/j.chb.2013.01.010
Bányai, F., Zsila, Á., Király, O., Maraz, A., Elekes, Z., Griffiths, M. D., . . . Demetrovics, Z. (2017). Problematic social media use: Results from a large-scale nationally representative adolescent sample. PLoS One, 12, e0169839. https://doi.org/10.1371/journal.pone.0169839
Baumeister, R. F., & Leary, M. R. (1995). The need to belong: Desire for interpersonal attachment as a human motivation. Psychological Bulletin, 117, 497–529. https://doi.org/10.1037 /0033-2909.117.3.497
Błachnio, A., Przepiorka, A., & Pantic, I. (2016). Association between Facebook addiction, self-esteem and life satisfaction: A cross-sectional study. Computers in Human Behavior, 55, 701–705. https://doi.org/10.1016/j.chb.2015.10.026
Błachnio, A., Przepiorka, A., Senol-Durak, E., Durak, M., & Sherstyuk, L. (2017). The role of personality traits in Facebook and Internet addictions: A study on Polish, Turkish, and Ukrainian samples. Computers in Human Behavior, 68, 269–275. https://doi.org/10.1016/j.chb.2016 .11.037
Caplan, S. E. (2010). Theory and measurement of generalized problematic Internet use: A two-step approach. Computers in Human Behavior, 26, 1089–1097. https://doi.org/10.1016/j.chb.2010 .03.012
Casale, S., Rugai, L., & Fioravanti, G. (2018). Exploring the role of positive metacognitions in explaining the association between the fear of missing out and social media addiction. Addictive Behaviors, 85, 83–87. https://doi.org/10.1016/j.addbeh.2018.05.020
Caselli, G., Fernie, B., Canfora, F., Mascolo, C., Ferrari, A., Antonioni, M., Spada, M. M. (2018). The metacognitions about gambling questionnaire: Development and psychometric properties. Psychiatry Research, 261, 367–374. https://doi.org/10.1016/j.psychres .2018.01.018
Chou, H. T. G., & Edge, N. (2012). “They are happier and having better lives than I am”: The impact of using Facebook on perceptions of others’ lives. Cyberpsychology, Behavior, and Social Networking, 15, 117–121. https://doi.org/10.1089/cyber.2011.0324
Davis, R. A. (2001). A cognitive-behavioral model of pathological Internet use. Computers in Human Behavior, 17, 187–195. https://doi.org/10.1016/S0747-5632(00)00041-8
Echeburua, E., & de Corral, P. (2010). Addiction to new technologies and to online social networking in young people: A new challenge. Adicciones, 22, 91–95. https://doi.org/10.1016/j.addbeh.2009.09.003
Eraslan-Capan, B. (2015). Interpersonal sensitivity and problematic Facebook use in Turkish university students. The Anthropologist, 21, 395–403. https://doi.org/10.1080/09720073.2015 .11891829 Fang, L. T., Shi, K., & Zhang, F. H. (2008). Research on reliability and validity of Utrecht Work Engagement Scale- student. Chinese Journal of Clinical Psychology, 16, 618–620. https://doi.org/1005- 3611(2008)16:6<618:ZWBXXT>2.0.TX;2-X
Goldberg, D. P. (1972). The detection of psychiatric illness by questionnaire: A technique for the identification and assessment of nonpsychotic psychiatric illness. London: Oxford University Press.
Gonzales, A. L., & Hancock, J. T. (2011). Mirror, mirror on my Facebook wall: Effects of exposure to Facebook on self-esteem. Cyberpsychology, Behavior, and Social Networking, 14, 79–83. https://doi.org/10.1089/cyber.2009.0411
Griffiths, M. D. (2000). Internet addiction: Time to be taken seriously? Addiction Research, 8, 413–418. https://doi.org/10.3109/16066350009005587
Griffiths, M. D. (2010). The role of context in online gaming excess and addiction: Some case study evidence. International Journal of Mental Health and Addiction, 8, 119–125. https://doi.org/10.1007/s11469-009-9229-x
Griffiths, M. D. (2012). Facebook addiction: Concerns, criticism, and recommendations: A response to Andreassen and colleagues. Psychological Reports, 110, 518–520. https://doi.org/10.2466/01.07.18.PR0.110.2.518- 520
Gupta, V. K., Arora, S., & Gupta, M. (2013). Computer-related illnesses and Facebook syndrome: What are they and how do we tackle them. Medicine Update, 23, 676–679.
Hawi, N. S., & Samaha, M. (2017). The relations among social media addiction, self-esteem, and life satisfaction in university students. Social Science Computer Review, 35, 576–586. https://doi.org/10.1177/0894439316660340
Hong, F. Y., Huang, D. H., Lin, H. Y., & Chiu, S. L. (2014). Analysis of the psychological traits, Facebook usage, and Facebook addiction model of Taiwanese university students. Telematics and Informatics, 31, 597–606. https://doi.org/10.1016/j.tele.2014.01.001
Huang, H. (2014). Social media generation in urban China: A study of social media use and addiction among adolescents. Berlin: Springer.
Jelenchick, L. A., Eickhoff, J. C., & Moreno, M. A. (2013). “Facebook depression?” Social networking site use and depression in older adolescents. Journal of Adolescent Health, 52, 128–130. https://doi.org/10.1016/j.jadohealth.2012.05.008
Ji, Y. F., & Yu, X. (1993). Self-esteem scale (SES). Manual of mental health rating scale. Chinese Journal of Mental Health, 251.
Jiang, T., Hou, Y., & Wang, Q. (2016). Does micro-blogging make us “shallow”? Sharing information online interferes with information comprehension. Computers in Human Behavior, 59, 210–214. https://doi.org/10.1016/j.chb.2016.02.008
Junco, R. (2012). The relationship between frequency of Facebook use, participation in Facebook activities, and student engagement. Computers & Education, 58, 162–171. https://doi.org/10.1016/j.compedu.2011.08.004
Karpinski, A. C., Kirschner, P. A., Ozer, I., Mellott, J. A., & Ochwo, P. (2013). An exploration of social networking site use, multitasking, and academic performance among United States and European university students. Computers in Human Behavior, 29, 1182–1192. https://doi.org/10.1016/j.chb.2012.10.011
Kirschner, P. A., & Karpinski, A. C. (2010). Facebook® and academic performance. Computers in Human Behavior, 26, 1237–1245. https://doi.org/10.1016/j.chb.2010.03.024 Koc, M., & Gulyagci, S. (2013). Facebook addiction among Turkish college students: The role of psychological health, demographic, and usage characteristics. Cyberpsychology, Behavior, and Social Networking, 16, 279–284. https://doi.org/10.1089/cyber.2012.0249
Kuss, D. J., & Griffiths, M. D. (2011). Online social networking and addiction: A review of the psychological literature. International Journal of Environmental Research and Public Health, 8, 3528–3552. https://doi.org/10.3390/ijerph8093528
Lane, J., Lane, A. M., & Kyprianou, A. (2004). Self-efficacy, self-esteem and their impact on academic performance. Social Behavior and Personality, 32, 247–256. https://doi.org/10.2224/sbp.2004.32.3.247
LaRose, R., Lin, C. A., & Eastin, M. S. (2003). Unregulated Internet usage: Addiction, habit, or deficient self- regulation? Media Psychology, 5, 225–253. https://doi.org/10.1207 /S1532785XMEP0503_01
Lau, W. W. (2017). Effects of social media usage and social media multitasking on the academic performance of university students. Computers in Human Behavior, 68, 286–291. https://doi.org/10.1016/j.chb.2016.11.043
Lent, R. W., Brown, S. D., & Larkin, K. C. (1986). Self-efficacy in the prediction of academic performance and perceived career options. Journal of Counseling Psychology, 33, 265–269. https://doi.org/10.1037/0022- 0167.33.3.265
Li, H., & Kam, W. B. (2002). Assessing psychological well-being of college student: Psychometric properties of GHQ-20. Psychological Development and Education, 1, 75–79. https://doi.org/10.16187/j.cnki.issn1001- 4918.2002.01.016
Lin, C. Y., Broström, A., Nilsen, P., Griffiths, M. D., & Pakpour, A. H. (2017). Psychometric validation of the Persian Bergen Social Media Addiction Scale using classic test theory and Rasch models. Journal of Behavioral Addictions, 6, 620–629. https://doi.org/10.1556 /2006.6.2017.071
Lin, L. Y., Sidani, J. E., Shensa, A., Radovic, A., Miller, E., Colditz, J. B., & Primack, B. A. (2016). Association between social media use and depression among US young adults. Depression and Anxiety, 33, 323–331. https://doi.org/10.1002/da.22466
Liu, M., & Peng, W. (2009). Cognitive and psychological predictors of the negative outcomes associated with playing MMOGs (massively multiplayer online games). Computers in Human Behavior, 25, 1306–1311. https://doi.org/10.1016/j.chb.2009.06.002
Macan, T. H., Shahani, C., Dipboye, R. L., & Phillips, A. P. (1990). College students’ time management: Correlations with academic performance and stress. Journal of Educational Psychology, 82, 760–768. https://doi.org/10.1037/0022-0663.82.4.760
Malik, S., & Khan, M. (2015). Impact of Facebook addiction on narcissistic behavior and self-esteem among students. Journal of Pakistan Medical Association, 65, 260–263.
Marino, C., Finos, L., Vieno, A., Lenzi, M., & Spada, M. M. (2017). Objective Facebook behaviour: Differences between problematic and non-problematic users. Computers in Human Behavior, 73, 541–546. https://doi.org/10.1016/j.chb.2017.04.015
Marino, C., Gini, G., Vieno, A., & Spada, M. M. (2018). A comprehensive meta-analysis on problematic Facebook use. Computers in Human Behavior, 83, 262–277. https://doi.org/10.1016 /j.chb.2018.02.009
Mehdizadeh, S. (2010). Self-presentation 2.0: Narcissism and self-esteem on Facebook. Cyberpsychology, Behavior, and Social Networking, 13, 357–364. https://doi.org/10.1089 /cyber.2009.0257 Monacis, L., De Palo, V., Griffiths, M. D., & Sinatra, M. (2017). Social networking addiction, attachment style, and validation of the Italian version of the Bergen Social Media Addiction Scale. Journal of Behavioral Addictions, 6, 178–186. https://doi.org/10.1556 /2006.6.2017.023
Nida, T. K. (2018). Facebook addiction and its association with academic performance. Biomedical Journal of Scientific & Technical Research, 3(5), 1–3. https://doi.org/10.26717 /BJSTR.2018.03.000950
Ophir, E., Nass, C., & Wagner, A. D. (2009). Cognitive control in media multitaskers. Proceedings of the National Academy of Sciences, 106, 15583–15587. https://doi.org/10.1073 /pnas.0903620106
Orth, U., & Robins, R. W. (2013). Understanding the link between low self-esteem and depression. Current Directions in Psychological Science, 22, 455–460. https://doi.org/10.1177 /0963721413492763
Orth, U., Robins, R. W., & Roberts, B. W. (2008). Low self-esteem prospectively predicts depression in adolescence and young adulthood. Journal of Personality and Social Psychology, 95, 695-708. https://doi.org/10.1037/0022- 3514.95.3.695
Oulasvirta, A., & Saariluoma, P. (2006). Surviving task interruptions: Investigating the implications of long-term working memory theory. International Journal of Human-Computer Studies, 64, 941–961. https://doi.org/10.1016/j.ijhcs.2006.04.006
Pantic, I. (2014). Online social networking and mental health. Cyberpsychology, Behavior, and Social Networking, 17, 652–657. https://doi.org/10.1089/cyber.2014.0070
Pantic, I., Damjanovic, A., Todorovic, J., Topalovic, D., Bojovic-Jovic, D., Ristic, S., & Pantic, S. (2012). Association between online social networking and depression in high school students: Behavioral physiology viewpoint. Psychiatria Danubina, 24, 90–93. https://doi.org/10.1002/mpr.1348
Pasek, J., & Hargittai, E. (2009). Facebook and academic performance: Reconciling a media sensation with data. First Monday, 14. https://doi.org/10.5210/fm.v14i5.2498
Preacher, K. J., & Hayes, A. F. (2008). Asymptotic and resampling strategies for assessing and comparing indirect effects in multiple mediator models. Behavior Research Methods, 40, 879–891. https://doi.org/10.3758/BRM.40.3.879
Raskauskas, J., Rubiano, S., Offen, I., & Wayland, A. K. (2015). Do social self-efficacy and self-esteem moderate the relationship between peer victimization and academic performance? Social Psychology of Education, 18, 297–314. https://doi.org/10.1007/s11218-015-9292-z
Rosenberg, M. (1965). Rosenberg self-esteem scale (RSE). Acceptance and commitment therapy. Measures Package, 61, 61-62.
Rosenberg, J., & Egbert, N. (2011). Online impression management: Personality traits and concerns for secondary goals as predictors of self-presentation tactics on Facebook. Journal of Computer-Mediated Communication, 17, 1– 18. https://doi.org/10.1111/j.1083-6101.2011.01560.x
Shakya, H. B., & Christakis, N. A. (2017). Association of Facebook use with compromised well-being: A longitudinal study. American Journal of Epidemiology, 185, 203–211. https://doi.org/10.1093/aje/kww189
Shensa, A., Escobar-Viera, C. G., Sidani, J. E., Bowman, N. D., Marshal, M. P., & Primack, B. A. (2017). Problematic social media use and depressive symptoms among US young adults: A nationally-representative study. Social Science & Medicine, 182, 150–157. https://doi.org/10.1016/j.socscimed.2017.03.061
Smith, A., & Anderson, M. (2018). Social media use in 2018. Retrieved from http://www.pewinternet.org/2018/03/01/social-media-use-in-2018/ Sowislo, J. F., & Orth, U. (2013). Does low self-esteem predict depression and anxiety? A meta-analysis of longitudinal studies. Psychological Bulletin, 139, 213–240. https://doi.org/10.1037/a0028931
Spada, M. M., Langston, B., Nikčević, A. V., & Moneta, G. B. (2008). The role of meta-cognitions in problematic internet use. Computers in Human Behavior, 24, 2325–2335. https://doi.org/10.1016/j.chb.2007.12.002
Starcevic, V. (2013). Is Internet addiction a useful concept? Australian and New Zealand Journal of Psychiatry, 47, 16–19. https://doi.org/10.1177/0004867412461693
Stone, C. B., & Wang, Q. (2018). From conversations to digital communication: The mnemonic consequences of consuming and sharing information via social media. Topics in Cognitive Science, 2018, 1-20. https://doi.org/10.1111/tops.12369
Tang, J. H., Chen, M. C., Yang, C. Y., Chung, T. Y., & Lee, Y. A. (2016). Personality traits, interpersonal relationships, online social support, and Facebook addiction. Telematics and Informatics, 33, 102–108. https://doi.org/10.1016/j.tele.2015.06.003
Toker, S., & Baturay, M. H. (2016). Antecedents and consequences of game addiction. Computers in Human Behavior, 55, 668–679. https://doi.org/10.1016/j.chb.2015.10.002
Turel, O., Serenko, A., & Giles, P. (2011). Integrating technology addiction and use: An empirical investigation of online auction users. Mis Quarterly, 35, 1043–1062. https://doi.org/10.2307/41409972
Uysal, R., Satici, S. A., & Akin, A. (2013). Mediating effect of Facebook addiction on the relationship between subjective vitality and subjective happiness. Psychological Reports, 113, 948–953. https://doi.org/10.2466/02.09.18.PR0.113x32z3
Valkenburg, P. M., Peter, J., & Schouten, A. P. (2006). Friend networking sites and their relationship to adolescents’ well-being and social self-esteem. CyberPsychology & Behavior, 9, 584–590. https://doi.org/10.1089/cpb.2006.9.584
van Rooij, A. J., Ferguson, C. J., van de Mheen, D., & Schoenmakers, T. M. (2017). Time to abandon Internet addiction? Predicting problematic Internet, game, and social media use from psychosocial well-being and application use. Clinical Neuropsychiatry, 14, 113–121.
Vogel, E. A., Rose, J. P., Roberts, L. R., & Eckles, K. (2014). Social comparison, social media, and self- esteem. Psychology of Popular Media Culture, 3, 206–222. https://doi.org/10.1037/ppm0000047
Wang, Q. (2013). The autobiographical self in time and culture. New York, NY: Oxford University Press.
Wen, Z., Hou, J., & Zhang, L. (2005). A comparison of moderator and mediator and their applications. Acta Psychologica Sinica, 37, 268–274. https://doi.org/0439-755X(2005)37:2<268:TJXYYZ>2.0.TX;2-M
Wolniczak, I., Caceres-DelAguila, J. A., Palma-Ardiles, G., Arroyo, K. J., Solís-Visscher, R., Paredes-Yauri, S., & Bernabe-Ortiz, A. (2013). Association between Facebook dependence and poor sleep quality: A study in a sample of undergraduate students in Peru. PLoS One, 8, e59087. https://doi.org/10.1371/journal.pone.0059087
Wood, E., Zivcakova, L., Gentile, P., Archer, K., De Pasquale, D., & Nosko, A. (2011). Examining the impact of distracting multitasking with technology on real-time classroom learning. Computers & Education, 58, 365–374. https://doi.org/10.1016/j.compedu.2011.08.029
Wu, A. M., Cheung, V. I., Ku, L., & Hung, E. P. (2013). Psychological risk factors of addiction to social networking sites among Chinese smartphone users. Journal of Behavioral Addictions, 2, 160–166. https://doi.org/10.1556/JBA.2.2013.006 Yam, C. W., Pakpour, A. H., Griffiths, M. D., Yau, W. Y., Lo, C. L. M., Ng, J. M., . . . Leung, H. (2018). Psychometric testing of three Chinese online-related addictive behavior instruments among Hong Kong university students. Psychiatric Quarterly, 2018, 1–12. https://doi.org/10.1007/s11126-018-9610-7
Young, K. S. (1999). Internet addiction: Symptoms, evaluation and treatment. In L. VandeCreek & T. L. Jackson (Eds.), Innovations in clinical practice: A source book (Vol 17, pp. 19–31). Sarasota, FL: Professional Resource Press.
Young, K. S. (2007). Cognitive behavior therapy with Internet addicts: Treatment outcomes and implications. CyberPsychology & Behavior, 10, 671–679. https://doi.org/10.1089/cpb.2007.9971
Zaremohzzabieh, Z., Samah, B. A., Omar, S. Z., Bolong, J., & Kamarudin, N. A. (2014). Addictive Facebook use among university students. Asian Social Science, 10, 107. https://doi.org/10.5539/ass.v10n6p107
Zywica, J., & Danowski, J. (2008). The faces of Facebookers: Investigating social enhancement and social compensation hypotheses: Predicting Facebook™ and offline popularity from sociability and self-esteem, and mapping the meanings of popularity with semantic networks. Journal of Computer-Mediated Communication, 14, 1–34. https://doi.org/10.1111/j.1083-6101.2008.01429.x
Introduction
Human beings have fundamental needs to belong and to relate, for which interpersonal communication is the key (Baumeiste<tar, 1995; Wang, 2013). In recent decades, with the development of information technology, especially with the rapid proliferation of Internet-based social media (e.g., Facebook, WeChat, or Instagram), the ways of interpersonal communication have drastically changed (Smith & Anderson, 2018; Stone, & Wang, 2018). The ubiquitous social media platforms and the easy access to the Internet bring about the potential for social media addiction, namely, the irrational and excessive use of social media to the extent that it interferes with other aspects of daily life (Griffiths, 2000, 2012). Social media addiction has been found to be associated with a host of emotional, relational, health, and performance problems (e.g., Echeburua & de Corral, 2010; Kuss & Griffiths, 2011; Marino, Finos, Vieno, Lenzi, & Spada, 2017; Marino, Gini, Vieno, & Spada, 2018). Understanding the causes, consequences, and remedies of social media addiction is thus of paramount importance. In the current research, we examined the relations of social media addiction to college students' mental health and academic performance and the role of self-esteem as a mediator for the relations (Study 1). We further tested the effectiveness of an intervention in reducing social media addiction and its potential adverse outcomes (Study 2).
Social Media Addiction and the Negative Outcomes
Social media addiction can be viewed as one form of Internet addiction, where individuals exhibit a compulsion to use social media to excess (Griffiths, 2000; Starcevic, 2013). Individuals with social media addiction are often overly concerned about social media and are driven by an uncontrollable urge to log on to and use social media (Andreassen & Pallesen, 2014). Studies have shown that the symptoms of social media addiction can be manifested in mood, cognition, physical and emotional reactions, and interpersonal and psychological problems (Balakrishnan & Shamim, 2013; Błachnio, Przepiorka, Senol-Durak, Durak, & Sherstyuk, 2017; Kuss & Griffiths, 2011; Tang, Chen, Yang, Chung, & Lee, 2016; Zaremohzzabieh, Samah, Omar, Bolong, & Kamarudin, 2014). It has been reported that social media addiction affects approximately 12% of users across social networking sites (Alabi, 2012; Wolniczak et al., 2013; Wu, Cheung, Ku, & Hung, 2013).
Many studies on social media usage and mental health have shown that the prolonged use of social media such as Facebook is positively associated with mental health problems such as stress, anxiety, and depression and negatively associated with long-term well-being (Eraslan-Capan, 2015; Hong, Huang, Lin & Chiu, 2014; Malik & Khan, 2015; Marino et al., 2017; Pantic, 2014; Shakya & Christakis, 2017; Toker & Baturay, 2016). For example, the time spent on social media was positively related to depressive symptoms among high school students in Central Serbia (Pantic, Damjanovic, Todorovic, et al., 2012) and among young adults in the United States (Lin et al., 2016). Furthermore, certain categories of social media use have been shown to be associated with reduced academic performance (Al-Menayes, 2014, 2015; Junco, 2012; Kirschner & Karpinski, 2010; Junco, 2012; Karpinski, Kirschner, Ozer, Mellott, & Ochwo, 2013; Al-Menayes, 2014, 2015). For example, Lau (2017) found whereas using social media for academic purposes did not predict academic performance indexed by the cumulative grade point average, using social media for nonacademic purposes (video gaming in particular) and social media multitasking negatively predicted academic performance. A large sample (N = 1893) survey conducted in the United States also found that the time students spent on Facebook was negatively associated with their total GPAs (Junco, 2012). Laboratory experiments have provided further evidence for the negative relation between social media use and academic outcomes. For example, Wood et al. (2012) found that multi-tasking via texting, email, MSN, and Facebook had negative effects on real-time learning performance. Jiang, Hou, and Wang (2016) found that the use of Weibo, the Chinese equivalence of Twitter, had negative effects on information comprehension.
Importantly, frequent social media usage does not necessarily indicate social media addiction (Griffiths, 2010) and therefore does not always have negative implications for individuals’ mental health (e.g., Jelenchick, Eickhoff, & Moreno, 2013) or academic performance (Pasek & Hargittai, 2009). A key distinction between normal over-engagement in social media that may be occasionally experienced by many and social media addiction is that the latter is associated with unfavorable consequences when online social networking becomes uncontrollable and compulsive (Andreassen, 2015). Studies investigating social media addiction have mainly focused on Facebook addiction (e.g., Andreassen et al., 2012; Koc & Gulyagci, 2013; Hong et al., 2014). It has been shown that addiction to Facebook is positively associated with depression, anxiety, and insomnia (Bányai et al., 2017; Koc & Gulyagci, 2013; Shensa et al., 2017; Van Rooij, Ferguson, Van de Mheen, & Schoenmakers, 2017) and negatively associated with subjective well-being, subjective vigor, and life satisfaction (Błachnio, Przepiorka, & Pantic, 2016; Hawi & Samaha, 2017; Uysal, Satici, & Akin, 2013). Research has also suggested the negative impact of social media addiction, and Facebook addiction in particular, on academic performance (Huang, 2014; Nida, 2017).
The Role of Self-Esteem
One factor that may underlie the negative effects of social media addiction is self-esteem. Although viewing or editing one's own online profile enhances self-esteem, according to the Hyperpersonal Model (Amy & Hancock, 2010), social media users are frequently exposed to others’ selective and glorified online self-presentations, which can, in turn, reduce the viewers’ self-esteem (Rosenberg & Egbert, 2011). For example, frequent Facebook users believe that others are happier and more successful than themselves, especially when they do not know well the other users offline (Chou & Edge, 2012). Vogel, Rose, Roberts, & Eckles (2014) suggest that the extent of upward social comparisons on Facebook is greater than the extent of downward social comparisons and that upward social comparisons on social media may diminish self-esteem. Empirical studies have provided support to this proposal. For example, a study by Mehdizadeh (2010) showed that the use of Facebook was correlated with reduced self-esteem, such that individuals who spent a greater amount of time on Facebook per session and who made a greater number of Facebook logins per day had lower self-esteem. Another study found that adolescents’ self-esteem was lowered after receiving negative feedback on social media (Valkenburg, Peter, & Schouten, 2006). Moreover, recent studies have revealed a negative relation between addictive use of social media and self-esteem (e.g., Andreassen et al., 2017; Błachnio, et al., 2016).
A considerable number of studies have shown that low self-esteem is associated with many psychological dysfunctions such as depression and anxiety (e.g., Orth, Robins, & Roberts, 2008; Orth & Robins, 2013; Sowislo & Orth, 2013). Self-esteem has also been shown to be positively associated with academic performance (e.g., Lane, Lane, & Kyprianou, 2004; Lent, Brown, & Larkin, 1986) and further serve as a protective factor against adversities in aiding academic and emotional resilience (Raskauskas, Rubiano, Offen, & Wayland, 2015). It is possible that social media addiction contributes to lower self-esteem, which, in turn, leads to a decrease in mental health and academic performance. In other words, self-esteem may play a mediating role in the relations of social media addiction to mental health and academic performance.
The Present Study
To further examine the relations of social media addictions to individuals’ mental health and academic performance, we conducted two studies. In Study 1, we investigated the relations of social media addictions to mental health and academic performance in college students and examined the role of self-esteem as a potential mediator for the relations. A survey method was used in which participants reported their addiction to social media, as well as their mental health, academic performance, and self-esteem. Built on the findings of Study 1, we designed an experimental intervention in Study 2 to reduce social media addiction and further promote college students' mental health and academic performance.
In both studies, we used the Bergen Social Media Addiction Scale (BSMAS; Andreassen et al., 2017) to measure social media addiction. Based on the general addiction theory, Andreassen and colleagues (2012) first developed the Bergen Facebook Addiction Scale (BFAS), with six items each describing one dimension of addictive behavior (i.e., salience, mood modification, tolerance, withdrawal symptoms, conflict, and relapse). The scale has good psychometric properties, and the addiction can be scored using a polythetic scoring scheme (i.e., scoring 3 or above on at least four of the six items) or a monothetic scoring scheme (i.e., scoring 3 or above on all six items) (Andreassen et al., 2012). One critique of BFAS is that it is specific to Facebook addiction and thus may not be appropriate for examining addiction to online social networking more generally (Griffiths, 2012). Andreassen and colleagues (2017) later revised BFAS into BSMAS, replacing "Facebook" with "social media." It has been shown to have excellent reliability (Cronbach's alpha = .88) for measuring social media addiction. In addition, BSMAS has been used with non-English populations such as Iranian, Italian, and Hong Kong samples and demonstrated robust psychometric properties (Lin, Broström, Nilsen, Griffiths, & Pakpour, 2017; Monacis, De Palo, Griffiths, & Sinatra, 2017; Yam et al., 2018).
Based on the findings of previous studies (e.g., Jiang et al., 2016; Koc & Gulyagci, 2013; Pantic et al., 2012; Rosen et al., 2011; Valkenburg et al., 2006), we hypothesized that social media addiction would be negatively associated with college students’ mental health and academic performance, and that these relations would be mediated by the students’ self-esteem. We further expected that an intervention to reduce social media addiction would alleviate its negative associations with mental health and academic performance.
Study 1 utilized a survey method to investigate the relations of social media addiction to mental health and academic performance in college students and to examine the role of self-esteem as a potential mediator for the relations.
Participants. The participants were undergraduate students recruited through a social psychology course at Peking University, China. A total of 250 students who enrolled in the course participated in the study for one course credit. Among the students, 18 did not complete the questionnaires and were excluded. The final sample thus included 232 participants (117 males, 115 females; Mean age = 19.18 years, SD age = 1.32).
Procedure and Materials. Participants each completed a set of questionnaires in class. They were told that the questionnaires were unrelated to each other and that they should carefully answer all questions.
Social media addiction. The 6-item Bergen Social Media Addiction Scale (BSMAS; Andreassen et al., 2017) was used to measure the participants’ addictive use of social media. The items concern experiences occurring over the past year and are rated on 5-point scales ranging from 1 (Very rarely) to 5 (Very often) (e.g., “ How often during the last year have you felt an urge to use social media more and more?” ). Given the characteristics of social networking sites in mainland China, we replaced the examples of social media sites in the original scale, namely “Facebook, Twitter, Instagram and the like,” with those popular in China, "QQ, Weibo, WeChat and the like” in the instruction. A bilingual researcher translated the scale into Chinese, which was then back translated into English by another researcher. The original English version was compared with the back-translated version to resolve any discrepancies between them. The Cronbach's alpha of the Chinese version in the current sample was 0.81. Participants’ ratings were summed across the 6 items to form a social media addiction score, with higher scores indicating greater social media addiction.
Mental health . Mental health was measured by a 20-item questionnaire adapted by Li and Kam (2002) from the 30-item General Health Questionnaire (GHQ-30; Goldberg, 1972). This questionnaire includes three sub-scales: depression ( Cronbach's α = .65), anxiety ( Cronbach's α = .73), and sense of adequacy ( Cronbach's α = .63). Participants were asked to answer “Yes” or “No” about their feelings in recent weeks (e.g., “I feel that being alive has no meaning,” “I feel unsettled or nervous all day long ,” and “I go happily through daily life” ). The scores for depression and anxiety were reverse-coded. Scores of the three sub-scales were then summed ( Cronbach's α = .80), with higher scores indicating better mental health.
Academic performance. Given that the participants came from diverse majors and different classes, their academic performance was measured by self-reported ranking relative to their respective peers. Participants were asked to rank their academic performance relative to their peers in the past semester as 1) 20% or below; 2) 20 - 40%; 3) 40 - 60%; 4) 60 - 80%; or 5) 80 - 100%.
Self-esteem. The 10-item Chinese version of the Self-esteem Scale ( Cronbach's α = .82; Ji & Yu, 1993) adapted from Rosenberg (1965) was used to measure self-esteem (e.g., “ I feel that I have a number of good qualities ”). Participants answered the questions on 4-point scales ranging from 1 (strongly disagree) to 4 (strongly agree). Higher scores indicated higher levels of self-esteem.
At last, participants were asked to report demographic information including age, gender, only child or non-only child status, and urban or rural residence, and they were fully debriefed and thanked.
Results and Discussion
In the current sample, 41.4% of the participants scored 3 or above on at least four of the six items (the polythetic scoring scheme of BSMAS), and 9.9% scored 3 or above on all six items (the monothetic scoring scheme of BSMAS; Andreassen et al., 2012). Also, 14.7% of the participants could be classified as having social media addiction, whose composite score was above 18 and who scored 3 or above on at least four of the six items. This percentage was close to what was previously reported (12%) in a Chinese sample (Wu et al., 2013). Participants who were only children had poorer academic performance, t (194) = 2.71, p = .007, d = .44, higher levels of self-esteem, t (228) = 2.44, p = .02, d = .38, and lower social media addiction scores, t (228) = -2.58, p = .01, d = -.40, than did those with siblings. Participants who came from cities had higher levels of self-esteem, t (214) = 2.87, p = .005, d = .57, than did those from rural areas. Gender and age were not significantly correlated with any variables.
Following previous studies (Andreassen et al., 2012, 2017; Koc & Gulyagci, 2013; Hong et al., 2014), we treated the social media addiction score as a continuous variable to examine the degree of additive use of social media in relation to mental health and academic performance. Table1 presents the means and standard deviations (SDs) of key variables and the correlations among them. Social media addiction was negatively correlated with mental health, whereby the higher one scored on social media addiction, the poorer mental health he or she had. Social media addiction was also negatively correlated with academic performance as well as self-esteem. Self-esteem, on the other hand, was positively related to mental health. Mental health and academic performance were also positively correlated.
Table 1: Means, SDs and Correlations among Study Variables.
|
|
|
|
|
|
1 Social media addiction | 14.77 | 4.13 |
|
|
|
2 Self-esteem | 29.10 | 4.19 | -.23*** |
|
|
3 Mental health | 14.28 | 3.91 | -.29*** | .55** |
|
4 Academic performance | 3.26 | 1.15 | -.16* | .13 | .20* |
* < .05, ** < .01, *** < .001 |
We further conducted partial correlation analyses among the key variables, controlling for demographic variables (i.e., age, gender, only child status, and residence). The pattern of results remained identical. The partial correlations between social media addiction and mental health, academic performance, and self-esteem remained significant, r s(232) = -.29 ( p <.001), -.15 ( p = .048), and -.20 ( p = .007), respectively. Self-esteem and mental health were also significantly correlated, r (232) = .55, p <.001, so were mental health and academic performance, r (232) = .20, p = .007.
Because self-esteem was not correlated with academic performance, the mediation effect was not tested further for academic performance. To test whether self-esteem played a mediating role in the relations of social media addiction to mental health, we conducted three steps of regression analyses (Wen, Hou, & Zhang, 2005). In the first step, we regressed mental health on demographic variables and social media addiction. Social media addiction uniquely predicted mental health, β = - .29, t (210) = -4.28, p < .001. In the second step, we regressed self-esteem on demographic variables and social media addiction. Social media addiction uniquely predicted self-esteem. β = - .19, t (210) = -2.75, p = .007. In the third step, demographic variables were entered in the first layer, social media addiction was entered in the second layer, and self-esteem was entered in the third layer to predict mental health. After self-esteem was entered, the size of the standard regression coefficient of social media addiction decreased from -.29 to -.19, t (209) = -3.26, p = .001, △R 2 = .26, p < .001. Thus, the relation between social media addiction and mental health was at least partially mediated by self-esteem. The mediating effect of self-esteem is shown in Figure 1.
Figure 1. Mediating effect of self-esteem (Study 1). ** p < .01, p *** < .001.

To corroborate the findings, we further tested the mediating effect of self-esteem using a bootstrapping analysis with 5,000 iterations (Preacher & Hayes, 2008). The 95% confidence interval was [-.1807, -.0215], excluding 0, which indicates that the mediating effect of self-esteem was significant. To explore an alternative pathway, we tested the mediating effect of self-esteem with social media addiction as the dependent variable and mental health as the independent variable. The 95% confidence interval was [-.1422, .0844], including 0, indicating that the reverse mediating effect of self-esteem was not significant. Thus, the results support our hypothesis that social media addiction was associated with reduced mental health through lowering individuals’ self-esteem.
Results from Study 1 confirmed our hypotheses that social media addiction was negatively associated with mental health, consistent with findings from previous studies (e.g., Koc & Gulyagci, 2013). Furthermore, as expected, we found that self-esteem played a mediating role in the relation between social media addiction and mental health, and that the reverse mediating effect was not significant. These findings suggest that the negative association between social media addiction and mental health is at least partially accounted for by reduced self-esteem.
In addition, results from Study 1 also confirmed our prediction that social media addiction was negatively related to academic performance, although the relation was not strong. On the other hand, self-esteem was not significantly associated with academic performance, which differed from previous studies (Lane et al., 2004; Lent et al., 1986; Raskauskas et al., 2015). This might be because there was only one self-report item to measure academic performance, which could be vulnerable to the influence of social desirability concerns. In addition, given that we did not measure the time participants spent on social media, it is unclear how social media use may differ from social media addiction in relation to mental health and academic performance. We addressed these limitations in Study 2.
Study 1 showed that the addictive use of social media was common among college students and that it was negatively associated with mental health and academic performance. One important follow-up question is whether social media addiction can be reduced and thus its negative associations with health and academic outcomes be alleviated. No study that we know of has considered intervention options for social media addiction. We therefore designed an intervention program for social media addiction based on Young's (1999) recommendations for the treatment of Internet addiction, and we conducted an experiment to verify its effectiveness.
To design an intervention program for social media addiction, we referred to previous studies on Internet addiction interventions. Research has shown that metacognitive beliefs about one’s thinking and self-regulation influence problematic Internet use and social media addiction (Casale, Rugai, & Fioravanti, 2018; Caselli et al., 2018; Spada, Langston, Nikĉević, & Moneta, 2008). According to the cognitive-behavioral model, cognitive distortions such as the ruminative cognitive style are the primary cause of excessive Internet use (Davis, 2001). These cognitive distortions can be automatically activated whenever there is a stimulus associated with the Internet. A vicious cycle of cognitive distortions and reinforcement then results in negative outcomes. This model has been widely used in addiction research related to pathological Internet overuse (Larose, Lin, & Eastin, 2003; Liu & Peng, 2009; Turel, Serenko, & Giles, 2011). A number of cognitive-behavioral therapy techniques have been recommended for treating Internet addiction (Young, 2007; Gupta, Arora, & Gupta, 2013). Based on this literature, we believe that the cognitive-behavioral approach will be a helpful way to mitigate the negative associations of social media addiction with health and academic outcomes. It will help individuals with social media addiction to recognize their cognitive distortions and further guide them to reconstruct their thinking and behavior.
In Study 2, we combined cognitive reconstruction, reminder cards, and the diary technique (Young, 1999) into a novel intervention program and designed a 2 by 2 mixed-model experiment to test its effectiveness. In line with findings of previous studies on Internet addiction (e.g., Gupta et al., 2013; Turel et al., 2011; Young, 2007), we predicted that compared with a control group, the experimental group who experienced the intervention would show reduced social media addiction and improved outcomes in mental health and academic efficiency. We included measures of multiple outcome variables to achieve more reliable results, including daily social media use time, self-esteem, sleep quality, mental health, emotional state, learning time, and learning engagement.
Participants. Study 2 was conducted at Peking University, China. Participants who exhibited social media addiction were preselected from a pool of 242 undergraduate students who enrolled in a social psychology course (a different pool from Study 1). The students were asked to complete the 6-item BSMAS (Andreassen et al.,2017). Among them, 43 students scored higher than 18 on the composite score and also scored 3 or above on at least four of the six items. These students were selected to participate in Study 2. They were randomly assigned to either an experimental or a control group and were tested both before (Time 1) and after the intervention (Time 2). The study was thus a 2 x 2 mixed-model design. The 21 participants in the experimental group completed all aspects of the intervention and both tests. Five of the 22 participants in the control group dropped out before the completion. Hence, the final sample included 38 participants (18 males, 18 females, two unreported; M age = 19.71, SD age =1.43).
Procedures and Measures. The intervention program was approved by the Research Ethics Committee of the School of Psychological and Cognitive Sciences at Peking University. Prior to the intervention at Time 1, all participants were informed that the purpose of this study was to investigate social media addiction and they were asked to provide informed consent. Participants then completed a survey, which included the measures of social media addiction, self-esteem, and mental health, same as in Study 1. In addition, participants were asked to report their daily social media use time, indicating the number of hours they spent on social media per day. Participants also reported their sleep quality, rating on a 5-point scale ranging from 1 (very bad) to 5 (very good).
Participants in the experimental group then participated in a one-week intervention program, while those in the control group did not receive any instruction during this time. The intervention included two stages. The first stage involved cognitive reconstruction and took approximately 30 minutes (Young, 1999). Participants visited the lab, where they were asked to reflect on their social media use from five respects: How much time they spent on social media per day and per week? What other meaningful things they could do with that time? What were the benefits of not using social media? Why did they use social media and were there alternative way to achieve the purposes? What were the adverse effects of social media use? Participants wrote down their responses. After the reflection, participants were asked to each list on a card five advantages of reducing the use of social media and five disadvantages of excessive use of social media. They were then asked to take a photo of the card and use it as a lock screen of their phones that would serve as a reminder for themselves. They were also instructed to post the card on their desks during the following week.
The second stage of the intervention took place in the following week, during which participants in the experimental group were asked to keep a daily to record their thoughts, emotions, and behaviors related to social media use, as part of the cognitive-behavioral techniques (Young, 1999). Participants reflected on their daily use of social media every night before going to bed, including what social media they used, how long and how they used the social media, their thoughts and emotions related to their social media use, and the strategies they would like to use to reduce social media use. They were also asked to indicate their emotional state and learning engagement, as well as their expected social media use the next day. To ensure that the participants followed the instruction, daily reminders were sent to them to complete the recording. Participants were further instructed to take a photo of their completed recording and send it to a contact researcher of the lab to confirm its completion. The participants’ responses in the daily reflection task were part of the intervention and were not used in analysis.
After the intervention, at Time 2, all participants completed another survey. The measures included social media addiction, daily social media use time, self-esteem, sleep quality, and mental health, same as those at Time 1. In addition, the participants’ learning engagement in the past week was measured by the 17-item Utrecht Work Engagement Scale-Student (UWES-S, Fang, Shi, & Zhang, 2008). Participants answered the questions (e.g., “My study inspires me ”) on 5-point scales ranging from 1 (strongly disagree) to 5 (strongly agree) ( Cronbach's α = 0.93 for the current sample). A total score was summed, with higher scores indicating higher levels of learning engagement. Participants also reported their daily learning time outside the class in the past week and rated on their emotional state in the past week on a scale ranging from 1 (very bad) to 100 (very good).
Finally, participants in the experimental group provided feedback on the effectiveness of the intervention. They answered 7 questions concerning the various aspects of the intervention (e.g., “ Generally, I think the intervention is effective” ) on 5-point scales from 1 (strongly disagree) to 5 (strongly agree) ( Cronbach's α = 0.81). At last, participants were fully debriefed and thanked.
Across all dependent variables, 2 (Group: Experimental vs. Control) x 2 (Test time: Time 1 vs. Time 2) mixed-model analyses were conducted to examine the effect of intervention. First, the analysis on social media addiction score revealed main effects of group, F (1, 36) = 7.89, p = .008, η p 2 = .18, and test time, F (1, 36) = 33.74, p < .001, η p 2 = .48, qualified by a significant interaction, F (1, 36) = 17.92, p < .001, η p 2 = .33. For participants in the experimental group, there was a significant decrease in social media addiction from Time 1 to Time 2 , changing from 20.62 (higher than 18) to 14.62 (lower than 18), t (20) = 7.17, p < .001, d = 1.97. In contrast, for participants in the control group, there was no significant change in their social media addiction, t (16) = 1.13, p = .28, d = .35. Figure 2 illustrates the interaction effect.
Figure 2. Social media addiction as a function of test time and group (Study 2).

The same analysis was conducted to examine the effect of intervention on daily social media use time, self-esteem, sleep quality, and mental health, respectively. Table 2 presents means and standard deviations for all variables and t-tests within each group. For daily social media use time, there was a main effect of test time, F (1, 36) = 26.54, p < .001, η p 2 = .42, qualified by a Group x Test time interaction, F (1, 36) = 10.47, p = .003, η p 2 = .23. Further t-tests within each group showed that whereas the average daily time participants spent on social media was reduced significantly from Time 1 to Time 2 for both groups, the reduction was larger for the experimental group. There was only a main effect of test time for self-esteem, F (1, 36) = 12.67, p = .001, η p 2 = .26, and sleep quality, F (1, 36) = 9.10, p = .005, η p 2 = .20, whereby self-esteem and sleep quality increased from Time 1 to Time 2. However, further t-tests within each group showed that the improvements were only significant for the experimental group, but not the control group. For mental health, a significant Group x Test time interaction emerged, F (1, 36) = 5.69, p = .02, η p 2 = .14. Whereas mental health scores increased from Time 1 to Time 2 for the experimental group, t (20) = 2.55, p = .02, d =.59, there was no change for the control group, t (16) = -.86, p = .40, d =-.19. Taken together, these results suggest that our intervention effectively reduced social media addiction and improved mental health and other outcomes.
Further analyses of the remaining outcome variables at Time 2 showed that compared with the control group, participants in the experimental group exhibited better learning engagement, t (36) = .2.31, p = .03, d =.77, spent more time on their study outside the class, t (36)= 2.28, p = .03, d = .75, and experienced a better emotional state, t (36) = 2.74, p = .01, d =.86, during the intervention period. In addition, participants in the experimental group reported that the intervention was effective: all participants rated over 3 for the overall intervention; 81% rated over 3 for the first stage of the intervention and 90% for the second. All participants reported that the daily reflections were helpful, and 86% of them were willing to continue to participate in similar studies.
Table 2. Mean and standard deviation of Time 1andTime2's test scores of key variables.
Outcome variables | Group(n) | Time 1 | Time 2 | t | p | |||
M | SD | M | SD | |||||
Social media addiction | Experimental(21) | 20.62 | 2.16 | 14.62 | 3.72 | 7.17 | <.001*** | |
Control(17) | 20.12 | 2.15 | 19.18 | 3.07 | 1.13 | .275 | ||
Daily social media use time | Experimental(21) | 4.65 | 2.67 | 1.56 | .98 | 5.09 | <.001*** | |
Control(17) | 3.85 | 2.31 | 3.15 | 1.48 | 2.16 | .046* | ||
Self-esteem | Experimental(21) | 28.67 | 3.68 | 30.67 | 3.17 | -3.87 | .001** | |
Control(17) | 27.41 | 3.18 | 28.35 | 3.81 | -1.42 | .174 | ||
Sleep quality | Experimental(21) | 3.38 | .86 | 3.95 | .86 | -3.51 | .002** | |
Control(17) | 3.35 | 1.00 | 3.59 | .80 | -1.07 | .299 | ||
Mental health | Experimental(21) | 13.24 | 4.45 | 15.71 | 3.89 | -2.55 | .019* | |
Control(17) | 13.18 | 4.07 | 12.35 | 4.58 | .86 | .403 |
In sum, participants in the experimental group exhibited reduced social media addiction and improved mental health as well as self-esteem and sleep quality after a two-stage intervention, whereas there was no significant change in the control group. The experimental group participants evaluated the intervention to be effective, in line with prior research showing that cognitive reconstruction, the reminder card technique, and daily reflections are effective methods in reducing Internet addiction (Young, 1999). Furthermore, compared with those in the control group, participants who received the intervention spent more time on learning and experienced a higher level of learning engagement and better emotional state. It is noteworthy that although control group participants reported reduced social media use time at Time 2, they did not exhibit reduced social media addiction or significant improvement in any outcome measures. This is consistent with the theoretical notion that the mere social media use time is not equivalent with or sufficient to index social media addiction (Griffiths, 2010; Andreassen, 2015). Together, these findings suggest that our intervention was effective in reducing social media addiction and improving college students’ mental health and learning efficiency.
General discussion
The current studies provided empirical support that social media addiction was negatively associated with college students’ mental health and academic performance (Pantic et al., 2012; Jelenchick et al., 2013). Furthermore, in line with previous findings that social media addiction negatively affects self-esteem (Andreassen et al., 2017; Błachnio, et al., 2016; Chou & Edge, 2012; Vogel et al., 2014) and that low self-esteem is associated with mental disorders (Orth et al., 2008; Orth & Robins, 2013; Sowislo & Orth, 2013), the current research yielded the first empirical finding that self-esteem mediated the relation of social media addiction to mental health. Furthermore, the implementation of an intervention based on the cognitive-behavioral approach (Young, 1999, 2007; Gupta et al., 2013) effectively reduced social media addiction and improved mental health and academic efficiency.
Notably, our results showed that social media addiction was associated with reduced mental health partly through lowering individuals’ self-esteem, and that the reverse mediating effect of self-esteem with mental health as the predictor and social media addiction as the outcome variable was not significant. Nevertheless, it does not rule out the possibility that poor mental health can further contribute to social media addiction. Individuals in poor mental health, including those with low self-worth, may use social media as a compensation for their real-life interpersonal deficiency and further develop excessive dependence on social media (Zywica & Danowski, 2008). Also, individuals in poor mental health often try to use social media to improve their mood and, when this need is not met, their mental condition tends to become worse (Caplan, 2010). Thus, the relation between poor mental health and social media addiction is likely to be bidirectional.
The present studies provided strong support for the relation of social media addiction to academic outcomes by using a variety of measures. Study 1 showed that a self-rank measure of academic performance was negatively associated with social media addiction. This relation was not mediated by self-esteem. Study 2 further showed that an intervention to reduce social media addiction improved learning engagement and increased the time spent on learning outside the class. We speculate that there may be three explanations for the negative relation of social media addiction to academic performance. First, social media addiction may mean more time spent online and less time spent on study. Excessive social media use interrupts students’ time management, which further affects academic performance (Macan et al., 1990). Second, social media addiction may interfere with students’ work by distracting them and making them unable to stay focused. Research has shown that multitasking has negative effects on the performance of specific tasks (Ophir, Nass, & Wagner, 2009). Finally, given that students with social media addiction may be easily distracted, it can be difficult for them to encode and remember what they are learning (Oulasvirta & Saariluoma, 2006).
Our intervention program effectively reduced social media addiction and improved students’ mental health and learning efficiency. This has important practical implications by showing that social media addiction can be mitigated through cognitive reconstruction and the supporting techniques. The stage of cognitive reconstruction helped students realize the negative consequences of their addiction to social media as well as the potential benefits of reducing social media usage. The subsequent application of the reminder card as a lock screen of their phones as well as the daily reflections further reinforced this awareness. These findings suggest that helping college students to gain a better understanding of the adverse effects of social media addiction through cost-efficient self-help interventions can reduce social media addiction and have the potential to improve mental health and academic performance.
The current studies have some limitations. First, participants were recruited through psychology courses at Peking University and the sample sizes were relatively small especially in Study 2, which may limit the generalizability of the findings. Future studies should include more diverse and larger samples to increase external validity. Second, participants in the control group of Study 2 did not receive any instruction during the one-week interval and they could be distracted by things unrelated to the study. Future research should establish more strict control conditions to eliminate any confounding variables. Third, the intervention in Study 2 was limited in length and the post-treatment data were collected only once, right after the intervention ended. It is therefore unclear whether the intervention effects on social media addiction and other outcomes would persist over time. Given that the current intervention program for reducing social media addiction was newly developed, it requires further refinement to improve its effectiveness. In addition, future studies should investigate the bidirectional relation between social media addiction and mental health, using longitudinal approaches to further validate the mediating role of self-esteem and examine other potential mediators such as cognitive distortions for the relations of social media addiction to mental health and other outcomes.
In conclusion, the current research revealed negative associations between social media addiction and college students' mental health and academic performance, and the role of self-esteem as an underlying mechanism for the relation between social media addiction and mental health. A cost-efficient intervention that included cognitive reconstruction, reminder cards, and a week-long diary keeping effectively reduced the addiction to social media and further improved mental health and academic efficiency.

Crossref Cited-by

This work is licensed under a Creative Commons Attribution-NonCommercial-NoDerivatives 4.0 International License .
Copyright © 2019 Yubo Hou, Dan Xiong, Tonglin Jiang, Lily Song, Qi Wang

An official website of the United States government
The .gov means it’s official. Federal government websites often end in .gov or .mil. Before sharing sensitive information, make sure you’re on a federal government site.
The site is secure. The https:// ensures that you are connecting to the official website and that any information you provide is encrypted and transmitted securely.
- Publications
- Account settings
The PMC website is updating on October 15, 2024. Learn More or Try it out now .
- Advanced Search
- Journal List
- Front Psychol
Social Media Use and Mental Health and Well-Being Among Adolescents – A Scoping Review
Viktor schønning.
1 Department of Health Promotion, Norwegian Institute of Public Health, Bergen, Norway
Gunnhild Johnsen Hjetland
Leif edvard aarø, jens christoffer skogen.
2 Alcohol and Drug Research Western Norway, Stavanger University Hospital, Stavanger, Norway
3 Faculty of Health Sciences, University of Stavanger, Stavanger, Norway

Associated Data
Introduction: Social media has become an integrated part of daily life, with an estimated 3 billion social media users worldwide. Adolescents and young adults are the most active users of social media. Research on social media has grown rapidly, with the potential association of social media use and mental health and well-being becoming a polarized and much-studied subject. The current body of knowledge on this theme is complex and difficult-to-follow. The current paper presents a scoping review of the published literature in the research field of social media use and its association with mental health and well-being among adolescents.
Methods and Analysis: First, relevant databases were searched for eligible studies with a vast range of relevant search terms for social media use and mental health and well-being over the past five years. Identified studies were screened thoroughly and included or excluded based on prior established criteria. Data from the included studies were extracted and summarized according to the previously published study protocol.
Results: Among the 79 studies that met our inclusion criteria, the vast majority (94%) were quantitative, with a cross-sectional design (57%) being the most common study design. Several studies focused on different aspects of mental health, with depression (29%) being the most studied aspect. Almost half of the included studies focused on use of non-specified social network sites (43%). Of specified social media, Facebook (39%) was the most studied social network site. The most used approach to measuring social media use was frequency and duration (56%). Participants of both genders were included in most studies (92%) but seldom examined as an explanatory variable. 77% of the included studies had social media use as the independent variable.
Conclusion: The findings from the current scoping review revealed that about 3/4 of the included studies focused on social media and some aspect of pathology. Focus on the potential association between social media use and positive outcomes seems to be rarer in the current literature. Amongst the included studies, few separated between different forms of (inter)actions on social media, which are likely to be differentially associated with mental health and well-being outcomes.
In just a few decades, the use of social media have permeated most areas of our society. For adolescents, social media play a particularly large part in their lives as indicated by their extensive use of several different social media platforms ( Ofcom, 2018 ). Furthermore, the use of social media and types of platforms offered have increased at such a speed that there is reason to believe that scientific knowledge about social media in relation to adolescents’ health and well-being is scattered and incomplete ( Orben, 2020 ). Nevertheless, research findings indicating the potential negative effects of social media on mental health and well-being are frequently reported in traditional media (newspapers, radio, TV) ( Bell et al., 2015 ). Within the scientific community, however, there are ongoing debates regarding the impact and relevance of social media in relation to mental health and well-being. For instance, Twenge and Campbell (2019) stated that use of digital technology and social media have a negative impact on well-being, while Orben and Przybylski (2019) argued that the association between digital technology use and adolescent well-being is so small that it is more or less inconsequential. Research on social media use is a new focus area, and it is therefore important to get an overview of the studies performed to date, and describe the subject matter studies have investigated in relation to the effect of social media use on adolescents mental health and well-being. Also, research gaps in this emerging research field is important to highlight as it may guide future research in new and meritorious directions. A scoping review is therefore deemed necessary to provide a foundation for further research, which in time will provide a knowledge base for policymaking and service delivery.
This scoping review will help provide an overall understanding of the main foci of research within the field of social media and mental health and well-being among adolescents, as well as the type of data sources and research instruments used so far. Furthermore, we aim to highlight potential gaps in the research literature ( Arksey and O’Malley, 2005 ). Even though a large number of studies on social media use and mental health with different vantage points has been conducted over the last decade, we are not aware of any broad-sweeping scoping review covering this area.
This scoping review aims to give an overview of the main research questions that have been focused on with regard to use of social media among adolescents in relation to mental health and well-being. Both quantitative and qualitative studies are of interest. Three specific secondary research questions will be addressed and together with the main research question serve as a template for organizing the results:
- • Which aspects of mental health and well-being have been the focus or foci of research so far?
- • Has the research focused on different research aims across gender, ethnicity, socio-economic status, geographic location? What kind of findings are reported across these groups?
- • Organize and describe the main sources of evidence related to social media that have been used in the studies identified.
Defining Adolescence and Social Media
In the present review, adolescents are defined as those between 13 and 19 years of age. We chose the mean age of 13 as our lower limit as nearly all social media services require users to be at least 13 years of age to access and use their services ( Childnet International, 2018 ). All pertinent studies which present results relevant for this age range is within the scope of this review. For social media we used the following definition by Kietzmann et al. (2011 , p. 1): “Social media employ mobile and web-based technologies to create highly interactive platforms via which individuals and communities share, co-create, discuss, and modify user-generated content.” We also employed the typology described by Kaplan and Haenlein’s classification scheme across two axes: level of self-presentation and social presence/media richness ( Kaplan and Haenlein, 2010 ). The current scoping review adheres to guidelines and recommendations stated by Tricco et al. (2018) .
See protocol for further details about the definitions used ( Schønning et al., 2020 ).
Data Sources and Search Strategy
A literature search was performed in OVID Medline, OVID Embase, OVID PsycINFO, Sociological Abstracts (proquest), Social Services Abstracts (proquest), ERIC (proquest), and CINAHL. The search strategy combined search terms for adolescents, social media and mental health or wellbeing. The database-controlled vocabulary was used for searching subject headings, and a large spectrum of synonyms with appropriate truncations was used for searching title, abstract, and author keywords. A filter for observational studies was applied to limit the results. The search was also limited to publications from 2014 to current. The search strategy was translated between each database. An example of full strategy for Embase is attached as Supplementary Material .
Study Selection: Exclusion and Inclusion Criteria
The exclusion and inclusion criteria are detailed in the protocol ( Schønning et al., 2020 ). Briefly, we included English language peer-reviewed quantitative- or qualitative papers or systematic reviews published within the last 5 years with an explicit focus on mental health/well-being and social media. Non-empirical studies, intervention studies, clinical studies and publications not peer-reviewed were excluded. Intervention studies and clinical studies were excluded as we sought to not introduce too much heterogeneity in design and our focus was on observational studies. The criteria used for study selection was part of an iterative process which was described in detail in the protocol ( Schønning et al., 2020 ). As per the study protocol ( Schønning et al., 2020 ), and in line with scoping review guidelines ( Peters et al., 2015 , 2017 ; Tricco et al., 2018 ), we did not assess methodological quality or risk of bias of the included studies.
The selection process is illustrated by a flow-chart indicating the stages from unsorted search results to the number of included studies (see Figure 1 ). Study selection was accomplished and organized using the Rayyan QCRI software 1 . The inclusion and exclusion process was performed independently by VS and JCS. The interrater agreement was κ = 0.87, indicating satisfactory agreement.

Flowchart of exclusion process from unsorted results to included studies.
Data Extraction and Organization
Details of the data extracted is described in the protocol. Three types of information were extracted, bibliographic information, information about study design and subject matter information. Subject matter information included aim of study, how social media and mental health/well-being was measured, and main findings of the study.
Visualization of Words From the Titles of the Included Studies
The most frequently occurring words and bigrams in the titles of the included studies are presented in Figures 2 , ,3. 3 . The following procedure was used to generate Figure 1 : First, a text file containing all titles were imported into R as a data frame ( R Core Team, 2014 ). The data frame was processed using the “tidy text”-package with required additional packages ( Silge and Robinson, 2016 ). Second, numbers and commonly used words with little inherent meaning (so called “stop words,” such as “and,” “of,” and “in”), were removed from the data frame using the three available lexicons in the “tidy-text”-package ( Silge and Robinson, 2016 ). Furthermore, variations of “adolescents” (e.g., “adolescent,” “adolescence,” and “adolescents”) and “social media” (e.g., “social media,” “social networking,” “online social networks”) were removed from the data frame. Third, the resulting data frame was sorted based on frequency of unique words, and words occurring only once were removed. The final data frame is presented as a word cloud in Figure 1 ( N = 113). The same procedure as described above was employed to generate commonly occurring bigrams (two words occurring adjacent to each other), but without removing bigrams occurring only once ( N = 231). The word clouds were generated using the “wordcloud2”-package in R ( Lang and Chien, 2018 ). For Figure 1 , shades of blue indicate word frequencies >2 and green a frequency of 2. For Figure 2 , shades of blue indicate bigram frequencies of >1 and green a frequency of 1.

Word cloud from the titles of the included studies. Most frequent words, excluding variations of “adolescence” and “social media.” N = 113. Shades of blue indicate word frequencies >2 and green a frequency of 2. The size of each word is indicative of its relative frequency of occurrence.

Word cloud from the titles of the included studies. Bigrams from the titles of the included studies, excluding variations of “adolescence” and “social media.” N = 231. Shades of blue indicate bigram frequencies of >1 and green a frequency of 1. The size of each bigram is indicative of its relative frequency of occurrence.
Characteristics of the Included Studies
Of 7927 unique studies, 79 (1%) met our inclusion criteria ( Aboujaoude et al., 2015 ; Banjanin et al., 2015 ; Banyai et al., 2017 ; Barry et al., 2017 ; Best et al., 2014 , 2015 ; Booker et al., 2018 ; Bourgeois et al., 2014 ; Boyle et al., 2016 ; Brunborg et al., 2017 ; Burnette et al., 2017 ; Colder Carras et al., 2017 ; Critchlow et al., 2019 ; Cross et al., 2015 ; Curtis et al., 2018 ; de Lenne et al., 2018 ; de Vries et al., 2016 ; Erfani and Abedin, 2018 ; Erreygers et al., 2018 ; Fahy et al., 2016 ; Ferguson et al., 2014 ; Fisher et al., 2016 ; Foerster and Roosli, 2017 ; Foody et al., 2017 ; Fredrick and Demaray, 2018 ; Frison and Eggermont, 2016 , 2017 ; Geusens and Beullens, 2017 , 2018 ; Hamm et al., 2015 ; Hanprathet et al., 2015 ; Harbard et al., 2016 ; Hase et al., 2015 ; Holfeld and Mishna, 2019 ; Houghton et al., 2018 ; Jafarpour et al., 2017 ; John et al., 2018 ; Kim et al., 2019 ; Kim, 2017 ; Koo et al., 2015 ; Lai et al., 2018 ; Larm et al., 2017 , 2019 ; Marchant et al., 2017 ; Marengo et al., 2018 ; Marques et al., 2018 ; Meier and Gray, 2014 ; Memon et al., 2018 ; Merelle et al., 2017 ; Neira and Barber, 2014 ; Nesi et al., 2017a , b ; Niu et al., 2018 ; Nursalam et al., 2018 ; Oberst et al., 2017 ; O’Connor et al., 2014 ; O’Reilly et al., 2018 ; Przybylski and Bowes, 2017 ; Przybylski and Weinstein, 2017 ; Richards et al., 2015 ; Rousseau et al., 2017 ; Salmela-Aro et al., 2017 ; Sampasa-Kanyinga and Chaput, 2016 ; Sampasa-Kanyinga and Lewis, 2015 ; Sampasa-Kanyinga et al., 2018 ; Scott and Woods, 2018 ; Settanni et al., 2018 ; Spears et al., 2015 ; Throuvala et al., 2019 ; Tiggemann and Slater, 2017 ; Tseng and Yang, 2015 ; Twenge and Campbell, 2019 ; Twenge et al., 2018 ; van den Eijnden et al., 2018 ; Wang et al., 2018 ; Wartberg et al., 2018 ; Wolke et al., 2017 ; Woods and Scott, 2016 ; Yan et al., 2017 ). Among the included studies, 74 (94%) are quantitative ( Aboujaoude et al., 2015 ; Banjanin et al., 2015 ; Banyai et al., 2017 ; Barry et al., 2017 ; Best et al., 2014 ; Booker et al., 2018 ; Bourgeois et al., 2014 ; Boyle et al., 2016 ; Brunborg et al., 2017 ; Colder Carras et al., 2017 ; Critchlow et al., 2019 ; Cross et al., 2015 ; Curtis et al., 2018 ; de Lenne et al., 2018 ; de Vries et al., 2016 ; Erfani and Abedin, 2018 ; Erreygers et al., 2018 ; Fahy et al., 2016 ; Ferguson et al., 2014 ; Fisher et al., 2016 ; Foerster and Roosli, 2017 ; Foody et al., 2017 ; Fredrick and Demaray, 2018 ; Frison and Eggermont, 2016 , 2017 ; Geusens and Beullens, 2017 , 2018 ; Hamm et al., 2015 ; Hanprathet et al., 2015 ; Harbard et al., 2016 ; Hase et al., 2015 ; Houghton et al., 2018 ; Jafarpour et al., 2017 ; John et al., 2018 ; Kim et al., 2019 ; Kim, 2017 ; Koo et al., 2015 ; Lai et al., 2018 ; Larm et al., 2017 , 2019 ; Marchant et al., 2017 ; Marengo et al., 2018 ; Marques et al., 2018 ; Meier and Gray, 2014 ; Memon et al., 2018 ; Merelle et al., 2017 ; Neira and Barber, 2014 ; Nesi et al., 2017a , b ; Niu et al., 2018 ; Nursalam et al., 2018 ; Oberst et al., 2017 ; O’Connor et al., 2014 ; Przybylski and Bowes, 2017 ; Przybylski and Weinstein, 2017 ; Richards et al., 2015 ; Rousseau et al., 2017 ; Salmela-Aro et al., 2017 ; Sampasa-Kanyinga and Chaput, 2016 ; Sampasa-Kanyinga and Lewis, 2015 ; Sampasa-Kanyinga et al., 2018 ; Scott and Woods, 2018 ; Settanni et al., 2018 ; Spears et al., 2015 ; Tiggemann and Slater, 2017 ; Tseng and Yang, 2015 ; Twenge and Campbell, 2019 ; Twenge et al., 2018 ; van den Eijnden et al., 2018 ; Wang et al., 2018 ; Wartberg et al., 2018 ; Wolke et al., 2017 ; Woods and Scott, 2016 ; Yan et al., 2017 ), three are qualitative ( O’Reilly et al., 2018 ; Burnette et al., 2017 ; Throuvala et al., 2019 ), and two use mixed methods ( Best et al., 2015 ; Holfeld and Mishna, 2019 ) (see Supplementary Tables 1 , 2 in the Supplementary Material for additional details extracted from all included studies). In relation to study design, 45 (57%) used a cross-sectional design ( Bourgeois et al., 2014 ; Ferguson et al., 2014 ; Meier and Gray, 2014 ; Neira and Barber, 2014 ; O’Connor et al., 2014 ; Banjanin et al., 2015 ; Hanprathet et al., 2015 ; Hase et al., 2015 ; Koo et al., 2015 ; Sampasa-Kanyinga and Lewis, 2015 ; Spears et al., 2015 ; Tseng and Yang, 2015 ; Frison and Eggermont, 2016 ; Sampasa-Kanyinga and Chaput, 2016 ; Woods and Scott, 2016 ; Banyai et al., 2017 ; Barry et al., 2017 ; Brunborg et al., 2017 ; Colder Carras et al., 2017 ; Larm et al., 2017 , 2019 ; Merelle et al., 2017 ; Oberst et al., 2017 ; Przybylski and Bowes, 2017 ; Przybylski and Weinstein, 2017 ; Tiggemann and Slater, 2017 ; Wolke et al., 2017 ; Yan et al., 2017 ; de Lenne et al., 2018 ; Erreygers et al., 2018 ; Fredrick and Demaray, 2018 ; Geusens and Beullens, 2018 ; Lai et al., 2018 ; Marengo et al., 2018 ; Marques et al., 2018 ; Niu et al., 2018 ; Nursalam et al., 2018 ; Sampasa-Kanyinga et al., 2018 ; Scott and Woods, 2018 ; Settanni et al., 2018 ; Wang et al., 2018 ; Wartberg et al., 2018 ; Critchlow et al., 2019 ; Kim et al., 2019 ; Twenge and Campbell, 2019 ), 17 used a longitudinal design ( Cross et al., 2015 ; Boyle et al., 2016 ; de Vries et al., 2016 ; Fahy et al., 2016 ; Frison and Eggermont, 2016 ; Harbard et al., 2016 ; Foerster and Roosli, 2017 ; Geusens and Beullens, 2017 ; Kim, 2017 ; Nesi et al., 2017a , b ; Rousseau et al., 2017 ; Salmela-Aro et al., 2017 ; Booker et al., 2018 ; Houghton et al., 2018 ; van den Eijnden et al., 2018 ; Holfeld and Mishna, 2019 ), seven were systematic reviews ( Aboujaoude et al., 2015 ; Best et al., 2015 ; Fisher et al., 2016 ; Marchant et al., 2017 ; Erfani and Abedin, 2018 ; John et al., 2018 ; Memon et al., 2018 ), two were meta-analyses ( Foody et al., 2017 : Curtis et al., 2018 ), one was a causal-comparative study ( Jafarpour et al., 2017 ), one was a review article ( Richards et al., 2015 ), one used a time-lag design ( Twenge et al., 2018 ), one was a scoping review ( Hamm et al., 2015 ), three used a focus-group interview design ( Burnette et al., 2017 ; O’Reilly et al., 2018 ; Throuvala et al., 2019 ), and one study used a combined survey and focus-group design ( Best et al., 2014 ).
The most common study settings were schools [ N = 42 (54%)] ( Best et al., 2014 ; Bourgeois et al., 2014 ; Meier and Gray, 2014 ; Neira and Barber, 2014 ; O’Connor et al., 2014 ; Banjanin et al., 2015 ; Hanprathet et al., 2015 ; Hase et al., 2015 ; Sampasa-Kanyinga and Lewis, 2015 ; Frison and Eggermont, 2016 ; Sampasa-Kanyinga and Chaput, 2016 ; Woods and Scott, 2016 ; Banyai et al., 2017 ; Brunborg et al., 2017 ; Colder Carras et al., 2017 ; Foerster and Roosli, 2017 ; Geusens and Beullens, 2017 , 2018 ; Kim, 2017 ; Larm et al., 2017 , 2019 ; Merelle et al., 2017 ; Nesi et al., 2017a , b ; Przybylski and Bowes, 2017 ; Rousseau et al., 2017 ; Salmela-Aro et al., 2017 ; Tiggemann and Slater, 2017 ; de Lenne et al., 2018 ; Fredrick and Demaray, 2018 ; Houghton et al., 2018 ; Lai et al., 2018 ; Marengo et al., 2018 ; Niu et al., 2018 ; Nursalam et al., 2018 ; Sampasa-Kanyinga et al., 2018 ; Scott and Woods, 2018 ; Settanni et al., 2018 ; van den Eijnden et al., 2018 ; Wang et al., 2018 ; Holfeld and Mishna, 2019 ; Kim et al., 2019 ). Fourteen of the included studies were based on data from a home setting ( Cross et al., 2015 ; Koo et al., 2015 ; Spears et al., 2015 ; Boyle et al., 2016 ; de Vries et al., 2016 ; Harbard et al., 2016 ; Barry et al., 2017 ; Frison and Eggermont, 2017 ; Oberst et al., 2017 ; Yan et al., 2017 ; Booker et al., 2018 ; Marques et al., 2018 ; Wartberg et al., 2018 ; Critchlow et al., 2019 ). Eleven publications were reviews or meta-analyses and included primary studies from different settings ( Aboujaoude et al., 2015 ; Best et al., 2015 ; Hamm et al., 2015 ; Richards et al., 2015 ; Fisher et al., 2016 ; Foody et al., 2017 ; Marchant et al., 2017 ; Curtis et al., 2018 ; Erfani and Abedin, 2018 ; John et al., 2018 ; Memon et al., 2018 ). One study used both a home and school setting ( Erreygers et al., 2018 ), and 11 (14%) of the included studies did not mention the study setting for data collection ( Ferguson et al., 2014 ; Tseng and Yang, 2015 ; Fahy et al., 2016 ; Burnette et al., 2017 ; Jafarpour et al., 2017 ; Przybylski and Weinstein, 2017 ; Wolke et al., 2017 ; O’Reilly et al., 2018 ; Twenge et al., 2018 ; Throuvala et al., 2019 ; Twenge and Campbell, 2019 ).
Mental Health Foci of Included Studies
For a visual overview of the mental health foci of the included studies see Figures 2 , ,3. 3 . Most studies had a focus on different negative aspects of mental health, as evident from the frequently used terms in Figures 2 , ,3. 3 . The most studied aspect was depression, with 23 (29%) studies examining the relationship between social media use and depressive symptoms ( Ferguson et al., 2014 ; Neira and Barber, 2014 ; O’Connor et al., 2014 ; Banjanin et al., 2015 ; Richards et al., 2015 ; Spears et al., 2015 ; Tseng and Yang, 2015 ; Fahy et al., 2016 ; Frison and Eggermont, 2016 , 2017 ; Woods and Scott, 2016 ; Banyai et al., 2017 ; Brunborg et al., 2017 ; Colder Carras et al., 2017 ; Larm et al., 2017 ; Nesi et al., 2017a ; Salmela-Aro et al., 2017 ; Fredrick and Demaray, 2018 ; Houghton et al., 2018 ; Niu et al., 2018 ; Twenge et al., 2018 ; Wang et al., 2018 ; Wartberg et al., 2018 ). Twenty of the included studies focused on different aspects of good mental health, such as well-being, happiness, or quality of life ( Best et al., 2014 , 2015 ; Bourgeois et al., 2014 ; Ferguson et al., 2014 ; Cross et al., 2015 ; Koo et al., 2015 ; Richards et al., 2015 ; Spears et al., 2015 ; Fahy et al., 2016 ; Foerster and Roosli, 2017 ; Przybylski and Bowes, 2017 ; Przybylski and Weinstein, 2017 ; Yan et al., 2017 ; Booker et al., 2018 ; de Lenne et al., 2018 ; Erfani and Abedin, 2018 ; Erreygers et al., 2018 ; Lai et al., 2018 ; van den Eijnden et al., 2018 ; Twenge and Campbell, 2019 ). Nineteen studies had a more broad-stroke approach, and covered general mental health or psychiatric problems ( Aboujaoude et al., 2015 ; Hanprathet et al., 2015 ; Hase et al., 2015 ; Sampasa-Kanyinga and Lewis, 2015 ; Spears et al., 2015 ; Fisher et al., 2016 ; Barry et al., 2017 ; Jafarpour et al., 2017 ; Kim, 2017 ; Merelle et al., 2017 ; Oberst et al., 2017 ; Wolke et al., 2017 ; Marengo et al., 2018 ; Marques et al., 2018 ; Sampasa-Kanyinga et al., 2018 ; Holfeld and Mishna, 2019 ; Kim et al., 2019 ; Larm et al., 2019 ). Eight studies examined the link between social media use and body dissatisfaction and eating disorder symptoms ( Ferguson et al., 2014 ; Meier and Gray, 2014 ; de Vries et al., 2016 ; Burnette et al., 2017 ; Rousseau et al., 2017 ; Tiggemann and Slater, 2017 ; Marengo et al., 2018 ; Wartberg et al., 2018 ). Anxiety was the focus of seven studies ( O’Connor et al., 2014 ; Koo et al., 2015 ; Spears et al., 2015 ; Fahy et al., 2016 ; Woods and Scott, 2016 ; Colder Carras et al., 2017 ; Yan et al., 2017 ), and 13 studies included a focus on the relationship between alcohol use and social media use ( O’Connor et al., 2014 ; Boyle et al., 2016 ; Sampasa-Kanyinga and Chaput, 2016 ; Brunborg et al., 2017 ; Geusens and Beullens, 2017 , 2018 ; Larm et al., 2017 ; Merelle et al., 2017 ; Nesi et al., 2017b ; Curtis et al., 2018 ; Sampasa-Kanyinga et al., 2018 ; Critchlow et al., 2019 ; Kim et al., 2019 ). Seven studies examined the effect of social media use on sleep ( Harbard et al., 2016 ; Woods and Scott, 2016 ; Yan et al., 2017 ; Nursalam et al., 2018 ; Sampasa-Kanyinga et al., 2018 ; Scott and Woods, 2018 ; Larm et al., 2019 ). Five studies saw how drug use and social media use affected each other ( O’Connor et al., 2014 ; Merelle et al., 2017 ; Sampasa-Kanyinga et al., 2018 ; Kim et al., 2019 ; Larm et al., 2019 ). Self-harm and suicidal behavior was the focus of eleven studies ( O’Connor et al., 2014 ; Sampasa-Kanyinga and Lewis, 2015 ; Tseng and Yang, 2015 ; Kim, 2017 ; Marchant et al., 2017 ; Merelle et al., 2017 ; Fredrick and Demaray, 2018 ; John et al., 2018 ; Memon et al., 2018 ; Twenge et al., 2018 ; Kim et al., 2019 ). Other areas of focus other than the aforementioned are loneliness, self-esteem, fear of missing out and other non-pathological measures ( Neira and Barber, 2014 ; Banyai et al., 2017 ; Barry et al., 2017 ; Colder Carras et al., 2017 ).
Social Media Metrics of Included Studies
The studies included in the current scoping review often focus on specific, widely used, social media and social networking services, such as 31 (39%) studies focusing on Facebook ( Bourgeois et al., 2014 ; Meier and Gray, 2014 ; Banjanin et al., 2015 ; Cross et al., 2015 ; Hanprathet et al., 2015 ; Richards et al., 2015 ; Sampasa-Kanyinga and Lewis, 2015 ; Spears et al., 2015 ; Boyle et al., 2016 ; de Vries et al., 2016 ; Frison and Eggermont, 2016 ; Harbard et al., 2016 ; Sampasa-Kanyinga and Chaput, 2016 ; Banyai et al., 2017 ; Barry et al., 2017 ; Brunborg et al., 2017 ; Larm et al., 2017 ; Merelle et al., 2017 ; Nesi et al., 2017a , b ; Rousseau et al., 2017 ; Tiggemann and Slater, 2017 ; Booker et al., 2018 ; de Lenne et al., 2018 ; Lai et al., 2018 ; Marengo et al., 2018 ; Marques et al., 2018 ; Memon et al., 2018 ; Sampasa-Kanyinga et al., 2018 ; Settanni et al., 2018 ; Twenge et al., 2018 ), 11 on Instagram ( Sampasa-Kanyinga and Lewis, 2015 ; Boyle et al., 2016 ; Sampasa-Kanyinga and Chaput, 2016 ; Barry et al., 2017 ; Brunborg et al., 2017 ; Frison and Eggermont, 2017 ; Nesi et al., 2017a ; Marengo et al., 2018 ; Memon et al., 2018 ; Sampasa-Kanyinga et al., 2018 ), 11 including Twitter ( Richards et al., 2015 ; Sampasa-Kanyinga and Lewis, 2015 ; Spears et al., 2015 ; Harbard et al., 2016 ; Sampasa-Kanyinga and Chaput, 2016 ; Barry et al., 2017 ; Brunborg et al., 2017 ; Merelle et al., 2017 ; Nesi et al., 2017a ; Memon et al., 2018 ; Sampasa-Kanyinga et al., 2018 ), and five studies asking about Snapchat ( Boyle et al., 2016 ; Barry et al., 2017 ; Brunborg et al., 2017 ; Nesi et al., 2017a ; Marengo et al., 2018 ). Eight studies mentioned Myspace ( Richards et al., 2015 ; Sampasa-Kanyinga and Lewis, 2015 ; de Vries et al., 2016 ; Harbard et al., 2016 ; Sampasa-Kanyinga and Chaput, 2016 ; Larm et al., 2017 ; Booker et al., 2018 ; Sampasa-Kanyinga et al., 2018 ) and two asked about Tumblr ( Barry et al., 2017 ; Nesi et al., 2017a ). Other media such as Skype ( Merelle et al., 2017 ), Youtube ( Richards et al., 2015 ), WhatsApp ( Brunborg et al., 2017 ), Ping ( Merelle et al., 2017 ), Bebo ( Booker et al., 2018 ), Hyves ( de Vries et al., 2016 ), Kik ( Brunborg et al., 2017 ), Ask ( Brunborg et al., 2017 ), and Qzone ( Niu et al., 2018 ) were only included in one study each.
Almost half ( n = 34, 43%) of the included studies focus on use of social network sites or online communication in general, without specifying particular social media sites, leaving this up to the study participants to decide ( Best et al., 2014 , 2015 ; Ferguson et al., 2014 ; Neira and Barber, 2014 ; O’Connor et al., 2014 ; Koo et al., 2015 ; Tseng and Yang, 2015 ; Fahy et al., 2016 ; Woods and Scott, 2016 ; Burnette et al., 2017 ; Colder Carras et al., 2017 ; Foerster and Roosli, 2017 ; Foody et al., 2017 ; Geusens and Beullens, 2017 , 2018 ; Jafarpour et al., 2017 ; Kim, 2017 ; Marchant et al., 2017 ; Oberst et al., 2017 ; Przybylski and Weinstein, 2017 ; Salmela-Aro et al., 2017 ; Yan et al., 2017 ; Curtis et al., 2018 ; Erfani and Abedin, 2018 ; Erreygers et al., 2018 ; Nursalam et al., 2018 ; Scott and Woods, 2018 ; van den Eijnden et al., 2018 ; Wartberg et al., 2018 ; Critchlow et al., 2019 ; Holfeld and Mishna, 2019 ; Larm et al., 2019 ; Throuvala et al., 2019 ; Twenge and Campbell, 2019 ). Seven of the included studies examined the relationship between virtual game worlds or socially oriented video games and mental health ( Ferguson et al., 2014 ; Best et al., 2015 ; Spears et al., 2015 ; Yan et al., 2017 ; van den Eijnden et al., 2018 ; Larm et al., 2019 ; Twenge and Campbell, 2019 ).
In the 79 studies included in this scoping review, several approaches to measuring social media use are utilized. The combination of frequency and duration of social media use is by far the most used measurement of social media use, and 44 (56%) of the included studies collected data on these parameters ( Ferguson et al., 2014 ; Meier and Gray, 2014 ; Neira and Barber, 2014 ; Banjanin et al., 2015 ; Best et al., 2015 ; Hanprathet et al., 2015 ; Sampasa-Kanyinga and Lewis, 2015 ; Tseng and Yang, 2015 ; Boyle et al., 2016 ; de Vries et al., 2016 ; Frison and Eggermont, 2016 , 2017 ; Harbard et al., 2016 ; Sampasa-Kanyinga and Chaput, 2016 ; Woods and Scott, 2016 ; Banyai et al., 2017 ; Brunborg et al., 2017 ; Colder Carras et al., 2017 ; Foerster and Roosli, 2017 ; Jafarpour et al., 2017 ; Kim, 2017 ; Larm et al., 2017 , 2019 ; Merelle et al., 2017 ; Nesi et al., 2017b ; Oberst et al., 2017 ; Rousseau et al., 2017 ; Tiggemann and Slater, 2017 ; Yan et al., 2017 ; Booker et al., 2018 ; de Lenne et al., 2018 ; Erreygers et al., 2018 ; Houghton et al., 2018 ; Lai et al., 2018 ; Marengo et al., 2018 ; Marques et al., 2018 ; Niu et al., 2018 ; Nursalam et al., 2018 ; Sampasa-Kanyinga et al., 2018 ; Scott and Woods, 2018 ; Settanni et al., 2018 ; Twenge et al., 2018 ; van den Eijnden et al., 2018 ; Twenge and Campbell, 2019 ). Eight studies focused on the relationship between social media addiction or excessive use and mental health ( Banjanin et al., 2015 ; Tseng and Yang, 2015 ; Banyai et al., 2017 ; Merelle et al., 2017 ; Nursalam et al., 2018 ; Settanni et al., 2018 ; Wang et al., 2018 ). Bergen Social Media Addiction Scale is a commonly used questionnaire amongst the included studies ( Hanprathet et al., 2015 ; Banyai et al., 2017 ; Settanni et al., 2018 ). Seven studies asked about various specific actions on social media, such as liking or commenting on photos, posting something or participating in a discussion ( Meier and Gray, 2014 ; Koo et al., 2015 ; Nesi et al., 2017b ; Geusens and Beullens, 2018 ; Marques et al., 2018 ; van den Eijnden et al., 2018 ; Critchlow et al., 2019 ).
Five studies had a specific and sole focus on the link between social media use and alcohol, and examined how various alcohol-related social media use affected alcohol intake ( Boyle et al., 2016 ; Geusens and Beullens, 2017 , 2018 ; Nesi et al., 2017b ; Critchlow et al., 2019 ). Some studies had a more theory-based focus and investigated themes such as peer comparison, social media intrusion or pro-social behavior on social media and its effect on mental health ( Bourgeois et al., 2014 ; Rousseau et al., 2017 ; de Lenne et al., 2018 ). One of the included studies looked into night-time specific social media use ( Scott and Woods, 2018 ) and one looked into pre-bedtime social media behavior ( Harbard et al., 2016 ) to study the link between this use and sleep.
Amongst the 79 included studies, only six (8%) studies had participants of one gender ( Ferguson et al., 2014 ; Meier and Gray, 2014 ; Best et al., 2015 ; Burnette et al., 2017 ; Jafarpour et al., 2017 ; Tiggemann and Slater, 2017 ). Sixteen studies (20%) did not mention the gender distribution of the participants ( Aboujaoude et al., 2015 ; Best et al., 2015 ; Hamm et al., 2015 ; Richards et al., 2015 ; Fisher et al., 2016 ; Woods and Scott, 2016 ; Foody et al., 2017 ; Marchant et al., 2017 ; Przybylski and Weinstein, 2017 ; Curtis et al., 2018 ; Erfani and Abedin, 2018 ; John et al., 2018 ; Memon et al., 2018 ; O’Reilly et al., 2018 ; Twenge et al., 2018 ; Twenge and Campbell, 2019 ). Several of these were meta-analyses or reviews ( Aboujaoude et al., 2015 ; Best et al., 2014 ; Curtis et al., 2018 ; Foody et al., 2017 ; John et al., 2018 ; Erfani and Abedin, 2018 ; Wallaroo, 2020 ). The studies that included both genders as participants generally had a well-balanced gender distribution with no gender below 40% of the participants. Eight of the studies did not report gender-specific results ( Harbard et al., 2016 ; Nesi et al., 2017b ; Curtis et al., 2018 ; de Lenne et al., 2018 ; Niu et al., 2018 ; Nursalam et al., 2018 ; Wang et al., 2018 ; Twenge and Campbell, 2019 ). Of the included studies, gender was seldom examined as an explanatory variable, and other sociodemographic variables (e.g., ethnicity, socioeconomic status) were not included at all.
Implicit Causation Based on Direction of Association
Sixty-one (77%) of the included studies has social media use as the independent variable and some of the mentioned measurements of mental health as the dependent variable ( Aboujaoude et al., 2015 ; Banjanin et al., 2015 ; Banyai et al., 2017 ; Barry et al., 2017 ; Best et al., 2014 ; Booker et al., 2018 ; Bourgeois et al., 2014 ; Boyle et al., 2016 ; Brunborg et al., 2017 ; Colder Carras et al., 2017 ; Critchlow et al., 2019 ; Cross et al., 2015 ; Curtis et al., 2018 ; de Lenne et al., 2018 ; de Vries et al., 2016 ; Erfani and Abedin, 2018 ; Fahy et al., 2016 ; Fisher et al., 2016 ; Foerster and Roosli, 2017 ; Fredrick and Demaray, 2018 ; Frison and Eggermont, 2016 ; Geusens and Beullens, 2018 ; Hamm et al., 2015 ; Hanprathet et al., 2015 ; Harbard et al., 2016 ; Hase et al., 2015 ; Holfeld and Mishna, 2019 ; Jafarpour et al., 2017 ; John et al., 2018 ; Kim et al., 2019 ; Kim, 2017 ; Lai et al., 2018 ; Larm et al., 2017 , 2019 ; Marengo et al., 2018 ; Marques et al., 2018 ; Meier and Gray, 2014 ; Memon et al., 2018 ; Neira and Barber, 2014 ; Nesi et al., 2017b ; Niu et al., 2018 ; Nursalam et al., 2018 ; O’Connor et al., 2014 ; O’Reilly et al., 2018 ; Przybylski and Bowes, 2017 ; Przybylski and Weinstein, 2017 ; Richards et al., 2015 ; Sampasa-Kanyinga and Chaput, 2016 ; Sampasa-Kanyinga and Lewis, 2015 ; Sampasa-Kanyinga et al., 2018 ; Scott and Woods, 2018 ; Spears et al., 2015 ; Tseng and Yang, 2015 ; Twenge and Campbell, 2019 ; Twenge et al., 2018 ; van den Eijnden et al., 2018 ; Wang et al., 2018 ; Wartberg et al., 2018 ; Wolke et al., 2017 ; Woods and Scott, 2016 ; Yan et al., 2017 ). Most of the included studies hypothesize social media use pattern will affect youth mental health in certain ways. The majority of the included studies tend to find a correlation between more frequent social media use and poor well-being and/or mental health (see Supplementary Table 2 ). The strength of this correlation is however heterogeneous as social media use is measured substantially different across studies. Four (5%) of the included studies focus explicitly on how mental health can affect social media use ( Merelle et al., 2017 ; Nesi et al., 2017a ; Erreygers et al., 2018 ; Settanni et al., 2018 ). Fourteen studies included a mediating factor or focus on reciprocal relationships between social media use and mental health ( Ferguson et al., 2014 ; Koo et al., 2015 ; Tseng and Yang, 2015 ; Frison and Eggermont, 2017 ; Geusens and Beullens, 2017 ; Marchant et al., 2017 ; Oberst et al., 2017 ; Rousseau et al., 2017 ; Salmela-Aro et al., 2017 ; Tiggemann and Slater, 2017 ; Houghton et al., 2018 ; Marques et al., 2018 ; Niu et al., 2018 ; Wang et al., 2018 ). An example is a cross-sectional study by Ferguson et al. (2014) suggesting that exposure to social media contribute to later peer competition which was found to be a predictor of negative mental health outcomes such as eating disorder symptoms.
Cyberbullying as a Nexus
Thirteen of the 79 (17%) included studies investigated cyberbullying as the measurement of social media use ( Aboujaoude et al., 2015 ; Cross et al., 2015 ; Hamm et al., 2015 ; Hase et al., 2015 ; Spears et al., 2015 ; Fahy et al., 2016 ; Fisher et al., 2016 ; Foody et al., 2017 ; Przybylski and Bowes, 2017 ; Wolke et al., 2017 ; Fredrick and Demaray, 2018 ; John et al., 2018 ; Holfeld and Mishna, 2019 ). Most of the systematic reviews and meta-analyses included focused on cyberbullying. A cross-sectional study from 2017 suggests that cyberbullying has similar negative effects as direct or relational bullying, and that cyberbullying is “mainly a new tool to harm victims already bullied by traditional means” ( Wolke et al., 2017 ). A meta-analysis from 2016 concludes that “peer cybervictimization is indeed associated with a variety of internalizing and externalizing problems among adolescents” ( Fisher et al., 2016 ). A systematic review from 2018 concludes that both victims and perpetrators of cyberbullying are at greater risk of suicidal behavior compared with non-victims and non-perpetrators ( John et al., 2018 ).
Strengths and Limitations of Present Study
The main strength of this scoping review lies in the effort to give a broad overview of published research related to use of social media, and mental health and well-being among adolescents. Although a range of reviews on screen-based activities in general and mental health and well-being exist ( Dickson et al., 2018 ; Orben, 2020 ), they do not necessarily discern between social media use and other types of technology-based media. Also, some previous reviews tend to be more particular regarding mental health outcome ( Best et al., 2014 ; Seabrook et al., 2016 ; Orben, 2020 ), or do not focus on adolescents per se ( Seabrook et al., 2016 ). The main limitation is that, despite efforts to make the search strategy as comprehensive and inclusive as possible, we probably have not been able to identify all relevant studies – this is perhaps especially true when studies do include relevant information about social media and mental health/well-being, but this information is part of sub-group analyses or otherwise not the main aim of the studies. In a similar manner, related to qualitative studies, we do not know if our search strategy were as efficient in identifying studies of relevance if this was not the main theme or focus of the study. Despite this, we believe that we were able to strike a balance between specificity and sensitivity in our search strategy.
Description of Central Themes and Core Concepts
The findings from the present scoping review on social media use and mental health and well-being among adolescents revealed that the majority (about 3/4) of the included studies focused on social media and pathology. The core concepts identified are social media use and its statistical association with symptoms of depression, general psychiatric symptoms and other symptoms of psychopathology. Similar findings were made by Keles et al. (2020) in a systematic review from 2019. Focus on the potential association between social media use and positive outcomes seems to be rarer in the current literature, even though some studies focused on well-being which also includes positive aspects of mental health. Studies focusing on screen-based media in general and well-being is more prevalent than studies linking social media specifically with well-being ( Orben, 2020 ). The notion that excessive social media use is associated with poor mental health is well established within mainstream media. Our observation that this preconception seems to be the starting point for much research is not conducive to increased knowledge, but also alluded to elsewhere ( Coyne et al., 2020 ).
Why the Focus on Poor Mental Health/Pathology?
The relationship between social media and mental health is likely to be complex, and social media use can be beneficial for maintaining friendships and enriching social life ( Seabrook et al., 2016 ; Birkjær and Kaats, 2019 ; Coyne et al., 2020 ; Orben, 2020 ). This scoping review reveals that the majority of studies focusing on effects of social media use has a clearly stated focus on pathology and detrimental results of social media use. Mainstream media and the public discourse has contributed in creating a culture of fear around social media, with a focus on its negative elements ( Ahn, 2012 ; O’Reilly et al., 2018 ). It is difficult to pin-point why the one-sided focus on the negative effects of social media has been established within the research literature. But likely reasons are elements of “moral panic,” and reports of increases in mental health problems among adolescents in the same period that social media were introduced and became wide-spread ( Birkjær and Kaats, 2019 ). The phenomenon of moral panic typically resurges with the introduction and increasing use of new technologies, as happened with video games, TV, and radio ( Mueller, 2019 ).
The Metrics of Social Media
Social media trends change rapidly, and it is challenging for the research field to keep up. The included studies covered some of the most frequently used social media, but the amount of studies focusing on each social media did not accurately reflect the contemporary distribution of users. Even though sites such as Instagram and Snapchat were covered in some studies, the coverage did not do justice to the amount of users these sites had. Newer social media sites such as TikTok were not mentioned in the included studies even though it has several hundred million daily users ( Mediakix, 2019 ; Wallaroo, 2020 ).
Across the included studies there was some variation in how social media were gauged, but the majority of studies focused on the mere frequency and duration of use. There were little focus on separating between different forms of (inter)actions on social media, as these can vary between being a victim of cyberbullying to participating in healthy community work. Also, few studies differentiated between types of actions (i.e., posting, scrolling, reading), active and passive modes of social media use (i.e., production versus consumption, and level of interactivity), a finding similar to other reports ( Seabrook et al., 2016 ; Verduyn et al., 2017 ; Orben, 2020 ). There is reason to believe that different modes of use on social media platforms are differentially associated with mental health, and a recent narrative review highlight the need to address this in future research ( Orben, 2020 ). One of the included studies found for instance that it is not the total time spent on Facebook or the internet, but the specific amount of time allocated to photo-related activities that is associated with greater symptoms of eating disorders such as thin ideal internalization, self-objectification, weight dissatisfaction, and drive for thinness ( Meier and Gray, 2014 ). This observation can possibly be explained by social comparison mechanisms ( Appel et al., 2016 ) and passive use of social media ( Verduyn et al., 2017 ). The lack of research differentiating social media use and its association with mental health is an important finding of this scoping review and will hopefully contribute to this being included in future studies.
Few studies examined the motivation behind choosing to use social media, or the mental health status of the users when beginning a social media session. It has been reported that young people sometimes choose to enter sites such as Facebook and Twitter as an escape from threats to their mental health such as experiencing overwhelming pressure in daily life ( Boyd, 2014 ). This kind of escapism can be explained through uses and gratifications theory [see for instance ( Coyne et al., 2020 )]. On the other hand, more recent research suggest that additional motivational factors may include the need to control relationships, content, presentation, and impressions ( Throuvala et al., 2019 ), and it is possible that social media use can act as an reinforcement of adolescents’ current moods and motivations ( Birkjær and Kaats, 2019 ). Regardless, it seems obvious that the interplay between online and offline use and underlying motivational mechanisms needs to be better understood.
There has also been some questions about the accuracy when it comes to deciding the amount and frequency of one’s personal social media use. Without measuring duration and frequency of use directly and objectively it is unlikely that subjective self-report of general use is reliable ( Kobayashi and Boase, 2012 ; Scharkow, 2016 , 2019 ; Naab et al., 2019 ). Especially since the potential for social media use is almost omnipresent and the use itself is diverse in nature. Also, due to processes such as social desirability, it is likely that some participants report lower amounts of social media use as excessive use is seen largely undesirable ( Krumpal, 2013 ). Inaccurate reporting of prior social media use could also be a threat to the validity of the reported numbers and thus bias the results reported. Real-time tracking of actual use and modes of use is therefore recommended in future studies to ensure higher accuracy of these aspects of social media use ( Coyne et al., 2020 ; Orben, 2020 ), despite obvious legal and ethical challenges. Another aspect of social media use which does not seem to be addressed is potential spill-over effects, where use of social media leads to potential interest in or thinking about use of – and events or contents on – social media when the individual is offline. When this aspect has been addressed, it seems to be in relation to preoccupations and with a focus on excessive use or addictive behaviors ( Griffiths et al., 2014 ). Conversely, given the ubiquitous and important role of social media, experiences on social media – for better or for worse – are likely to be interconnected with the rest of an individual’s lived experience ( Birkjær and Kaats, 2019 ).
The Studies Seem to Implicitly Think That the Use of Social Media “Causes”/“Affects” Mental Health (Problems)
Most of the included studies establish an implicit causation between social media and mental health. It is assumed that social media use has an impact on mental health. The majority of studies included establish some correlation between more frequent use of social media and poor well-being/mental health, as evident from Supplementary Table 2 . As formerly mentioned, most of the included studies are cross-sectional and cannot shed light into temporality or cause-and-effect. In total, only 16 studies had a longitudinal design, using different types of regression models, latent growth curve models and cross-lagged models. Yet there seems to be an unspoken expectation that the direction of the association is social media use affecting mental health. The reason for this supposition is unclear, but again it is likely that the mainstream media discourse dominated by mostly negative stories and reports of social media use has some impact together with the observed moral panic.
With the increased popularity of social media and internet arrived a reduction of face-to-face contact and supposed increased social isolation ( Kraut et al., 1998 ; Espinoza and Juvonen, 2011 ). This view is described as the displacement hypothesis [see for instance ( Coyne et al., 2020 )]. Having a thriving social life and community with meaningful relations are for many considered vital for well-being and good mental health, and the supposed reduction of sociality were undoubtedly met with skepticism by some. Social media use has increased rapidly among young people over the last two decades along with reports that mental health problems are increasing. Several studies report that there is a rising prevalence of symptom of anxiety and depression among our adolescents ( Bor et al., 2014 ; Olfson et al., 2015 ). The observation that increases in social media use and mental health issues happened in more or less the same time period can have contributed to focus on how use of social media affects mental health problems.
The existence of an implicit causation is supported by the study variables chosen and the lack of positively worded outcomes. Depression, anxiety, alcohol use, psychiatric problems, suicidal behavior and eating disorders are amongst the most studied outcome-variables. On the other side of the spectrum we have well-being, which can oscillate from positive to negative, whilst the measures of pathology only vary from “ill” to “not ill” with positive outcomes not possible.
What Is the Gap in the Literature?
The current literature on social media and mental health among youth is still developing and has several gaps and shortcomings, as evident from this scoping review and other publications ( Seabrook et al., 2016 ; Coyne et al., 2020 ; Keles et al., 2020 ; Orben, 2020 ). Some of the gaps and shortcomings in the field we propose solutions for has been identified in a systematic review from 2019 by Keles et al. (2020) . The majority of the included studies in the current scoping review were cross-sectional, were limited in their inclusion of potential confounders and 3rd variables such as sociodemographics and personality, preventing knowledge about possible cause-and-effect between social media and mental health. There is a lack of longitudinal studies examining the effects of social media over extended periods of time, as well as investigations longitudinally of how mental health impacts social media use. However, since the formal search was ended for this scoping review, some innovative studies have emerged using longitudinal data ( Brunborg and Andreas, 2019 ; Orben et al., 2019 ; Coyne et al., 2020 ). More high quality longitudinal studies of social media use and mental health could help us identify the patterns over time and help us learn about possible cause-and-effect relationships, as well as disentangling between- and within-person associations ( Coyne et al., 2020 ; Orben, 2020 ). Furthermore, both social media use and mental health are complex phenomena in themselves, and future studies need to consider which aspects they want to investigate when trying to understand their relationship. Mechanisms linking social media use and eating disorders are for instance likely to be different than mechanisms linking social media use and symptoms of ADHD.
Our literature search also revealed a paucity of qualitative studies exploring the why’s and how’s of social media use in relation to mental health among adolescents. Few studies examine how youth themselves experience and perceive the relationship between social media and mental health, and the reasons for their continued and frequent use. Qualitatively oriented studies would contribute to a deeper understanding of adolescent’s social media sphere, and their thoughts about the relationship between social media use and mental health [see for instance ( Burnette et al., 2017 )]. For instance, O’Reilly et al. (2018) found that adolescents viewed social media as a threat to mental well-being, and concluded that they buy into the idea that “inherently social media has negative effects on mental wellbeing” and seem to “reify the moral panic that has become endemic to contemporary discourses.” On the other hand, Weinstein found using both quantitative and qualitative data that adolescents’ perceptions of the relationship between social media use and well-being probably is more nuanced, and mostly positive. Another clear gap in the research literature is the lack of focus on potentially positive aspects of social media use. It is obvious that there are some positive sides of the use of social media, and these also need to be investigated further ( Weinstein, 2018 ; Birkjær and Kaats, 2019 ). Gender-specific analyses are also lacking in the research literature, and there is reason to believe that social media use have different characteristics between the genders with different relationships to mental health. In fact, recent findings indicate that not only gender should be considered an important factor when investigating the role of social media in adolescents’ lives, but individual characteristics in general ( Orben et al., 2019 ; Orben, 2020 ). Analyses of socioeconomic status and geographic location are also lacking and it is likely that these factors might play a role the potential association between social media use and mental health. And finally, several studies point to the fact that social media potentially could be a fruitful arena for promoting mental well-being among youth, and developing mental health literacy to better equip our adolescents for the challenges that will surely arise ( O’Reilly et al., 2018 ; Teesson et al., 2020 ).
Research into the association between social media use and mental health and well-being among adolescents is rapidly emerging. The field is characterized by a focus on the association between social media use and negative aspects of mental health and well-being, and where studies focusing on the potentially positive aspects of social media use are lacking. Presently, the majority of studies in the field are quantitatively oriented, with most utilizing a cross-sectional design. An increase in qualitatively oriented studies would add to the field of research by increasing the understanding of adolescents’ social-media life and their own experiences of its association with mental health and well-being. More studies using a longitudinal design would contribute to examining the effects of social media over extended periods of time and help us learn about possible cause-and-effect relationships. Few studies look into individual factors, which may be important for our understanding of the association. Social media use and mental health and well-being are complex phenomena, and future studies could benefit from specifying the type of social media use they focus on when trying to understand its link to mental health. In conclusion, studies including more specific aspects of social media, individual differences and potential intermediate variables, and more studies using a longitudinal design are needed as the research field matures.
Author Contributions
JS conceptualized the review approach and provided general guidance to the research team. VS and JS drafted the first version of this manuscript. JS, GH, and LA developed the draft further based on feedback from the author group. All authors reviewed and approved the final version of the manuscript and have made substantive intellectual contributions to the development of this manuscript.
Conflict of Interest
The authors declare that the research was conducted in the absence of any commercial or financial relationships that could be construed as a potential conflict of interest.
Acknowledgments
We would like to thank Bergen municipality, Hordaland County Council and Western Norway University of Applied Sciences for their collaboration and help with the review. We would also like to thank Senior Librarian Marita Heinz at the Norwegian Institute for Public Health for vital help conducting the literature search.
Funding. This review was partly funded by Regional Research Funds in Norway, funding #RFF297031. No other specific funding was received for the present project. The present project is associated with a larger innovation-project lead by Bergen municipality in Western Norway related to the use of social media and mental health and well-being. The innovation-project is funded by a program initiated by the Norwegian Directorate of Health, and in Vestland county coordinated by the County Council (County Authority). The project aims to explore social media as platform for health-promotion among adolescents.
1 https://rayyan.qcri.org/welcome
Supplementary Material
The Supplementary Material for this article can be found online at: https://www.frontiersin.org/articles/10.3389/fpsyg.2020.01949/full#supplementary-material
- Aboujaoude E., Savage M. W., Starcevic V., Salame W. O. (2015). Cyberbullying: review of an old problem gone viral. J. Adolesc. Health 57 10–18. 10.1016/j.jadohealth.2015.04.011 [ PubMed ] [ CrossRef ] [ Google Scholar ]
- Ahn J. (2012). Teenagers’ experiences with social network sites: relationships to bridging and bonding social capital. Inform. Soc. 28 99–109. 10.1080/01972243.2011.649394 [ CrossRef ] [ Google Scholar ]
- Appel H., Gerlach A. L., Crusius J. (2016). The interplay between Facebook use, social comparison, envy, and depression. Curr. Opin. Psychol. 9 44–49. 10.1016/j.copsyc.2015.10.006 [ CrossRef ] [ Google Scholar ]
- Arksey H., O’Malley L. (2005). Scoping studies: towards a methodological framework. Int. J. Soc. Res. Methodol. 8 19–32. 10.1080/1364557032000119616 [ CrossRef ] [ Google Scholar ]
- Banjanin N., Banjanin N., Dimitrijevic I., Pantic I. (2015). Relationship between internet use and depression: focus on physiological mood oscillations, social networking and online addictive behavior. Comput. Hum. Behav. 43 308–312. 10.1016/j.chb.2014.11.013 [ CrossRef ] [ Google Scholar ]
- Banyai F., Zsila A., Kiraly O., Maraz A., Elekes Z., Griffiths M. D., et al. (2017). Problematic social media use: results from a large-scale nationally representative adolescent sample. PLoS One 12 : e0169839 . 10.1371/journal.pone.0169839 [ PMC free article ] [ PubMed ] [ CrossRef ] [ Google Scholar ]
- Barry C. T., Sidoti C. L., Briggs S. M., Reiter S. R., Lindsey R. A. (2017). Adolescent social media use and mental health from adolescent and parent perspectives. J. Adolesc. 61 1–11. 10.1016/j.adolescence.2017.08.005 [ PubMed ] [ CrossRef ] [ Google Scholar ]
- Bell V., Bishop D. V., Przybylski A. K. (2015). The debate over digital technology and young people. BMJ 351 : h3064 . 10.1136/bmj.h3064 [ PubMed ] [ CrossRef ] [ Google Scholar ]
- Best P., Manktelow R., Taylor B. (2014). Online communication, social media and adolescent wellbeing: a systematic narrative review. Child. Youth Serv. Rev. 41 27–36. 10.1016/j.childyouth.2014.03.001 [ CrossRef ] [ Google Scholar ]
- Best P., Taylor B., Manktelow R. (2015). I’ve 500 friends, but who are my mates? Investigating the influence of online friend networks on adolescent wellbeing. J. Public Ment. Health 14 135–148. 10.1108/jpmh-05-2014-0022 [ CrossRef ] [ Google Scholar ]
- Birkjær M., Kaats M. (2019). in Er sociale Medier Faktisk en Truss for Unges Trivsel? [Does Social Media Really Pose a Threat to Young People’s Well-Being?] , ed. N.M.H.R. Institute (København: Nordic Co-operation; ). [ Google Scholar ]
- Booker C. L., Kelly Y. J., Sacker A. (2018). Gender differences in the associations between age trends of social media interaction and well-being among 10-15 year olds in the UK. BMC Public Health 18 : 321 . 10.1186/s12889-018-5220-4 [ PMC free article ] [ PubMed ] [ CrossRef ] [ Google Scholar ]
- Bor W., Dean A. J., Najman J., Hayatbakhsh R. (2014). Are child and adolescent mental health problems increasing in the 21st century? A systematic review. Austr. N. Z. J. Psychiatry 48 606–616. 10.1177/0004867414533834 [ PubMed ] [ CrossRef ] [ Google Scholar ]
- Bourgeois A., Bower J., Carroll A. (2014). Social networking and the social and emotional wellbeing of adolescents in Australia. J. Psychol. Counsell. Sch. 24 167–182. 10.1017/jgc.2014.14 [ CrossRef ] [ Google Scholar ]
- Boyd D. (2014). It’s Complicated: The Social Lives of Networked Teens. New Haven, CT: Yale University Press. [ Google Scholar ]
- Boyle S. C., LaBrie J. W., Froidevaux N. M., Witkovic Y. D. (2016). Different digital paths to the keg? How exposure to peers’ alcohol-related social media content influences drinking among male and female first-year college students. Addict. Behav. 57 21–29. 10.1016/j.addbeh.2016.01.011 [ PMC free article ] [ PubMed ] [ CrossRef ] [ Google Scholar ]
- Brunborg G. S., Andreas J. B. (2019). Increase in time spent on social media is associated with modest increase in depression, conduct problems, and episodic heavy drinking. J. Adolesc. 74 201–209. 10.1016/j.adolescence.2019.06.013 [ PubMed ] [ CrossRef ] [ Google Scholar ]
- Brunborg G. S., Andreas J. B., Kvaavik E. (2017). Social media use and episodic heavy drinking among adolescents. Psychol. Rep. 120 475–490. 10.1177/0033294117697090 [ PubMed ] [ CrossRef ] [ Google Scholar ]
- Burnette C. B., Kwitowski M. A., Mazzeo S. E. (2017). “I don’t need people to tell me I’m pretty on social media:” A qualitative study of social media and body image in early adolescent girls. Body Image 23 114–125. 10.1016/j.bodyim.2017.09.001 [ PubMed ] [ CrossRef ] [ Google Scholar ]
- Childnet International (2018). Age Restrictions on Social Media Services. Available online at: https://www.childnet.com/blog/age-restrictions-on-social-media-services (accessed September 30, 2019). [ Google Scholar ]
- Colder Carras M., Van Rooij A. J., Van de Mheen D., Musci R., Xue Q., Mendelson T. (2017). Video gaming in a hyperconnected world: a cross-sectional study of heavy gaming, problematic gaming symptoms, and online socializing in adolescents. Comput. Hum. Bahav. 68 472–479. 10.1016/j.chb.2016.11.060 [ PMC free article ] [ PubMed ] [ CrossRef ] [ Google Scholar ]
- Coyne S. M., Rogers A. A., Zurcher J. D., Stockdale L., Booth M. (2020). Does time spent using social media impact mental health?: an eight year longitudinal study. Comput. Hum. Behav. 104 : 106160 10.1016/j.chb.2019.106160 [ CrossRef ] [ Google Scholar ]
- Critchlow N., MacKintosh A. M., Hooper L., Thomas C., Vohra J. (2019). Participation with alcohol marketing and user-created promotion on social media, and the association with higher-risk alcohol consumption and brand identification among adolescents in the UK. Addict. Res. Theory 27 515–526. 10.1080/16066359.2019.1567715 [ PMC free article ] [ PubMed ] [ CrossRef ] [ Google Scholar ]
- Cross D., Lester L., Barnes A. (2015). A longitudinal study of the social and emotional predictors and consequences of cyber and traditional bullying victimisation. Int. J. Public Health 60 207–217. 10.1007/s00038-015-0655-1 [ PubMed ] [ CrossRef ] [ Google Scholar ]
- Curtis B. L., Lookatch S. J., Ramo D. E., McKay J. R., Feinn R. S., Kranzler H. R. (2018). Meta-analysis of the association of alcohol-related social media use with alcohol consumption and alcohol-related problems in adolescents and young adults. Alcohol. Clin. Exp. Res. 42 978–986. 10.1111/acer.13642 [ PMC free article ] [ PubMed ] [ CrossRef ] [ Google Scholar ]
- de Lenne O., Vandenbosch L., Eggermont S., Karsay K., Trekels J. (2018). Picture-perfect lives on social media: a cross-national study on the role of media ideals in adolescent well-being. Med. Psychol. 23 52–78. 10.1080/15213269.2018.1554494 [ CrossRef ] [ Google Scholar ]
- de Vries D. A., Peter J., de Graaf H., Nikken P. (2016). Adolescents’ social network site use, peer appearance-related feedback, and body dissatisfaction: testing a mediation model. J. Youth Adolesc. 45 211–224. 10.1007/s10964-015-0266-4 [ PMC free article ] [ PubMed ] [ CrossRef ] [ Google Scholar ]
- Dickson K., Richardson M., Kwan I., MacDowall W., Burchett H., Stansfield C., et al. (2018). Screen-Based Activities and Children and Young People’s Mental Health: A Systematic Map of Reviews. London: University College London. [ PMC free article ] [ PubMed ] [ Google Scholar ]
- Erfani S. S., Abedin B. (2018). Impacts of the use of social network sites on users’ psychological well-being: a systematic review. J. Assoc. Inform. Sci. Technol. 69 900–912. 10.1002/asi.24015 [ CrossRef ] [ Google Scholar ]
- Erreygers S., Vandebosch H., Vranjes I., Baillien E., De Witte H. (2018). Feel good, do good online? Spillover and crossover effects of happiness on adolescents’ online prosocial behavior. Happiness Stud. 20 1241–1258. 10.1007/s10902-018-0003-2 [ CrossRef ] [ Google Scholar ]
- Espinoza G., Juvonen J. (2011). The pervasiveness, connectedness, and intrusiveness of social network site use among young adolescents. Cyberpsychol. Behav. Soc. Netw. 14 705–709. 10.1089/cyber.2010.0492 [ PubMed ] [ CrossRef ] [ Google Scholar ]
- Fahy A. E., Stansfield S. A., Smuk M., Smith N. R., Cummins S., Clark C. (2016). Longitudinal associations between cyberbullying involvement and adolescent mental health. J. Adolesc. Health 59 502–509. 10.1016/j.jadohealth.2016.06.006 [ PubMed ] [ CrossRef ] [ Google Scholar ]
- Ferguson C. J., Munoz M. E., Garza A., Galindo M. (2014). Concurrent and prospective analyses of peer, television and social media influences on body dissatisfaction, eating disorder symptoms and life satisfaction in adolescent girls. J. Youth Adolesc. 43 1–14. 10.1007/s10964-012-9898-9 [ PubMed ] [ CrossRef ] [ Google Scholar ]
- Fisher B. W., Gardella J. H., Teurbe-Tolon A. R. (2016). Peer Cybervictimization among adolescents and the associated internalizing and externalizing problems: a meta-analysis. J. Youth Adolesc. 45 1727–1743. 10.1007/s10964-016-0541-z [ PubMed ] [ CrossRef ] [ Google Scholar ]
- Foerster M., Roosli M. (2017). A latent class analysis on adolescents media use and associations with health related quality of life. Comput. Huma. Bahav. 71 266–274. 10.1016/j.chb.2017.02.015 [ CrossRef ] [ Google Scholar ]
- Foody M., Samara M., O’Higgins Norman J. (2017). Bullying and cyberbullying studies in the school-aged population on the island of Ireland: a meta-analysis. Br. J. Educ. Psychol. 87 535–557. 10.1111/bjep.12163 [ PubMed ] [ CrossRef ] [ Google Scholar ]
- Fredrick S. S., Demaray M. K. (2018). Peer victimization and suicidal ideation: the role of gender and depression in a school. Based sample. J. Sch. Psychol. 67 1–15. 10.1016/j.jsp.2018.02.001 [ PubMed ] [ CrossRef ] [ Google Scholar ]
- Frison E., Eggermont S. (2016). Exploring the relationships between different types of Facebook use, perceived online social support, and adolescents’ depressed mood. Soc. Sci. Comput. Rev. 34 153–171. 10.1177/0894439314567449 [ CrossRef ] [ Google Scholar ]
- Frison E., Eggermont S. (2017). Browsing, posting, and liking on instagram: the reciprocal relationships between different types of instagram use and adolescents’. Depressed Mood. 20 603–609. 10.1089/cyber.2017.0156 [ PubMed ] [ CrossRef ] [ Google Scholar ]
- Geusens F., Beullens K. (2017). The reciprocal associations between sharing alcohol references on social networking sites and binge drinking: a longitudinal study among late adolescents. Comput. Hum. Behav. 73 499–506. 10.1016/j.chb.2017.03.062 [ CrossRef ] [ Google Scholar ]
- Geusens F., Beullens K. (2018). The association between social networking sites and alcohol abuse among Belgian adolescents: the role of attitudes and social norms. J. Media Psychol. 30 207–216. 10.1027/1864-1105/a000196 [ CrossRef ] [ Google Scholar ]
- Griffiths M. D., Kuss D. J., Demetrovics Z. (2014). “ Chapter 6 - social networking addiction: an overview of preliminary findings ,” in Behavioral Addictions , eds Rosenberg K. P., Feder L. C. (San Diego: Academic Press), 119–141. [ Google Scholar ]
- Hamm M. P., Newton A. S., Chisholm A., Shulhan J., Milne A., Sundar P., et al. (2015). Prevalence and effect of cyberbullying on children and young people: a scoping review of social media studies. JAMA Pediatr. 169 770–777. [ PubMed ] [ Google Scholar ]
- Hanprathet N., Manwong M., Khumsri J., Yingyeun R., Phanasathit M. (2015). Facebook addiction and its relationship with mental health among thai high school students. J. Med. Assoc. Thailand 98(Suppl. 3) S81–S90. [ PubMed ] [ Google Scholar ]
- Harbard E., Allen N. B., Trinder J., Bei B. (2016). What’s keeping teenagers up? prebedtime behaviors and actigraphy-assessed sleep over school and vacation. J. Adolesc. Health 58 426–432. 10.1016/j.jadohealth.2015.12.011 [ PubMed ] [ CrossRef ] [ Google Scholar ]
- Hase C. N., Goldberg S. B., Smith D., Stuck A., Campain J. (2015). Impacts of traditional bullying and cyberbullying on the mental health of middle school and high school students. Psychol. Sch. 52 607–617. 10.1002/pits.21841 [ CrossRef ] [ Google Scholar ]
- Holfeld B., Mishna F. (2019). , Internalizing symptoms and externalizing problems: risk factors for or consequences of cyber victimization? J. Youth Adolesc. 48 567–580. 10.1007/s10964-018-0974-7 [ PubMed ] [ CrossRef ] [ Google Scholar ]
- Houghton S., Lawrence D., Hunter S. C., Rosenberg M., Zadow C., Wood L., et al. (2018). Reciprocal relationships between trajectories of depressive symptoms and screen media use during adolescence. Youth Adolesc. 47 2453–2467. 10.1007/s10964-018-0901-y [ PMC free article ] [ PubMed ] [ CrossRef ] [ Google Scholar ]
- Jafarpour S., Jadidi H., Almadani S. A. H. (2017). Comparing personality traits, mental health and self-esteem in users and non-users of social networks. Razavi Int. J. Med. 5 :e61401. 10.5812/rijm.61401 [ CrossRef ] [ Google Scholar ]
- John A., Glendenning A. C., Marchant A., Montgomery P., Stewart A., Wood S., et al. (2018). Self-harm, suicidal behaviours, and cyberbullying in children and young people: systematic review. J. Med. Int. Res. 20 : e129 . 10.2196/jmir.9044 [ PMC free article ] [ PubMed ] [ CrossRef ] [ Google Scholar ]
- Kaplan A. M., Haenlein M. (2010). Users of the world, unite! The challenges and opportunities of social media. Bus. Horiz. 53 59–68. 10.1016/j.bushor.2009.09.003 [ CrossRef ] [ Google Scholar ]
- Keles B., McCrae N., Grealish A. (2020). A systematic review: the influence of social media on depression, anxiety and psychological distress in adolescents. Int. J. Adolesc. Youth 25 79–93. 10.1080/02673843.2019.1590851 [ CrossRef ] [ Google Scholar ]
- Kietzmann J. H., Hermkens K., McCarthy I. P., Silvestre B. S. (2011). Social media? Get serious! Understanding the functional building blocks of social media. Bus. Horiz. 54 241–251. 10.1016/j.bushor.2011.01.005 [ CrossRef ] [ Google Scholar ]
- Kim H. H.-S. (2017). The impact of online social networking on adolescent psychological well-being (WB): a population-level analysis of Korean school-aged children. Int. J. Adolesc. Youth 22 364–376. 10.1080/02673843.2016.1197135 [ CrossRef ] [ Google Scholar ]
- Kim S., Kimber M., Boyle M. H., Georgiades K. (2019). Sex differences in the association between cyberbullying victimization and mental health. Subst. Suicid. Ideation Adolesc. 64 126–135. 10.1177/0706743718777397 [ PMC free article ] [ PubMed ] [ CrossRef ] [ Google Scholar ]
- Kobayashi T., Boase J. (2012). No Such Effect? The implications of measurement error in self-report measures of mobile communication use. Commun. Methods Meas. 6 126–143. 10.1080/19312458.2012.679243 [ CrossRef ] [ Google Scholar ]
- Koo H. J., Woo S., Yang E., Kwon J. H. (2015). The double meaning of online social space: three-way interactions among social anxiety, online social behavior, and offline social behavior. Cyberpsychol. Behav. Soc. Netw. 18 514–520. 10.1089/cyber.2014.0396 [ PubMed ] [ CrossRef ] [ Google Scholar ]
- Kraut R., Patterson M., Lundmark V., Kiesler S., Mukophadhyay T., Scherlis W. (1998). Internet paradox: a social technology that reduces social involvement and psychological well-being? Am. Psychol. 53 1017 . 10.1037/0003-066x.53.9.1017 [ PubMed ] [ CrossRef ] [ Google Scholar ]
- Krumpal I. (2013). Determinants of social desirability bias in sensitive surveys: a literature review. Qua. Quant. 47 2025–2047. 10.1007/s11135-011-9640-9 [ CrossRef ] [ Google Scholar ]
- Lai H.-M., Hsieh P.-J., Zhang R.-C. (2018). Understanding adolescent students’ use of facebook and their subjective wellbeing: a gender-based comparison. Behav. Inform. Technol. 38 533–548. 10.1080/0144929x.2018.1543452 [ CrossRef ] [ Google Scholar ]
- Lang D., Chien G. (2018). “wordcloud2”: a fast visualization tool for creating wordclouds by using “wordcloud2.js”. R Package Version 0.2.1. Available online at: https://cran.r-project.org/web/packages/wordcloud2/index.html [ Google Scholar ]
- Larm P., Aslund C., Nilsson K. W. (2017). The role of online social network chatting for alcohol use in adolescence: testing three peer-related pathways in a Swedish population-based sample. Comput. Hum. Behav. 71 284–290. 10.1016/j.chb.2017.02.012 [ CrossRef ] [ Google Scholar ]
- Larm P., Raninen J., Åslund C., Svensson J., Nilsson K. W. (2019). The increased trend of non-drinking alcohol among adolescents: what role do internet activities have? Eur. J. Public Health 29 27–32. 10.1093/eurpub/cky168 [ PMC free article ] [ PubMed ] [ CrossRef ] [ Google Scholar ]
- Marchant A., Hawton K., Stewart A., Montgomery P., Singaravelu V., Lloyd K., et al. (2017). A systematic review of the relationship between internet use, self-harm and suicidal behaviour in young people: the good, the bad and the unknown. PLoS One 12 : e0181722 . 10.1371/journal.pone.0181722 [ PMC free article ] [ PubMed ] [ CrossRef ] [ Google Scholar ]
- Marengo D., Longobardi C., Fabris M. A., Settanni M. (2018). Highly-visual social media and internalizing symptoms in adolescence: the mediating role of body image concerns. Comput. Hum. Behav. 82 63–69. 10.1016/j.chb.2018.01.003 [ CrossRef ] [ Google Scholar ]
- Marques T. P., Marques-Pinto A., Alvarez M. J., Pereira C. R. (2018). Facebook: risks and opportunities in brazilian and portuguese youths with different levels of psychosocial adjustment. Spanish J. Psychol. 21 : E31 . [ PubMed ] [ Google Scholar ]
- Mediakix (2019). 20 Tiktok Statistics Marketers Need To Know: Tiktok Demographics & Key Data. 2019. Available online at: https://mediakix.com/blog/top-tik-tok-statistics-demographics/ (accessed February 20, 2020). [ Google Scholar ]
- Meier E. P., Gray J. (2014). Facebook photo activity associated with body image disturbance in adolescent girls. Cyberpsychol. Behav. Soc. Netw. 17 199–206. 10.1089/cyber.2013.0305 [ PubMed ] [ CrossRef ] [ Google Scholar ]
- Memon A. M., Sharma S. G., Mohite S. S., Jain S. (2018). The role of online social networking on deliberate self-harm and suicidality in adolescents: a systematized review of literature. Indian J. Psychiatry 60 384–392. [ PMC free article ] [ PubMed ] [ Google Scholar ]
- Merelle S. Y. M., Kleiboer A., Schotanus M., Cluitmans T. L. M., Waardenburg C. M., Kramer D., et al. (2017). Which health-related problems are associated with problematic video-gaming or social media use in adolescents? A large-scale cross-sectional study. Clin. Neuropsych. 14 11–19. [ Google Scholar ]
- Mueller M. (2019). Challenging the Social Media Moral Panic: Preserving Free Expression under Hypertransparency. Washington, DC: Cato Institute Policy Analysis. [ Google Scholar ]
- Naab T. K., Karnowski V., Schlütz D. (2019). Reporting mobile social media use: how survey and experience sampling measures differ. Commun. Methods Meas. 13 126–147. 10.1080/19312458.2018.1555799 [ CrossRef ] [ Google Scholar ]
- Neira C. J., Barber B. L. (2014). Social networking site use: linked to adolescents’ social self-concept, self-esteem, and depressed mood. Austr. J. Psychol. 66 56–64. 10.1111/ajpy.12034 [ CrossRef ] [ Google Scholar ]
- Nesi J., Miller A. B., Prinstein M. J. (2017a). Adolescents’ depressive symptoms and subsequent technology-based interpersonal behaviors: a multi-wave study. J. Appl. Dev. Psychol. 51 12–19. 10.1016/j.appdev.2017.02.002 [ PMC free article ] [ PubMed ] [ CrossRef ] [ Google Scholar ]
- Nesi J., Rothenberg W. A., Hussong A. M., Jackson K. M. (2017b). Friends’ alcohol-related social networking site activity predicts escalations in adolescent drinking: mediation by peer norms. J. Adolesc. Health 60 641–647. 10.1016/j.jadohealth.2017.01.009 [ PMC free article ] [ PubMed ] [ CrossRef ] [ Google Scholar ]
- Niu G. F., Luo Y. J., Sun X. J., Zhou Z. K., Yu F., Yang S. L., et al. (2018). Qzone use and depression among Chinese adolescents: a moderated mediation model. J. Affect. Disord. 231 58–62. 10.1016/j.jad.2018.01.013 [ PubMed ] [ CrossRef ] [ Google Scholar ]
- Nursalam N., Octavia M., Tristiana R. D., Efendi F. (2018). Association between insomnia and social network site use in Indonesian adolescents. Nurs. Forum 54 149–156. 10.1111/nuf.12308 [ PubMed ] [ CrossRef ] [ Google Scholar ]
- Oberst U., Wegmann E., Stodt B., Brand M., Chamarro A. (2017). Negative consequences from heavy social networking in adolescents: the mediating role of fear of missing out. J. Adolesc. 55 51–60. 10.1016/j.adolescence.2016.12.008 [ PubMed ] [ CrossRef ] [ Google Scholar ]
- O’Connor R. C., Rasmussen S., Hawton K. (2014). Adolescent self-harm: a school-based study in Northern Ireland. J. Affect. Disord. 159 46–52. 10.1016/j.jad.2014.02.015 [ PubMed ] [ CrossRef ] [ Google Scholar ]
- Ofcom (2018). Children and Parents: Media Use and Attitudes Report. Warrington: Ofcom. [ Google Scholar ]
- Olfson M., Druss B. G., Marcus S. C. (2015). Trends in mental health care among children and adolescents. N. Engl. J. Med. 372 2029–2038. 10.1056/nejmsa1413512 [ PubMed ] [ CrossRef ] [ Google Scholar ]
- Orben A. (2020). Teenagers, screens and social media: a narrative review of reviews and key studies. J. Soc. Psychiatry Psychiatr. Epidemiol. 55 407–414. 10.1007/s00127-019-01825-4 [ PubMed ] [ CrossRef ] [ Google Scholar ]
- Orben A., Dienlin T., Przybylski A. K. (2019). Social media’s enduring effect on adolescent life satisfaction. Pro. Natl. Acad. Sci. U.S.A. 116 10226–10228. 10.1073/pnas.1902058116 [ PMC free article ] [ PubMed ] [ CrossRef ] [ Google Scholar ]
- Orben A., Przybylski A. K. (2019). The association between adolescent well-being and digital technology use. Nat. Hum. Behav. 3 173–182. 10.1038/s41562-018-0506-1 [ PubMed ] [ CrossRef ] [ Google Scholar ]
- O’Reilly M., Dogra N., Whiteman N., Hughes J., Eruyar S., Reilly P. (2018). Is social media bad for mental health and wellbeing? Exploring the perspectives of adolescents. Clin. Chld Psychol. Psychiatry 23 601–613. 10.1177/1359104518775154 [ PubMed ] [ CrossRef ] [ Google Scholar ]
- Peters M., Godfrey C., McInerney P. (2017). “ Chapter 11: scoping reviews ,” in Joanna Briggs Institute Reviewer’s Manual , eds Aromataris E., Munn Z. (Adelaide: The Joanna Briggs Institute; ). [ Google Scholar ]
- Peters M. D., Godfrey C., Khalil H., McInerney P., Parker D., Soares C. B. (2015). Guidance for conducting systematic scoping reviews. Int. J. Evi. -Based Healthc. 13 141–146. 10.1097/xeb.0000000000000050 [ PubMed ] [ CrossRef ] [ Google Scholar ]
- Przybylski A. K., Bowes L. (2017). Cyberbullying and adolescent well-being in England: a population-based cross-sectional study. Lancet Child Adolesc. Health 1 19–26. 10.1016/s2352-4642(17)30011-1 [ PubMed ] [ CrossRef ] [ Google Scholar ]
- Przybylski A. K., Weinstein N. (2017). A large-scale test of the goldilocks hypothesis. Psychol. Sci. 28 204–215. 10.1177/0956797616678438 [ PubMed ] [ CrossRef ] [ Google Scholar ]
- R Core Team (2014). R: A Language and Environment for Statistical Computing. Vienna: R Foundation for Statistical Computing. [ Google Scholar ]
- Richards D., Caldwell P. H., Go H. (2015). Impact of social media on the health of children and young people. J. Paediatr. Child Health 51 1152–1157. 10.1111/jpc.13023 [ PubMed ] [ CrossRef ] [ Google Scholar ]
- Rousseau A., Eggermont S., Frison E. (2017). The reciprocal and indirect relationships between passive Facebook use, comparison on Facebook, and adolescents’ body dissatisfaction. Comput. Hum. Behav. 73 336–344. 10.1016/j.chb.2017.03.056 [ CrossRef ] [ Google Scholar ]
- Salmela-Aro K., Upadyaya K., Hakkarainen K., Lonka K., Alho K. (2017). The dark side of internet use: two longitudinal studies of excessive internet use. depressive symptoms, school burnout and engagement among finnish early and late adolescents. J. Youth Adolesc. 46 343–357. 10.1007/s10964-016-0494-2 [ PubMed ] [ CrossRef ] [ Google Scholar ]
- Sampasa-Kanyinga H., Chaput J. P. (2016). Use of social networking sites and alcohol consumption among adolescents. Public Health 139 88–95. 10.1016/j.puhe.2016.05.005 [ PubMed ] [ CrossRef ] [ Google Scholar ]
- Sampasa-Kanyinga H., Hamilton H. A., Chaput J. P. (2018). Use of social media is associated with short sleep duration in a dose-response manner in students aged 11 to 20 years. Acta Paediatr. 107 694–700. 10.1111/apa.14210 [ PubMed ] [ CrossRef ] [ Google Scholar ]
- Sampasa-Kanyinga H., Lewis R. F. (2015). Frequent use of social networking sites is associated with poor psychological functioning among children and adolescents. Cyberpsychol. Behav. Soc. Netw. 18 380–385. 10.1089/cyber.2015.0055 [ PubMed ] [ CrossRef ] [ Google Scholar ]
- Scharkow M. (2016). The accuracy of self-reported internet Use—A validation study using client log data. Commun. Methods Meas. 10 13–27. 10.1080/19312458.2015.1118446 [ CrossRef ] [ Google Scholar ]
- Scharkow M. (2019). The reliability and temporal stability of self-reported media exposure: a meta-analysis. Commun. Methods Meas. 13 198–211. 10.1080/19312458.2019.1594742 [ CrossRef ] [ Google Scholar ]
- Schønning V., Aarø L. E., Skogen J. C. (2020). Central themes, core concepts and knowledge gaps concerning social media use, and mental health and well-being among adolescents: a protocol of a scoping review of published literature. BMJ Open 10 : e031105 . 10.1136/bmjopen-2019-031105 [ PMC free article ] [ PubMed ] [ CrossRef ] [ Google Scholar ]
- Scott H., Woods H. C. (2018). Fear of missing out and sleep: cognitive behavioural factors in adolescents’ nighttime social media use. J. Adolesc. 68 61–65. 10.1016/j.adolescence.2018.07.009 [ PubMed ] [ CrossRef ] [ Google Scholar ]
- Seabrook E. M., Kern M. L., Rickard N. S. (2016). Social networking sites, depression, and anxiety: a systematic review. JMIR Ment. Health 3 : e50 . 10.2196/mental.5842 [ PMC free article ] [ PubMed ] [ CrossRef ] [ Google Scholar ]
- Settanni M., Marengo D., Fabris M. A., Longobardi C. (2018). The interplay between ADHD symptoms and time perspective in addictive social media use: a study on adolescent Facebook users. Child. Youth Serv. Rev. 89 165–170. 10.1016/j.childyouth.2018.04.031 [ CrossRef ] [ Google Scholar ]
- Silge J., Robinson D. (2016). tidytext: text mining and analysis using tidy data principles in RJ. Open Source Softw. 1 : 37 10.21105/joss.00037 [ CrossRef ] [ Google Scholar ]
- Spears B. A., Taddeo C. M., Daly A. L., Stretton A., Karklins L. T. (2015). Cyberbullying, help-seeking and mental health in young Australians: implications for public health. Int. J. Public Health 60 219–226. 10.1007/s00038-014-0642-y [ PubMed ] [ CrossRef ] [ Google Scholar ]
- Teesson M., Newton N. C., Slade T., Chapman C., Birrell L., Mewton L., et al. (2020). Combined prevention for substance use, depression, and anxiety in adolescence: a cluster-randomised controlled trial of a digital online intervention. Lancet Digital Health 2 e74–e84. 10.1016/s2589-7500(19)30213-4 [ PubMed ] [ CrossRef ] [ Google Scholar ]
- Throuvala M. A., Griffiths M. D., Rennoldson M., Kuss D. J. (2019). Motivational processes and dysfunctional mechanisms of social media use among adolescents: a qualitative focus group study. Comput. Hum. Behav. 93 164–175. 10.1016/j.chb.2018.12.012 [ CrossRef ] [ Google Scholar ]
- Tiggemann M., Slater A. (2017). Facebook and body image concern in adolescent girls: a prospective study. Int. J. Eat. Disord. 50 80–83. 10.1002/eat.22640 [ PubMed ] [ CrossRef ] [ Google Scholar ]
- Tricco A. C., Lillie E., Zarin W., O’Brien K. K., Colquhoun H., Levac D., et al. (2018). PRISMA extension for scoping reviews (PRISMA-ScR): checklist and explanation. Ann. Int. Med. 169 467–473. [ PubMed ] [ Google Scholar ]
- Tseng F.-Y., Yang H.-J. (2015). Internet use and web communication networks, sources of social support, and forms of suicidal and nonsuicidal self-injury among adolescents: different patterns between genders. Suicide Life Threat. Behav. 45 178–191. 10.1111/sltb.12124 [ PubMed ] [ CrossRef ] [ Google Scholar ]
- Twenge J. M., Campbell W. K. (2019). Media use is linked to lower psychological well-being: evidence from three datasets. Psychiatr. Q. 11 311–331. 10.1007/s11126-019-09630-7 [ PubMed ] [ CrossRef ] [ Google Scholar ]
- Twenge J. M., Joiner T. E., Rogers M. L., Martin G. N. (2018). Increases in depressive symptoms, suicide-related outcomes, and suicide rates among U.S. adolescents after 2010 and links to increased new media screen time. Clin. Psychol. Sci. 6 3–17. 10.1177/2167702617723376 [ CrossRef ] [ Google Scholar ]
- van den Eijnden R., Koning I., Doornwaard S., van Gurp F., ter Bogt T. (2018). The impact of heavy and disordered use of games and social media on adolescents’ psychological, social, and school functioning. J. Behav. Addict. 7 697–706. 10.1556/2006.7.2018.65 [ PMC free article ] [ PubMed ] [ CrossRef ] [ Google Scholar ]
- Verduyn P., Ybarra O., Resibois M., Jonides J., Kross E. (2017). Do social network sites enhance or undermine subjective well-being? a critical review: do social network sites enhance or undermine subjective well-being?. Soc. Issues Policy Rev. 11 274–302. 10.1111/sipr.12033 [ CrossRef ] [ Google Scholar ]
- Wallaroo (2020). TikTok Statistics – Updated February 2020. Available online at: https://wallaroomedia.com/blog/social-media/tiktok-statistics/ (accessed February 20, 2020). [ Google Scholar ]
- Wang P., Wang X., Wu Y., Xie X., Wang X., Zhao F., et al. (2018). Social networking sites addiction and adolescent depression: A moderated mediation model of rumination and self-esteem. Personal. Individ. Differ. 127 162–167. 10.1016/j.paid.2018.02.008 [ CrossRef ] [ Google Scholar ]
- Wartberg L., Kriston L., Thomasius R. (2018). Depressive symptoms in adolescents. Dtsch. Arztebl. Int. 115 549–555. [ PMC free article ] [ PubMed ] [ Google Scholar ]
- Weinstein E. (2018). The social media see-saw: positive and negative influences on adolescents’ affective well-being. New Media Soc. 20 3597–3623. 10.1177/1461444818755634 [ CrossRef ] [ Google Scholar ]
- Wolke D., Lee K., Guy A. (2017). Cyberbullying: a storm in a teacup? Eur. Child Adolesc. Psychiatry 26 899–908. 10.1007/s00787-017-0954-6 [ PMC free article ] [ PubMed ] [ CrossRef ] [ Google Scholar ]
- Woods H. C., Scott H. (2016). #Sleepyteens: social media use in adolescence is associated with poor sleep quality, anxiety, depression and low self-esteem. J. Adolesc. 51 41–49. 10.1016/j.adolescence.2016.05.008 [ PubMed ] [ CrossRef ] [ Google Scholar ]
- Yan H., Zhang R., Oniffrey T. M., Chen G., Wang Y., Wu Y., et al. (2017). Associations among screen time and unhealthy behaviors. academic performance, and well-being in chinese adolescents. Int. J. Envion. Res. Public Heath. 14 : 596 . 10.3390/ijerph14060596 [ PMC free article ] [ PubMed ] [ CrossRef ] [ Google Scholar ]

COMMENTS
This research examined the relations of social media addiction to college students' mental health and academic performance, investigated the role of self-esteem as a mediator for the relations ...
Social media addiction is increasing both in Turkey. and all around the world. This study aims to determine the level of social media addiction in. young people in Turkey, and to make suggestions ...
These research questions will be answered using bibliometric analysis of the literature on social media addiction and problematic use. This will allow for an overview of the research that has been conducted in this area, including information on the most influential authors, journals, countries of publication, and subject areas of study.
Quantitative Research on the Level of Social Media Addiction among Young People EUROPEAN ACADEMIC RESEARCH - Vol. VIII, Issue 8 / November 2020 4697 Khurana N revealed that in India 66% of the youth practices social media for at least 2 hours a day. He also exposed that a very large number of youths have been persecuted by cybercrimes.
Despite the limited research available on the relationship between suicidal ideation and addiction to social media such as Instagram and TikTok, this study aims to examine this connection.
Introduction. Today, social media (SM) (e.g., WhatsApp, Instagram, Facebook, etc.) have enjoyed such rapidly-growing popularity[] that around 2.67 billion users of social networks have been estimated worldwide.[] After China, India, and Indonesia, Iran ranks fourth in terms of using SM, having approximately 40 million active online social network users over the past decade, these networks have ...
Excessive and compulsive use of social media may lead to social media addiction (SMA). The main aim of this study was to investigate whether demographic factors (including age and gender), impulsivity, self-esteem, emotions, and attentional bias were risk factors associated with SMA. The study was conducted in a non-clinical sample of college ...
Researchers have employed a variety of different methods to understand the effect of using social media, defined as platforms that afford personalized profiles for self-presentation, private and public messaging capabilities, articulation of a person's network or social ties, and a stream of frequently updated content (Verduyn et al., 2017).The most prominent methodology for studying social ...
Social media addiction is one of the most burning problems among young adults, especially among college students. The sample of the present research work consisted of 140 college students from which we randomly selected only (35 boys, 35 girls). For this purpose social media addiction scale students form (smas-sf) developed by Cengiz Sahin was ...
link between teenagers' self-control and social media addiction, with the assumption that the more self-control, the less social media addiction (Facebook). On the other hand, the less self-control youngsters possess, the greater their social media addiction (Facebook). Thus, the coefficient of determination (r2) obtained in this study is 0.1758.
social media addiction scales, or general addiction in a population, and theories or models that have been applied in studies of social media addiction. Yet, it appears that 70 these reviews have a limited focus and narrow perspective. They do not cover up-to-date facets of social media addiction among young users. For example, Sun and Zhang
On the article of Dr. Wilson (2015), it states that spending more than one hour daily at social media sites is a sign of social media addiction. Pew Research asked users of each platform how often they engage with the site, whether on a daily, weekly or less frequent basis. Facebook users are highly engaged with the platform.
The excessive or addictive use of social media defined as 'a behavioral addiction that is characterized as being overly concerned about social media, driven by an uncontrollable urge to log on to or use social media, and devoting so much time and effort to social media that impair other important life areas'(Hilliard, Citation 2019).
A systematic review by Khan and Khan (20) has pointed out that social media addiction has a negative impact on users' mental health. For example, social media addiction can lead to stress levels rise, loneliness, and sadness (54). Anxiety is another common mental health problem associated with social media addiction.
The purpose of this study is to determine the relationship between self-control and teenage social. media addiction (Facebook). This study employs a quantitative approach and purposively samples ...
Terms, such as social media addiction, problematic social media use, and compulsive social media use, are used interchangeably to refer to the phenomenon of maladaptive social media use characterized by either addiction-like symptoms and/or reduced self-regulation (Bányai et al., 2017, Casale et al., 2018, Klobas et al., 2018, Marino et al., 2018, Tarafdar et al., 2020).
social media addiction and their encounters with feelings of loneliness. The research included a sample size of 172 individuals, encompassing both males and females, and using known ... loneliness, students, quantitative research, young adults he integration of social media platforms into everyday life has greatly transformed the dynamics of ...
Introduction. Nearly 90% of young adults ranging from 18-29 years old now use social media - a substantial 78% percent increase from just a little over a decade ago [1]. Additionally, those who have attended at least some college are more likely to use social media than those less educated (e.g., high school diploma or no diploma) [1 ...
This study adopts visualized bibliometrics to offer comprehensive insights into the relationship between SMA and students' academic performance, as reflected in research journals. Social media addiction (SMA) has become a significant concern affecting students in higher education institutions, with both positive and negative implications. As a result, scholars and practitioners have directed ...
The relationship between social media addiction and depression: a quantitative study among university students in Khost, Afghanistan Rahmatullah Haand a,b and Zhao Shuwanga aSchool of Journalism and Communication, University of Hebei, Baoding, China; bSchool of Journalism and Public Relation, Shaikh Zayed University, Khost, Afghanistan
reflected in academic performance and social capital. In short, social media addiction is an ever-increasing problem in the 21st century. For this reason, a number of studies were conducted in various countries on this subject. Each study presents a new outcome, explains reasons and effects of the social media addiction, and presents new ways
This research examined the relations of social media addiction to college students' mental health and academic performance, investigated the role of self-esteem as a mediator for the relations, and further tested the effectiveness of an intervention in reducing social media addiction and its potential adverse outcomes. In Study 1, we used a survey method with a sample of college students (N ...
Social media addiction is a behavioral dependency characterized by excessive and compulsive use of social media platforms, leading to negative impacts on various aspects of an individual's life. Bibliometric analysis is a research method used to quantitatively analyze academic literature, such as articles, books, and conference papers. It involves the application of statistical and ...
Introduction: Social media has become an integrated part of daily life, with an estimated 3 billion social media users worldwide. Adolescents and young adults are the most active users of social media. Research on social media has grown rapidly, with the potential association of social media use and mental health and well-being becoming a polarized and much-studied subject.